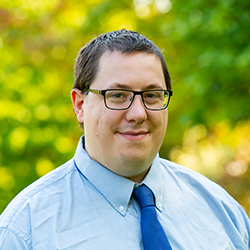
Dr Simon Hadfield
Academic and research departments
Centre for Vision, Speech and Signal Processing (CVSSP), School of Computer Science and Electronic Engineering.About
Biography
My research focuses on using cutting-edge visual sensing technologies such as event cameras to solve machine learning/autonomy and 3D computer vision tasks.
I am a lecturer in robot vision and autonomous systems within the University of Surrey’s Centre for Vision, Speech and Signal Processing (CVSSP). My long-term aim is to develop the first UK centre of excellence in perception and AI algorithms for asynchronous visual sensing.
Having studied for an MEng in Electronic and Computer Engineering at Surrey (finishing as the top student in my graduating year), I went on to do an EPSRC-funded PhD in computer vision at the University. Supervised by Professor Richard Bowden, this was entitled ‘The estimation and use of 3D information, for natural human action recognition’. I remained at Surrey as a Research Fellow and became a lecturer in February 2016.
Areas of specialism
University roles and responsibilities
- Manager of Dissertation allocation and assessment system for undergraduate and postgraduate taught programmes in the Department of Electrical and Electronic Engineering.
- Undergraduate Personal Tutor
- Health and Safety group – Representative for CVSSP labs (BA)
My qualifications
Affiliations and memberships
News
ResearchResearch interests
My research is in the field of computer vision and machine learning, with a particular emphasis on the effective exploitation of novel visual sensors.
I focus on taking computer vision techniques ‘out of the lab’ and making them practically applicable to the real world. For example, I’ve proposed a new paradigm for efficient dynamic reconstruction with a computational overhead several orders of magnitude lower than previous techniques, which is an important step towards using these techniques in real-time robotics applications. This research was published in both the top journal and top international conference in the field, generating more than 100 citations to date.
Ultimately, my research in robotic perception and automation could impact a range of areas of modern life. In the manufacturing industry, automation techniques are urgently needed to reduce costs and enable manufacturers to adapt to changing environments, while autonomous vehicles require new types of visual sensor and perception algorithms to push safety to human levels and beyond. Medical robotics needs increasing levels of intelligence and automation in order to proceed beyond the capabilities of the human doctors operating them. And in the space sector, spaceborne robotics – which allow in-orbit assembly and servicing – are necessitating new approaches to visual perception.
Research projects
NIMROD: Analogue visual sensors’Industrial project exploring perception algorithms for a new breed of adaptive visual sensor
ROSSINI: Reconstructing 3D structure from single images: a perceptual reconstruction approachEPSRC project looking at 3D reconstruction techniques which maximize human perceptual quality
PROTEIN: PeRsOnalized nutriTion for hEalthy livingEU Horizon2020 project looking at automated machine learning and vision technologies for personal nutrition monitoring. Partners include: The University of Brussels, Ocado, University of Thessaloniki, Centre for Research and Technology Hellas and others
Driver behaviour modelling for assistance & automationIndustrial project with McLaren Automotive, exploring deep learning for driver monitoring, and the correlations with external driving factors
SMILE: Scalable Multimodal Sign Language Technology for Sign Language Learning and AssessmentSwiss National Science Foundation project exploring technologies to automate learning and practicing sign language at home
Industrial project with Tesco Labs looking at intelligent grasping and manipulation of warehouse packing
Industrial project looking at automation of marine vessels
Learning to Recognise Dynamic Visual Content from Broadcast FootageEPSRC project exploring machine learning for automatically understanding TV footage, for search and archival purposes
The Internet of SurpriseEPSRC eFutures funding sandpit demonstrator project
Indicators of esteem
2018 – Winner of the Early Career Teacher of the Year (Faculty of Engineering & Physical Sciences )
Reviewer for more than 10 high impact international journals and conferences
Two NVidia GPU grants
2017 – Finalist for Supervisor of the Year (Faculty of Engineering & Physical Sciences) and the Tony Jeans Inspirational Teaching award
2016 – Second place in the international academic challenge on continuous gesture recognition (ChaLearn)
DTI MEng prize, for best all round performance of the entire graduating year, awarded by Department of Trade and Industry
Research interests
My research is in the field of computer vision and machine learning, with a particular emphasis on the effective exploitation of novel visual sensors.
I focus on taking computer vision techniques ‘out of the lab’ and making them practically applicable to the real world. For example, I’ve proposed a new paradigm for efficient dynamic reconstruction with a computational overhead several orders of magnitude lower than previous techniques, which is an important step towards using these techniques in real-time robotics applications. This research was published in both the top journal and top international conference in the field, generating more than 100 citations to date.
Ultimately, my research in robotic perception and automation could impact a range of areas of modern life. In the manufacturing industry, automation techniques are urgently needed to reduce costs and enable manufacturers to adapt to changing environments, while autonomous vehicles require new types of visual sensor and perception algorithms to push safety to human levels and beyond. Medical robotics needs increasing levels of intelligence and automation in order to proceed beyond the capabilities of the human doctors operating them. And in the space sector, spaceborne robotics – which allow in-orbit assembly and servicing – are necessitating new approaches to visual perception.
