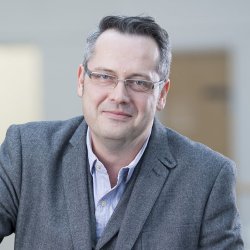
Professor Richard Bowden
Academic and research departments
Centre for Vision, Speech and Signal Processing (CVSSP), School of Computer Science and Electronic Engineering.About
Biography
Richard Bowden is Professor of Computer Vision and Machine Learning at the University of Surrey where he leads the Cognitive Vision Group within CVSSP and is Associate Dean for postgraduate research within his faculty. His research centres on the use of computer vision to locate, track, understand and learn from humans. He has held over 40 research grants from UK, EU funding bodies as well as industrial funded projects. These projects cover areas such as cognitive robotics and vision, sign and gesture recognition, lip-reading and nonverbal communication as well as many fundamental topics to computer vision such as tracking and detection. His research has been recognised by prizes, plenary talks and media/press coverage including the Sullivan thesis prize in 2000 and many best paper awards.
To date, he has published over 200 peer reviewed publications and has served as either program committee member or area chair for ICCV, CVPR, ECCV, BMVA, FG and ICPR in addition to numerous international workshops and conferences. He is an Associate Editor for the journals Image and Vision Computing and IEEE Trans Pattern Analysis and Machine Intelligence (the top journal in his field). He was awarded a Royal Society Leverhulme Trust Senior Research Fellowship in 2013 and is a member of the RS international exchanges committee. He was a member of the British Machine Vision Association (BMVA) executive committee and a company director for seven years. He is a member of the BMVA, a senior member of the IEEE and a Fellow of the Higher Education Academy. He was awarded a prestigious Fellowship of the International Association of Pattern Recognition in 2016.
Areas of specialism
University roles and responsibilities
- Professor of Computer Vision and Machine Learning
My qualifications
Previous roles
Affiliations and memberships
News
ResearchResearch interests
Richard's research focuses on the use of computer vision to locate, track and understand humans, with specific examples in Sign and Gesture recognition, Activity and Action recognition, lip-reading and facial feature tracking but also in HCI and cognitive robotics and machine perception where machines learn to understand and predict humans.
His research into tracking and artificial life received worldwide media coverage, appearing at the British Science Museum and the Minnesota Science Museum.
Research projects
The goal of the proposed project SMILE is to pioneer an assessment system for Swiss German Sign Language (Deutschschweizerische Gebärdensprache, DSGS) using automatic sign language recognition technology.
The project tackled the subject of automatically learn to recognise dynamic activity in broadcast footage with demonstration activities in both Sign Language and more general actions and activity
Improving investigative capability through the application of science and technology
Dicta-Sign aimed to enable communication between Deaf individuals by promoting the development of natural human computer interfaces (HCI) for Deaf users. It researched and develop recognition and synthesis systems for sign languages (SLs) at a level of detail necessary for recognising and generating authentic signing. Research outcomes were integrated into three prototypes (a Search-by-Example tool, a SL-to-SL translator and a sign-Wiki).
LiLiR looked at Language Independent Lip Reading from video
The DIPLECS project aimed to design an Artificial Cognitive System capable of learning and adapting to respond to everyday situations humans take for granted. The primary demonstration of its capability was providing assistance and advice to the driver of a car. The system learnt by watching humans, how they act and react while driving, building models of their behaviour and predicting what a driver would do when presented with a specific driving scenario.
In the COSPAL architecture we combined techniques from the field of artificial intelligence (AI) for symbolic reasoning and learning of artificial neural networks (ANN) for association of percepts and states. We establish feedback loops through the continuous and the symbolic parts of the system, which allow perception-action feedback at several levels in the system. After an initial bootstrapping phase, incremental learning techniques were used to train the system, allowing adaptation and exploration.
The central goal is to build a vision system that can be used in a wider variety of fields and that is re-usable by introducing self-adaptation at the level of perception, by providing categorisation capabilities, and by making explicit the knowledge base at the level of reasoning, and thereby enabling the knowledge base to be changed. In order to make these ideas concrete CogViSys aims at developing a virtual commentator which is able to translate visual information into a textual description.
Indicators of esteem
Awarded Fellow of the International Association of Pattern Recognition in 2016
Member of Royal Society’s International Exchanges Committee 2016
Royal Society Leverhulme Trust Senior Research Fellowship
Sullivan thesis prize in 2000
Executive Committee member and theme leader for EPSRC ViiHM Network 2015
TIGA Games award for Makaton Learning Environment with Gamelab UK 2013
Appointed Associate Editor for IEEE Pattern Analysis and Machine Intelligence 2013
Best Paper Award at VISAPP2012
Advisory Board for Springer Advances in Computer Vision and Pattern Recognition
General Chair BMVC2012
Outstanding Reviewer Award ICCV 2011
Best Paper Award at IbPRIA2011
Main Track Chair (Computer & Robot Vision) ICPR2012, Japan
Appointed Associate Editor International Journal of Image & Vision Comp
Research interests
Richard's research focuses on the use of computer vision to locate, track and understand humans, with specific examples in Sign and Gesture recognition, Activity and Action recognition, lip-reading and facial feature tracking but also in HCI and cognitive robotics and machine perception where machines learn to understand and predict humans.
His research into tracking and artificial life received worldwide media coverage, appearing at the British Science Museum and the Minnesota Science Museum.
Research projects
The goal of the proposed project SMILE is to pioneer an assessment system for Swiss German Sign Language (Deutschschweizerische Gebärdensprache, DSGS) using automatic sign language recognition technology.
The project tackled the subject of automatically learn to recognise dynamic activity in broadcast footage with demonstration activities in both Sign Language and more general actions and activity
Improving investigative capability through the application of science and technology
Dicta-Sign aimed to enable communication between Deaf individuals by promoting the development of natural human computer interfaces (HCI) for Deaf users. It researched and develop recognition and synthesis systems for sign languages (SLs) at a level of detail necessary for recognising and generating authentic signing. Research outcomes were integrated into three prototypes (a Search-by-Example tool, a SL-to-SL translator and a sign-Wiki).
LiLiR looked at Language Independent Lip Reading from video
The DIPLECS project aimed to design an Artificial Cognitive System capable of learning and adapting to respond to everyday situations humans take for granted. The primary demonstration of its capability was providing assistance and advice to the driver of a car. The system learnt by watching humans, how they act and react while driving, building models of their behaviour and predicting what a driver would do when presented with a specific driving scenario.
In the COSPAL architecture we combined techniques from the field of artificial intelligence (AI) for symbolic reasoning and learning of artificial neural networks (ANN) for association of percepts and states. We establish feedback loops through the continuous and the symbolic parts of the system, which allow perception-action feedback at several levels in the system. After an initial bootstrapping phase, incremental learning techniques were used to train the system, allowing adaptation and exploration.
The central goal is to build a vision system that can be used in a wider variety of fields and that is re-usable by introducing self-adaptation at the level of perception, by providing categorisation capabilities, and by making explicit the knowledge base at the level of reasoning, and thereby enabling the knowledge base to be changed. In order to make these ideas concrete CogViSys aims at developing a virtual commentator which is able to translate visual information into a textual description.
Indicators of esteem
Awarded Fellow of the International Association of Pattern Recognition in 2016
Member of Royal Society’s International Exchanges Committee 2016
Royal Society Leverhulme Trust Senior Research Fellowship
Sullivan thesis prize in 2000
Executive Committee member and theme leader for EPSRC ViiHM Network 2015
TIGA Games award for Makaton Learning Environment with Gamelab UK 2013
Appointed Associate Editor for IEEE Pattern Analysis and Machine Intelligence 2013
Best Paper Award at VISAPP2012
Advisory Board for Springer Advances in Computer Vision and Pattern Recognition
General Chair BMVC2012
Outstanding Reviewer Award ICCV 2011
Best Paper Award at IbPRIA2011
Main Track Chair (Computer & Robot Vision) ICPR2012, Japan
Appointed Associate Editor International Journal of Image & Vision Comp
Supervision
Postgraduate research supervision
Current:
Sampo Kuutti, Jamie Spencer Martin, Celyn Walters, Stephanie Stoll, Rebecca Allday, Cihan Camgoz, Matthew Marter, Oscar Koller
Postgraduate research supervision
Graduated:
James Elder (Graduated 2017), Oscar Mendez Maldonado (Graduated 2017), Karel Lebada (Graduated 2016), Phil Krejov (Graduated 2016), Brian Holt (Graduated 2014), Simon Hadfield (Graduated 2013), Ashish Gupta (Graduated 2013), Timothy Sheerman-Chase (Graduated 2013), Stephen Moore (Graduated 2012), Olusegun Oshin (Graduated 2011), Dumebi Okwechime (Graduated 2011), Liam Ellis (Graduated 2010), Helen Cooper (Graduated 2010), Andrew Gilbert (Graduated 2008), Nickolas Dowson (Graduated 2006), Antonio Micilotta (Graduated 2005), Pakorn KaewTraKulPong (Graduated 2002).
Teaching
I current teaching level 1 C programming and lever 3/4 Object Orientation Programming in C++
I'm currently preparing a new course on robotics.
Publications
We release six datasets aimed at sign language translation research. Raw data was collected by broadcast partners SWISSTXT and VRT between the March-Aug 2020 period. Manual subtitle-sign video alignment has been done on a subset of the data and released for research purposes. Dataset contains anonymized sign language videos, aligned and raw subtitles and 2D/3D human skeletal pose information.For further information and to access the dataset, please visit: https://www.cvssp.org/data/c4a-news-corpus/
Neural Sign Language Production (SLP) aims to automatically translate from spoken language sentences to sign language videos. Historically the SLP task has been broken into two steps; Firstly, translating from a spoken language sentence to a gloss sequence and secondly, producing a sign language video given a sequence of glosses. In this paper we apply Natural Language Processing techniques to the first step of the SLP pipeline. We use language models such as BERT and Word2Vec to create better sentence level embeddings, and apply several tokenization techniques, demonstrating how these improve performance on the low resource translation task of Text to Gloss. We introduce Text to HamNoSys (T2H) translation, and show the advantages of using a phonetic representation for sign language translation rather than a sign level gloss representation. Furthermore, we use HamNoSys to extract the hand shape of a sign and use this as additional supervision during training, further increasing the performance on T2H. Assembling best practise, we achieve a BLEU-4 score of 26.99 on the MineDGS dataset and 25.09 on PHOENIX14T, two new state-of-the-art baselines.
This paper discusses the results for the second edition of the Monocular Depth Estimation Challenge (MDEC). This edition was open to methods using any form of supervision, including fully-supervised, self-supervised, multi-task or proxy depth. The challenge was based around the SYNS-Patches dataset, which features a wide diversity of environments with high-quality dense ground-truth. This includes complex natural environments, e.g. forests or fields, which are greatly underrepresented in current benchmarks. The challenge received eight unique submissions that outperformed the provided SotA baseline on any of the pointcloud- or image-based metrics. The top supervised submission improved relative F-Score by 27.62%, while the top self-supervised improved it by 16.61%. Supervised submissions generally leveraged large collections of datasets to improve data diversity. Self-supervised submissions instead updated the network architecture and pretrained backbones. These results represent a significant progress in the field, while highlighting avenues for future research, such as reducing interpolation artifacts at depth boundaries, improving self-supervised indoor performance and overall natural image accuracy.
Visual Odometry (VO) estimation is an important source of information for vehicle state estimation and autonomous driving. Recently, deep learning based approaches have begun to appear in the literature. However, in the context of driving, single sensor based approaches are often prone to failure because of degraded image quality due to environmental factors, camera placement, etc. To address this issue, we propose a deep sensor fusion framework which estimates vehicle motion using both pose and uncertainty estimations from multiple on-board cameras. We extract spatio-temporal feature representations from a set of consecutive images using a hybrid CNN-RNN model. We then utilise a Mixture Density Network (MDN) to estimate the 6-DoF pose as a mixture of distributions and a fusion module to estimate the final pose using MDN outputs from multi-cameras. We evaluate our approach on the publicly available, large scale autonomous vehicle dataset, nuScenes. The results show that the proposed fusion approach surpasses the state-of-the-art, and provides robust estimates and accurate trajectories compared to individual camera-based estimations.
Visual Odometry (VO) is used in many applications including robotics and autonomous systems. However, traditional approaches based on feature matching are computationally expensive and do not directly address failure cases, instead relying on heuristic methods to detect failure. In this work, we propose a deep learning-based VO model to efficiently estimate 6-DoF poses, as well as a confidence model for these estimates. We utilise a CNN-RNN hybrid model to learn feature representations from image sequences. We then employ a Mixture Density Network (MDN) which estimates camera motion as a mixture of Gaussians, based on the extracted spatio-temporal representations. Our model uses pose labels as a source of supervision, but derives uncertainties in an unsupervised manner. We evaluate the proposed model on the KITTI and nuScenes datasets and report extensive quantitative and qualitative results to analyse the performance of both pose and uncertainty estimation. Our experiments show that the proposed model exceeds state-of-the-art performance in addition to detecting failure cases using the predicted pose uncertainty.
Causal reasoning is a crucial part of science and human intelligence. In order to discover causal relationships from data, we need structure discovery methods. We provide a review of background theory and a survey of methods for structure discovery. We primarily focus on modern, continuous optimization methods, and provide reference to further resources such as benchmark datasets and software packages. Finally, we discuss the assumptive leap required to take us from structure to causality.
Sign languages use multiple asynchronous information channels (articulators), not just the hands but also the face and body, which computational approaches often ignore. In this paper we tackle the multiarticulatory sign language translation task and propose a novel multichannel transformer architecture. The proposed architecture allows both the inter and intra contextual relationships between different sign articulators to be modelled within the transformer network itself, while also maintaining channel specific information. We evaluate our approach on the RWTH-PHOENIX-Weather-2014T dataset and report competitive translation performance. Importantly, we overcome the reliance on gloss annotations which underpin other state-of-the-art approaches, thereby removing the need for expensive curated datasets.
3D hand pose estimation from images has seen considerable interest from the literature, with new methods improving overall 3D accuracy. One current challenge is to address hand-to-hand interaction where self-occlusions and finger articulation pose a significant problem to estimation. Little work has applied physical constraints that minimize the hand intersections that occur as a result of noisy estimation. This work addresses the intersection of hands by exploiting an occupancy network that represents the hand’s volume as a continuous manifold. This allows us to model the probability distribution of points being inside a hand. We designed an intersection loss function to minimize the likelihood of hand-to-point intersections. Moreover, we propose a new hand mesh parameterization that is superior to the commonly used MANO model in many respects including lower mesh complexity, underlying 3D skeleton extraction, watertightness, etc. On the benchmark INTERHAND2.6M dataset, the models trained using our intersection loss achieve better results than the state-of-the-art by significantly decreasing the number of hand intersections while lowering the mean per-joint positional error. Additionally, we demonstrate superior performance for 3D hand uplift on RE:INTERHAND and SMILE datasets and show reduced hand-to-hand intersections for complex domains such as sign-language pose estimation.
Motion estimation approaches typically employ sensor fusion techniques, such as the Kalman Filter, to handle individual sensor failures. More recently, deep learning-based fusion approaches have been proposed, increasing the performance and requiring less model-specific implementations. However, current deep fusion approaches often assume that sensors are synchronised, which is not always practical, especially for low-cost hardware. To address this limitation, in this work, we propose AFT-VO, a novel transformer-based sensor fusion architecture to estimate VO from multiple sensors. Our framework combines predictions from asynchronous multi-view cameras and accounts for the time discrepancies of measurements coming from different sources. Our approach first employs a Mixture Density Network (MDN) to estimate the probability distributions of the 6-DoF poses for every camera in the system. Then a novel transformer-based fusion module, AFT-VO, is introduced, which combines these asynchronous pose estimations, along with their confidences. More specifically, we introduce Discretiser and Source Encoding techniques which enable the fusion of multi-source asynchronous signals. We evaluate our approach on the popular nuScenes and KITTI datasets. Our experiments demonstrate that multi-view fusion for VO estimation provides robust and accurate trajectories, outperforming the state of the art in both challenging weather and lighting conditions.
Sign languages, often categorised as low-resource languages, face significant challenges in achieving accurate translation due to the scarcity of parallel annotated datasets. This paper introduces Select and Reorder (S&R), a novel approach that addresses data scarcity by breaking down the translation process into two distinct steps: Gloss Selection (GS) and Gloss Reordering (GR). Our method leverages large spoken language models and the substantial lexical overlap between source spoken languages and target sign languages to establish an initial alignment. Both steps make use of Non-AutoRegressive (NAR) decoding for reduced computation and faster inference speeds. Through this disentanglement of tasks, we achieve state-of-the-art BLEU and Rouge scores on the Meine DGS Annotated (mDGS) dataset, demonstrating a substantial BLUE-1 improvement of 37.88% in Text to Gloss (T2G) Translation. This innovative approach paves the way for more effective translation models for sign languages, even in resource-constrained settings.
Automatic Sign Language Translation requires the integration of both computer vision and natural language processing to effectively bridge the communication gap between sign and spoken languages. However, the deficiency in large-scale training data to support sign language translation means we need to leverage resources from spoken language. We introduce, Sign2GPT, a novel framework for sign language translation that utilizes large-scale pretrained vision and language models via lightweight adapters for gloss-free sign language translation. The lightweight adapters are crucial for sign language translation, due to the constraints imposed by limited dataset sizes and the computational requirements when training with long sign videos. We also propose a novel pretraining strategy that directs our encoder to learn sign representations from automatically extracted pseudo-glosses without requiring gloss order information or annotations. We evaluate our approach on two public benchmark sign language translation datasets, namely RWTH-PHOENIX-Weather 2014T and CSL-Daily, and improve on state-of-the-art gloss-free translation performance with a significant margin.
This paper discusses the results for the second edition of the Monocular Depth Estimation Challenge (MDEC). This edition was open to methods using any form of supervision, including fully-supervised, self-supervised, multi-task or proxy depth. The challenge was based around the SYNS-Patches dataset, which features a wide diversity of environments with high-quality dense ground-truth. This includes complex natural environments, e.g. forests or fields, which are greatly underrepresented in current benchmarks. The challenge received eight unique submissions that outperformed the provided SotA baseline on any of the pointcloud- or image-based metrics. The top supervised submission improved relative F-Score by 27.62%, while the top self-supervised improved it by 16.61%. Supervised submissions generally leveraged large collections of datasets to improve data diversity. Self-supervised submissions instead updated the network architecture and pretrained backbones. These results represent a significant progress in the field, while highlighting avenues for future research, such as reducing interpolation artifacts at depth boundaries, improving self-supervised indoor performance and overall natural image accuracy.
Hand pose estimation from a single image has many applications. However, approaches to full 3D body pose estimation are typically trained on day-to-day activities or actions. As such, detailed hand-to-hand interactions are poorly represented, especially during motion. We see this in the failure cases of techniques such as OpenPose or MediaPipe. However, accurate hand pose estimation is crucial for many applications where the global body motion is less important than accurate hand pose estimation. This paper addresses the problem of 3D hand pose estimation from monocular images or sequences. We present a novel end-to-end framework for 3D hand regression that employs diffusion models that have shown excellent ability to capture the distribution of data for generative purposes. Moreover, we enforce kinematic constraints to ensure realistic poses are generated by incorporating an explicit forward kinematic layer as part of the network. The proposed model provides state-of-the-art performance when lifting a 2D single-hand image to 3D. However, when sequence data is available, we add a Transformer module over a temporal window of consecutive frames to refine the results, overcoming jittering and further increasing accuracy. The method is quantitatively and qualitatively evaluated showing state-of-the-art robustness, generalization, and accuracy on several different datasets.
This work addresses 3D human pose reconstruction in single images. We present a method that combines Forward Kinematics (FK) with neural networks to ensure a fast and valid prediction of 3D pose. Pose is represented as a hierarchical tree/graph with nodes corresponding to human joints that model their physical limits. Given a 2D detection of keypoints in the image, we lift the skeleton to 3D using neural networks to predict both the joint rotations and bone lengths. These predictions are then combined with skeletal constraints using an FK layer implemented as a network layer in PyTorch. The result is a fast and accurate approach to the estimation of 3D skeletal pose. Through quantitative and qualitative evaluation, we demonstrate the method is significantly more accurate than MediaPipe in terms of both per joint positional error and visual appearance. Furthermore, we demonstrate generalization over different datasets. The implementation in PyTorch runs at between 100-200 milliseconds per image (including CNN detection) using CPU only.
Sign Language Translation (SLT) is a challenging task that aims to generate spoken language sentences from sign language videos, both of which have different grammar and word/gloss order. From a Neural Machine Translation (NMT) perspective, the straightforward way of training translation models is to use sign language phrase-spoken language sentence pairs. However, human interpreters heavily rely on the context to understand the conveyed information, especially for sign language interpretation, where the vocabulary size may be significantly smaller than their spoken language equivalent. Taking direct inspiration from how humans translate, we propose a novel multi-modal transformer architecture that tackles the translation task in a context-aware manner, as a human would. We use the context from previous sequences and confident predictions to disambiguate weaker visual cues. To achieve this we use complementary transformer encoders, namely: (1) A Video Encoder, that captures the low-level video features at the frame-level, (2) A Spotting Encoder, that models the recognized sign glosses in the video, and (3) A Context Encoder, which captures the context of the preceding sign sequences. We combine the information coming from these encoders in a final transformer decoder to generate spoken language translations. We evaluate our approach on the recently published large-scale BOBSL dataset, which contains ~1.2M sequences, and on the SRF dataset, which was part of the WMT-SLT 2022 challenge. We report significant improvements on state-of-the-art translation performance using contextual information, nearly doubling the reported BLEU-4 scores of baseline approaches.
In natural language processing (NLP) of spoken languages, word embeddings have been shown to be a useful method to encode the meaning of words. Sign languages are visual languages, which require sign embeddings to capture the visual and linguistic semantics of sign. Unlike many common approaches to Sign Recognition, we focus on explicitly creating sign embeddings that bridge the gap between sign language and spoken language. We propose a learning framework to derive LCC (Learnt Contrastive Concept) embeddings for sign language, a weakly supervised contrastive approach to learning sign embeddings. We train a vocabulary of embeddings that are based on the linguistic labels for sign video. Additionally, we develop a conceptual similarity loss which is able to utilise word embeddings from NLP methods to create sign embeddings that have better sign language to spoken language correspondence. These learnt representations allow the model to automatically localise the sign in time. Our approach achieves state-of-the-art keypoint-based sign recognition performance on the WLASL and BOBSL datasets.
To be truly understandable and accepted by Deaf communities, an automatic Sign Language Production (SLP) system must generate a photo-realistic signer. Prior approaches based on graphical avatars have proven unpopular, whereas recent neural SLP works that produce skeleton pose sequences have been shown to be not understandable to Deaf viewers. In this paper, we propose SignGAN, the first SLP model to produce photo-realistic continuous sign language videos directly from spoken language. We employ a transformer architecture with a Mixture Density Network (MDN) formulation to handle the translation from spoken language to skeletal pose. A pose-conditioned human synthesis model is then introduced to generate a photo-realistic sign language video from the skeletal pose sequence. This allows the photo-realistic production of sign videos directly translated from written text. We further propose a novel keypoint-based loss function, which significantly improves the quality of synthesized hand images, operating in the keypoint space to avoid issues caused by motion blur. In addition, we introduce a method for controllable video generation, enabling training on large, diverse sign language datasets and providing the ability to control the signer appearance at inference. Using a dataset of eight different sign language interpreters extracted from broadcast footage, we show that SignGAN significantly outperforms all baseline methods for quantitative metrics and human perceptual studies.
Capturing and annotating Sign language datasets is a time consuming and costly process. Current datasets are orders of magnitude too small to successfully train unconstrained \acf{slt} models. As a result, research has turned to TV broadcast content as a source of large-scale training data, consisting of both the sign language interpreter and the associated audio subtitle. However, lack of sign language annotation limits the usability of this data and has led to the development of automatic annotation techniques such as sign spotting. These spottings are aligned to the video rather than the subtitle, which often results in a misalignment between the subtitle and spotted signs. In this paper we propose a method for aligning spottings with their corresponding subtitles using large spoken language models. Using a single modality means our method is computationally inexpensive and can be utilized in conjunction with existing alignment techniques. We quantitatively demonstrate the effectiveness of our method on the \acf{mdgs} and \acf{bobsl} datasets, recovering up to a 33.22 BLEU-1 score in word alignment.
This paper discusses the results for the second edition of the Monocular Depth Estimation Challenge (MDEC). This edition was open to methods using any form of supervision, including fully-supervised, self-supervised, multi-task or proxy depth. The challenge was based around the SYNS-Patches dataset, which features a wide diversity of environments with high-quality dense ground-truth. This includes complex natural environments, e.g. forests or fields, which are greatly underrepresented in current benchmarks.The challenge received eight unique submissions that outperformed the provided SotA baseline on any of the pointcloud- or image-based metrics. The top supervised submission improved relative F-Score by 27.62%, while the top self-supervised improved it by 16.61%. Supervised submissions generally leveraged large collections of datasets to improve data diversity. Self-supervised submissions instead updated the network architecture and pre-trained backbones. These results represent a significant progress in the field, while highlighting avenues for future research, such as reducing interpolation artifacts at depth boundaries, improving self-supervised indoor performance and overall natural image accuracy.
Graph convolutional networks (GCNs) enable end-to-end learning on graph structured data. However, many works assume a given graph structure. When the input graph is noisy or unavailable, one approach is to construct or learn a latent graph structure. These methods typically fix the choice of node degree for the entire graph, which is suboptimal. Instead, we propose a novel end-to-end differentiable graph generator which builds graph topologies where each node selects both its neighborhood and its size. Our module can be readily integrated into existing pipelines involving graph convolution operations, replacing the predetermined or existing adjacency matrix with one that is learned, and optimized, as part of the general objective. As such it is applicable to any GCN. We integrate our module into trajectory prediction, point cloud classification and node classification pipelines resulting in improved accuracy over other structure-learning methods across a wide range of datasets and GCN backbones.
Self-supervised monocular depth estimation (SS-MDE) has the potential to scale to vast quantities of data. Unfortunately, existing approaches limit themselves to the automotive domain, resulting in models incapable of generalizing to complex environments such as natural or indoor settings. To address this, we propose a large-scale SlowTV dataset curated from YouTube, containing an order of magnitude more data than existing automotive datasets. SlowTV contains 1.7M images from a rich diversity of environments, such as worldwide seasonal hiking, scenic driving and scuba diving. Using this dataset, we train an SS-MDE model that provides zero-shot generalization to a large collection of indoor/outdoor datasets. The resulting model outperforms all existing SSL approaches and closes the gap on supervised SoTA, despite using a more efficient architecture. We additionally introduce a collection of best-practices to further maximize performance and zero-shot generalization. This includes 1) aspect ratio augmentation, 2) camera intrinsic estimation, 3) support frame randomization and 4) flexible motion estimation. Code is available at https://github.com/jspenmar/slowtv_monodepth.
