11am - 12 noon
Friday 3 November 2023
Photo-realistic sign language production
PhD Viva Open Presentation by Ben Saunders.
All welcome!
Free
University of Surrey
Guildford
Surrey
GU2 7XH
This event has passed
Abstract
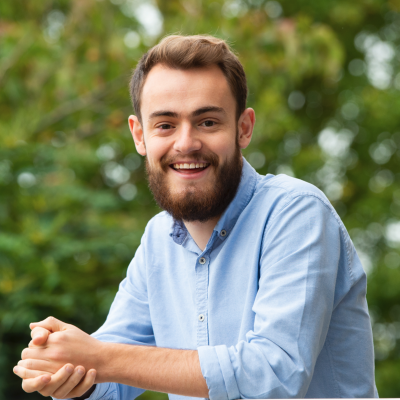
Most computational sign language research focuses on the recognition and translation of sign languages to spoken languages. Although useful, this technology is more applicable to a hearing person understanding a Deaf person, and often not that helpful for the Deaf community.
The opposite task of Sign Language Production (SLP), the translation of spoken language sentences into sign language videos, is far more relevant to the Deaf and could significantly increase the availability of sign language content. Traditional SLP research focused on avatar-based techniques that generated cartoon and robotic sign outputs, with a reliance on simple phrase lookup and expensive MoCap technology.
Recently, there has been an increase in deep learning approaches to SLP. However, these works often focus on the production of isolated signs without realistic transitions and a skeleton pose representation, resulting in robotic and non-realistic animations that are poorly received by the Deaf.
In this thesis, we improve the capability of SLP technology, focusing on the production of photo-realistic continuous sign language videos direct from spoken language text. We first present a baseline approach using concatenated isolated signs, and propose a novel ’back translation’ evaluation metric used for the rest of the thesis.
In our first contribution chapter, we present the first continuous SLP model to translate from spoken language sentences to continuous sign language sequences in an end-to-end manner. We introduce a Progressive Transformer architecture that uses an alternative formulation of transformer decoding for continuous sequences. We propose both adversarial training and Mixture Density Network (MDN) modelling to tackle under-articulated outputs, and show improved model performance through both quantitative back translation results and qualitative Deaf user studies.
Building on the feedback to our continuous SLP approach, we next attempt to combine the benefits of both continuous and isolated production. In our second contribution, we separate the SLP task into two distinct but jointly-trained sub-tasks of translation and animation, with an intermediary gloss supervision. We propose a Mixture of Motion Primitives (M O MP) architecture that learns to combine specialised skeleton motion primitives to produce novel sequences and reduces the adverse effect of ’regression to the mean’.
Although considerable progress towards continuous SLP was made in earlier contributions, Deaf feedback shows the produced sequences still under-articulate hand motion compared to baseline isolated methods. Our third contribution proposes a learnt co-articulation between isolated signs to better reflect continuous signing whilst still maintaining the inherent understandable nature seen in dictionary signs.
We propose a novel Frame Selection Network to learn the optimal subset of frames that best maps to a continuous signing sequence. We conduct extensive deaf user evaluation to show that this approach improves the natural signing motion of concatenated isolated sequences and is overwhelmingly preferred to both previous contributions and baseline models.
Previous contributions use an output skeleton pose representation, which has been shown to be a major factor in the lack of comprehension. In our final contribution, we introduce SignGAN, the first SLP model to produce photo-realistic sign language videos to a level understandable by native Deaf signers. We use skeleton pose sequences to condition a video-to-video synthesis model with a novel keypoint-based loss to improve hand synthesis quality and a style conditioning to generate novel human appearances.
Finally, we conduct a Deaf user evaluation to show that SignGAN photo-realistic outputs are more understandable than skeleton pose sequences.
Given the contributions above, this thesis proposes an end-to-end pipeline to produce photo-realistic sign language sequences from spoken language sentences. As we have shown a potential pipeline to produce large-scale unconstrained translation, we suggest future work focuses on the currently under-developed Text to Gloss translation step alongside an automated collection of the large-scale datasets this task requires.
Featured Academics
Visitor information
Find out how to get to the University, make your way around campus and see what you can do when you get here.