Research projects
Industrial project exploring perception algorithms for a new breed of adaptive visual sensor
EPSRC project looking at 3D reconstruction techniques which maximize human perceptual quality
EU Horizon2020 project looking at automated machine learning and vision technologies for personal nutrition monitoring. Partners include: The University of Brussels, Ocado, University of Thessaloniki, Centre for Research and Technology Hellas and others
Industrial project with McLaren Automotive, exploring deep learning for driver monitoring, and the correlations with external driving factors
Swiss National Science Foundation project exploring technologies to automate learning and practicing sign language at home
Industrial project with Tesco Labs looking at intelligent grasping and manipulation of warehouse packing
Industrial project looking at automation of marine vessels
EPSRC project exploring machine learning for automatically understanding TV footage, for search and archival purposes
EPSRC eFutures funding sandpit demonstrator project
Indicators of esteem
2018 – Winner of the Early Career Teacher of the Year (Faculty of Engineering & Physical Sciences )
Reviewer for more than 10 high impact international journals and conferences
Two NVidia GPU grants
2017 – Finalist for Supervisor of the Year (Faculty of Engineering & Physical Sciences) and the Tony Jeans Inspirational Teaching award
2016 – Second place in the international academic challenge on continuous gesture recognition (ChaLearn)
DTI MEng prize, for best all round performance of the entire graduating year, awarded by Department of Trade and Industry
Supervision
Postgraduate research supervision
Expected 2027 - Enis Baty - Satellite assisted autonomous vehicles
Expected 2027 - Isaac Baglin - Preventing deep leakage in federated learning
Expected 2027 - Alejandro Hernandez Diaz - Event-based vision for space situational awareness
Expected 2026 - Chris Thirgood - Raman spectroscopy for robot localization
Expected 2026 - Rogier Fransen - Learning to walk over complex terrain
Expected 2026 - Bucher Sahyouni - Fair and equitable recommender systems
Expected 2024 - Nikolina Kubiak - Relighting in video
Expected 2023 - Herman Yau - Explainable reinforcement learning
Expected 2023 - Violeta Menendez Gonzalez - Novel-view synthesis in video
Completed postgraduate research projects I have supervised
2023 - Xihan Bian - Multi-task autonomy for Robotics
2023 - Yusuf Duman - Active Sampling for Computer Vision
2022 - Lucy Elaine Jackson - Using Reinforcement Learning to Design and Control Free-Flying Space Robots
2022 - Jaime Spencer Martin - Learning Generic Deep Feature Representations
2021 - Rebecca Allday - Machine Learning for Robotic Grasping
2021 - Peter Blacker - Optimal Use of Machine Learning for Planetary Terrain Navigation
2020 - Celyn Walters - Extrinsic sensor calibration systems and methods
2020 - N. Cihan Camgöz - Neural Sign Language Translation: Continuous Sign Language REcognition from a Machine Translation Perspective (PGR student of the year - VC awards 2018)
2018 - Matthew Marter - Learning to Recognise Visual Content from Textual Annotation
2017- Oscar Mendez - Collaborative Strategies for Autonomous Localisation, 3D Reconstruction and Pathplanning (Sullivan Thesis Prize finalist, top UK thesis in computer vision)
2016 - Karel Lebeda - 2D and 3D Tracking and Modelling (Sullivan Thesis Prize winner, top UK thesis in computer vision)
Teaching
I currently teach two modules to undergraduates in the Department of Electrical and Electronic Engineering:
- C++ and Object-Oriented Design: Year 2 (EEE2047)
- Robotics: Year 3 (EEE3043)
In my teaching, I focus heavily on practical hands-on experience interleaved with the traditional taught material, to ensure that students have the chance to practice and receive feedback on the techniques they are learning.
Every year I also supervise around four undergraduate projects in the areas of deep learning, robotics and computer vision. Historically, the projects I have helped supervise have often won or been nominated for external and internal prizes including the BAE prize, national ‘hackaday’ prizes and Surrey’s Department of Electrical and Electronic Engineering prize.
I have received a number of awards and nominations for my supervision and teaching. These include winning the 2018 Early Career Teacher of the Year and being nominated for the 2017 Tony Jeans Inspiration Teaching award.
Publications
We introduce HyperGS, a novel framework for Hyperspectral Novel View Synthesis (HNVS), based on a new latent 3D Gaussian Splatting (3DGS) technique. Our approach enables simultaneous spatial and spectral renderings by encoding material properties from multi-view 3D hyperspectral datasets. HyperGS reconstructs high-fidelity views from arbitrary perspectives with improved accuracy and speed, outperforming currently existing methods. To address the challenges of high-dimensional data, we perform view synthesis in a learned latent space, incorporating a pixel-wise adaptive density function and a pruning technique for increased training stability and efficiency. Additionally, we introduce the first HNVS benchmark, implementing a number of new baselines based on recent SOTA RGB-NVS techniques, alongside the small number of prior works on HNVS. We demonstrate HyperGS's robustness through extensive evaluation of real and simulated hyperspectral scenes with a 14dB accuracy improvement upon previously published models.
We introduce HyperGS, a novel framework for Hyperspectral Novel View Synthesis (HNVS), based on a new latent 3D Gaussian Splatting (3DGS) technique. Our approach enables simultaneous spatial and spectral renderings by encoding material properties from multi-view 3D hyperspectral datasets. HyperGS reconstructs high-fidelity views from arbitrary perspectives with improved accuracy and speed, outperforming currently existing methods. To address the challenges of high-dimensional data, we perform view synthesis in a learned latent space, incorporating a pixel-wise adaptive density function and a pruning technique for increased training stability and efficiency. Additionally, we introduce the first HNVS benchmark, implementing a number of new baselines based on recent SOTA RGB-NVS techniques, alongside the small number of prior works on HNVS. We demonstrate HyperGS's robustness through extensive evaluation of real and simulated hyperspectral scenes with a 14dB accuracy improvement upon previously published models.