Sign languages are visual languages, with vocabularies as rich as their spoken language counterparts. However, current deep-learning based Sign Language Production (SLP) models produce under-articulated skeleton pose sequences from constrained vocabularies and this limits applicability. To be understandable and accepted by the deaf, an automatic SLP system must be able to generate co-articulated photo-realistic signing sequences for large domains of discourse. In this work, we tackle large-scale SLP by learning to co-articulate between dictionary signs, a method capable of producing smooth signing while scaling to unconstrained domains of discourse. To learn sign co-articulation, we propose a novel Frame Selection Network ( FS- NET) that improves the temporal alignment of interpolated dictionary signs to continuous signing sequences. Additionally, we propose SIGNGAN, a pose-conditioned human synthesis model that produces photo-realistic sign language videos direct from skeleton pose. We propose a novel keypoint- based loss function which improves the quality of synthesized hand images. We evaluate our SLP model on the large-scale meineDGS (mDGS) corpus, conducting extensive user evaluation showing our FS-NET approach improves coarticulation of interpolated dictionary signs. Additionally, we show that SIGNGAN significantly outperforms all baseline methods for quantitative metrics, human perceptual studies and native deaf signer comprehension.
This paper presents the results of the First WMT Shared Task on Sign Language Translation (WMT-SLT22) 1. This shared task is concerned with automatic translation between signed and spoken 2 languages. The task is novel in the sense that it requires processing visual information (such as video frames or human pose estimation) beyond the wellknown paradigm of text-to-text machine translation (MT). The task featured two tracks, translating from Swiss German Sign Language (DSGS) to German and vice versa. Seven teams participated in this first edition of the task, all submitting to the DSGS-to-German track. Besides a system ranking and system papers describing state-of-the-art techniques, this shared task makes the following scientific contributions: novel corpora, reproducible baseline systems and new protocols and software for human evaluation. Finally, the task also resulted in the first publicly available set of system outputs and human evaluation scores for sign language translation.
Accurate camera synchronisation is indispensable for many video processing tasks, such as surveillance and 3D modelling. Video-based synchronisation facilitates the design and setup of networks with moving cameras or devices without an external synchronisation capability, such as low-cost web cameras, or Kinects. In this paper, we present an algorithm which can work with such heterogeneous networks. The algorithm first finds the corresponding frame indices between each camera pair, by the help of image feature correspondences and epipolar geometry. Then, for each pair, a relative frame rate and offset are computed by fitting a 2D line to the index correspondences. These pairwise relations define a graph, in which each spanning cycle comprises an absolute synchronisation hypothesis. The optimal solution is found by an exhaustive search over the spanning cycles. The algorithm is experimentally demonstrated to yield highly accurate estimates in a number of scenarios involving static and moving cameras, and Kinect.
Autonomous agents that drive on roads shared with human drivers must reason about the nuanced interactions among traffic participants. This poses a highly challenging decision making problem since human behavior is influenced by a multitude of factors (e.g., human intentions and emotions) that are hard to model. This paper presents a decision making approach for autonomous driving, focusing on the complex task of merging into moving traffic where uncertainty emanates from the behavior of other drivers and imperfect sensor measurements. We frame the problem as a partially observable Markov decision process (POMDP) and solve it online with Monte Carlo tree search. The solution to the POMDP is a policy that performs high-level driving maneuvers, such as giving way to an approaching car, keeping a safe distance from the vehicle in front or merging into traffic. Our method leverages a model learned from data to predict the future states of traffic while explicitly accounting for interactions among the surrounding agents. From these predictions, the autonomous vehicle can anticipate the future consequences of its actions on the environment and optimize its trajectory accordingly. We thoroughly test our approach in simulation, showing that the autonomous vehicle can adapt its behavior to different situations. We also compare against other methods, demonstrating an improvement with respect to the considered performance metrics.
The use of neural networks and reinforcement learning has become increasingly popular in autonomous vehicle control. However, the opaqueness of the resulting control policies presents a significant barrier to deploying neural network-based control in autonomous vehicles. In this paper, we present a reinforcement learning based approach to autonomous vehicle longitudinal control, where the rule-based safety cages provide enhanced safety for the vehicle as well as weak supervision to the reinforcement learning agent. By guiding the agent to meaningful states and actions, this weak supervision improves the convergence during training and enhances the safety of the final trained policy. This rule-based supervisory controller has the further advantage of being fully interpretable, thereby enabling traditional validation and verification approaches to ensure the safety of the vehicle. We compare models with and without safety cages, as well as models with optimal and constrained model parameters, and show that the weak supervision consistently improves the safety of exploration, speed of convergence, and model performance. Additionally, we show that when the model parameters are constrained or sub-optimal, the safety cages can enable a model to learn a safe driving policy even when the model could not be trained to drive through reinforcement learning alone.
Designing a controller for autonomous vehicles capable of providing adequate performance in all driving scenarios is challenging due to the highly complex environment and inability to test the system in the wide variety of scenarios which it may encounter after deployment. However, deep learning methods have shown great promise in not only providing excellent performance for complex and non-linear control problems, but also in generalising previously learned rules to new scenarios. For these reasons, the use of deep learning for vehicle control is becoming increasingly popular. Although important advancements have been achieved in this field, these works have not been fully summarised. This paper surveys a wide range of research works reported in the literature which aim to control a vehicle through deep learning methods. Although there exists overlap between control and perception, the focus of this paper is on vehicle control, rather than the wider perception problem which includes tasks such as semantic segmentation and object detection. The paper identifies the strengths and limitations of available deep learning methods through comparative analysis and discusses the research challenges in terms of computation, architecture selection, goal specification, generalisation, verification and validation, as well as safety. Overall, this survey brings timely and topical information to a rapidly evolving field relevant to intelligent transportation systems.
To plan safe maneuvers and act with foresight, autonomous vehicles must be capable of accurately predicting the uncertain future. In the context of autonomous driving, deep neural networks have been successfully applied to learning predictive models of human driving behavior from data. However, the predictions suffer from cascading errors, resulting in large inaccuracies over long time horizons. Furthermore, the learned models are black boxes, and thus it is often unclear how they arrive at their predictions. In contrast, rule-based models-which are informed by human experts-maintain long-term coherence in their predictions and are human-interpretable. However, such models often lack the sufficient expressiveness needed to capture complex real-world dynamics. In this work, we begin to close this gap by embedding the Intelligent Driver Model, a popular hand-crafted driver model, into deep neural networks. Our model's transparency can offer considerable advantages, e.g., in debugging the model and more easily interpreting its predictions. We evaluate our approach on a simulated merging scenario, showing that it yields a robust model that is end-to-end trainable and provides greater transparency at no cost to the model's predictive accuracy.
Capturing and annotating Sign language datasets is a time consuming and costly process. Current datasets are orders of magnitude too small to successfully train unconstrained Sign Language Translation (SLT) models. As a result, research has turned to TV broadcast content as a source of large-scale training data, consisting of both the sign language interpreter and the associated audio subtitle. However, lack of sign language annotation limits the usability of this data and has led to the development of automatic annotation techniques such as sign spotting. These spottings are aligned to the video rather than the subtitle, which often results in a misalignment between the subtitle and spotted signs. In this paper we propose a method for aligning spottings with their corresponding subtitles using large spoken language models. Using a single modality means our method is computationally inexpensive and can be utilized in conjunction with existing alignment techniques. We quantitatively demonstrate the effectiveness of our method on the Meine DGS-Annotated (MeineDGS) and BBC-Oxford British Sign Language (BOBSL) datasets, recovering up to a 33.22 BLEU-1 score in word alignment.
Fair and unbiased machine learning is an important and active field of research, as decision processes are increasingly driven by models that learn from data. Unfortunately, any biases present in the data may be learned by the model, thereby inappropriately transferring that bias into the decision making process. We identify the connection between the task of bias reduction and that of isolating factors common between domains whilst encouraging domain specific invariance. To isolate the common factors we combine the theory of deep latent variable models with information bottleneck theory for scenarios whereby data may be naturally paired across domains and no additional supervision is required. The result is the Nested Variational AutoEncoder (NestedVAE). Two outer VAEs with shared weights attempt to reconstruct the input and infer a latent space, whilst a nested VAE attempt store construct the latent representation of one image,from the latent representation of its paired image. In so doing,the nested VAE isolates the common latent factors/causes and becomes invariant to unwanted factors that are not shared between paired images. We also propose a new metric to provide a balanced method of evaluating consistency and classifier performance across domains which we refer to as the Adjusted Parity metric. An evaluation of Nested VAE on both domain and attribute invariance, change detection,and learning common factors for the prediction of biological sex demonstrates that NestedVAE significantly outperforms alternative methods.
Sign Languages are rich multi-channel languages, requiring articulation of both manual (hands) and non-manual (face and body) features in a precise, intricate manner. Sign Language Production (SLP), the automatic translation from spoken to sign languages, must embody this full sign morphology to be truly understandable by the Deaf community. Previous work has mainly focused on manual feature production, with an under-articulated output caused by regression to the mean. In this paper, we propose an Adversarial Multi-Channel approach to SLP. We frame sign production as a minimax game between a transformer-based Generator and a conditional Discriminator. Our adversarial discriminator evaluates the realism of sign production conditioned on the source text, pushing the generator towards a realistic and articulate output. Additionally, we fully encapsulate sign articulators with the inclusion of non-manual features, producing facial features and mouthing patterns. We evaluate on the challenging RWTH-PHOENIX-Weather-2014T (PHOENIX14T) dataset, and report state-of-the art SLP back-translation performance for manual production. We set new benchmarks for the production of multi-channel sign to underpin future research into realistic SLP.
Human pose estimation in static images has received significant attention recently but the problem remains challenging. Using data acquired from a consumer depth sensor, our method combines a direct regression approach for the estimation of rigid body parts with the extraction of geodesic extrema to find extremities. We show how these approaches are complementary and present a novel approach to combine the results resulting in an improvement over the state-of-the-art. We report and compare our results a new dataset of aligned RGB-D pose sequences which we release as a benchmark for further evaluation. © 2013 IEEE.
The objective of the “Sign Language Recognition, Translation & Production” (SLRTP 2020) Workshop was to bring together researchers who focus on the various aspects of sign language understanding using tools from computer vision and linguistics. The workshop sought to promote a greater linguistic and historical understanding of sign languages within the computer vision community, to foster new collaborations and to identify the most pressing challenges for the field going forwards. The workshop was held in conjunction with the European Conference on Computer Vision (ECCV), 2020.
We investigate the recognition of actions "in the wild"’ using 3D motion information. The lack of control over (and knowledge of) the camera configuration, exacerbates this already challenging task, by introducing systematic projective inconsistencies between 3D motion fields, hugely increasing intra-class variance. By introducing a robust, sequence based, stereo calibration technique, we reduce these inconsistencies from fully projective to a simple similarity transform. We then introduce motion encoding techniques which provide the necessary scale invariance, along with additional invariances to changes in camera viewpoint. On the recent Hollywood 3D natural action recognition dataset, we show improvements of 40% over previous state-of-the-art techniques based on implicit motion encoding. We also demonstrate that our robust sequence calibration simplifies the task of recognising actions, leading to recognition rates 2.5 times those for the same technique without calibration. In addition, the sequence calibrations are made available.
This work presents a framework to recognise signer independent mouthings in continuous sign language, with no manual annotations needed. Mouthings represent lip-movements that correspond to pronunciations of words or parts of them during signing. Research on sign language recognition has focused extensively on the hands as features. But sign language is multi-modal and a full understanding particularly with respect to its lexical variety, language idioms and grammatical structures is not possible without further exploring the remaining information channels. To our knowledge no previous work has explored dedicated viseme recognition in the context of sign language recognition. The approach is trained on over 180.000 unlabelled frames and reaches 47.1% precision on the frame level. Generalisation across individuals and the influence of context-dependent visemes are analysed. © 2014 Springer International Publishing.
We organized a Grand Challenge and Workshop on Multi-Modal Gesture Recognition. The MMGR Grand Challenge focused on the recognition of continuous natural gestures from multi-modal data (including RGB, Depth, user mask, Skeletal model, and audio). We made available a large labeled video database of 13,858 gestures from a lexicon of 20 Italian gesture categories recorded with a Kinect TM camera. More than 54 teams participated in the challenge and a final error rate of 12% was achieved by the winner of the competition. Winners of the competition published their work in the workshop of the Challenge. The MMGR Workshop was held at ICMI conference 2013, Sidney. A total of 9 relevant papers with basis on multi-modal gesture recognition were accepted for presentation. This includes multi-modal descriptors, multi-class learning strategies for segmentation and classification in temporal data, as well as relevant applications in the field, including multi-modal Social Signal Processing and multi-modal Human Computer Interfaces. Five relevant invited speakers participated in the workshop: Profs. Leonid Signal from Disney Research, Antonis Argyros from FORTH, Institute of Computer Science, Cristian Sminchisescu from Lund University, Richard Bowden from University of Surrey, and Stan Sclaroff from Boston University. They summarized their research in the field and discussed past, current, and future challenges in Multi-Modal Gesture Recognition.
This paper attempts to tackle the problem of lipreading by building visual sequence classifiers that are based on salient temporal signatures. The temporal signatures used in this paper allow us to capture spatio-temporal information that can span multiple feature dimensions with gaps in the temporal axis. Selecting suitable temporal signatures by exhaustive search is not possible given the immensely large search space. As an example, the temporal sequence used in this paper would require exhaustively evaluating 2 2000 temporal signatures which is simply not possible. To address this, a novel gradient-descent based method is proposed to search for a suitable candidate temporal signature. Crucially, this is achieved very efficiently with O(nD) complexity, where D is the static feature vector dimensionality and n the maximum length of the temporal signatures considered. We then integrate this temporal search method into the AdaBoost algorithm. The results are spatio-temporal strong classifiers that can be applied to multi-class recognition in the lipreading domain. We provide experimental results evaluating the performance of our method against existing work in both subject dependent and subject independent cases demonstrating state of the art performance. Importantly, this was also achieved with a small set of temporal signatures.
The aim of this paper is to learn driving behaviour by associating the actions recorded from a human driver with pre-attentive visual input, implemented using holistic image features (GIST). All images are labelled according to a number of driving–relevant contextual classes (eg, road type, junction) and the driver’s actions (eg, braking, accelerating, steering) are recorded. The association between visual context and the driving data is learnt by Boosting decision stumps, that serve as input dimension selectors. Moreover, we propose a novel formulation of GIST features that lead to an improved performance for action prediction. The areas of the visual scenes that contribute to activation or inhibition of the predictors is shown by drawing activation maps for all learnt actions. We show good performance not only for detecting driving–relevant contextual labels, but also for predicting the driver’s actions. The classifier’s false positives and the associated activation maps can be used to focus attention and further learning on the uncommon and difficult situations.
Tracking hands and estimating their trajectories is useful in a number of tasks, including sign language recognition and human computer interaction. Hands are extremely difficult objects to track, their deformability, frequent self occlusions and motion blur cause appearance variations too great for most standard object trackers to deal with robustly. In this paper, the 3D motion field of a scene (known as the Scene Flow, in contrast to Optical Flow, which is it’s projection onto the image plane) is estimated using a recently proposed algorithm, inspired by particle filtering. Unlike previous techniques, this scene flow algorithm does not introduce blurring across discontinuities, making it far more suitable for object segmentation and tracking. Additionally the algorithm operates several orders of magnitude faster than previous scene flow estimation systems, enabling the use of Scene Flow in real-time, and near real-time applications. A novel approach to trajectory estimation is then introduced, based on clustering the estimated scene flow field in both space and velocity dimensions. This allows estimation of object motions in the true 3D scene, rather than the traditional approach of estimating 2D image plane motions. By working in the scene space rather than the image plane, the constant velocity assumption, commonly used in the prediction stage of trackers, is far more valid, and the resulting motion estimate is richer, providing information on out of plane motions. To evaluate the performance of the system, 3D trajectories are estimated on a multi-view sign-language dataset, and compared to a traditional high accuracy 2D system, with excellent results.
The main focus of this paper is to present a method of reusing motion captured data by learning a generative model of motion. The model allows synthesis and blending of cyclic motion, whilst providing it with the style and realism present in the original data. This is achieved by projecting the data into a lower dimensional space and learning a multivariate probability distribution of the motion sequences. Functioning as a generative model, the probability density estimation is used to produce novel motions from the model and gradient based optimisation used to generate the final animation. Results show plausible motion generation and lifelike blends between different actions.
An approach for fast tracking of arbitrary image features with no prior model and no offline learning stage is presented. Fast tracking is achieved using banks of linear displacement predictors learnt online. A multi-modal appearance model is also learnt on-the-fly that facilitates the selection of subsets of predictors suitable for prediction in the next frame. The approach is demonstrated in real-time on a number of challenging video sequences and experimentally compared to other simultaneous modeling and tracking approaches with favourable results.
In this paper, we propose using 3D Convolutional Neural Networks for large scale user-independent continuous gesture recognition. We have trained an end-to-end deep network for continuous gesture recognition (jointly learning both the feature representation and the classifier). The network performs three-dimensional (i.e. space-time) convolutions to extract features related to both the appearance and motion from volumes of color frames. Space-time invariance of the extracted features is encoded via pooling layers. The earlier stages of the network are partially initialized using the work of Tran et al. before being adapted to the task of gesture recognition. An earlier version of the proposed method, which was trained for 11,250 iterations, was submitted to ChaLearn 2016 Continuous Gesture Recognition Challenge and ranked 2nd with the Mean Jaccard Index Score of 0.269235. When the proposed method was further trained for 28,750 iterations, it achieved state-of-the-art performance on the same dataset, yielding a 0.314779 Mean Jaccard Index Score.
In this paper, we focus on the task of one-shot sign spotting, i.e. given an example of an isolated sign (query), we want to identify whether/where this sign appears in a continuous, co-articulated sign language video (target). To achieve this goal, we propose a transformer-based network, called Sign-Lookup. We employ 3D Convolutional Neural Networks (CNNs) to extract spatio-temporal representations from video clips. To solve the temporal scale discrepancies between the query and the target videos, we construct multiple queries from a single video clip using different frame-level strides. Self-attention is applied across these query clips to simulate a continuous scale space. We also utilize another self-attention module on the target video to learn the contextual within the sequence. Finally mutual-attention is used to match the temporal scales to localize the query within the target sequence. Extensive experiments demonstrate that the proposed approach can not only reliably identify isolated signs in continuous videos, regardless of the signers' appearance, but can also generalize to different sign languages. By taking advantage of the attention mechanism and the adaptive features, our model achieves state-of-the-art performance on the sign spotting task with accuracy as high as 96% on challenging benchmark datasets and significantly outperforming other approaches.
This paper presents an open and comprehensive framework to systematically evaluate state-of-the-art contributions to self-supervised monocular depth estimation. This includes pretraining, backbone, architectural design choices and loss functions. Many papers in this field claim novelty in either architecture design or loss formulation. However, simply updating the backbone of historical systems results in relative improvements of 25%, allowing them to outperform the majority of existing systems. A systematic evaluation of papers in this field was not straightforward. The need to compare like-with-like in previous papers means that longstanding errors in the evaluation protocol are ubiquitous in the field. It is likely that many papers were not only optimized for particular datasets, but also for errors in the data and evaluation criteria. To aid future research in this area, we release a modular codebase (this https URL), allowing for easy evaluation of alternate design decisions against corrected data and evaluation criteria. We re-implement, validate and re-evaluate 16 state-of-the-art contributions and introduce a new dataset (SYNS-Patches) containing dense outdoor depth maps in a variety of both natural and urban scenes. This allows for the computation of informative metrics in complex regions such as depth boundaries.
We present a new approach for synthesizing novel views of people in new poses. Our novel differentiable renderer enables the synthesis of highly realistic images from any viewpoint. Rather than operating over mesh-based structures, our renderer makes use of diffuse Gaussian primitives that directly represent the underlying skeletal structure of a human. Rendering these primitives gives results in a high-dimensional latent image, which is then transformed into an RGB image by a decoder network. The formulation gives rise to a fully differentiable framework that can be trained end-to-end. We demonstrate the effectiveness of our approach to image reconstruction on both the Human3.6M and Panoptic Studio datasets. We show how our approach can be used for motion transfer between individuals; novel view synthesis of individuals captured from just a single camera; to synthesize individuals from any virtual viewpoint; and to re-render people in novel poses.
Real-time segmentation of moving regions in image sequences is a fundamental step in many vision systems including automated visual surveillance, human-machine interface, and very low-bandwidth telecommunications. A typical method is background subtraction. Many background models have been introduced to deal with different problems. One of the successful solutions to these problems is to use a multi-colour background model per pixel proposed by Grimson et al [1, 2,3]. However, the method suffers from slow learning at the beginning, especially in busy environments. In addition, it can not distinguish between moving shadows and moving objects. This paper presents a method which improves this adaptive background mixture model. By reinvestigating the update equations, we utilise different equations at different phases. This allows our system learn faster and more accurately as well as adapts effectively to changing environment. A shadow detection scheme is also introduced in this paper. It is based on a computational colour space that makes use of our background model. A comparison has been made between the two algorithms. The results show the speed of learning and the accuracy of the model using our update algorithm over the Grimson et al’s tracker. When incorporate with the shadow detection, our method results in far better segmentation than The Thirteenth Conference on Uncertainty in Artificial Intelligence that of Grimson et al.
We propose a novel approach to tracking objects by low-level line correspondences. In our implementation we show that this approach is usable even when tracking objects with lack of texture, exploiting situations, when feature-based trackers fails due to the aperture problem. Furthermore, we suggest an approach to failure detection and recovery to maintain long-term stability. This is achieved by remembering configurations which lead to good pose estimations and using them later for tracking corrections. We carried out experiments on several sequences of different types. The proposed tracker proves itself as competitive or superior to state-of-the-art trackers in both standard and low-textured scenes. © 2013 Springer-Verlag.
This paper proposes a non-linear predictor for estimating the displacement of tracked feature points on faces that exhibit significant variations across pose and expression. Existing methods such as linear predictors, ASMs or AAMs are limited to a narrow range in pose. In order to track across a large pose range, separate pose-specific models are required that are then coupled via a pose-estimator. In our approach, we neither require a set of pose-specific models nor a pose-estimator. Using just a single tracking model, we are able to robustly and accurately track across a wide range of expression on poses. This is achieved by gradient boosting of regression trees for predicting the displacement vectors of tracked points. Additionally, we propose a novel algorithm for simultaneously configuring this hierarchical set of trackers for optimal tracking results. Experiments were carried out on sequences of naturalistic conversation and sequences with large pose and expression changes. The results show that the proposed method is superior to state of the art methods, in being able to robustly track a set of facial points whilst gracefully recovering from tracking failures. © 2013 IEEE.
We present an approach to automatically expand the annotation of images using the internet as an additional information source. The novelty of the work is in the expansion of image tags by automatically introducing new unseen complex linguistic labels which are collected unsupervised from associated webpages. Taking a small subset of existing image tags, a web based search retrieves additional textual information. Both a textual bag of words model and a visual bag of words model are combined and symbolised for data mining. Association rule mining is then used to identify rules which relate words to visual contents. Unseen images that fit these rules are re-tagged. This approach allows a large number of additional annotations to be added to unseen images, on average 12.8 new tags per image, with an 87.2% true positive rate. Results are shown on two datasets including a new 2800 image annotation dataset of landmarks, the results include pictures of buildings being tagged with the architect, the year of construction and even events that have taken place there. This widens the tag annotation impact and their use in retrieval. This dataset is made available along with tags and the 1970 webpages and additional images which form the information corpus. In addition, results for a common state-of-the-art dataset MIRFlickr25000 are presented for comparison of the learning framework against previous works. © 2013 Springer-Verlag.
This paper proposes a novel machine learning algorithm (SP-Boosting) to tackle the problem of lipreading by building visual sequence classifiers based on sequential patterns. We show that an exhaustive search of optimal sequential patterns is not possible due to the immense search space, and tackle this with a novel, efficient tree-search method with a set of pruning criteria. Crucially, the pruning strategies preserve our ability to locate the optimal sequential pattern. Additionally, the tree-based search method accounts for the training set's boosting weight distribution. This temporal search method is then integrated into the boosting framework resulting in the SP-Boosting algorithm. We also propose a novel constrained set of strong classifiers that further improves recognition accuracy. The resulting learnt classifiers are applied to lipreading by performing multi-class recognition on the OuluVS database. Experimental results show that our method achieves state of the art recognition performane, using only a small set of sequential patterns. © 2011. The copyright of this document resides with its authors.
We present an approach to iteratively cluster images and video in an efficient and intuitive manor. While many techniques use the traditional approach of time consuming groundtruthing large amounts of data [10, 16, 20, 23], this is increasingly infeasible as dataset size and complexity increase. Furthermore it is not applicable to the home user, who wants to intuitively group his/her own media without labelling the content. Instead we propose a solution that allows the user to select media that semantically belongs to the same class and use machine learning to "pull" this and other related content together. We introduce an "image signature" descriptor and use min-Hash and greedy clustering to efficiently present the user with clusters of the dataset using multi-dimensional scaling. The image signatures of the dataset are then adjusted by APriori data mining identifying the common elements between a small subset of image signatures. This is able to both pull together true positive clusters and push apart false positive examples. The approach is tested on real videos harvested from the web using the state of the art YouTube dataset [18]. The accuracy of correct group label increases from 60.4% to 81.7% using 15 iterations of pulling and pushing the media around. While the process takes only 1 minute to compute the pair wise similarities of the image signatures and visualise the youtube whole dataset. © 2011. The copyright of this document resides with its authors.
This paper presents a novel adaptation of fuzzy clustering and feature encoding for image classification. Visual word ambiguity has recently been successfully modeled by kernel codebooks to provide improvement in classification performance over the standard 'Bag-of-Features' (BoF) approach, which uses hard partitioning and crisp logic for assignment of features to visual words. Motivated by this progress we utilize fuzzy logic to model the ambiguity and combine it with clustering to discover fuzzy visual words. The feature descriptors of an image are encoded using the learned fuzzy membership function associated with each word. The codebook built using this fuzzy encoding technique is demonstrated to provide superior performance over BoF. We use the Gustafson-Kessel algorithm which is an improvement over Fuzzy C-Means clustering and can adapt to local distributions. We evaluate our approach on several popular datasets and demonstrate that it consistently provides superior performance to the BoF approach. © 2012 IEEE.
Estimating the pose of an object, be it articulated, deformable or rigid, is an important task, with applications ranging from Human-Computer Interaction to environmental understanding. The idea of a general pose estimation framework, capable of being rapidly retrained to suit a variety of tasks, is appealing. In this paper a solution isproposed requiring only a set of labelled training images in order to be applied to many pose estimation tasks. This is achieved bytreating pose estimation as a classification problem, with particle filtering used to provide non-discretised estimates. Depth information extracted from a calibrated stereo sequence, is used for background suppression and object scale estimation. The appearance and shape channels are then transformed to Local Binary Pattern histograms, and pose classification is performed via a randomised decision forest. To demonstrate flexibility, the approach is applied to two different situations, articulated hand pose and rigid head orientation, achieving 97% and 84% accurate estimation rates, respectively.
The paper provides a report on the user-centred showcase prototypes of the DICTA-SIGN project (http://www.dictasign.eu/), an FP7-ICT project which ended in January 2012. DICTA-SIGN researched ways to enable communication between Deaf individuals through the development of human-computer interfaces (HCI) for Deaf users, by means of Sign Language. Emphasis is placed on the Sign-Wiki prototype that demonstrates the potential of sign languages to participate in contemporary Web 2.0 applications where user contributions are editable by an entire community and sign language users can benefit from collaborative editing facilities. © 2012 Springer-Verlag.
