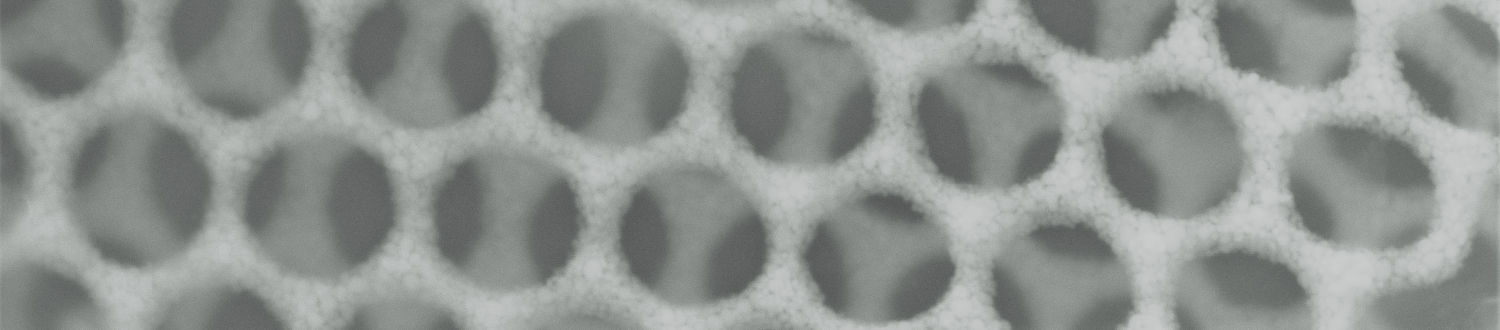
Medical physics
The medical physics team researches techniques to improve diagnosis and treatment using ionising radiation. On the diagnostic side, we work at the interface between instrumentation optimisation, in collaboration with the Detector Development Group at Rutherford Appleton Laboratories, and data processing.
We are investigating, in particular, the use of pixellated spectroscopic detectors coupled with AI for more accurate diagnosis of breast cancer and measurement of breast density as an indicator of cancer risk. On the treatment side, we are looking into improving the accuracy of small-field dosimetry and understanding the mechanisms of cell damage and repair for irradiation with different beam qualities and rates.
Research leads
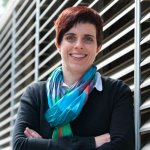
Dr Silvia Pani
Senior Lecturer in Applied Radiation Physics
Overview
Ionising radiation plays an essential role in both diagnosis and treatment. In diagnosis, it is essential to maximise the amount of information obtained from an image whilst minimising the radiation dose deposited in the patient. We are doing this by using novel X-ray detectors, which produce, for every image pixel, the full distribution of wavelength of the radiation detected – this is comparable to the increased amount of information available in colour vs black and white photography. When combined with AI, this allows more accurate identification of details or prediction of disease.
A fundamental aim of treatment with ionising radiation is to understand how different cancer cells react to different modes of dose delivery, and to accurately measure small variations in the dose distribution from a treatment beam in order to maximise the damage to cancer cells whilst sparing healthy tissue.
In collaboration with the National Physical Laboratory, we are working on both these aspects in order to improve the efficacy of radiotherapy alongside better long-term outcomes for patients.
Pixellated spectroscopic detectors
Pixellated spectroscopic detectors represent a step-change in X-ray and gamma-ray imaging, providing both spatial and spectral information. However, their use is not without challenges: corrections are needed for counting rate, and to account for charge deposition across different pixels. Moreover, the typical pixel size is larger than that of non-spectroscopic detectors, particularly for specific applications requiring high spatial resolution, such as mammography; therefore, algorithms need to be developed to better localise the position where a photon has hit the detector.
This project looks at optimising the information available from the detector for improved spatial resolution and quantitative imaging, through a combination of Monte Carlo and analytical models (e.g., http://doi.org/10.1109/TNS.2023.3269888) and AI-based algorithms.
Quantitative imaging using spectroscopic detectors
The spectral information available from a spectroscopic detector allows quantitative imaging with an accuracy rarely possible with conventional detectors. The possibility of identifying the beam components more useful for each imaging application also allows improvement of the dose/image quality trade-off. This project will look at quantitative imaging with a specific focus on the dosimetric aspects, and on how AI may help optimising the dose/image quality trade-off. We have been working on the calculation of breast density as an indicator of cancer risk, but similar approaches may be used on other imaging modalities.