The ability to generalize to previously unseen tasks with little to no supervision is a key challenge in modern machine learning research. It is also a cornerstone of a future "General AI". Any artificially intelligent agent deployed in a real world application, must adapt on the fly to unknown environments. Researchers often rely on reinforcement and imitation learning to provide online adaptation to new tasks, through trial and error learning. However, this can be challenging for complex tasks which require many timesteps or large numbers of subtasks to complete. These "long horizon" tasks suffer from sample inefficiency and can require extremely long training times before the agent can learn to perform the necessary longterm planning. In this work, we introduce CASE which attempts to address these issues by training an Imitation Learning agent using adaptive "near future" subgoals. These subgoals are recalculated at each step using compositional arithmetic in a learned latent representation space. In addition to improving learning efficiency for standard long-term tasks, this approach also makes it possible to perform one-shot generalization to previously unseen tasks, given only a single reference trajectory for the task in a different environment. Our experiments show that the proposed approach consistently outperforms the previous state-of-the-art compositional Imitation Learning approach by 30%.
Cross-view Geo-localisation is typically performed at a coarse granularity, because densely sampled satellite image patches overlap heavily. This heavy overlap would make dis-ambiguating patches very challenging. However, by opting for sparsely sampled patches, prior work has placed an artificial upper bound on the localisation accuracy that is possible. Even a perfect oracle system cannot achieve accuracy greater than the average separation of the tiles. To solve this limitation, we propose combining cross-view geo-localisation and relative pose estimation to increase precision to a level practical for real-world application. We develop PEnG, a 2-stage system which first predicts the most likely edges from a city-scale graph representation upon which a query image lies. It then performs relative pose estimation within these edges to determine a precise position. PEnG presents the first technique to utilise both viewpoints available within cross-view geo-localisation datasets, referring to this as Multi-View Geo-Localisation (MVGL). This enhances accuracy to a sub-metre level, with some examples achieving centimetre level precision. Our proposed ensemble achieves state-of-the-art accuracy-with relative Top-5m retrieval improvements on previous works of 213%. Decreasing the median Euclidean distance error by 96.90% from the previous best of 734m down to 22.77m, when evaluating with 90° horizontal FOV images. Code is available here: github.com/tavisshore/peng.
Self-supervised monocular depth estimation (SS-MDE) has the potential to scale to vast quantities of data. Unfortunately, existing approaches limit themselves to the automotive domain, resulting in models incapable of generalizing to complex environments such as natural or indoor settings. To address this, we propose a large-scale SlowTV dataset curated from YouTube, containing an order of magnitude more data than existing automotive datasets. SlowTV contains 1.7M images from a rich diversity of environments, such as worldwide seasonal hiking, scenic driving and scuba diving. Using this dataset, we train an SS-MDE model that provides zero-shot generalization to a large collection of indoor/outdoor datasets. The resulting model outperforms all existing SSL approaches and closes the gap on supervised SoTA, despite using a more efficient architecture. We additionally introduce a collection of best-practices to further maximize performance and zero-shot generalization. This includes 1) aspect ratio augmentation, 2) camera intrinsic estimation, 3) support frame randomization and 4) flexible motion estimation.
This paper presents an open and comprehensive framework to systematically evaluate state-of-the-art contributions to self-supervised monocular depth estimation. This includes pretraining, backbone, architectural design choices and loss functions. Many papers in this field claim novelty in either architecture design or loss formulation. However, simply updating the backbone of historical systems results in relative improvements of 25%, allowing them to outperform the majority of existing systems. A systematic evaluation of papers in this field was not straightforward. The need to compare like-with-like in previous papers means that longstanding errors in the evaluation protocol are ubiquitous in the field. It is likely that many papers were not only optimized for particular datasets, but also for errors in the data and evaluation criteria. To aid future research in this area, we release a modular codebase (this https URL), allowing for easy evaluation of alternate design decisions against corrected data and evaluation criteria. We re-implement, validate and re-evaluate 16 state-of-the-art contributions and introduce a new dataset (SYNS-Patches) containing dense outdoor depth maps in a variety of both natural and urban scenes. This allows for the computation of informative metrics in complex regions such as depth boundaries.
Cross-view image matching for geo-localisation is a challenging problem due to the significant visual difference between aerial and ground-level viewpoints. The method provides localisation capabilities from geo-referenced images, eliminating the need for external devices or costly equipment. This enhances the capacity of agents to autonomously determine their position, navigate, and operate effectively in GNSS-denied environments. Current research employs a variety of techniques to reduce the domain gap such as applying polar transforms to aerial images or synthesising between perspectives. However, these approaches generally rely on having a 360 degree field of view, limiting real-world feasibility. We propose BEV-CV, an approach introducing two key novelties with a focus on improving the real-world viability of cross-view geo-localisation. Firstly bringing ground-level images into a semantic Birds-Eye-View before matching embeddings, allowing for direct comparison with aerial image representations. Secondly, we adapt datasets into application realistic format-limited-FOV images aligned to vehicle direction. BEV-CV achieves state-of-the-art recall accuracies, improving Top-1 rates of 70 degree crops of CVUSA and CVACT by 23% and 24% respectively. Also decreasing computational requirements by reducing floating point operations to below previous works, and decreasing embedding dimensionality by 33%-together allowing for faster localisation capabilities.