This paper presents a humanoid computer interface (Jeremiah) that is capable of extracting moving objects from a video stream and responding by directing the gaze of an animated head toward it. It further responds through change of expression reflecting the emotional state of the system as a response to stimuli. As such, the system exhibits similar behavior to a child. The system was originally designed as a robust visual tracking system capable of performing accurately and consistently within a real world visual surveillance arena. As such, it provides a system capable of operating reliably in any environment both indoor and outdoor. Originally designed as a public interface to promote computer vision and the public understanding of science (exhibited in British Science Museum), Jeremiah provides the first step to a new form of intuitive computer interface. Copyright © ACM 2002.
In this chapter, we present a generic classifier for detecting spatio-temporal interest points within video, the premise being that, given an interest point detector, we can learn a classifier that duplicates its functionality and which is both accurate and computationally efficient. This means that interest point detection can be achieved independent of the complexity of the original interest point formulation. We extend the naive Bayesian classifier of Randomised Ferns to the spatio-temporal domain and learn classifiers that duplicate the functionality of common spatio-temporal interest point detectors. Results demonstrate accurate reproduction of results with a classifier that can be applied exhaustively to video at frame-rate, without optimisation, in a scanning window approach. © 2010, IGI Global.
Automatic estimation of human pose has long been a goal of computer vision, to which a solution would have a wide range of applications. In this paper we formulate the pose estimation task within a regression and Hough voting framework to predict 2D joint locations from depth data captured by a consumer depth camera. In our approach the offset from each pixel to the location of each joint is predicted directly using random regression forests. The predictions are accumulated in Hough images which are treated as likelihood distributions where maxima correspond to joint location hypotheses. Our approach is evaluated on a publicly available dataset with good results. © Springer-Verlag Berlin Heidelberg 2013.
We propose a method to generate linguistically meaningful subunits in a fully automated fashion for sign language corpora. The ability to automate the process of subunit annotation has profound effects on the data available for training sign language recognition systems. The approach is based on the idea that subunits are shared among different signs. With sufficient data and knowledge of possible signing variants, accurate automatic subunit sequences are produced, matching the specific characteristics of given sign language data. Specifically we demonstrate how an iterative forced alignment algorithm can be used to transfer the knowledge of a user-edited open sign language dictionary to the task of annotating a challenging, large vocabulary, multi-signer corpus recorded from public TV. Existing approaches focus on labour intensive manual subunit annotations or on data-driven approaches. Our method yields an average precision and recall of 15% under the maximum achievable accuracy with little user intervention beyond providing a simple word gloss. © 2013 IEEE.
Since the advent of multitouch screens users have been able to interact using fingertip gestures in a two dimensional plane. With the development of depth cameras, such as the Kinect, attempts have been made to reproduce the detection of gestures for three dimensional interaction. Many of these use contour analysis to find the fingertips, however the success of such approaches is limited due to sensor noise and rapid movements. This paper discusses an approach to identify fingertips during rapid movement at varying depths allowing multitouch without contact with a screen. To achieve this, we use a weighted graph that is built using the depth information of the hand to determine the geodesic maxima of the surface. Fingertips are then selected from these maxima using a simplified model of the hand and correspondence found over successive frames. Our experiments show real-time performance for multiple users providing tracking at 30fps for up to 4 hands and we compare our results with stateof- the-art methods, providing accuracy an order of magnitude better than existing approaches. © 2013 IEEE.
This paper presents a probabilistic framework of assembling detected human body parts into a full 2D human configuration. The face, torso, legs and hands are detected in cluttered scenes using boosted body part detectors trained by AdaBoost. Body configurations are assembled from the detected parts using RANSAC, and a coarse heuristic is applied to eliminate obvious outliers. An a priori mixture model of upper-body configurations is used to provide a pose likelihood for each configuration. A joint-likelihood model is then determined by combining the pose, part detector and corresponding skin model likelihoods. The assembly with the highest likelihood is selected by RANSAC, and the elbow positions are inferred. This paper also illustrates the combination of skin colour likelihood and detection likelihood to further reduce false hand and face detections.
Estimating a semantically segmented bird's-eye-view (BEV) map from a single image has become a popular technique for autonomous control and navigation. However, they show an increase in localization error with distance from the camera. While such an increase in error is entirely expected - localization is harder at distance - much of the drop in performance can be attributed to the cues used by current texture-based models, in particular, they make heavy use of object-ground intersections (such as shadows) [10]. which become increasingly sparse and uncertain for distant objects. In this work, we address these shortcomings in BEV-mapping by learning the spatial relationship between objects in a scene. We propose a graph neural network which predicts BEV objects from a monocular image by spatially reasoning about an object within the context of other objects. Our approach sets a new state-of-the-art in BEV estimation from monocular images across three large-scale datasets, including a 50% relative improvement for objects on nuScenes.
We present a novel data-driven regularizer for weakly-supervised learning of 3D human pose estimation that eliminates the drift problem that affects existing approaches. We do this by moving the stereo reconstruction problem into the loss of the network itself. This avoids the need to reconstruct 3D data prior to training and unlike previous semi-supervised approaches, avoids the need for a warm-up period of supervised training. The conceptual and implementational simplicity of our approach is fundamental to its appeal. Not only is it straightforward to augment many weakly-supervised approaches with our additional re-projection based loss, but it is obvious how it shapes reconstructions and prevents drift. As such we believe it will be a valuable tool for any researcher working in weakly-supervised 3D reconstruction. Evaluating on Panoptic, the largest multi-camera and markerless dataset available, we obtain an accuracy that is essentially indistinguishable from a strongly-supervised approach making full use of 3D groundtruth in training.
This paper presents the results of the First WMT Shared Task on Sign Language Translation (WMT-SLT22) 1. This shared task is concerned with automatic translation between signed and spoken 2 languages. The task is novel in the sense that it requires processing visual information (such as video frames or human pose estimation) beyond the wellknown paradigm of text-to-text machine translation (MT). The task featured two tracks, translating from Swiss German Sign Language (DSGS) to German and vice versa. Seven teams participated in this first edition of the task, all submitting to the DSGS-to-German track. Besides a system ranking and system papers describing state-of-the-art techniques, this shared task makes the following scientific contributions: novel corpora, reproducible baseline systems and new protocols and software for human evaluation. Finally, the task also resulted in the first publicly available set of system outputs and human evaluation scores for sign language translation.
This work addresses 3D human pose reconstruction in single images. We present a method that combines Forward Kinematics (FK) with neural networks to ensure a fast and valid prediction of 3D pose. Pose is represented as a hierarchical tree/graph with nodes corresponding to human joints that model their physical limits. Given a 2D detection of keypoints in the image, we lift the skeleton to 3D using neural networks to predict both the joint rotations and bone lengths. These predictions are then combined with skeletal constraints using an FK layer implemented as a network layer in Py-Torch. The result is a fast and accurate approach to the estimation of 3D skeletal pose. Through quantitative and qualitative evaluation , we demonstrate the method is significantly more accurate than MediaPipe in terms of both per joint positional error and visual appearance. Furthermore, we demonstrate generalization over different datasets and sign languages. The implementation in PyTorch runs at between 100-200 milliseconds per image (including CNN detection) using CPU only. Index Terms— 3D pose estimation, hand and body reconstruction .
Self-supervised monocular depth estimation (SS-MDE) has the potential to scale to vast quantities of data. Unfortunately, existing approaches limit themselves to the automotive domain, resulting in models incapable of generalizing to complex environments such as natural or indoor settings.To address this, we propose a large-scale SlowTV dataset curated from YouTube, containing an order of magnitude more data than existing automotive datasets. SlowTV contains 1.7M images from a rich diversity of environments, such as worldwide seasonal hiking, scenic driving and scuba diving. Using this dataset, we train an SS-MDE model that provides zero-shot generalization to a large collection of indoor/outdoor datasets. The resulting model outperforms all existing SSL approaches and closes the gap on supervised SoTA, despite using a more efficient architecture. We additionally introduce a collection of best-practices to further maximize performance and zero-shot generalization. This includes 1) aspect ratio augmentation, 2) camera intrinsic estimation, 3) support frame randomization and 4) flexible motion estimation.
We present a framework which allows standard stereo reconstruction to be unified with a wide range of classic top-down cues from urban scene understanding. The resulting algorithm is analogous to the human visual system where conflicting interpretations of the scene due to ambiguous data can be resolved based on a higher level understanding of urban environments. The cues which are reformulated within the framework include: recognising common arrangements of surface normals and semantic edges (e.g. concave, convex and occlusion boundaries), recognising connected or coplanar structures such as walls, and recognising collinear edges (which are common on repetitive structures such as windows). Recognition of these common configurations has only recently become feasible, thanks to the emergence of large-scale reconstruction datasets. To demonstrate the importance and generality of scene understanding during stereo-reconstruction, the proposed approach is integrated with 3 different state-of-the-art techniques for bottom-up stereo reconstruction. The use of high-level cues is shown to improve performance by up to 15 % on the Middlebury 2014 and KITTI datasets. We further evaluate the technique using the recently proposed HCI stereo metrics, finding significant improvements in the quality of depth discontinuities, planar surfaces and thin structures.
This paper deals with robust modelling of mouth shapes in the context of sign language recognition using deep convolutional neural networks. Sign language mouth shapes are difficult to annotate and thus hardly any publicly available annotations exist. As such, this work exploits related information sources as weak supervision. Humans mainly look at the face during sign language communication, where mouth shapes play an important role and constitute natural patterns with large variability. However, most scientific research on sign language recognition still disregards the face. Hardly any works explicitly focus on mouth shapes. This paper presents our advances in the field of sign language recognition. We contribute in following areas: We present a scheme to learn a convolutional neural network in a weakly supervised fashion without explicit frame labels. We propose a way to incorporate neural network classifier outputs into a HMM approach. Finally, we achieve a significant improvement in classification performance of mouth shapes over the current state of the art.
Imitation learning has been widely used to learn control policies for autonomous driving based on pre-recorded data. However, imitation learning based policies have been shown to be susceptible to compounding errors when encountering states outside of the training distribution. Further, these agents have been demonstrated to be easily exploitable by adversarial road users aiming to create collisions. To overcome these shortcomings, we introduce Adversarial Mixture Density Networks (AMDN), which learns two distributions from separate datasets. The first is a distribution of safe actions learned from a dataset of naturalistic human driving. The second is a distribution representing unsafe actions likely to lead to collision, learned from a dataset of collisions. During training, we leverage these two distributions to provide an additional loss based on the similarity of the two distributions. By penalising the safe action distribution based on its similarity to the unsafe action distribution when training on the collision dataset, a more robust and safe control policy is obtained. We demonstrate the proposed AMDN approach in a vehicle following use-case, and evaluate under naturalistic and adversarial testing environments. We show that despite its simplicity, AMDN provides significant benefits for the safety of the learned control policy, when compared to pure imitation learning or standard mixture density network approaches.
It is known that relative feature location is important in representing objects, but assumptions that make learning tractable often simplify how structure is encoded e.g. spatial pooling or star models. For example, techniques such as spatial pyramid matching (SPM), in-conjunction with machine learning techniques perform well [13]. However, there are limitations to such spatial encoding schemes which discard important information about the layout of features. In contrast, we propose to use the object itself to choose the basis of the features in an object centric approach. In doing so we return to the early work of geometric hashing [18] but demonstrate how such approaches can be scaled-up to modern day object detection challenges in terms of both the number of examples and their variability. We apply a two stage process, initially filtering background features to localise the objects and then hashing the remaining pairwise features in an affine invariant model. During learning, we identify class-wise key feature predictors. We validate our detection and classification of objects on the PASCAL VOC'07 and' 11 [6] and CarDb [21] datasets and compare with state of the art detectors and classifiers. Importantly we demonstrate how structure in features can be efficiently identified and how its inclusion can increase performance. This feature centric learning technique allows us to localise objects even without object annotation during training and the resultant segmentation provides accurate state of the art object localization, without the need for annotations.
Causal relationships can often be found in visual object tracking between the motions of the camera and that of the tracked object. This object motion may be an effect of the camera motion, e.g. an unsteady handheld camera. But it may also be the cause, e.g. the cameraman framing the object. In this paper we explore these relationships, and provide statistical tools to detect and quantify them, these are based on transfer entropy and stem from information theory. The relationships are then exploited to make predictions about the object location. The approach is shown to be an excellent measure for describing such relationships. On the VOT2013 dataset the prediction accuracy is increased by 62 % over the best non-causal predictor. We show that the location predictions are robust to camera shake and sudden motion, which is invaluable for any tracking algorithm and demonstrate this by applying causal prediction to two state-of-the-art trackers. Both of them benefit, Struck gaining a 7 % accuracy and 22 % robustness increase on the VTB1.1 benchmark, becoming the new state-of-the-art.
This paper proposes a clustered exemplar-based model for performing viewpoint invariant tracking of the 3D motion of a human subject from a single camera. Each exemplar is associated with multiple view visual information of a person and the corresponding 3D skeletal pose. The visual information takes the form of contours obtained from different viewpoints around the subject. The inclusion of multi-view information is important for two reasons: viewpoint invariance; and generalisation to novel motions. Visual tracking of human motion is performed using a particle filter coupled to the dynamics of human movement represented by the exemplar-based model. Dynamics are modelled by clustering 3D skeletal motions with similar movement and encoding the flow both within and between clusters. Results of single view tracking demonstrate that the exemplar-based models incorporating dynamics generalise to viewpoint invariant tracking of novel movements.
We set out a methodology for the automated generation of hidden Markov models (HMMs) of observed feature-space transitions in a noisy experimental environment that is maximally generalising under the assumed experimental constraints. Specifically, we provide an ICA-based feature-selection technique for determining the number, and the transition sequence of the underlying hidden states, along with the observed-state emission characteristics when the specified noise model assumptions are fulfilled. In retaining correlation information between features, the method is potentially more general than the commonly employed Gaussian mixture model HMM parameterisation methods, to which we demonstrate that our method reduces when an arbitrary separation of features, or an experimentally-limited feature-space is imposed. A practical demonstration of the application of this method to automated sign-language classification is given, for which we demonstrate that a performance improvement of the order of 40% over naive Markovian modelling of the observed transitions is possible.
Driving a car in an urban setting is an extremely difficult problem, incorporating a large number of complex visual tasks; however, this problem is solved daily by most adults with little apparent effort. This paper proposes a novel vision-based approach to autonomous driving that can predict and even anticipate a driver's behavior in real time, using preattentive vision only. Experiments on three large datasets totaling over 200 000 frames show that our preattentive model can (1) detect a wide range of driving-critical context such as crossroads, city center, and road type; however, more surprisingly, it can (2) detect the driver's actions (over 80% of braking and turning actions) and (3) estimate the driver's steering angle accurately. Additionally, our model is consistent with human data: First, the best steering prediction is obtained for a perception to action delay consistent with psychological experiments. Importantly, this prediction can be made before the driver's action. Second, the regions of the visual field used by the computational model strongly correlate with the driver's gaze locations, significantly outperforming many saliency measures and comparable to state-of-the-art approaches.
We present a novel approach to 3D reconstruction which is inspired by the human visual system. This system unifies standard appearance matching and triangulation techniques with higher level reasoning and scene understanding, in order to resolve ambiguities between different interpretations of the scene. The types of reasoning integrated in the approach includes recognising common configurations of surface normals and semantic edges (e.g. convex, concave and occlusion boundaries). We also recognise the coplanar, collinear and symmetric structures which are especially common in man made environments.
This paper proposes a learnt data-driven approach for accurate, real-time tracking of facial features using only intensity information. Constraints such as a-priori shape models or temporal models for dynamics are not required or used. Tracking facial features simply becomes the independent tracking of a set of points on the face. This allows us to cope with facial configurations not present in the training data. Tracking is achieved via linear predictors which provide a fast and effective method for mapping pixel-level information to tracked feature position displacements. To improve on this, a novel and robust biased linear predictor is proposed in this paper. Multiple linear predictors are grouped into a rigid flock to increase robustness. To further improve tracking accuracy, a novel probabilistic selection method is used to identify relevant visual areas for tracking a feature point. These selected flocks are then combined into a hierarchical multi-resolution LP model. Experimental results also show that this method performs more robustly and accurately than AAMs, without any a priori shape information and with minimal training examples.
Action recognition “in the wild” is extremely challenging, particularly when complex 3D actions are projected down to the image plane, losing a great deal of information. The recent growth of 3D data in broadcast content and commercial depth sensors, makes it possible to overcome this. However, there is little work examining the best way to exploit this new modality. In this paper we introduce the Hollywood 3D benchmark, which is the first dataset containing “in the wild” action footage including 3D data. This dataset consists of 650 stereo video clips across 14 action classes, taken from Hollywood movies. We provide stereo calibrations and depth reconstructions for each clip. We also provide an action recognition pipeline, and propose a number of specialised depth-aware techniques including five interest point detectors and three feature descriptors. Extensive tests allow evaluation of different appearance and depth encoding schemes. Our novel techniques exploiting this depth allow us to reach performance levels more than triple those of the best baseline algorithm using only appearance information. The benchmark data, code and calibrations are all made available to the community.
We introduce a new algorithm for real-time interactive motion control and demonstrate its application to motion captured data, prerecorded videos, and HCI. Firstly, a data set of frames are projected into a lower dimensional space. An appearance model is learnt using a multivariate probability distribution. A novel approach to determining transition points is presented based on k-medoids, whereby appropriate points of intersection in the motion trajectory are derived as cluster centers. These points are used to segment the data into smaller subsequences. A transition matrix combined with a kernel density estimation is used to determine suitable transitions between the subsequences to develop novel motion. To facilitate real-time interactive control, conditional probabilities are used to derive motion given user commands. The user commands can come from any modality including auditory, touch, and gesture. The system is also extended to HCI using audio signals of speech in a conversation to trigger nonverbal responses from a synthetic listener in real-time. We demonstrate the flexibility of the model by presenting results ranging from data sets composed of vectorized images, 2-D, and 3-D point representations. Results show real-time interaction and plausible motion generation between different types of movement.
The use of human-level semantic information to aid robotic tasks has recently become an important area for both Computer Vision and Robotics. This has been enabled by advances in Deep Learning that allow consistent and robust semantic understanding. Leveraging this semantic vision of the world has allowed human-level understanding to naturally emerge from many different approaches. Particularly, the use of semantic information to aid in localisation and reconstruction has been at the forefront of both fields. Like robots, humans also require the ability to localise within a structure. To aid this, humans have designed highlevel semantic maps of our structures called floorplans. We are extremely good at localising in them, even with limited access to the depth information used by robots. This is because we focus on the distribution of semantic elements, rather than geometric ones. Evidence of this is that humans are normally able to localise in a floorplan that has not been scaled properly. In order to grant this ability to robots, it is necessary to use localisation approaches that leverage the same semantic information humans use. In this paper, we present a novel method for semantically enabled global localisation. Our approach relies on the semantic labels present in the floorplan. Deep Learning is leveraged to extract semantic labels from RGB images, which are compared to the floorplan for localisation. While our approach is able to use range measurements if available, we demonstrate that they are unnecessary as we can achieve results comparable to state-of-the-art without them.
We present a novel approach to automatic Sign Language Production using recent developments in Neural Machine Translation (NMT), Generative Adversarial Networks, and motion generation. Our system is capable of producing sign videos from spoken language sentences. Contrary to current approaches that are dependent on heavily annotated data, our approach requires minimal gloss and skeletal level annotations for training. We achieve this by breaking down the task into dedicated sub-processes. We first translate spoken language sentences into sign pose sequences by combining an NMT network with a Motion Graph. The resulting pose information is then used to condition a generative model that produces photo realistic sign language video sequences. This is the first approach to continuous sign video generation that does not use a classical graphical avatar. We evaluate the translation abilities of our approach on the PHOENIX14T Sign Language Translation dataset. We set a baseline for text-to-gloss translation, reporting a BLEU-4 score of 16.34/15.26 on dev/test sets. We further demonstrate the video generation capabilities of our approach for both multi-signer and high-definition settings qualitatively and quantitatively using broadcast quality assessment metrics.
This paper presents a model based approach to human body tracking in which the 2D silhouette of a moving human and the corresponding 3D skeletal structure are encapsulated within a non-linear point distribution model. This statistical model allows a direct mapping to be achieved between the external boundary of a human and the anatomical position. It is shown how this information, along with the position of landmark features such as the hands and head can be used to reconstruct information about the pose and structure of the human body from a monocular view of a scene.
Visual tracking has attracted a significant attention in the last few decades. The recent surge in the number of publications on tracking-related problems have made it almost impossible to follow the developments in the field. One of the reasons is that there is a lack of commonly accepted annotated data-sets and standardized evaluation protocols that would allow objective comparison of different tracking methods. To address this issue, the Visual Object Tracking (VOT) workshop was organized in conjunction with ICCV2013. Researchers from academia as well as industry were invited to participate in the first VOT2013 challenge which aimed at single-object visual trackers that do not apply pre-learned models of object appearance (model-free). Presented here is the VOT2013 benchmark dataset for evaluation of single-object visual trackers as well as the results obtained by the trackers competing in the challenge. In contrast to related attempts in tracker benchmarking, the dataset is labeled per-frame by visual attributes that indicate occlusion, illumination change, motion change, size change and camera motion, offering a more systematic comparison of the trackers. Furthermore, we have designed an automated system for performing and evaluating the experiments. We present the evaluation protocol of the VOT2013 challenge and the results of a comparison of 27 trackers on the benchmark dataset. The dataset, the evaluation tools and the tracker rankings are publicly available from the challenge website (http://votchallenge. net)
This paper proposes a learnt {____em data-driven} approach for accurate, real-time tracking of facial features using only intensity information, a non-trivial task since the face is a highly deformable object with large textural variations and motion in certain regions. The framework proposed here largely avoids the need for apriori design of feature trackers by automatically identifying the optimal visual support required for tracking a single facial feature point. This is essentially equivalent to automatically determining the visual context required for tracking. Tracking is achieved via biased linear predictors which provide a fast and effective method for mapping pixel-intensities into tracked feature position displacements. Multiple linear predictors are grouped into a rigid flock to increase robustness. To further improve tracking accuracy, a novel probabilistic selection method is used to identify relevant visual areas for tracking a feature point. These selected flocks are then combined into a hierarchical multi-resolution LP model. Finally, we also exploit a simple shape constraint for correcting the occasional tracking failure of a minority of feature points. Experimental results also show that this method performs more robustly and accurately than AAMs, on example sequences that range from SD quality to Youtube quality.
Recently, Nonparametric (NP) Windows has been proposed to estimate the statistics of real 1D and 2D signals. NP Windows is accurate because it is equivalent to sampling images at a high (infinite) resolution for an assumed interpolation model. This paper extends the proposed approach to consider joint distributions of image pairs. Second, Green's Theorem is used to simplify the previous NP Windows algorithm. Finally, a resolution-aware NP Windows algorithm is proposed to improve robustness to relative scaling between an image pair. Comparative testing of 2D image registration was performed using translation only and affine transformations. Although it is more expensive than other methods, NP Windows frequently demonstrated superior performance for bias (distance between ground truth and global maximum) and frequency of convergence. Unlike other methods, the number of samples and the number of bins have little effect on NP Windows and the prior selection of a kernel is not required.
Research into facial expression recognition has predominantly been applied to face images at frontal view only. Some attempts have been made to produce pose invariant facial expression classifiers. However, most of these attempts have only considered yaw variations of up to 45°, where all of the face is visible. Little work has been carried out to investigate the intrinsic potential of different poses for facial expression recognition. This is largely due to the databases available, which typically capture frontal view face images only. Recent databases, BU3DFE and multi-pie, allows empirical investigation of facialexpressionrecognition for different viewing angles. A sequential 2 stage approach is taken for pose classification and view dependent facialexpression classification to investigate the effects of yaw variations from frontal to profile views. Local binary patterns (LBPs) and variations of LBPs as texture descriptors are investigated. Such features allow investigation of the influence of orientation and multi-resolution analysis for multi-view facial expression recognition. The influence of pose on different facial expressions is investigated. Others factors are investigated including resolution and construction of global and local feature vectors. An appearance based approach is adopted by dividing images into sub-blocks coarsely aligned over the face. Feature vectors contain concatenated feature histograms built from each sub-block. Multi-class support vector machines are adopted to learn pose and pose dependent facial expression classifiers.
This paper presents an approach to hand pose estimation that combines discriminative and model-based methods to leverage the advantages of both. Randomised Decision Forests are trained using real data to provide fast coarse segmentation of the hand. The segmentation then forms the basis of constraints applied in model fitting, using an efficient projected Gauss-Seidel solver, which enforces temporal continuity and kinematic limitations. However, when fitting a generic model to multiple users with varying hand shape, there is likely to be residual errors between the model and their hand. Also, local minima can lead to failures in tracking that are difficult to recover from. Therefore, we introduce an error regression stage that learns to correct these instances of optimisation failure. The approach provides improved accuracy over the current state of the art methods, through the inclusion of temporal cohesion and by learning to correct from failure cases. Using discriminative learning, our approach performs guided optimisation, greatly reducing model fitting complexity and radically improves efficiency. This allows tracking to be performed at over 40 frames per second using a single CPU thread.
A vision system is described which performs real-time inspection of dry carbon-fibre preforms during lay-up, the first stage in resin transfer moulding (RTM). The position of ply edges on the preform is determined in a two-stage process. Firstly, an optimized texture analysis method is used to estimate the approximate ply edge position. Secondly, boundary refinement is carried out using the texture estimate as a guiding template. Each potential edge point is evaluated using a merit function of edge magnitude, orientation and distance from the texture boundary estimate. The parameters of the merit function must be obtained by training on sample images. Once trained, the system has been shown to be accurate to better than ±1 pixel when used in conjunction with boundary models. Processing time is less than 1 s per image using commercially available convolution hardware. The system has been demonstrated in a prototype automated lay-up cell and used in a large number of manufacturing trials.
In this work we present a new approach to the field of weakly supervised learning in the video domain. Our method is relevant to sequence learning problems which can be split up into sub-problems that occur in parallel. Here, we experiment with sign language data. The approach exploits sequence constraints within each independent stream and combines them by explicitly imposing synchronisation points to make use of parallelism that all sub-problems share. We do this with multi-stream HMMs while adding intermediate synchronisation constraints among the streams. We embed powerful CNN-LSTM models in each HMM stream following the hybrid approach. This allows the discovery of attributes which on their own lack sufficient discriminative power to be identified. We apply the approach to the domain of sign language recognition exploiting the sequential parallelism to learn sign language, mouth shape and hand shape classifiers. We evaluate the classifiers on three publicly available benchmark data sets featuring challenging real-life sign language with over 1000 classes, full sentence based lip-reading and articulated hand shape recognition on a fine-grained hand shape taxonomy featuring over 60 different hand shapes. We clearly outperform the state-of-the-art on all data sets and observe significantly faster convergence using the parallel alignment approach.
In this paper, an algorithm is presented for estimating scene flow, which is a richer, 3D analogue of Optical Flow. The approach operates orders of magnitude faster than alternative techniques, and is well suited to further performance gains through parallelized implementation. The algorithm employs multiple hypothesis to deal with motion ambiguities, rather than the traditional smoothness constraints, removing oversmoothing errors and providing signi?cant performance improvements on benchmark data, over the previous state of the art. The approach is ?exible, and capable of operating with any combination of appearance and/or depth sensors, in any setup, simultaneously estimating the structure and motion if necessary. Additionally, the algorithm propagates information over time to resolve ambiguities, rather than performing an isolated estimation at each frame, as in contemporary approaches. Approaches to smoothing the motion ?eld without sacri?cing the bene?ts of multiple hypotheses are explored, and a probabilistic approach to Occlusion estimation is demonstrated, leading to 10% and 15% improved performance respectively. Finally, a data driven tracking approach is described, and used to estimate the 3D trajectories of hands during sign language, without the need to model complex appearance variations at each viewpoint.