Cross-View Geo-Localisation within urban regions is challenging in part due to the lack of geo-spatial struc-turing within current datasets and techniques. We propose utilising graph representations to model sequences of local observations and the connectivity of the target location. Modelling as a graph enables generating previously unseen sequences by sampling with new parameter configurations. To leverage this newly available information , we propose a GNN-based architecture, producing spatially strong embeddings and improving discriminabil-ity over isolated image embeddings. We outline SpaG-BOL, introducing three novel contributions. 1) The first graph-structured dataset for Cross-View Geo-Localisation, containing multiple streetview images per node to improve generalisation. 2) Introducing GNNs to the problem, we develop the first system that exploits the correlation between node proximity and feature similarity. 3) Lever-aging the unique properties of the graph representation-we demonstrate a novel retrieval filtering approach based on neighbourhood bearings. SpaGBOL achieves state-of-the-art accuracies on the unseen test graph-with relative Top-1 retrieval improvements on previous techniques of 11%, and 50% when filtering with Bearing Vector Matching on the SpaGBOL dataset. Code and dataset available: github.com/tavisshore/SpaGBOL.
Self-supervised monocular depth estimation (SS-MDE) has the potential to scale to vast quantities of data. Unfortunately, existing approaches limit themselves to the automotive domain, resulting in models incapable of generalizing to complex environments such as natural or indoor settings. To address this, we propose a large-scale SlowTV dataset curated from YouTube, containing an order of magnitude more data than existing automotive datasets. SlowTV contains 1.7M images from a rich diversity of environments, such as worldwide seasonal hiking, scenic driving and scuba diving. Using this dataset, we train an SS-MDE model that provides zero-shot generalization to a large collection of indoor/outdoor datasets. The resulting model outperforms all existing SSL approaches and closes the gap on supervised SoTA, despite using a more efficient architecture. We additionally introduce a collection of best-practices to further maximize performance and zero-shot generalization. This includes 1) aspect ratio augmentation, 2) camera intrinsic estimation, 3) support frame randomization and 4) flexible motion estimation. Code is available at https://github.com/jspenmar/slowtv_monodepth.
Sign language recognition (SLR) involves identifying the form and meaning of isolated signs or sequences of signs. To our knowledge, the combination of SLR and sign language assessment is novel. The goal of an ongoing three-year project in Switzerland is to pioneer an assessment system for lexical signs of Swiss German Sign Language (Deutschschweizerische Gebardensprache, DSGS) that relies on SLR. The assessment system aims to give adult L2 learners of DSGS feedback on the correctness of the manual parameters (handshape, hand position, location, and movement) of isolated signs they produce. In its initial version, the system will include automatic feedback for a subset of a DSGS vocabulary production test consisting of 100 lexical items. To provide the SLR component of the assessment system with sufficient training samples, a large-scale dataset containing videotaped repeated productions of the 100 items of the vocabulary test with associated transcriptions and annotations was created, consisting of data from 11 adult L1 signers and 19 adult L2 learners of DSGS. This paper introduces the dataset, which will be made available to the research community.
We present a novel form of explanation for Reinforcement Learning, based around the notion of intended outcome. These explanations describe the outcome an agent is trying to achieve by its actions. We provide a simple proof that general methods for post-hoc explanations of this nature are impossible in traditional reinforcement learning. Rather, the information needed for the explanations must be collected in conjunction with training the agent. We derive approaches designed to extract local explanations based on intention for several variants of Q-function approximation and prove consistency between the explanations and the Q-values learned. We demonstrate our method on multiple reinforcement learning problems, and provide code to help researchers introspecting their RL environments and algorithms.
This paper discusses the results for the second edition of the Monocular Depth Estimation Challenge (MDEC). This edition was open to methods using any form of supervision, including fully-supervised, self-supervised, multi-task or proxy depth. The challenge was based around the SYNS-Patches dataset, which features a wide diversity of environments with high-quality dense ground-truth. This includes complex natural environments, e.g. forests or fields, which are greatly underrepresented in current benchmarks. The challenge received eight unique submissions that outperformed the provided SotA baseline on any of the pointcloud- or image-based metrics. The top supervised submission improved relative F-Score by 27.62%, while the top self-supervised improved it by 16.61%. Supervised submissions generally leveraged large collections of datasets to improve data diversity. Self-supervised submissions instead updated the network architecture and pretrained backbones. These results represent a significant progress in the field, while highlighting avenues for future research, such as reducing interpolation artifacts at depth boundaries, improving self-supervised indoor performance and overall natural image accuracy.
This paper explores the potential of event cameras to enable continuous time reinforcement learning. We formalise this problem where a continuous stream of unsynchronised observations is used to produce a corresponding stream of output actions for the environment. This lack of synchronisation enables greatly enhanced reactivity. We present a method to train on event streams derived from standard RL environments, thereby solving the proposed continuous time RL problem. The CERiL algorithm uses specialised network layers which operate directly on an event stream, rather than aggregating events into quantised image frames. We show the advantages of event streams over less-frequent RGB images. The proposed system outperforms networks typically used in RL, even succeeding at tasks which cannot be solved traditionally. We also demonstrate the value of our CERiL approach over a standard SNN baseline using event streams.
The planning and execution of modern space missions rely on traditional SSA methods for detecting and tracking orbiting hazards. This often leads to sub-optimal responses due to remote sensing inaccuracies and transmission delays. On the other hand, deploying and maintaining space-based sensors is expensive and technically challenging due to the inadequacy of current vision technologies. In this paper, we propose a novel perception framework to enhance in-orbit autonomy and address the shortcomings of traditional SSA methods. We leverage the advances of neuromorphic cameras for a vastly superior sensing performance under space conditions. Additionally , we maximize the advantageous characteristics of the sensor by harnessing the modelling power and efficient design of selective State Space Models. Specifically, we introduce two novel event-based backbones, E-Mamba and E-Vim, for real-time on-board inference with linear scaling in complexity w.r.t. input length. Extensive evaluation across multiple neuromorphic datasets demonstrate the superior parameter efficiency or our approaches (
In this work, we present an end-to-end network for stereo-consistent image inpainting with the objective of inpainting large missing regions behind objects. The proposed model consists of an edge-guided UNet-like network using Partial Convolutions. We enforce multi-view stereo consistency by introducing a disparity loss. More importantly, we develop a training scheme where the model is learned from realistic stereo masks representing object occlusions, instead of the more common random masks. The technique is trained in a supervised way. Our evaluation shows competitive results compared to previous state-of-the-art techniques.
The successful performance of any system is dependant on the hardware of the agent, which is typically immutable during RL training. In this work, we present ORCHID (Optimisation of Robotic Control and Hardware In Design) which allows for truly simultaneous optimisation of hardware and control parameters in an RL pipeline. We show that by forming a complex differential path through a trajectory rollout we can leverage a vast amount of information from the system that was previously lost in the ‘black-box’ environment. Combining this with a novel hardware-conditioned critic network minimises variance during training and ensures stable updates are made. This allows for refinements to be made to both the morphology and control parameters simultaneously. The result is an efficient and versatile approach to holistic robot design, that brings the final system nearer to true optimality. We show improvements in performance across 4 different test environments with two different control algorithms - in all experiments the maximum performance achieved with ORCHID is shown to be unattainable using only policy updates with the default design. We also show how re-designing a robot using ORCHID in simulation, transfers to a vast improvement in the performance of a real-world robot.
Estimating the pose of an object, be it articulated, deformable or rigid, is an important task, with applications ranging from Human-Computer Interaction to environmental understanding. The idea of a general pose estimation framework, capable of being rapidly retrained to suit a variety of tasks, is appealing. In this paper a solution isproposed requiring only a set of labelled training images in order to be applied to many pose estimation tasks. This is achieved bytreating pose estimation as a classification problem, with particle filtering used to provide non-discretised estimates. Depth information extracted from a calibrated stereo sequence, is used for background suppression and object scale estimation. The appearance and shape channels are then transformed to Local Binary Pattern histograms, and pose classification is performed via a randomised decision forest. To demonstrate flexibility, the approach is applied to two different situations, articulated hand pose and rigid head orientation, achieving 97% and 84% accurate estimation rates, respectively.
In this paper, an algorithm is presented for estimating scene flow, which is a richer, 3D analogue of Optical Flow. The approach operates orders of magnitude faster than alternative techniques, and is well suited to further performance gains through parallelized implementation. The algorithm employs multiple hypothesis to deal with motion ambiguities, rather than the traditional smoothness constraints, removing oversmoothing errors and providing signi?cant performance improvements on benchmark data, over the previous state of the art. The approach is ?exible, and capable of operating with any combination of appearance and/or depth sensors, in any setup, simultaneously estimating the structure and motion if necessary. Additionally, the algorithm propagates information over time to resolve ambiguities, rather than performing an isolated estimation at each frame, as in contemporary approaches. Approaches to smoothing the motion ?eld without sacri?cing the bene?ts of multiple hypotheses are explored, and a probabilistic approach to Occlusion estimation is demonstrated, leading to 10% and 15% improved performance respectively. Finally, a data driven tracking approach is described, and used to estimate the 3D trajectories of hands during sign language, without the need to model complex appearance variations at each viewpoint.
We propose a novel deep learning approach to solve simultaneous alignment and recognition problems (referred to as “Sequence-to-sequence” learning). We decompose the problem into a series of specialised expert systems referred to as SubUNets. The spatio-temporal relationships between these SubUNets are then modelled to solve the task, while remaining trainable end-to-end. The approach mimics human learning and educational techniques, and has a number of significant advantages. SubUNets allow us to inject domain-specific expert knowledge into the system regarding suitable intermediate representations. They also allow us to implicitly perform transfer learning between different interrelated tasks, which also allows us to exploit a wider range of more varied data sources. In our experiments we demonstrate that each of these properties serves to significantly improve the performance of the overarching recognition system, by better constraining the learning problem. The proposed techniques are demonstrated in the challenging domain of sign language recognition. We demonstrate state-of-the-art performance on hand-shape recognition (outperforming previous techniques by more than 30%). Furthermore, we are able to obtain comparable sign recognition rates to previous research, without the need for an alignment step to segment out the signs for recognition.
We present a novel approach to automatic Sign Language Production using stateof- the-art Neural Machine Translation (NMT) and Image Generation techniques. Our system is capable of producing sign videos from spoken language sentences. Contrary to current approaches that are dependent on heavily annotated data, our approach requires minimal gloss and skeletal level annotations for training. We achieve this by breaking down the task into dedicated sub-processes. We first translate spoken language sentences into sign gloss sequences using an encoder-decoder network. We then find a data driven mapping between glosses and skeletal sequences. We use the resulting pose information to condition a generative model that produces sign language video sequences. We evaluate our approach on the recently released PHOENIX14T Sign Language Translation dataset. We set a baseline for text-to-gloss translation, reporting a BLEU-4 score of 16.34/15.26 on dev/test sets. We further demonstrate the video generation capabilities of our approach by sharing qualitative results of generated sign sequences given their skeletal correspondence.
This paper presents a new information source for supporting robot localisation: material composition. The proposed method complements the existing visual, structural, and semantic cues utilized in the literature. However, it has a distinct advantage in its ability to differentiate structurally, visually or categorically similar objects such as different doors, by using Raman spectrometers. Such devices can identify the material of objects it probes through the bonds between the material's molecules. Unlike similar sensors, such as mass spectroscopy, it does so without damaging the material or environment. In addition to introducing the first material-based localisation algorithm, this paper supports the future growth of the field by presenting a gazebo plugin for Raman spectrometers, material sensing demonstrations, as well as the first-ever localisation data-set with benchmarks for material-based localisation. This benchmarking shows that the proposed technique results in a significant improvement over current state-of-the-art localisation techniques, achieving 16\% more accurate localisation than the leading baseline.