There is a clear trend in the use of robots to accomplish services that can help humans. In this paper, robots acting in urban environments are considered for the task of person guiding. Nowadays, it is common to have ubiquitous sensors integrated within the buildings, such as camera networks, and wireless communications like 3G or WiFi. Such infrastructure can be directly used by robotic platforms. The paper shows how combining the information from the robots and the sensors allows tracking failures to be overcome, by being more robust under occlusion, clutter, and lighting changes. The paper describes the algorithms for tracking with a set of fixed surveillance cameras and the algorithms for position tracking using the signal strength received by a wireless sensor network (WSN). Moreover, an algorithm to obtain estimations on the positions of people from cameras on board robots is described. The estimate from all these sources are then combined using a decentralized data fusion algorithm to provide an increase in performance. This scheme is scalable and can handle communication latencies and failures. We present results of the system operating in real time on a large outdoor environment, including 22 nonoverlapping cameras,WSN, and several robots. © Institut Mines-Télécom and Springer-Verlag 2012.
This chapter discusses sign language recognition using linguistic sub-units. It presents three types of sub-units for consideration; those learnt from appearance data as well as those inferred from both 2D or 3D tracking data. These sub-units are then combined using a sign level classifier; here, two options are presented. The first uses Markov Models to encode the temporal changes between sub-units. The second makes use of Sequential Pattern Boosting to apply discriminative feature selection at the same time as encoding temporal information. This approach is more robust to noise and performs well in signer independent tests, improving results from the 54% achieved by the Markov Chains to 76%.
© Springer International Publishing Switzerland 2015. In recent years, dense trajectories have shown to be an efficient representation for action recognition and have achieved state-of-the art results on a variety of increasingly difficult datasets. However, while the features have greatly improved the recognition scores, the training process and machine learning used hasn’t in general deviated from the object recognition based SVM approach. This is despite the increase in quantity and complexity of the features used. This paper improves the performance of action recognition through two data mining techniques, APriori association rule mining and Contrast Set Mining. These techniques are ideally suited to action recognition and in particular, dense trajectory features as they can utilise the large amounts of data, to identify far shorter discriminative subsets of features called rules. Experimental results on one of the most challenging datasets, Hollywood2 outperforms the current state-of-the-art.
The field of Action Recognition has seen a large increase in activity in recent years. Much of the progress has been through incorporating ideas from single-frame object recognition and adapting them for temporal-based action recognition. Inspired by the success of interest points in the 2D spatial domain, their 3D (space-time) counterparts typically form the basic components used to describe actions, and in action recognition the features used are often engineered to fire sparsely. This is to ensure that the problem is tractable; however, this can sacrifice recognition accuracy as it cannot be assumed that the optimum features in terms of class discrimination are obtained from this approach. In contrast, we propose to initially use an overcomplete set of simple 2D corners in both space and time. These are grouped spatially and temporally using a hierarchical process, with an increasing search area. At each stage of the hierarchy, the most distinctive and descriptive features are learned efficiently through data mining. This allows large amounts of data to be searched for frequently reoccurring patterns of features. At each level of the hierarchy, the mined compound features become more complex, discriminative, and sparse. This results in fast, accurate recognition with real-time performance on high-resolution video. As the compound features are constructed and selected based upon their ability to discriminate, their speed and accuracy increase at each level of the hierarchy. The approach is tested on four state-of-the-art data sets, the popular KTH data set to provide a comparison with other state-of-the-art approaches, the Multi-KTH data set to illustrate performance at simultaneous multiaction classification, despite no explicit localization information provided during training. Finally, the recent Hollywood and Hollywood2 data sets provide challenging complex actions taken from commercial movie sequences. For all four data sets, the proposed hierarchical approa- h outperforms all other methods reported thus far in the literature and can achieve real-time operation.
Significant effort has been devoted within the visual tracking community to rapid learning of object properties on the fly. However, state-of-the-art approaches still often fail in cases such as rapid out-of-plane rotation, when the appearance changes suddenly. One of the major contributions of this work is a radical rethinking of the traditional wisdom of modelling 3D motion as appearance change during tracking. Instead, 3D motion is modelled as 3D motion. This intuitive but previously unexplored approach provides new possibilities in visual tracking research. Firstly, 3D tracking is more general, as large out-of-plane motion is often fatal for 2D trackers, but helps 3D trackers to build better models. Secondly, the tracker’s internal model of the object can be used in many different applications and it could even become the main motivation, with tracking supporting reconstruction rather than vice versa. This effectively bridges the gap between visual tracking and Structure from Motion. A new benchmark dataset of sequences with extreme out-ofplane rotation is presented and an online leader-board offered to stimulate new research in the relatively underdeveloped area of 3D tracking. The proposed method, provided as a baseline, is capable of successfully tracking these sequences, all of which pose a considerable challenge to 2D trackers (error reduced by 46 %).
This manuscript introduces the end-to-end embedding of a CNN into a HMM, while interpreting the outputs of the CNN in a Bayesian framework. The hybrid CNNHMM combines the strong discriminative abilities of CNNs with the sequence modelling capabilities of HMMs. Most current approaches in the field of gesture and sign language recognition disregard the necessity of dealing with sequence data both for training and evaluation. With our presented end-to-end embedding we are able to improve over the state-of-the-art on three challenging benchmark continuous sign language recognition tasks by between 15% and 38% relative reduction in word error rate and up to 20% absolute. We analyse the effect of the CNN structure, network pretraining and number of hidden states. We compare the hybrid modelling to a tandem approach and evaluate the gain of model combination.
Long term tracking of an object, given only a single instance in an initial frame, remains an open problem. We propose a visual tracking algorithm, robust to many of the difficulties which often occur in real-world scenes. Correspondences of edge-based features are used, to overcome the reliance on the texture of the tracked object and improve invariance to lighting. Furthermore we address long-term stability, enabling the tracker to recover from drift and to provide redetection following object disappearance or occlusion. The two-module principle is similar to the successful state-of-the-art long-term TLD tracker, however our approach offers better performance in benchmarks and extends to cases of low-textured objects. This becomes obvious in cases of plain objects with no texture at all, where the edge-based approach proves the most beneficial. We perform several different experiments to validate the proposed method. Firstly, results on short-term sequences show the performance of tracking challenging (low-textured and/or transparent) objects which represent failure cases for competing state-of-the-art approaches. Secondly, long sequences are tracked, including one of almost 30 000 frames which to our knowledge is the longest tracking sequence reported to date. This tests the redetection and drift resistance properties of the tracker. Finally, we report results of the proposed tracker on the VOT Challenge 2013 and 2014 datasets as well as on the VTB1.0 benchmark and we show relative performance of the tracker compared to its competitors. All the results are comparable to the state-ofthe-art on sequences with textured objects and superior on nontextured objects. The new annotated sequences are made publicly available.
"Actions in the wild" is the term given to examples of human motion that are performed in natural settings, such as those harvested from movies [1] or Internet databases [2]. This paper presents an approach to the categorisation of such activity in video, which is based solely on the relative distribution of spatio-temporal interest points. Presenting the Relative Motion Descriptor, we show that the distribution of interest points alone (without explicitly encoding their neighbourhoods) effectively describes actions. Furthermore, given the huge variability of examples within action classes in natural settings, we propose to further improve recognition by automatically detecting outliers, and breaking complex action categories into multiple modes. This is achieved using a variant of Random Sampling Consensus (RANSAC), which identifies and separates the modes. We employ a novel reweighting scheme within the RANSAC procedure to iteratively reweight training examples, ensuring their inclusion in the final classification model. We demonstrate state-of-the-art performance on five human action datasets. © 2014 Elsevier Inc. All rights reserved.
This paper proposes a Hybrid Approximate Representation (HAR) based on unifying several efficient approximations of the generalized reprojection error (which is known as the gold standard for multiview geometry). The HAR is an over-parameterization scheme where the approximation is applied simultaneously in multiple parameter spaces. A joint minimization scheme “HAR-Descent” can then solve the PnP problem efficiently, while remaining robust to approximation errors and local minima. The technique is evaluated extensively, including numerous synthetic benchmark protocols and the real-world data evaluations used in previous works. The proposed technique was found to have runtime complexity comparable to the fastest O(n) techniques, and up to 10 times faster than current state of the art minimization approaches. In addition, the accuracy exceeds that of all 9 previous techniques tested, providing definitive state of the art performance on the benchmarks, across all 90 of the experiments in the paper and supplementary material.
In the field of computer vision, principle component analysis (PCA) is often used to provide statistical models of shape, deformation or appearance. This simple statistical model provides a constrained, compact approach to model based vision. However. As larger problems are considered, high dimensionality and nonlinearity make linear PCA an unsuitable and unreliable approach. A nonlinear PCA (NLPCA) technique is proposed which uses cluster analysis and dimensional reduction to provide a fast, robust solution. Simulation results on both 2D contour models and greyscale images are presented.
Within the eld of image and video recognition, the traditional approach is a dataset split into xed training and test partitions. However, the labelling of the training set is time-consuming, especially as datasets grow in size and complexity. Furthermore, this approach is not applicable to the home user, who wants to intuitively group their media without tirelessly labelling the content. Consequently, we propose a solution similar in nature to an active learning paradigm, where a small subset of media is labelled as semantically belonging to the same class, and machine learning is then used to pull this and other related content together in the feature space. Our interactive approach is able to iteratively cluster classes of images and video. We reformulate it in an online learning framework and demonstrate competitive performance to batch learning approaches using only a fraction of the labelled data. Our approach is based around the concept of an image signature which, unlike a standard bag of words model, can express co-occurrence statistics as well as symbol frequency. We e ciently compute metric distances between signatures despite their inherent high dimensionality and provide discriminative feature selection, to allow common and distinctive elements to be identi ed from a small set of user labelled examples. These elements are then accentuated in the image signature to increase similarity between examples and pull correct classes together. By repeating this process in an online learning framework, the accuracy of similarity increases dramatically despite labelling only a few training examples. To demonstrate that the approach is agnostic to media type and features used, we evaluate on three image datasets (15 scene, Caltech101 and FG-NET), a mixed text and image dataset (ImageTag), a dataset used in active learning (Iris) and on three action recognition datasets (UCF11, KTH and Hollywood2). On the UCF11 video dataset, the accuracy is 86.7% despite using only 90 labelled examples from a dataset of over 1200 videos, instead of the standard 1122 training videos. The approach is both scalable and e cient, with a single iteration over the full UCF11 dataset of around 1200 videos taking approximately 1 minute on a standard desktop machine.
We approach instantaneous mapping, converting images to a top-down view of the world, as a translation problem. We show how a novel form of transformer network can be used to map from images and video directly to an overhead map or bird's-eye-view (BEV) of the world, in a single end-to-end network. We assume a 1-1 correspondence between a vertical scanline in the image, and rays passing through the camera location in an overhead map. This lets us formulate map generation from an image as a set of sequence-to-sequence translations. This constrained formulation , based upon a strong physical grounding of the problem, leads to a restricted transformer network that is convolutional in the horizontal direction only. The structure allows us to make efficient use of data when training, and obtains state-of-the-art results for instantaneous mapping of three large-scale datasets, including a 15% and 30% relative gain against existing best performing methods on the nuScenes and Argoverse datasets, respectively.
Hand pose estimation from a single image has many applications. However, approaches to full 3D body pose estimation are typically trained on day-to-day activities or actions. As such, detailed hand-to-hand interactions are poorly represented, especially during motion. We see this in the failure cases of techniques such as OpenPose [6] or MediaPipe[30]. However, accurate hand pose estimation is crucial for many applications where the global body motion is less important than accurate hand pose estimation. This paper addresses the problem of 3D hand pose estimation from monocular images or sequences. We present a novel end-to-end framework for 3D hand regression that employs diffusion models that have shown excellent ability to capture the distribution of data for generative purposes. Moreover, we enforce kinematic constraints to ensure realistic poses are generated by incorporating an explicit forward kinematic layer as part of the network. The proposed model provides state-of-the-art performance when lifting a 2D single-hand image to 3D. However, when sequence data is available, we add a Transformer module over a temporal window of consecutive frames to refine the results, overcoming jittering and further increasing accuracy. The method is quantitatively and qualitatively evaluated showing state-of-the-art robustness, generalization, and accuracy on several different datasets.
Graph convolutional networks (GCNs) enable end-to-end learning on graph structured data. However, many works assume a given graph structure. When the input graph is noisy or unavailable, one approach is to construct or learn a latent graph structure. These methods typically fix the choice of node degree for the entire graph, which is suboptimal. Instead, we propose a novel end-to-end differentiable graph generator which builds graph topologies where each node selects both its neighborhood and its size. Our module can be readily integrated into existing pipelines involving graph convolution operations, replacing the predetermined or existing adjacency matrix with one that is learned, and optimized, as part of the general objective. As such it is applicable to any GCN. We integrate our module into trajectory prediction, point cloud classification and node classification pipelines resulting in improved accuracy over other structure-learning methods across a wide range of datasets and GCN backbones. We will release the code.
Sign Language Translation (SLT) is a challenging task that aims to generate spoken language sentences from sign language videos, both of which have different grammar and word/gloss order. From a Neural Machine Translation (NMT) perspective, the straightforward way of training translation models is to use sign language phrase-spoken language sentence pairs. However, human interpreters heavily rely on the context to understand the conveyed information , especially for sign language interpretation, where the vocabulary size may be significantly smaller than their spoken language equivalent. Taking direct inspiration from how humans translate, we propose a novel multi-modal transformer architecture that tackles the translation task in a context-aware manner, as a human would. We use the context from previous sequences and confident predictions to disambiguate weaker visual cues. To achieve this we use complementary transformer encoders, namely: (1) A Video Encoder, that captures the low-level video features at the frame-level, (2) A Spotting Encoder, that models the recognized sign glosses in the video, and (3) A Context Encoder, which captures the context of the preceding sign sequences. We combine the information coming from these encoders in a final transformer decoder to generate spoken language translations. We evaluate our approach on the recently published large-scale BOBSL dataset, which contains ∼1.2M sequences , and on the SRF dataset, which was part of the WMT-SLT 2022 challenge. We report significant improvements on state-of-the-art translation performance using contextual information, nearly doubling the reported BLEU-4 scores of baseline approaches.
In natural language processing (NLP) of spoken languages , word embeddings have been shown to be a useful method to encode the meaning of words. Sign languages are visual languages, which require sign embeddings to capture the visual and linguistic semantics of sign. Unlike many common approaches to Sign Recognition, we focus on explicitly creating sign embeddings that bridge the gap between sign language and spoken language. We propose a learning framework to derive LCC (Learnt Con-trastive Concept) embeddings for sign language, a weakly supervised contrastive approach to learning sign embed-dings. We train a vocabulary of embeddings that are based on the linguistic labels for sign video. Additionally, we develop a conceptual similarity loss which is able to utilise word embeddings from NLP methods to create sign embed-dings that have better sign language to spoken language correspondence. These learnt representations allow the model to automatically localise the sign in time. Our approach achieves state-of-the-art keypoint-based sign recognition performance on the WLASL and BOBSL datasets.
Recent approaches to multi-task learning (MTL) have fo-cused on modelling connections between tasks at the de-coder level. This leads to a tight coupling between tasks, which need retraining if a new task is inserted or removed. We argue that MTL is a stepping stone towards universal feature learning (UFL), which is the ability to learn generic features that can be applied to new tasks without retraining. We propose Medusa to realize this goal, designing task heads with dual attention mechanisms. The shared feature attention masks relevant backbone features for each task, allowing it to learn a generic representation. Meanwhile, a novel Multi-Scale Attention head allows the network to better combine per-task features from different scales when making the final prediction. We show the effectiveness of Medusa in UFL (+13.18% improvement), while maintaining MTL performance and being 25% more efficient than previous approaches.
In the current monocular depth research, the dominant approach is to employ unsupervised training on large datasets, driven by warped photometric consistency. Such approaches lack robustness and are unable to generalize to challenging domains such as nighttime scenes or adverse weather conditions where assumptions about photometric consistency break down. We propose DeFeat-Net (Depth & Feature network), an approach to simultaneously learn a cross-domain dense feature representation, alongside a robust depth-estimation framework based on warped feature consistency. The resulting feature representation is learned in an unsupervised manner with no explicit ground-truth correspondences required. We show that within a single domain, our technique is comparable to both the current state of the art in monocular depth estimation and supervised feature representation learning. However, by simultaneously learning features, depth and motion, our technique is able to generalize to challenging domains, allowing DeFeat-Net to outperform the current state-of-the-art with around 10% reduction in all error measures on more challenging sequences such as nighttime driving.
“Like night and day” is a commonly used expression to imply that two things are completely different. Unfortunately, this tends to be the case for current visual feature representations of the same scene across varying seasons or times of day. The aim of this paper is to provide a dense feature representation that can be used to perform localization, sparse matching or image retrieval,regardless of the current seasonal or temporal appearance. Recently, there have been several proposed methodologies for deep learning dense feature representations. These methods make use of ground truth pixel-wise correspondences between pairs of images and focus on the spatial properties of the features. As such, they don’t address temporal or seasonal variation. Furthermore, obtaining the required pixel-wise correspondence data to train in cross-seasonal environments is highly complex in most scenarios. We propose Deja-Vu, a weakly supervised approach to learning season invariant features that does not require pixel-wise ground truth data. The proposed system only requires coarse labels indicating if two images correspond to the same location or not. From these labels, the network is trained to produce “similar” dense feature maps for corresponding locations despite environmental changes. Code will be made available at: https://github.com/ jspenmar/DejaVu_Features
How many times does a human have to drive through the same area to become familiar with it? To begin with, we might first build a mental model of our surroundings. Upon revisiting this area, we can use this model to extrapolate to new unseen locations and imagine their appearance. Based on this, we propose an approach where an agent is capable of modelling new environments after a single visitation. To this end, we introduce “Deep Imagination”, a combination of classical Visual-based Monte Carlo Localisation and deep learning. By making use of a feature embedded 3D map, the system can “imagine” the view from any novel location. These “imagined” views are contrasted with the current observation in order to estimate the agent’s current location. In order to build the embedded map, we train a deep Siamese Fully Convolutional U-Net to perform dense feature extraction. By training these features to be generic, no additional training or fine tuning is required to adapt to new environments. Our results demonstrate the generality and transfer capability of our learnt dense features by training and evaluating on multiple datasets. Additionally, we include several visualizations of the feature representations and resulting 3D maps, as well as their application to localisation.
How do computers and intelligent agents view the world around them? Feature extraction and representation constitutes one the basic building blocks towards answering this question. Traditionally, this has been done with carefully engineered hand-crafted techniques such as HOG, SIFT or ORB. However, there is no “one size fits all” approach that satisfies all requirements. In recent years, the rising popularity of deep learning has resulted in a myriad of end-to-end solutions to many computer vision problems. These approaches, while successful, tend to lack scalability and can’t easily exploit information learned by other systems. Instead, we propose SAND features, a dedicated deep learning solution to feature extraction capable of providing hierarchical context information. This is achieved by employing sparse relative labels indicating relationships of similarity/dissimilarity between image locations. The nature of these labels results in an almost infinite set of dissimilar examples to choose from. We demonstrate how the selection of negative examples during training can be used to modify the feature space and vary it’s properties. To demonstrate the generality of this approach, we apply the proposed features to a multitude of tasks, each requiring different properties. This includes disparity estimation, semantic segmentation, self-localisation and SLAM. In all cases, we show how incorporating SAND features results in better or comparable results to the baseline, whilst requiring little to no additional training. Code can be found at: https://github.com/jspenmar/SAND_features
How many times does a human have to drive through the same area to become familiar with it? To begin with, we might first build a mental model of our surroundings. Upon revisiting this area, we can use this model to extrapolate to new unseen locations and imagine their appearance. Based on this, we propose an approach where an agent is capable of modelling new environments after a single visitation. To this end, we introduce "Deep Imagination", a combination of classical Visual-based Monte Carlo Localisation and deep learning. By making use of a feature embedded 3D map, the system can "imagine" the view from any novel location. These "imagined" views are contrasted with the current observation in order to estimate the agent's current location. In order to build the embedded map, we train a deep Siamese Fully Convolutional U-Net to perform dense feature extraction. By training these features to be generic, no additional training or fine tuning is required to adapt to new environments. Our results demonstrate the generality and transfer capability of our learnt dense features by training and evaluating on multiple datasets. Additionally, we include several visualizations of the feature representations and resulting 3D maps, as well as their application to localisation.
How do computers and intelligent agents view the world around them? Feature extraction and representation constitutes one the basic building blocks towards answering this question. Traditionally, this has been done with carefully engineered hand-crafted techniques such as HOG, SIFT or ORB. However, there is no "one size fits all" approach that satisfies all requirements. In recent years, the rising popularity of deep learning has resulted in a myriad of end-to-end solutions to many computer vision problems. These approaches, while successful, tend to lack scalability and can't easily exploit information learned by other systems. Instead, we propose SAND features, a dedicated deep learning solution to feature extraction capable of providing hierarchical context information. This is achieved by employing sparse relative labels indicating relationships of similarity/dissimilarity between image locations. The nature of these labels results in an almost infinite set of dissimilar examples to choose from. We demonstrate how the selection of negative examples during training can be used to modify the feature space and vary it's properties. To demonstrate the generality of this approach, we apply the proposed features to a multitude of tasks, each requiring different properties. This includes disparity estimation, semantic segmentation, self-localisation and SLAM. In all cases, we show how incorporating SAND features results in better or comparable results to the baseline, whilst requiring little to no additional training. Code can be found at:https:github.com.jspenmar/SAND_features
Computational sign language research lacks the large-scale datasets that enables the creation of useful real-life applications. To date, most research has been limited to prototype systems on small domains of discourse, e.g. weather forecasts. To address this issue and to push the field forward, we release six datasets comprised of 190 hours of footage on the larger domain of news. From this, 20 hours of footage have been annotated by Deaf experts and interpreters and is made publicly available for research purposes. In this paper, we share the dataset collection process and tools developed to enable the alignment of sign language video and subtitles, as well as baseline translation results to underpin future research.
Constructing Birds-Eye-View (BEV) maps from monocular images is typically a complex multi-stage process involving the separate vision tasks of ground plane estimation, road segmentation and 3D object detection. However, recent approaches have adopted end-to-end solutions which warp image-based features from the image-plane to BEV while implicitly taking account of camera geometry. In this work, we show how such instantaneous BEV estimation of a scene can be learnt, and a better state estimation of the world can be achieved by incorporating temporal information. Our model learns a representation from monocular video through factorised 3D convolutions and uses this to estimate a BEV occupancy grid of the final frame. We achieve state-of-the-art results for BEV estimation from monocular images, and establish a new benchmark for single-scene BEV estimation from monocular video.
This paper presents a real time approach to locate and track the upper torso of the human body. Our main interest is not in 3D biometric accuracy, but rather a sufficient discriminatory representation for visual interaction. The algorithm employs background suppression and a general approximation to body shape, applied within a particle filter framework, making use of integral images to maintain real-time performance. Furthermore, we present a novel method to disambiguate the hands of the subject and to predict the likely position of elbows. The final system is demonstrated segmenting multiple subjects from a cluttered scene at above real time operation.
Deep learning is a promising class of techniques for controlling an autonomous vehicle. However, functional safety validation is seen as a critical issue for these systems due to the lack of transparency in deep neural networks and the safety-critical nature of autonomous vehicles. The black box nature of deep neural networks limits the effectiveness of traditional verification and validation methods. In this paper, we propose two software safety cages, which aim to limit the control action of the neural network to a safe operational envelope. The safety cages impose limits on the control action during critical scenarios, which if breached, change the control action to a more conservative value. This has the benefit that the behaviour of the safety cages is interpretable, and therefore traditional functional safety validation techniques can be applied. The work here presents a deep neural network trained for longitudinal vehicle control, with safety cages designed to prevent forward collisions. Simulated testing in critical scenarios shows the effectiveness of the safety cages in preventing forward collisions whilst under normal highway driving unnecessary interruptions are eliminated, and the deep learning control policy is able to perform unhindered. Interventions by the safety cages are also used to re-train the network, resulting in a more robust control policy.
We propose a novel deep learning approach to solve simultaneous alignment and recognition problems (referred to as "Sequence-to-sequence" learning). We decompose the problem into a series of specialised expert systems referred to as SubUNets. The spatio-temporal relationships between these SubUNets are then modelled to solve the task, while remaining trainable end-to-end. The approach mimics human learning and educational techniques, and has a number of significant advantages. SubUNets allow us to inject domain-specific expert knowledge into the system regarding suitable intermediate representations. They also allow us to implicitly perform transfer learning between different interrelated tasks, which also allows us to exploit a wider range of more varied data sources. In our experiments we demonstrate that each of these properties serves to significantly improve the performance of the overarching recognition system, by better constraining the learning problem. The proposed techniques are demonstrated in the challenging domain of sign language recognition. We demonstrate state-of-the-art performance on hand-shape recognition (outperforming previous techniques by more than 30%). Furthermore, we are able to obtain comparable sign recognition rates to previous research, without the need for an alignment step to segment out the signs for recognition.
This paper introduces the end-to-end embedding of a CNN into a HMM, while interpreting the outputs of the CNN in a Bayesian fashion. The hybrid CNN-HMM combines the strong discriminative abilities of CNNs with the sequence modelling capabilities of HMMs. Most current approaches in the field of gesture and sign language recognition disregard the necessity of dealing with sequence data both for training and evaluation. With our presented end-to-end embedding we are able to improve over the state-of-the-art on three challenging benchmark continuous sign language recognition tasks by between 15% and 38% relative and up to 13.3% absolute.
Sign languages are visual languages produced by the movement of the hands, face, and body. In this paper, we evaluate representations based on skeleton poses, as these are explainable, person-independent, privacy-preserving, low-dimensional representations. Basically, skeletal representations generalize over an individual's appearance and background, allowing us to focus on the recognition of motion. But how much information is lost by the skeletal representation? We perform two independent studies using two state-of-the-art pose estimation systems. We analyze the applicability of the pose estimation systems to sign language recognition by evaluating the failure cases of the recognition models. Importantly, this allows us to characterize the current limitations of skeletal pose estimation approaches in sign language recognition.
Attention is an important component of modern deep learning. However, less emphasis has been put on its inverse: ignoring distraction. Our daily lives require us to explicitly avoid giving attention to salient visual features that confound the task we are trying to accomplish. This visual prioritisation allows us to concentrate on important tasks while ignoring visual distractors.In this work, we introduce Neural Blindness, which gives an agent the ability to completely ignore objects or classes that are deemed distractors. More explicitly, we aim to render a neural network completely incapable of representing specific chosen classes in its latent space. In a very real sense, this makes the network "blind" to certain classes, allowing and agent to focus on what is important for a given task, and demonstrates how this can be used to improve localisation.
16th European Conference Glasgow UK August 23 to 28 2020 Proceedings Part XI We introduce an automatic, end-to-end method for recovering the 3D pose and shape of dogs from monocular internet images. The large variation in shape between dog breeds, significant occlusion and low quality of internet images makes this a challenging problem. We learn a richer prior over shapes than previous work, which helps regularize parameter estimation. We demonstrate results on the Stanford Dog dataset, an 'in the wild' dataset of 20,580 dog images for which we have collected 2D joint and silhouette annotations to split for training and evaluation. In order to capture the large shape variety of dogs, we show that the natural variation in the 2D dataset is enough to learn a detailed 3D prior through expectation maximization (EM). As a by-product of training, we generate a new parameterized model (including limb scaling) SMBLD which we release alongside our new annotation dataset StanfordExtra to the research community.