We investigate the recognition of actions "in the wild"’ using 3D motion information. The lack of control over (and knowledge of) the camera configuration, exacerbates this already challenging task, by introducing systematic projective inconsistencies between 3D motion fields, hugely increasing intra-class variance. By introducing a robust, sequence based, stereo calibration technique, we reduce these inconsistencies from fully projective to a simple similarity transform. We then introduce motion encoding techniques which provide the necessary scale invariance, along with additional invariances to changes in camera viewpoint. On the recent Hollywood 3D natural action recognition dataset, we show improvements of 40% over previous state-of-the-art techniques based on implicit motion encoding. We also demonstrate that our robust sequence calibration simplifies the task of recognising actions, leading to recognition rates 2.5 times those for the same technique without calibration. In addition, the sequence calibrations are made available.
The Internet of Things (IoT) poses important challenges requiring multidisciplinary solutions that take into account the potential mutual effects and interactions among the different dimensions of future IoT systems. A suitable platform is required for an accurate and realistic evaluation of such solutions. This paper presents a prototype developed in the context of the EPSRC/eFutures-funded project “Internet of Surprise: Self-Organising Data”. The prototype has been designed to effectively enable the joint evaluation and optimisation of multidisciplinary aspects of IoT systems, including aspects related with hardware design, communications and data processing. This paper provides a comprehensive description, discussing design and implementation details that may be helpful to other researchers and engineers in the development of similar tools. Examples illustrating the potentials and capabilities are presented as well. The developed prototype is a versatile tool that can be used for proof-of-concept, validation and cross-layer optimisation of multidisciplinary solutions for future IoT deployments.
The use of reinforcement learning (RL) has led to huge advancements in the field of robotics. However data scarcity, brittle convergence and the gap between simulation & real world environments, mean that most common RL approaches are subject to over fitting and fail to generalise to unseen environments. Hardware agnostic policies would mitigate this by allowing a single network to operate in a variety of test domains, where dynamics vary due to changes in robotic morphologies or internal parameters. We utilise the idea that learning to adapt a known and successful control policy is easier and more flexible than jointly learning numerous control policies for different morphologies. This paper presents the idea of Hardware Agnostic Reinforcement Learning using Adversarial selection (HARL-A). In this approach training examples are sampled using a novel adversarial loss function. This is designed to self regulate morphologies based on their learning potential. Simply applying our learning potential based loss function to current state-of- the-art already provides ~ 30% improvement in performance. Meanwhile experiments using the full implementation of HARL-A report an average increase of 70% to a standard RL baseline and 55% compared with current state-of-the-art.
In this paper, we propose using 3D Convolutional Neural Networks for large scale user-independent continuous gesture recognition. We have trained an end-to-end deep network for continuous gesture recognition (jointly learning both the feature representation and the classifier). The network performs three-dimensional (i.e. space-time) convolutions to extract features related to both the appearance and motion from volumes of color frames. Space-time invariance of the extracted features is encoded via pooling layers. The earlier stages of the network are partially initialized using the work of Tran et al. before being adapted to the task of gesture recognition. An earlier version of the proposed method, which was trained for 11,250 iterations, was submitted to ChaLearn 2016 Continuous Gesture Recognition Challenge and ranked 2nd with the Mean Jaccard Index Score of 0.269235. When the proposed method was further trained for 28,750 iterations, it achieved state-of-the-art performance on the same dataset, yielding a 0.314779 Mean Jaccard Index Score.
This paper explores the potential of event cameras to enable continuous time Reinforcement Learning. We formalise this problem where a continuous stream of unsynchronised observations is used to produce a corresponding stream of output actions for the environment. This lack of synchronisation enables greatly enhanced reactivity. We present a method to train on event streams derived from standard RL environments, thereby solving the proposed continuous time RL problem. The CERiL algorithm uses specialised network layers which operate directly on an event stream, rather than aggregating events into quantised image frames. We show the advantages of event streams over less-frequent RGB images. The proposed system outperforms networks typically used in RL, even succeeding at tasks which cannot be solved traditionally. We also demonstrate the value of our CERiL approach over a standard SNN baseline using event streams. Code is available at https: //gitlab.surrey.ac.uk/cw0071/ceril.
The relationship between the feedback given in Reinforcement Learning (RL) and visual data input is often extremely complex. Given this, expecting a single system trained end-to-end to learn both how to perceive and interact with its environment is unrealistic for complex domains. In this paper we propose Auto-Perceptive Reinforcement Learning (APRiL), separating the perception and the control elements of the task. This method uses an auto-perceptive network to encode a feature space. The feature space may explicitly encode available knowledge from the semantically understood state space but the network is also free to encode unanticipated auxiliary data. By decoupling visual perception from the RL process, APRiL can make use of techniques shown to improve performance and efficiency of RL training, which are often difficult to apply directly with a visual input. We present results showing that APRiL is effective in tasks where the semantically understood state space is known. We also demonstrate that allowing the feature space to learn auxiliary information, allows it to use the visual perception system to improve performance by approximately 30%. We also show that maintaining some level of semantics in the encoded state, which can then make use of state-of-the art RL techniques, saves around 75% of the time that would be used to collect simulation examples.