Deep neural networks have demonstrated their capability to learn control policies for a variety of tasks. However, these neural network-based policies have been shown to be susceptible to exploitation by adversarial agents. Therefore, there is a need to develop techniques to learn control policies that are robust against adversaries. We introduce Adversarially Robust Control (ARC), which trains the protagonist policy and the adversarial policy end-to-end on the same loss. The aim of the protagonist is to maximise this loss, whilst the adversary is attempting to minimise it. We demonstrate the proposed ARC training in a highway driving scenario, where the protagonist controls the follower vehicle whilst the adversary controls the lead vehicle. By training the protagonist against an ensemble of adversaries, it learns a significantly more robust control policy, which generalises to a variety of adversarial strategies. The approach is shown to reduce the amount of collisions against new adversaries by up to 90.25%, compared to the original policy. Moreover, by utilising an auxiliary distillation loss, we show that the fine-tuned control policy shows no drop in performance across its original training distribution.
Across a wide range of applications, from autonomous vehicles to medical imaging, multi-spectral images provide an opportunity to extract additional information not present in color images. One of the most important steps in making this information readily available is the accurate estimation of dense correspondences between different spectra. Due to the nature of cross-spectral images, most correspondence solving techniques for the visual domain are simply not applicable. Furthermore, most cross-spectral techniques utilize spectra-specific characteristics to perform the alignment. In this work, we aim to address the dense correspondence estimation problem in a way that generalizes to more than one spectrum. We do this by introducing a novel cycle-consistency metric that allows us to self-supervise. This, combined with our spectra-agnostic loss functions, allows us to train the same network across multiple spectra. We demonstrate our approach on the challenging task of dense RGB-FIR correspondence estimation. We also show the performance of our unmodified network on the cases of RGB-NIR and RGB-RGB, where we achieve higher accuracy than similar self-supervised approaches. Our work shows that cross-spectral correspondence estimation can be solved in a common framework that learns to generalize alignment across spectra.
This paper presents a probabilistic framework of assembling detected human body parts into a full 2D human configuration. The face, torso, legs and hands are detected in cluttered scenes using boosted body part detectors trained by AdaBoost. Body configurations are assembled from the detected parts using RANSAC, and a coarse heuristic is applied to eliminate obvious outliers. An a priori mixture model of upper-body configurations is used to provide a pose likelihood for each configuration. A joint-likelihood model is then determined by combining the pose, part detector and corresponding skin model likelihoods. The assembly with the highest likelihood is selected by RANSAC, and the elbow positions are inferred. This paper also illustrates the combination of skin colour likelihood and detection likelihood to further reduce false hand and face detections.
This paper presents a flexible monocular system capable of recognising sign lexicons far greater in number than previous approaches. The power of the system is due to four key elements: (i) Head and hand detection based upon boosting which removes the need for temperamental colour segmentation; (ii) A body centred description of activity which overcomes issues with camera placement, calibration and user; (iii) A two stage classification in which stage I generates a high level linguistic description of activity which naturally generalises and hence reduces training; (iv) A stage II classifier bank which does not require HMMs, further reducing training requirements. The outcome of which is a system capable of running in real-time, and generating extremely high recognition rates for large lexicons with as little as a single training instance per sign. We demonstrate classification rates as high as 92% for a lexicon of 164 words with extremely low training requirements outperforming previous approaches where thousands of training examples are required.
In this paper, we propose a novel particle filter based probabilistic forced alignment approach for training spatio-temporal deep neural networks using weak border level annotations. The proposed method jointly learns to localize and recognize isolated instances in continuous streams. This is done by drawing training volumes from a prior distribution of likely regions and training a discriminative 3D-CNN from this data. The classifier is then used to calculate the posterior distribution by scoring the training examples and using this as the prior for the next sampling stage. We apply the proposed approach to the challenging task of large-scale user-independent continuous gesture recognition. We evaluate the performance on the popular ChaLearn 2016 Continuous Gesture Recognition (ConGD) dataset. Our method surpasses state-of-the-art results by obtaining 0.3646 and 0.3744 Mean Jaccard Index Score on the validation and test sets of ConGD, respectively. Furthermore, we participated in the ChaLearn 2017 Continuous Gesture Recognition Challenge and was ranked 3rd. It should be noted that our method is learner independent, it can be easily combined with other approaches.
The Visual Object Tracking challenge VOT2017 is the fifth annual tracker benchmarking activity organized by the VOT initiative. Results of 51 trackers are presented; many are state-of-the-art published at major computer vision conferences or journals in recent years. The evaluation included the standard VOT and other popular methodologies and a new "real-time" experiment simulating a situation where a tracker processes images as if provided by a continuously running sensor. Performance of the tested trackers typically by far exceeds standard baselines. The source code for most of the trackers is publicly available from the VOT page. The VOT2017 goes beyond its predecessors by (i) improving the VOT public dataset and introducing a separate VOT2017 sequestered dataset, (ii) introducing a realtime tracking experiment and (iii) releasing a redesigned toolkit that supports complex experiments. The dataset, the evaluation kit and the results are publicly available at the challenge website(1).
This paper presents a scalable solution to the problem of tracking objects across spatially separated, uncalibrated, non-overlapping cameras. Unlike other approaches this technique uses an incremental learning method to create the spatio-temporal links between cameras, and thus model the posterior probability distribution of these links. This can then be used with an appearance model of the object to track across cameras. It requires no calibration or batch preprocessing and becomes more accurate over time as evidence is accumulated.
Disentangled representations support a range of downstream tasks including causal reasoning, generative modeling, and fair machine learning. Unfortunately, disentanglement has been shown to be impossible without the incorporation of supervision or inductive bias. Given that supervision is often expensive or infeasible to acquire, we choose to incorporate structural inductive bias and present an unsupervised, deep State-Space-Model for Video Disentanglement (VDSM). The model disentangles latent time-varying and dynamic factors via the incorporation of hierarchical structure with a dynamic prior and a Mixture of Experts decoder. VDSM learns separate disentangled representations for the identity of the object or person in the video, and for the action being performed. We evaluate VDSM across a range of qualitative and quantitative tasks including identity and dynamics transfer, sequence generation, Fréchet Inception Distance, and factor classification. VDSM achieves state-of-the-art performance and exceeds adversarial methods, even when the methods use additional supervision.
This paper proposes a novel machine learning algorithm (SP-Boosting) to tackle the problem of lipreading by building visual sequence classifiers based on sequential patterns. We show that an exhaustive search of optimal sequential patterns is not possible due to the immense search space, and tackle this with a novel, efficient tree-search method with a set of pruning criteria. Crucially, the pruning strategies preserve our ability to locate the optimal sequential pattern. Additionally, the tree-based search method accounts for the training set's boosting weight distribution. This temporal search method is then integrated into the boosting framework resulting in the SP-Boosting algorithm. We also propose a novel constrained set of strong classifiers that further improves recognition accuracy. The resulting learnt classifiers are applied to lipreading by performing multi-class recognition on the OuluVS database. Experimental results show that our method achieves state of the art recognition performane, using only a small set of sequential patterns. © 2011. The copyright of this document resides with its authors.
This paper introduces a fully-automated, unsupervised method to recognise sign from subtitles. It does this by using data mining to align correspondences in sections of videos. Based on head and hand tracking, a novel temporally constrained adaptation of apriori mining is used to extract similar regions of video, with the aid of a proposed contextual negative selection method. These regions are refined in the temporal domain to isolate the occurrences of similar signs in each example. The system is shown to automatically identify and segment signs from standard news broadcasts containing a variety of topics.
We present a novel data-driven regularizer for weakly-supervised learning of 3D human pose estimation that eliminates the drift problem that affects existing approaches. We do this by moving the stereo reconstruction problem into the loss of the network itself. This avoids the need to reconstruct 3D data prior to training and unlike previous semi-supervised approaches, avoids the need for a warm-up period of supervised training. The conceptual and implementational simplicity of our approach is fundamental to its appeal. Not only is it straightforward to augment many weakly-supervised approaches with our additional re-projection based loss, but it is obvious how it shapes reconstructions and prevents drift. As such we believe it will be a valuable tool for any researcher working in weakly-supervised 3D reconstruction. Evaluating on Panoptic, the largest multi-camera and markerless dataset available, we obtain an accuracy that is essentially indistinguishable from a strongly-supervised approach making full use of 3D groundtruth in training.
Recent approaches to Sign Language Production (SLP) have adopted spoken language Neural Machine Translation (NMT) architectures, applied without sign-specific modifications. In addition, these works represent sign language as a sequence of skeleton pose vectors, projected to an abstract representation with no inherent skeletal structure. In this paper, we represent sign language sequences as a skeletal graph structure, with joints as nodes and both spatial and temporal connections as edges. To operate on this graphical structure, we propose Skeletal Graph Self-Attention (SGSA), a novel graphical attention layer that embeds a skeleton inductive bias into the SLP model. Retaining the skeletal feature representation throughout, we directly apply a spatio-temporal adjacency matrix into the self-attention formulation. This provides structure and context to each skeletal joint that is not possible when using a non-graphical abstract representation, enabling fluid and expressive sign language production. We evaluate our Skeletal Graph Self-Attention architecture on the challenging RWTH-PHOENIX-Weather-2014T (PHOENIX14T) dataset, achieving state-of-the-art back translation performance with an 8% and 7% improvement over competing methods for the dev and test sets.
The Visual Object Tracking challenge VOT2018 is the sixth annual tracker benchmarking activity organized by the VOT initiative. Results of over eighty trackers are presented; many are state-of-the-art trackers published at major computer vision conferences or in journals in the recent years. The evaluation included the standard VOT and other popular methodologies for short-term tracking analysis and a "real-time" experiment simulating a situation where a tracker processes images as if provided by a continuously running sensor. A long-term tracking subchallenge has been introduced to the set of standard VOT sub-challenges. The new subchallenge focuses on long-term tracking properties, namely coping with target disappearance and reappearance. A new dataset has been compiled and a performance evaluation methodology that focuses on long-term tracking capabilities has been adopted. The VOT toolkit has been updated to support both standard short-term and the new long-term tracking subchallenges. Performance of the tested trackers typically by far exceeds standard baselines. The source code for most of the trackers is publicly available from the VOT page. The dataset, the evaluation kit and the results are publicly available at the challenge website (http://votchallenge.net).
An important goal across most scientific fields is the discovery of causal structures underling a set of observations. Unfortunately, causal discovery methods which are based on correlation or mutual information can often fail to identify causal links in systems which exhibit dynamic relationships. Such dynamic systems (including the famous coupled logistic map) exhibit 'mirage' correlations which appear and disappear depending on the observation window. This means not only that correlation is not causation but, perhaps counter-intuitively, that causa-tion may occur without correlation. In this paper we describe Neural Shadow-Mapping, a neural network based method which embeds high-dimensional video data into a low-dimensional shadow representation, for subsequent estimation of causal links. We demonstrate its performance at discovering causal links from video-representations of dynamic systems.
—Undertaking causal inference with observational data is incredibly useful across a wide range of tasks including the development of medical treatments, advertisements and marketing, and policy making. There are two significant challenges associated with undertaking causal inference using observational data: treatment assignment heterogeneity (i.e., differences between the treated and untreated groups), and an absence of counterfactual data (i.e., not knowing what would have happened if an individual who did get treatment, were instead to have not been treated). We address these two challenges by combining structured inference and targeted learning. In terms of structure, we factorize the joint distribution into risk, confounding, instrumental, and miscellaneous factors, and in terms of targeted learning, we apply a regularizer derived from the influence curve in order to reduce residual bias. An ablation study is undertaken, and an evaluation on benchmark datasets demonstrates that TVAE has competitive and state of the art performance across.
Reinforcement learning has been used widely for autonomous longitudinal control algorithms. However, many existing algorithms suffer from sample inefficiency in reinforcement learning as well as the jerky driving behaviour of the learned systems. In this paper, we propose a reinforcement learning algorithm and a training framework to address these two disadvantages of previous algorithms proposed in this field. The proposed system uses an Advantage Actor Critic (A2C) learning system with recurrent layers to introduce temporal context within the network. This allows the learned system to evaluate continuous control actions based on previous states and actions in addition to current states. Moreover, slow training of the algorithm caused by its sample inefficiency is addressed by utilising another neural network to approximate the vehicle dynamics. Using a neural network as a proxy for the simulator has significant benefit to training as it reduces the requirement for reinforcement learning to query the simulation (which is a major bottleneck) in learning and as both reinforcement learning network and proxy network can be deployed on the same GPU, learning speed is considerably improved. Simulation results from testing in IPG CarMaker show the effectiveness of our recurrent A2C algorithm, compared to an A2C without recurrent layers.
The goal of automatic Sign Language Production (SLP) is to translate spoken language to a continuous stream of sign language video at a level comparable to a human translator. If this was achievable, then it would revolutionise Deaf hearing communications. Previous work on predominantly isolated SLP has shown the need for architectures that are better suited to the continuous domain of full sign sequences. In this paper, we propose Progressive Transformers, the first SLP model to translate from discrete spoken language sentences to continuous 3D sign pose sequences in an end-to-end manner. A novel counter decoding technique is introduced, that enables continuous sequence generation at training and inference. We present two model configurations, an end-to end network that produces sign direct from text and a stacked network that utilises a gloss intermediary. We also provide several data augmentation processes to overcome the problem of drift and drastically improve the performance of SLP models. We propose a back translation evaluation mechanism for SLP, presenting benchmark quantitative results on the challenging RWTH-PHOENIXWeather- 2014T (PHOENIX14T) dataset and setting baselines for future research. Code available at https://github.com/BenSaunders27/ ProgressiveTransformersSLP.
Variational AutoEncoders (VAEs) provide a means to generate representational latent embeddings. Previous research has highlighted the benefits of achieving representations that are disentangled, particularly for downstream tasks. However, there is some debate about how to encourage disentanglement with VAEs, and evidence indicates that existing implementations do not achieve disentanglement consistently. The evaluation of how well a VAE’s latent space has been disentangled is often evaluated against our subjective expectations of which attributes should be disentangled for a given problem. Therefore, by definition, we already have domain knowledge of what should be achieved and yet we use unsupervised approaches to achieve it. We propose a weakly supervised approach that incorporates any available domain knowledge into the training process to form a Gated-VAE. The process involves partitioning the representational embedding and gating backpropagation. All partitions are utilised on the forward pass but gradients are backpropagated through different partitions according to selected image/target pairings. The approach can be used to modify existing VAE models such as beta-VAE, InfoVAE and DIP-VAE-II. Experiments demonstrate that using gated backpropagation, latent factors are represented in their intended partition. The approach is applied to images of faces for the purpose of disentangling head-pose from facial expression. Quantitative metrics show that using Gated-VAE improves average disentanglement, completeness and informativeness, as compared with un-gated implementations. Qualitative assessment of latent traversals demonstrate its disentanglement of head-pose from expression, even when only weak/noisy supervision is available.
Most of the vision-based sign language research to date has focused on Isolated Sign Language Recognition (ISLR), where the objective is to predict a single sign class given a short video clip. Although there has been significant progress in ISLR, its real-life applications are limited. In this paper, we focus on the challenging task of Sign Spotting instead, where the goal is to simultaneously identify and localise signs in continuous co-articulated sign videos. To address the limitations of current ISLR-based models, we propose a hierarchical sign spotting approach which learns coarse-to-fine spatio-temporal sign features to take advantage of representations at various temporal levels and provide more precise sign localisation. Specifically, we develop Hierarchical Sign I3D model (HS-I3D) which consists of a hierarchical network head that is attached to the existing spatio-temporal I3D model to exploit features at different layers of the network. We evaluate HS-I3D on the ChaLearn 2022 Sign Spotting Challenge-MSSL track and achieve a state-of-the-art 0.607 F1 score, which was the top-1 winning solution of the competition.
Prior work on Sign Language Translation has shown that having a mid-level sign gloss representation(effectively recognizing the individual signs) improves the translation performance drastically. In fact, the current state-of-theart in translation requires gloss level tokenization in order to work. We introduce a novel transformer based architecture that jointly learns Continuous Sign Language Recognition and Translation whilebeing trainable in an end-to-end manner. This is achieved by using a Connectionist Temporal Classification(CTC)loss to bind the recognition and translation problems into a single unified architecture. This joint approach does not require any ground-truth timing information,simultaneously solving two co-dependant sequence to sequence learning problems and leads to significant performance gains. We evaluate the recognition and translation performances of our approaches on the challenging RWTHPHOENIX-Weather-2014T(PHOENIX14T)dataset. Wereport state-of-the-art sign language recognition and translation results achieved by our Sign Language Transformers. Our translation net works out perform both sign video to spoken language and gloss to spoken language translation models, in some cases more than doubling the performance (9.58vs. 21.80BLEU-4Score). We also share new baseline translation results using transformer networks for several other text-to-text sign language translation tasks.
Accurate extrinsic sensor calibration is essential for both autonomous vehicles and robots. Traditionally this is an involved process requiring calibration targets, known fiducial markers and is generally performed in a lab. Moreover, even a small change in the sensor layout requires recalibration. With the anticipated arrival of consumer autonomous vehicles, there is demand for a system which can do this automatically, after deployment and without specialist human expertise. To solve these limitations, we propose a flexible framework which can estimate extrinsic parameters without an explicit calibration stage, even for sensors with unknown scale. Our first contribution builds upon standard hand-eye calibration by jointly recovering scale. Our second contribution is that our system is made robust to imperfect and degenerate sensor data, by collecting independent sets of poses and automatically selecting those which are most ideal. We show that our approach’s robustness is essential for the target scenario. Unlike previous approaches, ours runs in real time and constantly estimates the extrinsic transform. For both an ideal experimental setup and a real use case, comparison against these approaches shows that we outperform the state-of-the-art. Furthermore, we demonstrate that the recovered scale may be applied to the full trajectory, circumventing the need for scale estimation via sensor fusion.
We approach instantaneous mapping, converting images to a top-down view of the world, as a translation problem. We show how a novel form of transformer network can be used to map from images and video directly to an overhead map or bird's-eye-view (BEV) of the world, in a single end-to-end network. We assume a 1-1 correspondence between a vertical scanline in the image, and rays passing through the camera location in an overhead map. This lets us formulate map generation from an image as a set of sequence-to-sequence translations. Posing the problem as translation allows the network to use the context of the image when interpreting the role of each pixel. This constrained formulation, based upon a strong physical grounding of the problem, leads to a restricted transformer network that is convolutional in the horizontal direction only. The structure allows us to make efficient use of data when training, and obtains state-of-the-art results for instantaneous mapping of three large-scale datasets, including a 15% and 30% relative gain against existing best performing methods on the nuScenes and Argoverse datasets, respectively.
Predicting 3D human pose from a single monoscopic video can be highly challenging due to factors such as low resolution, motion blur and occlusion, in addition to the fundamental ambiguity in estimating 3D from 2D. Approaches that directly regress the 3D pose from independent images can be particularly susceptible to these factors and result in jitter, noise and/or inconsistencies in skeletal estimation. Much of which can be overcome if the temporal evolution of the scene and skeleton are taken into account. However, rather than tracking body parts and trying to temporally smooth them, we propose a novel transformer based network that can learn a distribution over both pose and motion in an unsupervised fashion. We call our approach Skeletor. Skeletor overcomes inaccuracies in detection and corrects partial or entire skeleton corruption. Skeletor uses strong priors learn from on 25 million frames to correct skeleton sequences smoothly and consistently. Skeletor can achieve this as it implicitly learns the spatio-temporal context of human motion via a transformer based neural network. Extensive experiments show that Skeletor achieves improved performance on 3D human pose estimation and further provides benefits for downstream tasks such as sign language translation.
In this work, we propose a framework to collect a large-scale, diverse sign language dataset that can be used to train automatic sign language recognition models. The first contribution of this work is SDTRACK, a generic method for signer tracking and diarisation in the wild. Our second contribution is SEEHEAR, a dataset of 90 hours of British Sign Language (BSL) content featuring a wide range of signers, and including interviews, monologues and debates. Using SDTRACK, the SEEHEAR dataset is annotated with 35K active signing tracks, with corresponding signer identities and subtitles, and 40K automatically localised sign labels. As a third contribution, we provide benchmarks for signer diarisation and sign recognition on SEEHEAR
In the context of self-driving vehicles there is strong competition between approaches based on visual localisa-tion and Light Detection And Ranging (LiDAR). While LiDAR provides important depth information, it is sparse in resolution and expensive. On the other hand, cameras are low-cost and recent developments in deep learning mean they can provide high localisation performance. However, several fundamental problems remain, particularly in the domain of uncertainty, where learning based approaches can be notoriously over-confident. Markov, or grid-based, localisation was an early solution to the localisation problem but fell out of favour due to its computational complexity. Representing the likelihood field as a grid (or volume) means there is a trade off between accuracy and memory size. Furthermore, it is necessary to perform expensive convolutions across the entire likelihood volume. Despite the benefit of simultaneously maintaining a likelihood for all possible locations, grid based approaches were superseded by more efficient particle filters and Monte Carlo sampling (MCL). However, MCL introduces its own problems e.g. particle deprivation. Recent advances in deep learning hardware allow large likelihood volumes to be stored directly on the GPU, along with the hardware necessary to efficiently perform GPU-bound 3D convolutions and this obviates many of the disadvantages of grid based methods. In this work, we present a novel CNN-based localisation approach that can leverage modern deep learning hardware. By implementing a grid-based Markov localisation approach directly on the GPU, we create a hybrid Convolutional Neural Network (CNN) that can perform image-based localisation and odometry-based likelihood propagation within a single neural network. The resulting approach is capable of outperforming direct pose regression methods as well as state-of-the-art localisation systems.
—The ability to produce large-scale maps for navigation , path planning and other tasks is a crucial step for autonomous agents, but has always been challenging. In this work, we introduce BEV-SLAM, a novel type of graph-based SLAM that aligns semantically-segmented Bird's Eye View (BEV) predictions from monocular cameras. We introduce a novel form of occlusion reasoning into BEV estimation and demonstrate its importance to aid spatial aggregation of BEV predictions. The result is a versatile SLAM system that can operate across arbitrary multi-camera configurations and can be seamlessly integrated with other sensors. We show that the use of multiple cameras significantly increases performance, and achieves lower relative error than high-performance GPS. The resulting system is able to create large, dense, globally-consistent world maps from monocular cameras mounted around an ego vehicle. The maps are metric and correctly-scaled, making them suitable for downstream navigation tasks.
We present SignSynth, a fully automatic and holistic approach to generating sign language video. Traditionally, Sign Language Production (SLP) relies on animating 3D avatars using expensively annotated data, but so far this approach has not been able to simultaneously provide a realistic, and scalable solution. We introduce a gloss2pose network architecture that is capable of generating human pose sequences conditioned on glosses.1 Combined with a generative adversarial pose2video network, we are able to produce natural-looking, high definition sign language video. For sign pose sequence generation, we outperform the SotA by a factor of 18, with a Mean Square Error of 1.0673 in pixels. For video generation we report superior results on three broadcast quality assessment metrics. To evaluate our full gloss-to-video pipeline we introduce two novel error metrics, to assess the perceptual quality and sign representativeness of generated videos. We present promising results, significantly outperforming the SotA in both metrics. Finally we evaluate our approach qualitatively by analysing example sequences.
— The visual anonymisation of sign language data is an essential task to address privacy concerns raised by large-scale dataset collection. Previous anonymisation techniques have either significantly affected sign comprehension or required manual, labour-intensive work. In this paper, we formally introduce the task of Sign Language Video Anonymisation (SLVA) as an automatic method to anonymise the visual appearance of a sign language video whilst retaining the meaning of the original sign language sequence. To tackle SLVA, we propose ANONYSIGN, a novel automatic approach for visual anonymisation of sign language data. We first extract pose information from the source video to remove the original signer appearance. We next generate a photo-realistic sign language video of a novel appearance from the pose sequence, using image-to-image translation methods in a conditional variational autoencoder framework. An approximate posterior style distribution is learnt, which can be sampled from to synthesise novel human appearances. In addition, we propose a novel style loss that ensures style consistency in the anonymised sign language videos. We evaluate ANONYSIGN for the SLVA task with extensive quantitative and qualitative experiments highlighting both realism and anonymity of our novel human appearance synthesis. In addition, we formalise an anonymity perceptual study as an evaluation criteria for the SLVA task and showcase that video anonymisation using ANONYSIGN retains the original sign language content.
It is common practice to represent spoken languages at their phonetic level. However, for sign languages, this implies breaking motion into its constituent motion primi-tives. Avatar based Sign Language Production (SLP) has traditionally done just this, building up animation from sequences of hand motions, shapes and facial expressions. However, more recent deep learning based solutions to SLP have tackled the problem using a single network that estimates the full skeletal structure. We propose splitting the SLP task into two distinct jointly-trained sub-tasks. The first translation sub-task translates from spoken language to a latent sign language representation, with gloss supervision. Subsequently, the animation sub-task aims to produce expressive sign language sequences that closely resemble the learnt spatio-temporal representation. Using a progressive transformer for the translation sub-task, we propose a novel Mixture of Motion Primitives (MOMP) architecture for sign language animation. A set of distinct motion primitives are learnt during training, that can be temporally combined at inference to animate continuous sign language sequences. We evaluate on the challenging RWTH-PHOENIX-Weather-2014T (PHOENIX14T) dataset, presenting extensive ablation studies and showing that MOMP outperforms baselines in user evaluations. We achieve state-of-the-art back translation performance with an 11% improvement over competing results. Importantly, and for the first time, we showcase stronger performance for a full translation pipeline going from spoken language to sign, than from gloss to sign.
The applications of causal inference may be life-critical, including the evaluation of vaccinations, medicine, and social policy. However, when undertaking estimation for causal inference, practitioners rarely have access to what might be called ‘ground-truth’ in a supervised learning setting, meaning the chosen estimation methods cannot be evaluated and must be assumed to be reliable. It is therefore crucial that we have a good understanding of the performance consistency of typical methods available to practitioners. In this work we provide a comprehensive evaluation of recent semiparametric methods (including neural network approaches) for average treatment effect estimation. Such methods have been proposed as a means to derive unbiased causal effect estimates and statistically valid confidence intervals, even when using otherwise non-parametric, data-adaptive machine learning techniques. We also propose a new estimator ‘MultiNet’, and a variation on the semiparametric update step ‘MultiStep’, which we evaluate alongside existing approaches. The performance of both semiparametric and ‘regular’ methods are found to be dataset dependent, indicating an interaction between the methods used, the sample size, and nature of the data generating process. Our experiments highlight the need for practitioners to check the consistency of their findings, potentially by undertaking multiple analyses with different combinations of estimators.
Devising planning algorithms for autonomous driving is non-trivial due to the presence of complex and uncertain interaction dynamics between road users. In this paper, we introduce a planning framework encompassing multiple action policies that are learned jointly from episodes of human-human interactions in naturalistic driving. The policy model is composed of encoder-decoder recurrent neural networks for modeling the sequential nature of interactions and mixture density networks for characterizing the probability distributions over driver actions. The model is used to simultaneously generate a finite set of context-dependent candidate plans for an autonomous car and to anticipate the probable future plans of human drivers. This is followed by an evaluation stage to select the plan with the highest expected utility for execution. Our approach leverages rapid sampling of action distributions in parallel on a graphic processing unit, offering fast computation even when modeling the interactions among multiple vehicles and over several time steps. We present ablation experiments and comparison with two existing baseline methods to highlight several design choices that we found to be essential to our model's success. We test the proposed planning approach in a simulated highway driving environment, showing that by using the model, the autonomous car can plan actions that mimic the interactive behavior of humans.