We propose a novel deep learning approach to solve simultaneous alignment and recognition problems (referred to as "Sequence-to-sequence" learning). We decompose the problem into a series of specialised expert systems referred to as SubUNets. The spatio-temporal relationships between these SubUNets are then modelled to solve the task, while remaining trainable end-to-end. The approach mimics human learning and educational techniques, and has a number of significant advantages. SubUNets allow us to inject domain-specific expert knowledge into the system regarding suitable intermediate representations. They also allow us to implicitly perform transfer learning between different interrelated tasks, which also allows us to exploit a wider range of more varied data sources. In our experiments we demonstrate that each of these properties serves to significantly improve the performance of the overarching recognition system, by better constraining the learning problem. The proposed techniques are demonstrated in the challenging domain of sign language recognition. We demonstrate state-of-the-art performance on hand-shape recognition (outperforming previous techniques by more than 30%). Furthermore, we are able to obtain comparable sign recognition rates to previous research, without the need for an alignment step to segment out the signs for recognition.
We present a novel form of explanation for Reinforcement Learning, based around the notion of intended outcome. These explanations describe the outcome an agent is trying to achieve by its actions. We provide a simple proof that general methods for post-hoc explanations of this nature are impossible in traditional reinforcement learning. Rather, the information needed for the explanations must be collected in conjunction with training the agent. We derive approaches designed to extract local explanations based on intention for several variants of Q-function approximation and prove consistency between the explanations and the Q-values learned. We demonstrate our method on multiple reinforcement learning problems, and provide code 1 to help researchers introspecting their RL environments and algorithms.
Advancements in onboard data processing capabilities of small EO satellites represent an avenue for mission integrators, satellite customers and end-users alike to maximise the return on investment of space-borne remote sensing platforms. Surrey Satellite Technology Limited's (SSTL's) Flexible and Intelligent Payload Chain (FIPC) subsystem is an integrated solution which aims to address the data bottleneck challenges of small EO satellites, leveraging capabilities which include onboard data processing. This publication describes SSTL's recent coupled developments in the FIPC space segment, towards a tightly integrated hardware architecture; a new Linux-based custom onboard processing environment; and an end-user segment with a tailored Application Development Framework. Together these facilitate the deployment of in-house and third-party developed software onboard processing Applications and pipelines, including those which exploit machine learning (ML) libraries and frameworks.
Despite the success of neural networks in computer vision tasks, digital 'neurons' are a very loose approximation of biological neurons. Today's learning approaches are designed to function on digital devices with digital data representations such as image frames. In contrast, biological vision systems are generally much more capable and efficient than state-of-the-art digital computer vision algorithms. Event cameras are an emerging sensor technology which imitates biological vision with asynchronously firing pixels, eschewing the concept of the image frame. To leverage modern learning techniques, many event-based algorithms are forced to accumulate events back to image frames, somewhat squandering the advantages of event cameras. We follow the opposite paradigm and develop a new type of neural network which operates closer to the original event data stream. We demonstrate state-of-the-art performance in angular velocity regression and competitive optical flow estimation, while avoiding difficulties related to training SNN. Furthermore, the processing latency of our proposed approach is less than 1/10 any other implementation, while continuous inference increases this improvement by another order of magnitude.
We present a novel approach to 3D reconstruction which is inspired by the human visual system. This system unifies standard appearance matching and triangulation techniques with higher level reasoning and scene understanding, in order to resolve ambiguities between different interpretations of the scene. The types of reasoning integrated in the approach includes recognising common configurations of surface normals and semantic edges (e.g. convex, concave and occlusion boundaries). We also recognise the coplanar, collinear and symmetric structures which are especially common in man made environments.
Tracking hands and estimating their trajectories is useful in a number of tasks, including sign language recognition and human computer interaction. Hands are extremely difficult objects to track, their deformability, frequent self occlusions and motion blur cause appearance variations too great for most standard object trackers to deal with robustly. In this paper, the 3D motion field of a scene (known as the Scene Flow, in contrast to Optical Flow, which is it’s projection onto the image plane) is estimated using a recently proposed algorithm, inspired by particle filtering. Unlike previous techniques, this scene flow algorithm does not introduce blurring across discontinuities, making it far more suitable for object segmentation and tracking. Additionally the algorithm operates several orders of magnitude faster than previous scene flow estimation systems, enabling the use of Scene Flow in real-time, and near real-time applications. A novel approach to trajectory estimation is then introduced, based on clustering the estimated scene flow field in both space and velocity dimensions. This allows estimation of object motions in the true 3D scene, rather than the traditional approach of estimating 2D image plane motions. By working in the scene space rather than the image plane, the constant velocity assumption, commonly used in the prediction stage of trackers, is far more valid, and the resulting motion estimate is richer, providing information on out of plane motions. To evaluate the performance of the system, 3D trajectories are estimated on a multi-view sign-language dataset, and compared to a traditional high accuracy 2D system, with excellent results.
Causal relationships can often be found in visual object tracking between the motions of the camera and that of the tracked object. This object motion may be an effect of the camera motion, e.g. an unsteady handheld camera. But it may also be the cause, e.g. the cameraman framing the object. In this paper we explore these relationships, and provide statistical tools to detect and quantify them, these are based on transfer entropy and stem from information theory. The relationships are then exploited to make predictions about the object location. The approach is shown to be an excellent measure for describing such relationships. On the VOT2013 dataset the prediction accuracy is increased by 62 % over the best non-causal predictor. We show that the location predictions are robust to camera shake and sudden motion, which is invaluable for any tracking algorithm and demonstrate this by applying causal prediction to two state-of-the-art trackers. Both of them benefit, Struck gaining a 7 % accuracy and 22 % robustness increase on the VTB1.1 benchmark, becoming the new state-of-the-art.