Sign languages are multi-channel visual languages, where signers use a continuous 3D space to communicate. Sign language production (SLP), the automatic translation from spoken to sign languages, must embody both the continuous articulation and full morphology of sign to be truly understandable by the Deaf community. Previous deep learning-based SLP works have produced only a concatenation of isolated signs focusing primarily on the manual features, leading to a robotic and non-expressive production. In this work, we propose a novel Progressive Transformer architecture, the first SLP model to translate from spoken language sentences to continuous 3D multi-channel sign pose sequences in an end-to-end manner. Our transformer network architecture introduces a counter decoding that enables variable length continuous sequence generation by tracking the production progress over time and predicting the end of sequence. We present extensive data augmentation techniques to reduce prediction drift, alongside an adversarial training regime and a mixture density network (MDN) formulation to produce realistic and expressive sign pose sequences. We propose a back translation evaluation mechanism for SLP, presenting benchmark quantitative results on the challenging PHOENIX14T dataset and setting baselines for future research. We further provide a user evaluation of our SLP model, to understand the Deaf reception of our sign pose productions.
Estimating a semantically segmented bird's-eye-view (BEV) map from a single image has become a popular technique for autonomous control and navigation. However, they show an increase in localization error with distance from the camera. While such an increase in error is entirely expected – localization is harder at distance – much of the drop in performance can be attributed to the cues used by current texture-based models, in particular, they make heavy use of object-ground intersections (such as shadows) [9], which become increasingly sparse and uncertain for distant objects. In this work, we address these shortcomings in BEV-mapping by learning the spatial relationship between objects in a scene. We propose a graph neural network which predicts BEV objects from a monocular image by spatially reasoning about an object within the context of other objects. Our approach sets a new state-of-the-art in BEV estimation from monocular images across three large-scale datasets, including a 50% relative improvement for objects on nuScenes.
This paper summarizes the results of the first Monocular Depth Estimation Challenge (MDEC) organized at WACV2023. This challenge evaluated the progress of selfsupervised monocular depth estimation on the challenging SYNS-Patches dataset. The challenge was organized on CodaLab and received submissions from 4 valid teams. Participants were provided a devkit containing updated reference implementations for 16 State-of-the-Art algorithms and 4 novel techniques. The threshold for acceptance for novel techniques was to outperform every one of the 16 SotA baselines. All participants outperformed the baseline in traditional metrics such as MAE or AbsRel. However, pointcloud reconstruction metrics were challenging to improve upon.We found predictions were characterized by interpolation artefacts at object boundaries and errors in relative object positioning. We hope this challenge is a valuable contribution to the community and encourage authors to participate in future editions
Motion estimation algorithms are typically based upon the assumption of brightness constancy or related assumptions such as gradient constancy. This manuscript evaluates several common cost functions from the motion estimation literature, which embody these assumptions. We demonstrate that such assumptions break for real world data, and the functions are therefore unsuitable. We propose a simple solution, which significantly increases the discriminatory ability of the metric, by learning a nonlinear relationship using techniques from machine learning. Furthermore, we demonstrate how context and a nonlinear combination of metrics, can provide additional gains, and demonstrating a 44% improvement in the performance of a state of the art scene flow estimation technique. In addition, smaller gains of 20% are demonstrated in optical flow estimation tasks.
A general framework for learning to respond appropriately to visual stimulus is presented. By hierarchically clustering percept-action exemplars in the action space, contextually important features and relationships in the perceptual input space are identified and associated with response models of varying generality. Searching the hierarchy for a set of best matching percept models yields a set of action models with likelihoods. By posing the problem as one of cost surface optimisation in a probabilistic framework, a particle filter inspired forward exploration algorithm is employed to select actions from multiple hypotheses that move the system toward a goal state and to escape from local minima. The system is quantitatively and qualitatively evaluated in both a simulated shape sorter puzzle and a real-world autonomous navigation domain.
We introduce a new algorithm for real-time interactive motion control and demonstrate its application to motion captured data, pre-recorded videos and HCI. Firstly, a data set of frames are projected into a lower dimensional space. An appearance model is learnt using a multivariate probability distribution. A novel approach to determining transition points is presented based on k-medoids, whereby appropriate points of intersection in the motion trajectory are derived as cluster centres. These points are used to segment the data into smaller subsequences. A transition matrix combined with a kernel density estimation is used to determine suitable transitions between the subsequences to develop novel motion. To facilitate real-time interactive control, conditional probabilities are used to derive motion given user commands. The user commands can come from any modality including auditory, touch and gesture. The system is also extended to HCI using audio signals of speech in a conversation to trigger non-verbal responses from a synthetic listener in real-time. We demonstrate the flexibility of the model by presenting results ranging from data sets composed of vectorised images, 2D and 3D point representations. Results show real-time interaction and plausible motion generation between different types of movement.
This paper introduces a novel approach to social behaviour recognition governed by the exchange of non-verbal cues between people. We conduct experiments to try and deduce distinct rules that dictate the social dynamics of people in a conversation, and utilise semi-supervised computer vision techniques to extract their social signals such as laughing and nodding. Data mining is used to deduce frequently occurring patterns of social trends between a speaker and listener in both interested and not interested social scenarios. The confidence values from rules are utilised to build a Social Dynamic Model (SDM), that can then be used for classification and visualisation. By visualising the rules generated in the SDM, we can analyse distinct social trends between an interested and not interested listener in a conversation. Results show that these distinctions can be applied generally and used to accurately predict conversational interest.
This paper presents a flexible monocular system capable of recognising sign lexicons far greater in number than previous approaches. The power of the system is due to four key elements: (i) Head and hand detection based upon boosting which removes the need for temperamental colour segmentation; (ii) A body centred description of activity which overcomes issues with camera placement, calibration and user; (iii) A two stage classification in which stage I generates a high level linguistic description of activity which naturally generalises and hence reduces training; (iv) A stage II classifier bank which does not require HMMs, further reducing training requirements. The outcome of which is a system capable of running in real-time, and generating extremely high recognition rates for large lexicons with as little as a single training instance per sign. We demonstrate classification rates as high as 92% for a lexicon of 164 words with extremely low training requirements outperforming previous approaches where thousands of training examples are required.
We propose a novel hybrid approach to static pose estimation called Connected Poselets. This representation combines the best aspects of part-based and example-based estimation. First detecting poselets extracted from the training data; our method then applies a modified Random Decision Forest to identify Poselet activations. By combining keypoint predictions from poselet activitions within a graphical model, we can infer the marginal distribution over each keypoint without any kinematic constraints. Our approach is demonstrated on a new publicly available dataset with promising results.
A novel approach to learning and tracking arbitrary image features is presented. Tracking is tackled by learning the mapping from image intensity differences to displacements. Linear regression is used, resulting in low computational cost. An appearance model of the target is built on-the-fly by clustering sub-sampled image templates. The medoidshift algorithm is used to cluster the templates thus identifying various modes or aspects of the target appearance, each mode is associated to the most suitable set of linear predictors allowing piecewise linear regression from image intensity differences to warp updates. Despite no hard-coding or offline learning, excellent results are shown on three publicly available video sequences and comparisons with related approaches made.
In this paper, we propose a computational model for social interaction between three people in a conversation, and demonstrate results using human video motion synthesis. We utilised semi-supervised computer vision techniques to label social signals between the people, like laughing, head nod and gaze direction. Data mining is used to deduce frequently occurring patterns of social signals between a speaker and a listener in both interested and not interested social scenarios, and the mined confidence values are used as conditional probabilities to animate social responses. The human video motion synthesis is done using an appearance model to learn a multivariate probability distribution, combined with a transition matrix to derive the likelihood of motion given a pose configuration. Our system uses social labels to more accurately define motion transitions and build a texture motion graph. Traditional motion synthesis algorithms are best suited to large human movements like walking and running, where motion variations are large and prominent. Our method focuses on generating more subtle human movement like head nods. The user can then control who speaks and the interest level of the individual listeners resulting in social interactive conversational agents.
The motion field of a scene can be used for object segmentation and to provide features for classification tasks like action recognition. Scene flow is the full 3D motion field of the scene, and is more difficult to estimate than it's 2D counterpart, optical flow. Current approaches use a smoothness cost for regularisation, which tends to over-smooth at object boundaries. This paper presents a novel formulation for scene flow estimation, a collection of moving points in 3D space, modelled using a particle filter that supports multiple hypotheses and does not oversmooth the motion field. In addition, this paper is the first to address scene flow estimation, while making use of modern depth sensors and monocular appearance images, rather than traditional multi-viewpoint rigs. The algorithm is applied to an existing scene flow dataset, where it achieves comparable results to approaches utilising multiple views, while taking a fraction of the time.
This paper demonstrates a novel method for automatically discovering and recognising characters in video without any labelled examples or user intervention. Instead weak supervision is obtained via a rough script-to-subtitle alignment. The technique uses pose invariant features, extracted from detected faces and clustered to form groups of co-occurring characters. Results show that with 9 characters, 29% of the closest exemplars are correctly identified, increasing to 50% as additional exemplars are considered.
We present a novel approach to 3D reconstruction which is inspired by the human visual system. This system unifies standard appearance matching and triangulation techniques with higher level reasoning and scene understanding, in order to resolve ambiguities between different interpretations of the scene. The types of reasoning integrated in the approach includes recognising common configurations of surface normals and semantic edges (e.g. convex, concave and occlusion boundaries). We also recognise the coplanar, collinear and symmetric structures which are especially common in man made environments.
This paper presents a method for learning distance functions of arbitrary feature representations that is based on the concept of wormholes. We introduce wormholes and describe how it provides a method for warping the topology of visual representation spaces such that a meaningful distance between examples is available. Additionally, we show how a more general distance function can be learnt through the combination of many wormholes via an inter-wormhole network. We then demonstrate the application of the distance learning method on a variety of problems including nonlinear synthetic data, face illumination detection and the retrieval of images containing natural landscapes and man-made objects (e.g. cities).
This paper proposes a novel machine learning algorithm (SP-Boosting) to tackle the problem of lipreading by building visual sequence classifiers based on sequential patterns. We show that an exhaustive search of optimal sequential patterns is not possible due to the immense search space, and tackle this with a novel, efficient tree-search method with a set of pruning criteria. Crucially, the pruning strategies preserve our ability to locate the optimal sequential pattern. Additionally, the tree-based search method accounts for the training set’s boosting weight distribution. This temporal search method is then integrated into the boosting framework resulting in the SP-Boosting algorithm. We also propose a novel constrained set of strong classifiers that further improves recognition accuracy. The resulting learnt classifiers are applied to lipreading by performing multi-class recognition on the OuluVS database. Experimental results show that our method achieves state of the art recognition performane, using only a small set of sequential patterns.
In this work we describe a novel approach to online dense non-rigid structure from motion. The problem is reformulated, incorporating ideas from visual object tracking, to provide a more general and unified technique, with feedback between the reconstruction and point-tracking algorithms. The resulting algorithm overcomes the limitations of many conventional techniques, such as the need for a reference image/template or precomputed trajectories. The technique can also be applied in traditionally challenging scenarios, such as modelling objects with strong self-occlusions or from an extreme range of viewpoints. The proposed algorithm needs no offline pre-learning and does not assume the modelled object stays rigid at the beginning of the video sequence. Our experiments show that in traditional scenarios, the proposed method can achieve better accuracy than the current state of the art while using less supervision. Additionally we perform reconstructions in challenging new scenarios where state-of-the-art approaches break down and where our method improves performance by up to an order of magnitude.
We describe a prototype Search-by-Example or look-up tool for signs, based on a newly developed 1000-concept sign lexicon for four national sign languages (GSL, DGS, LSF,BSL), which includes a spoken language gloss, a HamNoSys description, and a video for each sign. The look-up tool combines an interactive sign recognition system, supported by Kinect technology, with a real-time sign synthesis system,using a virtual human signer, to present results to the user. The user performs a sign to the system and is presented with animations of signs recognised as similar. The user also has the option to view any of these signs performed in the other three sign languages. We describe the supporting technology and architecture for this system, and present some preliminary evaluation results.
Action recognition in unconstrained situations is a difficult task, suffering from massive intra-class variations. It is made even more challenging when complex 3D actions are projected down to the image plane, losing a great deal of information. The recent emergence of 3D data, both in broadcast content, and commercial depth sensors, provides the possibility to overcome this issue. This paper presents a new dataset, for benchmarking action recognition algorithms in natural environments, while making use of 3D information. The dataset contains around 650 video clips, across 14 classes. In addition, two state of the art action recognition algorithms are extended to make use of the 3D data, and five new interest point detection strategies are also proposed, that extend to the 3D data. Our evaluation compares all 4 feature descriptors, using 7 different types of interest point, over a variety of threshold levels, for the Hollywood 3D dataset. We make the dataset including stereo video, estimated depth maps and all code required to reproduce the benchmark results, available to the wider community.
Distance functions are an important component in many learning applications. However, the correct function is context dependent, therefore it is advantageous to learn a distance function using available training data. Many existing distance functions is the requirement for data to exist in a space of constant dimensionality and not possible to be directly used on symbolic data. To address these problems, this paper introduces an alternative learnable distance function, based on multi-kernel distance bases or "wormholes that connects spaces belonging to similar examples that were originally far away close together. This work only assumes the availability of a set data in the form of relative comparisons, avoiding the need for having labelled or quantitative information. To learn the distance function, two algorithms were proposed: 1) Building a set of basic wormhole bases using a Boosting-inspired algorithm. 2) Merging different distance bases together for better generalisation. The learning algorithms were then shown to successfully extract suitable distance functions in various clustering problems, ranging from synthetic 2D data to symbolic representations of unlabelled images
In this paper, we propose a novel particle filter based probabilistic forced alignment approach for training spatiotemporal deep neural networks using weak border level annotations. The proposed method jointly learns to localize and recognize isolated instances in continuous streams. This is done by drawing training volumes from a prior distribution of likely regions and training a discriminative 3D-CNN from this data. The classifier is then used to calculate the posterior distribution by scoring the training examples and using this as the prior for the next sampling stage. We apply the proposed approach to the challenging task of large-scale user-independent continuous gesture recognition. We evaluate the performance on the popular ChaLearn 2016 Continuous Gesture Recognition (ConGD) dataset. Our method surpasses state-of-the-art results by obtaining 0:3646 and 0:3744 Mean Jaccard Index Score on the validation and test sets of ConGD, respectively. Furthermore, we participated in the ChaLearn 2017 Continuous Gesture Recognition Challenge and was ranked 3rd. It should be noted that our method is learner independent, it can be easily combined with other approaches.
Tracking hands and estimating their trajectories is useful in a number of tasks, including sign language recognition and human computer interaction. Hands are extremely difficult objects to track, their deformability, frequent self occlusions and motion blur cause appearance variations too great for most standard object trackers to deal with robustly. In this paper, the 3D motion field of a scene (known as the Scene Flow, in contrast to Optical Flow, which is it's projection onto the image plane) is estimated using a recently proposed algorithm, inspired by particle filtering. Unlike previous techniques, this scene flow algorithm does not introduce blurring across discontinuities, making it far more suitable for object segmentation and tracking. Additionally the algorithm operates several orders of magnitude faster than previous scene flow estimation systems, enabling the use of Scene Flow in real-time, and near real-time applications. A novel approach to trajectory estimation is then introduced, based on clustering the estimated scene flow field in both space and velocity dimensions. This allows estimation of object motions in the true 3D scene, rather than the traditional approach of estimating 2D image plane motions. By working in the scene space rather than the image plane, the constant velocity assumption, commonly used in the prediction stage of trackers, is far more valid, and the resulting motion estimate is richer, providing information on out of plane motions. To evaluate the performance of the system, 3D trajectories are estimated on a multi-view sign-language dataset, and compared to a traditional high accuracy 2D system, with excellent results.
In this paper we present an approach to hand pose estimation that combines both discriminative and modelbased methods to overcome the limitations of each technique in isolation. A Randomised Decision Forests (RDF) is used to provide an initial estimate of the regions of the hand. This initial segmentation provides constraints to which a 3D model is fitted using Rigid Body Dynamics. Model fitting is guided using point to surface constraints which bind a kinematic model of the hand to the depth cloud using the segmentation of the discriminative approach. This combines the advantages of both techniques, reducing the training requirements for discriminative classification and simplifying the optimization process involved in model fitting by incorporating physical constraints from the segmentation. Our experiments on two challenging sequences show that this combined method outperforms the current state-of-the-art approach.
Robust and fast algorithms for estimating the pose of a human given an image would have a far reaching impact on many fields in and outside of computer vision. We address the problem using depth data that can be captured inexpensively using consumer depth cameras such as the Kinect sensor. To achieve robustness and speed on a small training dataset, we formulate the pose estimation task within a regression and Hough voting framework. Our approach uses random regression forests to predict joint locations from each pixel and accumulate these predictions with Hough voting. The Hough accumulator images are treated as likelihood distributions where maxima correspond to joint location hypotheses. We demonstrate our approach and compare to the state-ofthe-art on a publicly available dataset.
This paper presents a surface segmentation method which uses a simulated inflating balloon model to segment surface structure from volumetric data using a triangular mesh. The model employs simulated surface tension and an inflationary force to grow from within an object and find its boundary. Mechanisms are described which allow both evenly spaced and minimal polygonal count surfaces to be generated. The work is based on inflating balloon models by Terzopolous[8]. Simplifications are made to the model, and an approach proposed which provides a technique robust to noise regardless of the feature detection scheme used. The proposed technique uses no explicit attraction to data features, and as such is less dependent on the initialisation of the model and parameters. The model grows under its own forces, and is never anchored to boundaries, but instead constrained to remain inside the desired object. Results are presented which demonstrate the technique’s ability and speed at the segmentation of a complex, concave object with narrow features.
Actions in the wild” is the term given to examples of human motion that are performed in natural settings, such as those harvested from movies [10] or the Internet [9]. State-of-the-art approaches in this domain are orders of magnitude lower than in more contrived settings. One of the primary reasons being the huge variability within each action class. We propose to tackle recognition in the wild by automatically breaking complex action categories into multiple modes/group, and training a separate classifier for each mode. This is achieved using RANSAC which identifies and separates the modes while rejecting outliers. We employ a novel reweighting scheme within the RANSAC procedure to iteratively reweight training examples, ensuring their inclusion in the final classification model. Our results demonstrate the validity of the approach, and for classes which exhibit multi-modality, we achieve in excess of double the performance over approaches that assume single modality.
Visual category recognition is a difficult task of significant interest to the machine learning and vision community. One of the principal hurdles is the high dimensional feature space. This paper evaluates several linear and non-linear dimensionality reduction techniques. A novel evaluation metric, the rényi entropy of the inter-vector euclidean distance distribution, is introduced. This information theoretic measure judges the techniques on their preservation of structure in lower-dimensional sub-space. The popular dataset, Caltech-101 is utilized in the experiments. The results indicate that the techniques which preserve local neighborhood structure performed best amongst the techniques evaluated in this paper. © 2011 EURASIP.
This paper presents an autonomous robotic system that incorporates novel Computer Vision, Machine Learning and Data Mining algorithms in order to learn to navigate and discover important visual entities. This is achieved within a Learning from Demonstration (LfD) framework, where policies are derived from example state-to-action mappings. For autonomous navigation, a mapping is learnt from holistic image features (GIST) onto control parameters using Random Forest regression. Additionally, visual entities (road signs e.g. STOP sign) that are strongly associated to autonomously discovered modes of action (e.g. stopping behaviour) are discovered through a novel Percept-Action Mining methodology. The resulting sign detector is learnt without any supervision (no image labeling or bounding box annotations are used). The complete system is demonstrated on a fully autonomous robotic platform, featuring a single camera mounted on a standard remote control car. The robot carries a PC laptop, that performs all the processing on board and in real-time. © 2013 IEEE.
This manuscript outlines our current demonstration system for translating visual Sign to written text. The system is based around a broad description of scene activity that naturally generalizes, reducing training requirements and allowing the knowledge base to be explicitly stated. This allows the same system to be used for different sign languages requiring only a change of the knowledge base.
This work presents a piecewise linear approximation to non-linear Point Distribution Models for modelling the human hand. The work utilises the natural segmentation of shape space, inherent to the technique, to apply temporal constraints which can be used with CONDENSATION to support multiple hypotheses and quantum leaps through shape space. This paper presents a novel method by which the one-state transitions of the English Language are projected into shape space for tracking and model prediction using a HMM like approach.
We present a framework for autonomous behaviour in vision based artificial cognitive systems by imitation through coupled percept-action (stimulus and response) exemplars. Attributed Relational Graphs (ARGs) are used as a symbolic representation of scene information (percepts). A measure of similarity between ARGs is implemented with the use of a graph isomorphism algorithm and is used to hierarchically group the percepts. By hierarchically grouping percept exemplars into progressively more general models coupled to progressively more general Gaussian action models, we attempt to model the percept space and create a direct mapping to associated actions. The system is built on a simulated shape sorter puzzle that represents a robust vision system. Spatio temporal hypothesis exploration is performed ef- ficiently in a Bayesian framework using a particle filter to propagate game play over time.
This paper presents a model based approach to human body tracking in which the 2D silhouette of a moving human and the corresponding 3D skeletal structure are encapsulated within a non-linear Point Distribution Model. This statistical model allows a direct mapping to be achieved between the external boundary of a human and the anatomical position. It is shown how this information, along with the position of landmark features such as the hands and head can be used to reconstruct information about the pose and structure of the human body from a monoscopic view of a scene.
In this paper, we aim to tackle the problem of recognising temporal sequences in the context of a multi-class problem. In the past, the representation of sequential patterns was used for modelling discriminative temporal patterns for different classes. Here, we have improved on this by using the more general representation of episodes, of which sequential patterns are a special case. We then propose a novel tree structure called a MultI-Class Episode Tree (MICE-Tree) that allows one to simultaneously model a set of different episodes in an efficient manner whilst providing labels for them. A set of MICE-Trees are then combined together into a MICE-Forest that is learnt in a Boosting framework. The result is a strong classifier that utilises episodes for performing classification of temporal sequences. We also provide experimental evidence showing that the MICE-Trees allow for a more compact and efficient model compared to sequential patterns. Additionally, we demonstrate the accuracy and robustness of the proposed method in the presence of different levels of noise and class labels.
Many variants of MI exist in the literature. These vary primarily in how the joint histogram is populated. This paper places the four main variants of MI: Standard sampling, Partial Volume Estimation (PVE), In-Parzen Windowing and Post-Parzen Windowing into a single mathematical framework. Jacobians and Hessians are derived in each case. A particular contribution is that the non-linearities implicit to standard sampling and post-Parzen windowing are explicitly dealt with. These non-linearities are a barrier to their use in optimisation. Side-by-side comparison of the MI variants is made using eight diverse data-sets, considering computational expense and convergence. In the experiments, PVE was generally the best performer, although standard sampling often performed nearly as well (if a higher sample rate was used). The widely used sum of squared differences metric performed as well as MI unless large occlusions and non-linear intensity relationships occurred. The binaries and scripts used for testing are available online.
This paper presents an approach to object tracking which, given a single example of a target, learns a hierarchical constellation model of appearance and structure on the fly. The model becomes more robust over time as evidence of the variability of the object is acquired and added to the model. Tracking is performed in an optimised Lucas-Kanade type framework, using Mutual Information as a similarity metric. Several novelties are presented: an improved template update strategy using Bayes theorem, a multi-tier model topology, and a semi-automatic testing method. A critical comparison with other methods is made using exhaustive testing. In all 11 challenging test sequences were used with a mean length of 568 frames.
Over the last two decades automatic facial expression recognition has become an active research area. Facial expressions are an important channel of non-verbal communication, and can provide cues to emotions and intentions. This paper introduces a novel method for facial expression recognition, by assembling contour fragments as discriminatory classifiers and boosting them to form a strong accurate classifier. Detection is fast as features are evaluated using an efficient lookup to a chamfer image, which weights the response of the feature. An Ensemble classification technique is presented using a voting scheme based on classifiers responses. The results of this research are a 6-class classifier (6 basic expressions of anger, joy, sadness, surprise, disgust and fear) which demonstrate competitive results achieving rates as high as 96% for some expressions. As classifiers are extremely fast to compute the approach operates at well above frame rate. We also demonstrate how a dedicated classifier can be consrtucted to give optimal automatic parameter selection of the detector, allowing real time operation on unconstrained video.
This paper outlines a method of estimating the 3D pose of the upper human body from a single uncalibrated camera. The objective application lies in 3D Human Computer Interaction where hand depth information offers extended functionality when interacting with a 3D virtual environment, but it is equally suitable to animation and motion capture. A database of 3D body configurations is built from a variety of human movements using motion capture data. A hierarchical structure consisting of three subsidiary databases, namely the frontal-view Hand Position (top-level), Silhouette and Edge Map Databases, are pre-extracted from the 3D body configuration database. Using this hierarchy, subsets of the subsidiary databases are then matched to the subject in real-time. The examples of the subsidiary databases that yield the highest matching score are used to extract the corresponding 3D configuration from the motion capture data, thereby estimating the upper body 3D pose.
A non-parametric (NP) sampling method is introduced for obtaining the joint distribution of a pair of images. This method based on NP windowing and is equivalent to sampling the images at infinite resolution. Unlike existing methods, arbitrary selection of kernels is not required and the spatial structure of images is used. NP windowing is applied to a registration application where the mutual information (MI) between a reference image and a warped template is maximised with respect to the warp parameters. In comparisons against the current state of the art MI registration methods NP windowing yielded excellent results with lower bias and improved convergence rates
Non-linear statistical models of deformation provide methods to learn a priori shape and deformation for an object or class of objects by example. This paper extends these models of deformation to that of motion by augmenting the discrete representation of piecewise nonlinear principle component analysis of shape with a markov chain which represents the temporal dynamics of the model. In this manner, mean trajectories can be learnt and reproduced for either the simulation of movement or for object tracking. This paper demonstrates the use of these techniques in learning human motion from capture data.
Research into facial expression recognition has predominantly been based upon near frontal view data. However, a recent 3D facial expression database (BU-3DFE database) has allowed empirical investigation of facial expression recognition across pose. In this paper, we investigate the effects of pose from frontal to profile view on facial expression recognition. Experiments are carried out on 100 subjects with 5 yaw angles over 6 prototypical expressions. Expressions have 4 levels of intensity from subtle to exaggerated. We evaluate features such as local binary patterns (LBPs) as well as various extensions of LBPs. In addition, a novel approach to facial expression recognition is proposed using local gabor binary patterns (LGBPs). Multi class support vector machines (SVMs) are used for classification. We investigate the effects of image resolution and pose on facial expression classification using a variety of different features.
This work proposes to learn linguistically-derived sub-unit classifiers for sign language. The responses of these classifiers can be combined by Markov models, producing efficient sign-level recognition. Tracking is used to create vectors of hand positions per frame as inputs for sub-unit classifiers learnt using AdaBoost. Grid-like classifiers are built around specific elements of the tracking vector to model the placement of the hands. Comparative classifiers encode the positional relationship between the hands. Finally, binary-pattern classifiers are applied over the tracking vectors of multiple frames to describe the motion of the hands. Results for the sub-unit classifiers in isolation are presented, reaching averages over 90%. Using a simple Markov model to combine the sub-unit classifiers allows sign level classification giving an average of 63%, over a 164 sign lexicon, with no grammatical constraints.
We propose a novel deep learning approach to solve simultaneous alignment and recognition problems (referred to as “Sequence-to-sequence” learning). We decompose the problem into a series of specialised expert systems referred to as SubUNets. The spatio-temporal relationships between these SubUNets are then modelled to solve the task, while remaining trainable end-to-end. The approach mimics human learning and educational techniques, and has a number of significant advantages. SubUNets allow us to inject domain-specific expert knowledge into the system regarding suitable intermediate representations. They also allow us to implicitly perform transfer learning between different interrelated tasks, which also allows us to exploit a wider range of more varied data sources. In our experiments we demonstrate that each of these properties serves to significantly improve the performance of the overarching recognition system, by better constraining the learning problem. The proposed techniques are demonstrated in the challenging domain of sign language recognition. We demonstrate state-of-the-art performance on hand-shape recognition (outperforming previous techniques by more than 30%). Furthermore, we are able to obtain comparable sign recognition rates to previous research, without the need for an alignment step to segment out the signs for recognition.
This paper outlines a system design and implementation of a 3D input device for graphical applications. It is shown how computer vision can be used to track a users movements within the image frame allowing interaction with 3D worlds and objects. Point Distribution Models (PDMs) have been shown to be successful at tracking deformable objects. This system demonstrates how these ‘smart snakes’ can be used in real time with real world applications, demonstrating how computer vision can provide a low cost, intuitive interface that has few hardware constraints. The compact mathematical model behind the PDM allows simple static gesture recognition to be performed providing the means to communicate with an application. It is shown how movement of both the hand and face can be used to drive 3D engines. The system is based upon Open Inventor and designed for use with Silicon Graphics Indy Workstations but allowances have been made to facilitate the inclusion of the tracker within third party applications. The reader is also provided with an insight into the next generation of HCI and Multimedia. Access to this work can be gained through the above web address.
This paper presents a novel approach to learning a codebook for visual categorization, that resolves the key issue of intra-category appearance variation found in complex real world datasets. The codebook of visual-topics (semantically equivalent descriptors) is made by grouping visual-words (syntactically equivalent descriptors) that are scattered in feature space. We analyze the joint distribution of images and visual-words using information theoretic co-clustering to discover visual-topics. Our approach is compared with the standard 'Bagof- Words' approach. The statistically significant performance improvement in all the datasets utilized (Pascal VOC 2006; VOC 2007; VOC 2010; Scene-15) establishes the efficacy of our approach.
This work presents a new approach to learning a framebased classifier on weakly labelled sequence data by embedding a CNN within an iterative EM algorithm. This allows the CNN to be trained on a vast number of example images when only loose sequence level information is available for the source videos. Although we demonstrate this in the context of hand shape recognition, the approach has wider application to any video recognition task where frame level labelling is not available. The iterative EM algorithm leverages the discriminative ability of the CNN to iteratively refine the frame level annotation and subsequent training of the CNN. By embedding the classifier within an EM framework the CNN can easily be trained on 1 million hand images. We demonstrate that the final classifier generalises over both individuals and data sets. The algorithm is evaluated on over 3000 manually labelled hand shape images of 60 different classes which will be released to the community. Furthermore, we demonstrate its use in continuous sign language recognition on two publicly available large sign language data sets, where it outperforms the current state-of-the-art by a large margin. To our knowledge no previous work has explored expectation maximization without Gaussian mixture models to exploit weak sequence labels for sign language recognition.
This paper presents a novel solution to the difficult task of both detecting and estimating the 3D pose of humans in monoscopic images. The approach consists of two parts. Firstly the location of a human is identified by a probabalistic assembly of detected body parts. Detectors for the face, torso and hands are learnt using adaBoost. A pose likliehood is then obtained using an a priori mixture model on body configuration and possible configurations assembled from available evidence using RANSAC. Once a human has been detected, the location is used to initialise a matching algorithm which matches the silhouette and edge map of a subject with a 3D model. This is done efficiently using chamfer matching, integral images and pose estimation from the initial detection stage. We demonstrate the application of the approach to large, cluttered natural images and at near framerate operation (16fps) on lower resolution video streams.
How does a person work out their location using a floorplan? It is probably safe to say that we do not explicitly measure depths to every visible surface and try to match them against different pose estimates in the floorplan. And yet, this is exactly how most robotic scan-matching algorithms operate. Similarly, we do not extrude the 2D geometry present in the floorplan into 3D and try to align it to the real-world. And yet, this is how most vision-based approaches localise. Humans do the exact opposite. Instead of depth, we use high level semantic cues. Instead of extruding the floorplan up into the third dimension, we collapse the 3D world into a 2D representation. Evidence of this is that many of the floorplans we use in everyday life are not accurate, opting instead for high levels of discriminative landmarks. In this work, we use this insight to present a global localisation approach that relies solely on the semantic labels present in the floorplan and extracted from RGB images. While our approach is able to use range measurements if available, we demonstrate that they are unnecessary as we can achieve results comparable to state-of-the-art without them.
Reconstruction of 3D environments is a problem that has been widely addressed in the literature. While many approaches exist to perform reconstruction, few of them take an active role in deciding where the next observations should come from. Furthermore, the problem of travelling from the camera’s current position to the next, known as pathplanning, usually focuses on minimising path length. This approach is ill-suited for reconstruction applications, where learning about the environment is more valuable than speed of traversal. We present a novel Scenic Route Planner that selects paths which maximise information gain, both in terms of total map coverage and reconstruction accuracy. We also introduce a new type of collaborative behaviour into the planning stage called opportunistic collaboration, which allows sensors to switch between acting as independent Structure from Motion (SfM) agents or as a variable baseline stereo pair. We show that Scenic Planning enables similar performance to state-of-the-art batch approaches using less than 0.00027% of the possible stereo pairs (3% of the views). Comparison against length-based pathplanning approaches show that our approach produces more complete and more accurate maps with fewer frames. Finally, we demonstrate the Scenic Pathplanner’s ability to generalise to live scenarios by mounting cameras on autonomous ground-based sensor platforms and exploring an environment.
The Visual Object Tracking challenge 2015, VOT2015, aims at comparing short-term single-object visual trackers that do not apply pre-learned models of object appearance. Results of 62 trackers are presented. The number of tested trackers makes VOT 2015 the largest benchmark on short-term tracking to date. For each participating tracker, a short description is provided in the appendix. Features of the VOT2015 challenge that go beyond its VOT2014 predecessor are: (i) a new VOT2015 dataset twice as large as in VOT2014 with full annotation of targets by rotated bounding boxes and per-frame attribute, (ii) extensions of the VOT2014 evaluation methodology by introduction of a new performance measure. The dataset, the evaluation kit as well as the results are publicly available at the challenge website.
Long term tracking of an object, given only a single instance in an initial frame, remains an open problem. We propose a visual tracking algorithm, robust to many of the difficulties which often occur in real-world scenes. Correspondences of edge-based features are used, to overcome the reliance on the texture of the tracked object and improve invariance to lighting. Furthermore we address long-term stability, enabling the tracker to recover from drift and to provide redetection following object disappearance or occlusion. The two-module principle is similar to the successful state-of-the-art long-term TLD tracker, however our approach extends to cases of low-textured objects. Besides reporting our results on the VOT Challenge dataset, we perform two additional experiments. Firstly, results on short-term sequences show the performance of tracking challenging objects which represent failure cases for competing state-of-the-art approaches. Secondly, long sequences are tracked, including one of almost 30000 frames which to our knowledge is the longest tracking sequence reported to date. This tests the re-detection and drift resistance properties of the tracker. All the results are comparable to the state-of-the-art on sequences with textured objects and superior on non-textured objects. The new annotated sequences are made publicly available
Often within the field of tracking people within only fixed cameras are used. This can mean that when the the illumination of the image changes or object occlusion occurs, the tracking can fail. We propose an approach that uses three simultaneous separate sensors. The fixed surveillance cameras track objects of interest cross camera through incrementally learning relationships between regions on the image. Cameras and laser rangefinder sensors onboard robots also provide an estimate of the person. Moreover, the signal strength of mobile devices carried by the person can be used to estimate his position. The estimate from all these sources are then combined using data fusion to provide an increase in performance. We present results of the fixed camera based tracking operating in real time on a large outdoor environment of over 20 non-overlapping cameras. Moreover, the tracking algorithms for robots and wireless nodes are described. A decentralized data fusion algorithm for combining all these information is presented.
This paper presents a solution to the problem of tracking people within crowded scenes. The aim is to maintain individual object identity through a crowded scene which contains complex interactions and heavy occlusions of people. Our approach uses the strengths of two separate methods; a global object detector and a localised frame by frame tracker. A temporal relationship model of torso detections built during low activity period, is used to further disambiguate during periods of high activity. A single camera with no calibration and no environmental information is used. Results are compared to a standard tracking method and groundtruth. Two video sequences containing interactions, overlaps and occlusions between people are used to demonstrate our approach. The results show that our technique performs better that a standard tracking method and can cope with challenging occlusions and crowd interactions.
The use of sparse invariant features to recognise classes of actions or objects has become common in the literature. However, features are often ”engineered” to be both sparse and invariant to transformation and it is assumed that they provide the greatest discriminative information. To tackle activity recognition, we propose learning compound features that are assembled from simple 2D corners in both space and time. Each corner is encoded in relation to its neighbours and from an over complete set (in excess of 1 million possible features), compound features are extracted using data mining. The final classifier, consisting of sets of compound features, can then be applied to recognise and localise an activity in real-time while providing superior performance to other state-of-the-art approaches (including those based upon sparse feature detectors). Furthermore, the approach requires only weak supervision in the form of class labels for each training sequence. No ground truth position or temporal alignment is required during training.
This paper presents an approach to the categorisation of spatio-temporal activity in video, which is based solely on the relative distribution of feature points. Introducing a Relative Motion Descriptor for actions in video, we show that the spatio-temporal distribution of features alone (without explicit appearance information) effectively describes actions, and demonstrate performance consistent with state-of-the-art. Furthermore, we propose that for actions where noisy examples exist, it is not optimal to group all action examples as a single class. Therefore, rather than engineering features that attempt to generalise over noisy examples, our method follows a different approach: We make use of Random Sampling Consensus (RANSAC) to automatically discover and reject outlier examples within classes. We evaluate the Relative Motion Descriptor and outlier rejection approaches on four action datasets, and show that outlier rejection using RANSAC provides a consistent and notable increase in performance, and demonstrate superior performance to more complex multiple-feature based approaches.
It is known that relative feature location is important in representing objects, but assumptions that make learning tractable often simplify how structure is encoded e.g. spatial pooling or star models. For example, techniques such as spatial pyramid matching (SPM), in-conjunction with machine learning techniques perform well [13]. However, there are limitations to such spatial encoding schemes which discard important information about the layout of features. In contrast, we propose to use the object itself to choose the basis of the features in an object centric approach. In doing so we return to the early work of geometric hashing [18] but demonstrate how such approaches can be scaled-up to modern day object detection challenges in terms of both the number of examples and their variability. We apply a two stage process; initially filtering background features to localise the objects and then hashing the remaining pairwise features in an affine invariant model. During learning, we identify class-wise key feature predictors. We validate our detection and classification of objects on the PASCAL VOC’07 and ’11 [6] and CarDb [21] datasets and compare with state of the art detectors and classifiers. Importantly we demonstrate how structure in features can be efficiently identified and how its inclusion can increase performance. This feature centric learning technique allows us to localise objects even without object annotation during training and the resultant segmentation provides accurate state of the art object localization, without the need for annotations.
We present a generic, efficient and iterative algorithm for interactively clustering classes of images and videos. The approach moves away from the use of large hand labelled training datasets, instead allowing the user to find natural groups of similar content based upon a handful of “seed” examples. Two efficient data mining tools originally developed for text analysis; min-Hash and APriori are used and extended to achieve both speed and scalability on large image and video datasets. Inspired by the Bag-of-Words (BoW) architecture, the idea of an image signature is introduced as a simple descriptor on which nearest neighbour classification can be performed. The image signature is then dynamically expanded to identify common features amongst samples of the same class. The iterative approach uses APriori to identify common and distinctive elements of a small set of labelled true and false positive signatures. These elements are then accentuated in the signature to increase similarity between examples and “pull” positive classes together. By repeating this process, the accuracy of similarity increases dramatically despite only a few training examples, only 10% of the labelled groundtruth is needed, compared to other approaches. It is tested on two image datasets including the caltech101 [9] dataset and on three state-of-the-art action recognition datasets. On the YouTube [18] video dataset the accuracy increases from 72% to 97% using only 44 labelled examples from a dataset of over 1200 videos. The approach is both scalable and efficient, with an iteration on the full YouTube dataset taking around 1 minute on a standard desktop machine.
This paper presents a generic method for recognising and localising human actions in video based solely on the distribution of interest points. The use of local interest points has shown promising results in both object and action recognition. While previous methods classify actions based on the appearance and/or motion of these points, we hypothesise that the distribution of interest points alone contains the majority of the discriminatory information. Motivated by its recent success in rapidly detecting 2D interest points, the semi-naive Bayesian classification method of Randomised Ferns is employed. Given a set of interest points within the boundaries of an action, the generic classifier learns the spatial and temporal distributions of those interest points. This is done efficiently by comparing sums of responses of interest points detected within randomly positioned spatio-temporal blocks within the action boundaries. We present results on the largest and most popular human action dataset using a number of interest point detectors, and demostrate that the distribution of interest points alone can perform as well as approaches that rely upon the appearance of the interest points.
Intelligent visual surveillance is an important application area for computer vision. In situations where networks of hundreds of cameras are used to cover a wide area, the obvious limitation becomes the users’ ability to manage such vast amounts of information. For this reason, automated tools that can generalise about activities or track objects are important to the operator. Key to the users’ requirements is the ability to track objects across (spatially separated) camera scenes. However, extensive geometric knowledge about the site and camera position is typically required. Such an explicit mapping from camera to world is infeasible for large installations as it requires that the operator know which camera to switch to when an object disappears. To further compound the problem the installation costs of CCTV systems outweigh those of the hardware. This means that geometric constraints or any form of calibration (such as that which might be used with epipolar constraints) is simply not realistic for a real world installation. The algorithms cannot afford to dictate to the installer. This work attempts to address this problem and outlines a method to allow objects to be related and tracked across cameras without any explicit calibration, be it geometric or colour.
Although 3D reconstruction from a monocular video has been an active area of research for a long time, and the resulting models offer great realism and accuracy, strong conditions must be typically met when capturing the video to make this possible. This prevents general reconstruction of moving objects in dynamic, uncontrolled scenes. In this paper, we address this issue. We present a novel algorithm for modelling 3D shapes from unstructured, unconstrained discontinuous footage. The technique is robust against distractors in the scene, background clutter and even shot cuts. We show reconstructed models of objects, which could not be modelled by conventional Structure from Motion methods without additional input. Finally, we present results of our reconstruction in the presence of shot cuts, showing the strength of our technique at modelling from existing footage.
Human lip-readers are increasingly being presented as useful in the gathering of forensic evidence but, like all humans, suffer from unreliability. Here we report the results of a long-term study in automatic lip-reading with the objective of converting video-to-text (V2T). The V2T problem is surprising in that some aspects that look tricky, such as real-time tracking of the lips on poor-quality interlaced video from hand-held cameras, but prove to be relatively tractable. Whereas the problem of speaker independent lip-reading is very demanding due to unpredictable variations between people. Here we review the problem of automatic lip-reading for crime fighting and identify the critical parts of the problem.
heute gibt es aber auch mehr angebote in der kultur oder woanders (trans: Today, however, there are also more offers in culture or elsewhere) HEUTE1 MEHR1 VERSCHIEDENES2 KULTUR1A VERSCHIEDENES1 wir konnen uns selber andern oder freude geben (trans: we can change ourselves or give joy) KORPER1 SELBST1A ODER6B FROH1 GEBEN1 a) b) d) c) Figure 1. Photo-Realistic Sign Language Production: Given a spoken language sentence from an unconstrained domain of discourse (a), an initial translation is conducted to a gloss sequence (b). FS-NET next produces a co-articulated continuous skeleton pose sequence from dictionary signs (c), which SIGNGAN generates into a photo-realistic sign language video in a given style (d). Abstract Sign languages are visual languages, with vocabularies as rich as their spoken language counterparts. However , current deep-learning based Sign Language Production (SLP) models produce under-articulated skeleton pose sequences from constrained vocabularies and this limits applicability. To be understandable and accepted by the deaf, an automatic SLP system must be able to generate co-articulated photo-realistic signing sequences for large domains of discourse. In this work, we tackle large-scale SLP by learning to co-articulate between dictionary signs, a method capable of producing smooth signing while scaling to unconstrained domains of discourse. To learn sign co-articulation, we propose a novel Frame Selection Network (FS-NET) that improves the temporal alignment of interpolated dictionary signs to continuous signing sequences. Additionally, we propose SIGNGAN, a pose-conditioned human synthesis model that produces photo-realistic sign language videos direct from skeleton pose. We propose a novel keypoint-based loss function which improves the quality of synthesized hand images. We evaluate our SLP model on the large-scale meineDGS (mDGS) corpus, conducting extensive user evaluation showing our FS-NET approach improves co-articulation of interpolated dictionary signs. Additionally, we show that SIGNGAN significantly outperforms all base-line methods for quantitative metrics, human perceptual studies and native deaf signer comprehension.
Neural Sign Language Production (SLP) aims to automatically translate from spoken language sentences to sign language videos. Historically the SLP task has been broken into two steps; Firstly, translating from a spoken language sentence to a gloss sequence and secondly, producing a sign language video given a sequence of glosses. In this paper we apply Natural Language Processing techniques to the first step of the SLP pipeline. We use language models such as BERT and Word2Vec to create better sentence level embeddings, and apply several tokenization techniques, demonstrating how these improve performance on the low resource translation task of Text to Gloss. We introduce Text to HamNoSys (T2H) translation, and show the advantages of using a phonetic representation for sign language translation rather than a sign level gloss representation. Furthermore, we use HamNoSys to extract the hand shape of a sign and use this as additional supervision during training, further increasing the performance on T2H. Assembling best practise, we achieve a BLEU-4 score of 26.99 on the MineDGS dataset and 25.09 on PHOENIX14T, two new state-of-the-art baselines.
Sign language recognition (SLR) involves identifying the form and meaning of isolated signs or sequences of signs. To our knowledge, the combination of SLR and sign language assessment is novel. The goal of an ongoing three-year project in Switzerland is to pioneer an assessment system for lexical signs of Swiss German Sign Language (Deutschschweizerische Geb¨ardensprache, DSGS) that relies on SLR. The assessment system aims to give adult L2 learners of DSGS feedback on the correctness of the manual parameters (handshape, hand position, location, and movement) of isolated signs they produce. In its initial version, the system will include automatic feedback for a subset of a DSGS vocabulary production test consisting of 100 lexical items. To provide the SLR component of the assessment system with sufficient training samples, a large-scale dataset containing videotaped repeated productions of the 100 items of the vocabulary test with associated transcriptions and annotations was created, consisting of data from 11 adult L1 signers and 19 adult L2 learners of DSGS. This paper introduces the dataset, which will be made available to the research community.
Sign language conveys information through multiple channels, such as hand shape, hand movement, and mouthing. Modeling this multichannel information is a highly challenging problem. In this paper, we elucidate the link between spoken language and sign language in terms of production phenomenon and perception phenomenon. Through this link we show that hidden Markov model-based approaches developed to model "articulatory" features for spoken language processing can be exploited to model the multichannel information inherent in sign language for sign language processing.
We propose a novel approach to tracking objects by low-level line correspondences. In our implementation we show that this approach is usable even when tracking objects with lack of texture, exploiting situations, when feature-based trackers fails due to the aperture problem. Furthermore, we suggest an approach to failure detection and recovery to maintain long-term stability. This is achieved by remembering configurations which lead to good pose estimations and using them later for tracking corrections. We carried out experiments on several sequences of different types. The proposed tracker proves itself as competitive or superior to state-of-the-art trackers in both standard and low-textured scenes.
This paper tackles the problem of spotting a set of signs occuring in videos with sequences of signs. To achieve this, we propose to model the spatio-temporal signatures of a sign using an extension of sequential patterns that contain temporal intervals called Sequential Interval Patterns (SIP). We then propose a novel multi-class classifier that organises different sequential interval patterns in a hierarchical tree structure called a Hierarchical SIP Tree (HSP-Tree). This allows one to exploit any subsequence sharing that exists between different SIPs of different classes. Multiple trees are then combined together into a forest of HSP-Trees resulting in a strong classifier that can be used to spot signs. We then show how the HSP-Forest can be used to spot sequences of signs that occur in an input video. We have evaluated the method on both concatenated sequences of isolated signs and continuous sign sequences. We also show that the proposed method is superior in robustness and accuracy to a state of the art sign recogniser when applied to spotting a sequence of signs.
This article presents an interactive hand shape recognition user interface for American Sign Language (ASL) finger-spelling. The system makes use of a Microsoft Kinect device to collect appearance and depth images, and of the OpenNI+NITE framework for hand detection and tracking. Hand-shapes corresponding to letters of the alphabet are characterized using appearance and depth images and classified using random forests. We compare classification using appearance and depth images, and show a combination of both lead to best results, and validate on a dataset of four different users. This hand shape detection works in real-time and is integrated in an interactive user interface allowing the signer to select between ambiguous detections and integrated with an English dictionary for efficient writing.
This paper presents a head pose and facial feature estimation technique that works over a wide range of pose variations without a priori knowledge of the appearance of the face. Using simple LK trackers, head pose is estimated by Levenberg-Marquardt (LM) pose estimation using the feature tracking as constraints. Factored sampling and RANSAC are employed to both provide a robust pose estimate and identify tracker drift by constraining outliers in the estimation process. The system provides both a head pose estimate and the position of facial features and is capable of tracking over a wide range of head poses.
This paper presents a method to perform person independent sign recognition. This is achieved by implementing generalising features based on sign linguistics. These are combined using two methods. The first is traditional Markov models, which are shown to lack the required generalisation. The second is a discriminative approach called Sequential Pattern Boosting, which combines feature selection with learning. The resulting system is introduced as a dictionary application, allowing signers to query by performing a sign in front of a Kinect. Two data sets are used and results shown for both, with the query-return rate reaching 99.9% on a 20 sign multi-user dataset and 85.1% on a more challenging and realistic subject independent, 40 sign test set.
This article presents a dictionary for Sign Language using visual sign recognition based on linguistic subcomponents. We demonstrate a system where the user makes a query, receiving in response a ranked selection of similar results. The approach uses concepts from linguistics to provide sign sub-unit features and classifiers based on motion, sign-location and handshape. These sub-units are combined using Markov Models for sign level recognition. Results are shown for a video dataset of 984 isolated signs performed by a native signer. Recognition rates reach 71.4% for the first candidate and 85.9% for retrieval within the top 10 ranked signs.
In this paper, we address the problem of tracking an unknown object in 3D space. Online 2D tracking often fails for strong outof-plane rotation which results in considerable changes in appearance beyond those that can be represented by online update strategies. However, by modelling and learning the 3D structure of the object explicitly, such effects are mitigated. To address this, a novel approach is presented, combining techniques from the fields of visual tracking, structure from motion (SfM) and simultaneous localisation and mapping (SLAM). This algorithm is referred to as TMAGIC (Tracking, Modelling And Gaussianprocess Inference Combined). At every frame, point and line features are tracked in the image plane and are used, together with their 3D correspondences, to estimate the camera pose. These features are also used to model the 3D shape of the object as a Gaussian process. Tracking determines the trajectories of the object in both the image plane and 3D space, but the approach also provides the 3D object shape. The approach is validated on several video-sequences used in the tracking literature, comparing favourably to state-of-the-art trackers for simple scenes (error reduced by 22 %) with clear advantages in the case of strong out-of-plane rotation, where 2D approaches fail (error reduction of 58 %).
This paper presents an approach to large lexicon sign recog- nition that does not require tracking. This overcomes the issues of how to accurately track the hands through self occlusion in unconstrained video, instead opting to take a detection strategy, where patterns of motion are identi ed. It is demonstrated that detection can be achieved with only minor loss of accuracy compared to a perfectly tracked sequence using coloured gloves. The approach uses two levels of classi cation. In the rst, a set of viseme classi ers detects the presence of sub-Sign units of activity. The second level then assembles visemes into word level Sign using Markov chains. The system is able to cope with a large lexicon and is more expandable than traditional word level approaches. Using as few as 5 training examples the proposed system has classi cation rates as high as 74.3% on a randomly selected 164 sign vocabulary performing at a comparable level to other tracking based systems.
This paper presents a scalable solution to the problem of tracking objects across spatially separated, uncalibrated, non-overlapping cameras. Unlike other approaches this technique uses an incremental learning method to create the spatio-temporal links between cameras, and thus model the posterior probability distribution of these links. This can then be used with an appearance model of the object to track across cameras. It requires no calibration or batch preprocessing and becomes more accurate over time as evidence is accumulated.
The Thermal Infrared Visual Object Tracking challenge 2016, VOT-TIR2016, aims at comparing short-term single-object visual trackers that work on thermal infrared (TIR) sequences and do not apply pre-learned models of object appearance. VOT-TIR2016 is the second benchmark on short-term tracking in TIR sequences. Results of 24 trackers are presented. For each participating tracker, a short description is provided in the appendix. The VOT-TIR2016 challenge is similar to the 2015 challenge, the main difference is the introduction of new, more difficult sequences into the dataset. Furthermore, VOT-TIR2016 evaluation adopted the improvements regarding overlap calculation in VOT2016. Compared to VOT-TIR2015, a significant general improvement of results has been observed, which partly compensate for the more difficult sequences. The dataset, the evaluation kit, as well as the results are publicly available at the challenge website.
This paper proposes a method for sign language recognition that bypasses the need for tracking by classifying the motion directly. The method uses the natural extension of haar like features into the temporal domain, computed efficiently using an integral volume. These volumetric features are assembled into spatio-temporal classifiers using boosting. Results are presented for a fast feature extraction method and 2 different types of boosting. These configurations have been tested on a data set consisting of both seen and unseen signers performing 5 signs producing competitive results.
Driving a car in an urban setting is an extremely difficult problem, incorporating a large number of complex visual tasks; yet, this problem is solved daily by most adults with little apparent effort. This article proposes a novel vision-based approach to autonomous driving that can predict and even anticipate a driver’s behaviour in real-time, using preattentive vision only. Experiments on three large datasets totalling over 200,000 frames show that our pre-attentive model can: 1) detect a wide range of driving-critical context such as crossroads, city centre and road type; however, more surprisingly it can 2) detect the driver’s actions (over 80% of braking and turning actions); and 3) estimate the driver’s steering angle accurately. Additionally, our model is consistent with human data: first, the best steering prediction is obtained for a perception to action delay consistent with psychological experiments. Importantly, this prediction can be made before the driver’s action. Second, the regions of the visual field used by the computational model correlate strongly with the driver’s gaze locations, significantly outperforming many saliency measures and comparably to state-of-the-art approaches.
We present a solution to the problem of tracking intermittent targets that can overcome long-term occlusions as well as movement between camera views. Unlike other approaches, our system does not require topological knowledge of the site or labelled training patterns during the learning period. The approach uses the statistical consistency of data obtained automatically over an extended period of time rather than explicit geometric calibration to automatically learn the salient reappearance periods for objects. This allows us to predict where objects may reappear and within how long. We demonstrate how these salient reappearance periods can be used with a model of physical appearance to track objects between spatially separate regions in single and separated views.
This paper presents a novel, discriminative, multi-class classifier based on Sequential Pattern Trees. It is efficient to learn, compared to other Sequential Pattern methods, and scalable for use with large classifier banks. For these reasons it is well suited to Sign Language Recognition. Using deterministic robust features based on hand trajectories, sign level classifiers are built from sub-units. Results are presented both on a large lexicon single signer data set and a multi-signer Kinect™ data set. In both cases it is shown to out perform the non-discriminative Markov model approach and be equivalent to previous, more costly, Sequential Pattern (SP) techniques.
Recognition of human communication has previously focused on deliberately acted emotions or in structured or artificial social contexts. This makes the result hard to apply to realistic social situations. This paper describes the recording of spontaneous human communication in a specific and common social situation: conversation between two people. The clips are then annotated by multiple observers to reduce individual variations in interpretation of social signals. Temporal and static features are generated from tracking using heuristic and algorithmic methods. Optimal features for classifying examples of spontaneous communication signals are then extracted by AdaBoost. The performance of the boosted classifier is comparable to human performance for some communication signals, even on this challenging and realistic data set.
The relationship between the feedback given in Reinforcement Learning (RL) and visual data input is often extremely complex. Given this, expecting a single system trained end-to-end to learn both how to perceive and interact with its environment is unrealistic for complex domains. In this paper we propose Auto-Perceptive Reinforcement Learning (APRiL), separating the perception and the control elements of the task. This method uses an auto-perceptive network to encode a feature space. The feature space may explicitly encode available knowledge from the semantically understood state space but the network is also free to encode unanticipated auxiliary data. By decoupling visual perception from the RL process, APRiL can make use of techniques shown to improve performance and efficiency of RL training, which are often difficult to apply directly with a visual input. We present results showing that APRiL is effective in tasks where the semantically understood state space is known. We also demonstrate that allowing the feature space to learn auxiliary information, allows it to use the visual perception system to improve performance by approximately 30%. We also show that maintaining some level of semantics in the encoded state, which can then make use of state-of-the art RL techniques, saves around 75% of the time that would be used to collect simulation examples.
Deep learning has become an increasingly common technique for various control problems, such as robotic arm manipulation, robot navigation, and autonomous vehicles. However, the downside of using deep neural networks to learn control policies is their opaque nature and the difficulties of validating their safety. As the networks used to obtain state-of-the-art results become increasingly deep and complex, the rules they have learned and how they operate become more challenging to understand. This presents an issue, since in safety-critical applications the safety of the control policy must be ensured to a high confidence level. In this paper, we propose an automated black box testing framework based on adversarial reinforcement learning. The technique uses an adversarial agent, whose goal is to degrade the performance of the target model under test. We test the approach on an autonomous vehicle problem, by training an adversarial reinforcement learning agent, which aims to cause a deep neural network-driven autonomous vehicle to collide. Two neural networks trained for autonomous driving are compared, and the results from the testing are used to compare the robustness of their learned control policies. We show that the proposed framework is able to find weaknesses in both control policies that were not evident during online testing and therefore, demonstrate a significant benefit over manual testing methods.
This work employs data mining algorithms to discover visual entities that are strongly associated to autonomously discovered modes of action, in an embodied agent. Mappings are learnt from these perceptual entities, onto the agents action space. In general, low dimensional action spaces are better suited to unsupervised learning than high dimensional percept spaces, allowing for structure to be discovered in the action space, and used to organise the perceptual space. Local feature configurations that are strongly associated to a particular ‘type’ of action (and not all other action types) are considered likely to be relevant in eliciting that action type. By learning mappings from these relevant features onto the action space, the system is able to respond in real time to novel visual stimuli. The proposed approach is demonstrated on an autonomous navigation task, and the system is shown to identify the relevant visual entities to the task and to generate appropriate responses.
This paper presents a real time approach to locate and track the upper torso of the human body. Our main interest is not in 3D biometric accuracy, but rather a sufficient discriminatory representation for visual interaction. The algorithm employs background suppression and a general approximation to body shape, applied within a particle filter framework, making use of integral images to maintain real-time performance. Furthermore, we present a novel method to disambiguate the hands of the subject and to predict the likely position of elbows. The final system is demonstrated segmenting multiple subjects from a cluttered scene at above real time operation.
Interactive learning platforms are in the top choices to acquire new languages. Such applications or platforms are more easily available for spoken languages, but rarely for sign languages. Assessment of the production of signs is a challenging problem because of the multichannel aspect (e.g., hand shape, hand movement, mouthing, facial expression) inherent in sign languages. In this paper, we propose an automatic sign language production assessment approach which allows assessment of two linguistic aspects: (i) the produced lexeme and (ii) the produced forms. On a linguistically annotated Swiss German Sign Language dataset, SMILE DSGS corpus, we demonstrate that the proposed approach can effectively assess the two linguistic aspects in an integrated manner.
Within the field of action recognition, features and descriptors are often engineered to be sparse and invariant to transformation. While sparsity makes the problem tractable, it is not necessarily optimal in terms of class separability and classification. This paper proposes a novel approach that uses very dense corner features that are spatially and temporally grouped in a hierarchical process to produce an overcomplete compound feature set. Frequently reoccurring patterns of features are then found through data mining, designed for use with large data sets. The novel use of the hierarchical classifier allows real time operation while the approach is demonstrated to handle camera motion, scale, human appearance variations, occlusions and background clutter. The performance of classification, outperforms other state-of-the-art action recognition algorithms on the three datasets; KTH, multi-KTH, and Hollywood. Multiple action localisation is performed, though no groundtruth localisation data is required, using only weak supervision of class labels for each training sequence. The Hollywood dataset contain complex realistic actions from movies, the approach outperforms the published accuracy on this dataset and also achieves real time performance. ©2009 IEEE.
In this paper, we propose using 3D Convolutional Neural Networks for large scale user-independent continuous gesture recognition. We have trained an end-to-end deep network for continuous gesture recognition (jointly learning both the feature representation and the classifier). The network performs three-dimensional (i.e. space-time) convolutions to extract features related to both the appearance and motion from volumes of color frames. Space-time invariance of the extracted features is encoded via pooling layers. The earlier stages of the network are partially initialized using the work of Tran et al. before being adapted to the task of gesture recognition. An earlier version of the proposed method, which was trained for 11,250 iterations, was submitted to ChaLearn 2016 Continuous Gesture Recognition Challenge and ranked 2nd with the Mean Jaccard Index Score of 0.269235. When the proposed method was further trained for 28,750 iterations, it achieved state-of-the-art performance on the same dataset, yielding a 0.314779 Mean Jaccard Index Score.
This chapter covers the key aspects of sign-language recognition (SLR), starting with a brief introduction to the motivations and requirements, followed by a précis of sign linguistics and their impact on the field. The types of data available and the relative merits are explored allowing examination of the features which can be extracted. Classifying the manual aspects of sign (similar to gestures) is then discussed from a tracking and non-tracking viewpoint before summarising some of the approaches to the non-manual aspects of sign languages. Methods for combining the sign classification results into full SLR are given showing the progression towards speech recognition techniques and the further adaptations required for the sign specific case. Finally the current frontiers are discussed and the recent research presented. This covers the task of continuous sign recognition, the work towards true signer independence, how to effectively combine the different modalities of sign, making use of the current linguistic research and adapting to larger more noisy data sets
Estimating the pose of an object, be it articulated, deformable or rigid, is an important task, with applications ranging from Human-Computer Interaction to environmental understanding. The idea of a general pose estimation framework, capable of being rapidly retrained to suit a variety of tasks, is appealing. In this paper a solution is proposed requiring only a set of labelled training images in order to be applied to many pose estimation tasks. This is achieved by treating pose estimation as a classification problem, with particle filtering used to provide non-discretised estimates. Depth information extracted from a calibrated stereo sequence, is used for background suppression and object scale estimation. The appearance and shape channels are then transformed to Local Binary Pattern histograms, and pose classification is performed via a randomised decision forest. To demonstrate flexibility, the approach is applied to two different situations, articulated hand pose and rigid head orientation, achieving 97% and 84% accurate estimation rates, respectively.
Most 3D reconstruction approaches passively optimise over all data, exhaustively matching pairs, rather than actively selecting data to process. This is costly both in terms of time and computer resources, and quickly becomes intractable for large datasets. This work proposes an approach to intelligently filter large amounts of data for 3D reconstructions of unknown scenes using monocular cameras. Our contributions are twofold: First, we present a novel approach to efficiently optimise the Next-Best View ( NBV ) in terms of accuracy and coverage using partial scene geometry. Second, we extend this to intelligently selecting stereo pairs by jointly optimising the baseline and vergence to find the NBV ’s best stereo pair to perform reconstruction. Both contributions are extremely efficient, taking 0.8ms and 0.3ms per pose, respectively. Experimental evaluation shows that the proposed method allows efficient selection of stereo pairs for reconstruction, such that a dense model can be obtained with only a small number of images. Once a complete model has been obtained, the remaining computational budget is used to intelligently refine areas of uncertainty, achieving results comparable to state-of-the-art batch approaches on the Middlebury dataset, using as little as 3.8% of the views.
Sign language and Web 2.0 applications are currently incompatible, because of the lack of anonymisation and easy editing of online sign language contributions. This paper describes Dicta-Sign, a project aimed at developing the technologies required for making sign language-based Web contributions possible, by providing an integrated framework for sign language recognition, animation, and language modelling. It targets four different European sign languages: Greek, British, German, and French. Expected outcomes are three showcase applications for a search-by-example sign language dictionary, a sign language-to-sign language translator, and a sign language-based Wiki.
This paper introduces a fully-automated, unsupervised method to recognise sign from subtitles. It does this by using data mining to align correspondences in sections of videos. Based on head and hand tracking, a novel temporally constrained adaptation of apriori mining is used to extract similar regions of video, with the aid of a proposed contextual negative selection method. These regions are refined in the temporal domain to isolate the occurrences of similar signs in each example. The system is shown to automatically identify and segment signs from standard news broadcasts containing a variety of topics.
This paper proposes a generic approach combining a bottom-up (low-level) visual detector with a top-down (high-level) fuzzy first-order logic (FOL) reasoning framework in order to detect pedestrians from a moving vehicle. Detections from the low-level visual corner based detector are fed into the logical reasoning framework as logical facts. A set of FOL clauses utilising fuzzy predicates with piecewise linear continuous membership functions associates a fuzzy confidence (a degree-of-truth) to each detector input. Detections associated with lower confidence functions are deemed as false positives and blanked out, thus adding top-down constraints based on global logical consistency of detections. We employ a state of the art visual detector on a challenging pedestrian detection dataset, and demonstrate an increase in detection performance when used in a framework that combines bottom-up detections with (fuzzy FOL-based) top-down constraints. © 2012 ICPR Org Committee.
This paper presents a scalable solution to the problem of tracking objects across spatially separated, uncalibrated, non-overlapping cameras. Unlike other approaches this technique uses an incremental learning method, to model both the colour variations and posterior probability distributions of spatio-temporal links between cameras. These operate in parallel and are then used with an appearance model of the object to track across spatially separated cameras. The approach requires no pre-calibration or batch preprocessing, is completely unsupervised, and becomes more accurate over time as evidence is accumulated.
This article proposes an approach to learning steering and road following behaviour from a human driver using holistic visual features. We use a random forest (RF) to regress a mapping between these features and the driver's actions, and propose an alternative to classical random forest regression based on the Medoid (RF-Medoid), that reduces the underestimation of extreme control values. We compare prediction performance using different holistic visual descriptors: GIST, Channel-GIST (C-GIST) and Pyramidal-HOG (P-HOG). The proposed methods are evaluated on two different datasets: predicting human behaviour on countryside roads and also for autonomous control of a robot on an indoor track. We show that 1) C-GIST leads to the best predictions on both sequences, and 2) RF-Medoid leads to a better estimation of extreme values, where a classical RF tends to under-steer. We use around 10% of the data for training and show excellent generalization over a dataset of thousands of images. Importantly, we do not engineer the solution but instead use machine learning to automatically identify the relationship between visual features and behaviour, providing an efficient, generic solution to autonomous control.
This work presents our recent advances in the field of automatic processing of sign language corpora targeting continuous sign language recognition. We demonstrate how generic annotations at the articulator level, such as HamNoSys, can be exploited to learn subunit classifiers. Specifically, we explore cross-language-subunits of the hand orientation modality, which are trained on isolated signs of publicly available lexicon data sets for Swiss German and Danish Sign Language and are applied to continuous sign language recognition of the challenging RWTH-PHOENIX-Weather corpus featuring German Sign Language. We observe a significant reduction in word error rate using this method.
Real-time segmentation of moving regions in image sequences is a fundamental step in many vision systems including automated visual surveillance, human-machine interface, and very low-bandwidth telecommunications. A typical method is background subtraction. Many background models have been introduced to deal with different problems. One of the successful solutions to these problems is to use a multi-colour background model per pixel proposed by Grimson et al [1, 2,3]. However, the method suffers from slow learning at the beginning, especially in busy environments. In addition, it can not distinguish between moving shadows and moving objects. This paper presents a method which improves this adaptive background mixture model. By reinvestigating the update equations, we utilise different equations at different phases. This allows our system learn faster and more accurately as well as adapts effectively to changing environment. A shadow detection scheme is also introduced in this paper. It is based on a computational colour space that makes use of our background model. A comparison has been made between the two algorithms. The results show the speed of learning and the accuracy of the model using our update algorithm over the Grimson et al’s tracker. When incorporate with the shadow detection, our method results in far better segmentation than The Thirteenth Conference on Uncertainty in Artificial Intelligence that of Grimson et al.
The Visual Object Tracking challenge 2014, VOT2014, aims at comparing short-term single-object visual trackers that do not apply pre-learned models of object appearance. Results of 38 trackers are presented. The number of tested trackers makes VOT 2014 the largest benchmark on short-term tracking to date. For each participating tracker, a short description is provided in the appendix. Features of the VOT2014 challenge that go beyond its VOT2013 predecessor are introduced: (i) a new VOT2014 dataset with full annotation of targets by rotated bounding boxes and per-frame attribute, (ii) extensions of the VOT2013 evaluation methodology, (iii) a new unit for tracking speed assessment less dependent on the hardware and (iv) the VOT2014 evaluation toolkit that significantly speeds up execution of experiments. The dataset, the evaluation kit as well as the results are publicly available at the challenge website.
This paper introduces the end-to-end embedding of a CNN into a HMM, while interpreting the outputs of the CNN in a Bayesian fashion. The hybrid CNN-HMM combines the strong discriminative abilities of CNNs with the sequence modelling capabilities of HMMs. Most current approaches in the field of gesture and sign language recognition disregard the necessity of dealing with sequence data both for training and evaluation. With our presented end-to-end embedding we are able to improve over the state-of-the-art on three challenging benchmark continuous sign language recognition tasks by between 15% and 38% relative and up to 13.3% absolute.
Sign Language Recognition (SLR) has been an active research field for the last two decades. However, most research to date has considered SLR as a naive gesture recognition problem. SLR seeks to recognize a sequence of continuous signs but neglects the underlying rich grammatical and linguistic structures of sign language that differ from spoken language. In contrast, we introduce the Sign Language Translation (SLT) problem. Here, the objective is to generate spoken language translations from sign language videos, taking into account the different word orders and grammar. We formalize SLT in the framework of Neural Machine Translation (NMT) for both end-to-end and pretrained settings (using expert knowledge). This allows us to jointly learn the spatial representations, the underlying language model, and the mapping between sign and spoken language. To evaluate the performance of Neural SLT, we collected the first publicly available Continuous SLT dataset, RWTHPHOENIX-Weather 2014T1. It provides spoken language translations and gloss level annotations for German Sign Language videos of weather broadcasts. Our dataset contains over .95M frames with >67K signs from a sign vocabulary of >1K and >99K words from a German vocabulary of >2.8K. We report quantitative and qualitative results for various SLT setups to underpin future research in this newly established field. The upper bound for translation performance is calculated at 19.26 BLEU-4, while our end-to-end frame-level and gloss-level tokenization networks were able to achieve 9.58 and 18.13 respectively.
The Visual Object Tracking challenge 2014, VOT2014, aims at comparing short-term single-object visual trackers that do not apply pre-learned models of object appearance. Results of 38 trackers are presented. The number of tested trackers makes VOT 2014 the largest benchmark on short-term tracking to date. For each participating tracker, a short description is provided in the appendix. Features of the VOT2014 challenge that go beyond its VOT2013 predecessor are introduced: (i) a new VOT2014 dataset with full annotation of targets by rotated bounding boxes and per-frame attribute, (ii) extensions of the VOT2013 evaluation methodology, (iii) a new unit for tracking speed assessment less dependent on the hardware and (iv) the VOT2014 evaluation toolkit that significantly speeds up execution of experiments. The dataset, the evaluation kit as well as the results are publicly available at the challenge website (http://votchallenge.net).
This paper discusses sign language recognition using linguistic sub-units. It presents three types of sub-units for consideration; those learnt from appearance data as well as those inferred from both 2D or 3D tracking data. These sub-units are then combined using a sign level classifier; here, two options are presented. The first uses Markov Models to encode the temporal changes between sub-units. The second makes use of Sequential Pattern Boosting to apply discriminative feature selection at the same time as encoding temporal information. This approach is more robust to noise and performs well in signer independent tests, improving results from the 54% achieved by the Markov Chains to 76%.
The Visual Object Tracking challenge VOT2016 aims at comparing short-term single-object visual trackers that do not apply pre-learned models of object appearance. Results of 70 trackers are presented, with a large number of trackers being published at major computer vision conferences and journals in the recent years. The number of tested state-of-the-art trackers makes the VOT 2016 the largest and most challenging benchmark on short-term tracking to date. For each participating tracker, a short description is provided in the Appendix. The VOT2016 goes beyond its predecessors by (i) introducing a new semi-automatic ground truth bounding box annotation methodology and (ii) extending the evaluation system with the no-reset experiment. The dataset, the evaluation kit as well as the results are publicly available at the challenge website (http:// votchallenge. net).
Grasping is one of the oldest problems in robotics and is still considered challenging, especially when grasping unknown objects with unknown 3D shape. We focus on exploiting recent advances in computer vision recognition systems. Object classification problems tend to have much larger datasets to train from and have far fewer practical constraints around the size of the model and speed to train. In this paper we will investigate how to adapt Convolutional Neural Networks (CNNs), traditionally used for image classification, for planar robotic grasping. We consider the differences in the problems and how a network can be adjusted to account for this. Positional information is far more important to robotics than generic image classification tasks, where max pooling layers are used to improve translation invariance. By using a more appropriate network structure we are able to obtain improved accuracy while simultaneously improving run times and reducing memory consumption by reducing model size by up to 69%.
Although there have been some recent advances in sign language recognition, part of the problem is that most computer scientists in this research area do not have the required in-depth knowledge of sign language, and often have no connection with the Deaf community or sign linguists. For example, one project described as translation into sign language aimed to take subtitles and turn them into fingerspelling. This is one of many reasons why much of this technology, including sign-language gloves, simply doesn’t address the challenges. However there are benefits to achieving automatic sign language recognition. The process of annotating and analysing sign language data on video is extremely labour-intensive. Sign language recognition technology could help speed this up. Until recently we have lacked large signed video datasets that have been precisely and consistently transcribed and translated – these are needed to train computers for automation. But sign language corpora – large datasets like the British Sign Language Corpus (Schembri et al., 2014) - bring new possibilities for this technology. Here we describe the project “ExTOL: End to End Translation of British Sign Language” – which has one aim of building the world's first British Sign Language to English translation system and the first practically functional machine translation system for any sign language. Annotation work previously done on the BSL Corpus is providing essential data to be used by computer vision tools to assist with automatic recognition. To achieve this the computer must be able to recognise not only the shape, motion and location of the hands but also nonmanual features – including facial expression, mouth movements, and body posture of the signer. It must also understand how all of this activity in connected signing can be translated into written/spoken language. The technology for recognising hand, face and body positions and movements is improving all the time, which will allow significant progress in speeding up automatic recognition and identification of these elements (e.g. recognising specific facial expressions or mouth movements or head movements). Full translation from BSL to English is of course more complex but the automatic recognition of basic position and movements will help pave the way towards automatic translation. In addition, a secondary aim of the project is to create automatic annotation tools to be integrated into the software annotation package ELAN. We will additionally make the software tools available as independent packages, thus potentially allowing their inclusion into other annotation software such as iLex. In this poster we report on some initial progress on the ExTOL project. This includes (1) automatic recognition of English mouthings, which is being trained using 600+ hours of audiovisual spoken English from British television and TED videos, and developed by testing on English mouthing annotations from the BSL Corpus. It also includes (2) translation of IDGloss to Free Translation, where the aim is to produce English-like sentences given sign glosses in BSL word order. We report baseline results on a subset of the BSL Corpus, which contains 10,000+ sequences and over 5,000 unique tokens, using the state-of-the-art attention based Neural Machine Translation approaches (Camgoz et al., 2018; Vaswani et al., 2017). Although it is clear that free translation (i.e. full English translation) cannot be achieved via ID glosses alone, this baseline translation task will help contribute to the overall BSL to English translation process - at least at the level of manual signs.
Here we present the outcomes of Dicta-Sign FP7-ICT project. Dicta-Sign researched ways to enable communication between Deaf individuals through the development of human-computer interfaces (HCI) for Deaf users, by means of Sign Language. It has researched and developed recognition and synthesis engines for sign languages (SLs) that have brought sign recognition and generation technologies significantly closer to authentic signing. In this context, Dicta-Sign has developed several technologies demonstrated via a sign language aware Web 2.0, combining work from the fields of sign language recognition, sign language animation via avatars and sign language resources and language models development, with the goal of allowing Deaf users to make, edit, and review avatar-based sign language contributions online, similar to the way people nowadays make text-based contributions on the Web.
We present a novel approach to automatic Sign Language Production using stateof- the-art Neural Machine Translation (NMT) and Image Generation techniques. Our system is capable of producing sign videos from spoken language sentences. Contrary to current approaches that are dependent on heavily annotated data, our approach requires minimal gloss and skeletal level annotations for training. We achieve this by breaking down the task into dedicated sub-processes. We first translate spoken language sentences into sign gloss sequences using an encoder-decoder network. We then find a data driven mapping between glosses and skeletal sequences. We use the resulting pose information to condition a generative model that produces sign language video sequences. We evaluate our approach on the recently released PHOENIX14T Sign Language Translation dataset. We set a baseline for text-to-gloss translation, reporting a BLEU-4 score of 16.34/15.26 on dev/test sets. We further demonstrate the video generation capabilities of our approach by sharing qualitative results of generated sign sequences given their skeletal correspondence.
For automatic lipreading, there are many competing methods for feature extraction. Often, because of the complexity of the task these methods are tested on only quite restricted datasets, such as the letters of the alphabet or digits, and from only a few speakers. In this paper we compare some of the leading methods for lip feature extraction and compare them on the GRID dataset which uses a constrained vocabulary over, in this case, 15 speakers. Previously the GRID data has had restricted attention because of the requirements to track the face and lips accurately. We overcome this via the use of a novel linear predictor (LP) tracker which we use to control an Active Appearance Model (AAM). By ignoring shape and/or appearance parameters from the AAM we can quantify the effect of appearance and/or shape when lip-reading. We find that shape alone is a useful cue for lipreading (which is consistent with human experiments). However, the incremental effect of shape on appearance appears to be not significant which implies that the inner appearance of the mouth contains more information than the shape.
Disentangled representations support a range of downstream tasks including causal reasoning, generative modeling, and fair machine learning. Unfortunately, disentanglement has been shown to be impossible without the incorporation of supervision or inductive bias. Given that supervision is often expensive or infeasible to acquire, we choose to incorporate structural inductive bias and present an unsupervised, deep State-Space-Model for Video Disentanglement (VDSM). The model disentangles latent time-varying and dynamic factors via the incorporation of hierarchical structure with a dynamic prior and a Mixture of Experts decoder. VDSM learns separate disentangled representations for the identity of the object or person in the video, and for the action being performed. We evaluate VDSM across a range of qualitative and quantitative tasks including identity and dynamics transfer, sequence generation, Fréchet Inception Distance, and factor classification. VDSM achieves state-of-the-art performance and exceeds adversarial methods, even when the methods use additional supervision.
Meaningful Non-Verbal Communication (NVC) signals can be recognised by facial deformations based on video tracking. However, the geometric features previously used contain a significant amount of redundant or irrelevant information. A feature selection method is described for selecting a subset of features that improves performance and allows for the identification and visualisation of facial areas involved in NVC. The feature selection is based on a sequential backward elimination of features to find a effective subset of components. This results in a significant improvement in recognition performance, as well as providing evidence that brow lowering is involved in questioning sentences. The improvement in performance is a step towards a more practical automatic system and the facial areas identified provide some insight into human behaviour.
Deep learning is a promising class of techniques for controlling an autonomous vehicle. However, functional safety validation is seen as a critical issue for these systems due to the lack of transparency in deep neural networks and the safety-critical nature of autonomous vehicles. The black box nature of deep neural networks limits the effectiveness of traditional verification and validation methods. In this paper, we propose two software safety cages, which aim to limit the control action of the neural network to a safe operational envelope. The safety cages impose limits on the control action during critical scenarios, which if breached, change the control action to a more conservative value. This has the benefit that the behaviour of the safety cages is interpretable, and therefore traditional functional safety validation techniques can be applied. The work here presents a deep neural network trained for longitudinal vehicle control, with safety cages designed to prevent forward collisions. Simulated testing in critical scenarios shows the effectiveness of the safety cages in preventing forward collisions whilst under normal highway driving unnecessary interruptions are eliminated, and the deep learning control policy is able to perform unhindered. Interventions by the safety cages are also used to re-train the network, resulting in a more robust control policy.
Recognition of non-verbal communication (NVC) is important for understanding human communication and designing user centric user interfaces. Cultural differences affect the expression and perception of NVC but no previous automatic system considers these cultural differences. Annotation data for the LILiR TwoTalk corpus, containing dyadic (two person) conversations, was gathered using Internet crowdsourcing, with a significant quantity collected from India, Kenya and the United Kingdom (UK). Many studies have investigated cultural differences based on human observations but this has not been addressed in the context of automatic emotion or NVC recognition. Perhaps not surprisingly, testing an automatic system on data that is not culturally representative of the training data is seen to result in low performance. We address this problem by training and testing our system on a specific culture to enable better modeling of the cultural differences in NVC perception. The system uses linear predictor tracking, with features generated based on distances between pairs of trackers. The annotations indicated the strength of the NVC which enables the use of v-SVR to perform the regression.
The availability of video format sign language corpora limited. This leads to a desire for techniques which do not rely on large, fully-labelled datasets. This paper covers various methods for learning sign either from small data sets or from those without ground truth labels. To avoid non-trivial tracking issues; sign detection is investigated using volumetric spatio-temporal features. Following this the advantages of recognising the component parts of sign rather than the signs themselves is demonstrated and finally the idea of using a weakly labelled data set is considered and results shown for work in this area.
Machine Learning for Human Motion Analysis: Theory and Practice highlights thedevelopment of robust and effective vision-based motion understanding systems.