How does a person work out their location using a floorplan? It is probably safe to say that we do not explicitly measure depths to every visible surface and try to match them against different pose estimates in the floorplan. And yet, this is exactly how most robotic scan-matching algorithms operate. Similarly, we do not extrude the 2D geometry present in the floorplan into 3D and try to align it to the real-world. And yet, this is how most vision-based approaches localise. Humans do the exact opposite. Instead of depth, we use high level semantic cues. Instead of extruding the floorplan up into the third dimension, we collapse the 3D world into a 2D representation. Evidence of this is that many of the floorplans we use in everyday life are not accurate, opting instead for high levels of discriminative landmarks. In this work, we use this insight to present a global localisation approach that relies solely on the semantic labels present in the floorplan and extracted from RGB images. While our approach is able to use range measurements if available, we demonstrate that they are unnecessary as we can achieve results comparable to state-of-the-art without them.
The Visual Object Tracking challenge 2014, VOT2014, aims at comparing short-term single-object visual trackers that do not apply pre-learned models of object appearance. Results of 38 trackers are presented. The number of tested trackers makes VOT 2014 the largest benchmark on short-term tracking to date. For each participating tracker, a short description is provided in the appendix. Features of the VOT2014 challenge that go beyond its VOT2013 predecessor are introduced: (i) a new VOT2014 dataset with full annotation of targets by rotated bounding boxes and per-frame attribute, (ii) extensions of the VOT2013 evaluation methodology, (iii) a new unit for tracking speed assessment less dependent on the hardware and (iv) the VOT2014 evaluation toolkit that significantly speeds up execution of experiments. The dataset, the evaluation kit as well as the results are publicly available at the challenge website (http://votchallenge.net).
The development of industrial automation is closely related to the evolution of mobile robot positioning and navigation mode. In this paper, we introduce ASL-SLAM, the first line-based SLAM system operating directly on robots using the event sensor only. This approach maximizes the advantages of the event information generated by a bio-inspired sensor. We estimate the local Surface of Active Events (SAE) to get the planes for each incoming event in the event stream. Then the edges and their motion are recovered by our line extraction algorithm. We show how the inclusion of event-based line tracking significantly improves performance compared to state-of-the-art frame-based SLAM systems. The approach is evaluated on publicly available datasets. The results show that our approach is particularly effective with poorly textured frames when the robot faces simple or low texture environments. We also experimented with challenging illumination situations to order to be suitable for various industrial environments, including low-light and high motion blur scenarios. We show that our approach with the event-based camera has natural advantages and provides up to 85% reduction in error when performing SLAM under these conditions compared to the traditional approach.
In this work we describe a novel approach to online dense non-rigid structure from motion. The problem is reformulated, incorporating ideas from visual object tracking, to provide a more general and unified technique, with feedback between the reconstruction and point-tracking algorithms. The resulting algorithm overcomes the limitations of many conventional techniques, such as the need for a reference image/template or precomputed trajectories. The technique can also be applied in traditionally challenging scenarios, such as modelling objects with strong self-occlusions or from an extreme range of viewpoints. The proposed algorithm needs no offline pre-learning and does not assume the modelled object stays rigid at the beginning of the video sequence. Our experiments show that in traditional scenarios, the proposed method can achieve better accuracy than the current state of the art while using less supervision. Additionally we perform reconstructions in challenging new scenarios where state-of-the-art approaches break down and where our method improves performance by up to an order of magnitude.
Action recognition in unconstrained situations is a difficult task, suffering from massive intra-class variations. It is made even more challenging when complex 3D actions are projected down to the image plane, losing a great deal of information. The recent emergence of 3D data, both in broadcast content, and commercial depth sensors, provides the possibility to overcome this issue. This paper presents a new dataset, for benchmarking action recognition algorithms in natural environments, while making use of 3D information. The dataset contains around 650 video clips, across 14 classes. In addition, two state of the art action recognition algorithms are extended to make use of the 3D data, and five new interest point detection strategies are also proposed, that extend to the 3D data. Our evaluation compares all 4 feature descriptors, using 7 different types of interest point, over a variety of threshold levels, for the Hollywood 3D dataset. We make the dataset including stereo video, estimated depth maps and all code required to reproduce the benchmark results, available to the wider community.
In this paper, we propose using 3D Convolutional Neural Networks for large scale user-independent continuous gesture recognition. We have trained an end-to-end deep network for continuous gesture recognition (jointly learning both the feature representation and the classifier). The network performs three-dimensional (i.e. space-time) convolutions to extract features related to both the appearance and motion from volumes of color frames. Space-time invariance of the extracted features is encoded via pooling layers. The earlier stages of the network are partially initialized using the work of Tran et al. before being adapted to the task of gesture recognition. An earlier version of the proposed method, which was trained for 11,250 iterations, was submitted to ChaLearn 2016 Continuous Gesture Recognition Challenge and ranked 2nd with the Mean Jaccard Index Score of 0.269235. When the proposed method was further trained for 28,750 iterations, it achieved state-of-the-art performance on the same dataset, yielding a 0.314779 Mean Jaccard Index Score.
In this paper we present S3R-Net, the Self-Supervised Shadow Removal Network. The two-branch WGAN model achieves self-supervision relying on the unify-and-adaptphenomenon - it unifies the style of the output data and infers its characteristics from a database of unaligned shadow-free reference images. This approach stands in contrast to the large body of supervised frameworks. S3R-Net also differentiates itself from the few existing self-supervised models operating in a cycle-consistent manner, as it is a non-cyclic, unidirectional solution. The proposed framework achieves comparable numerical scores to recent selfsupervised shadow removal models while exhibiting superior qualitative performance and keeping the computational cost low. Code & pretrained models are available at https://github.com/n-kubiak/S3R-Net
This paper presents a new information source for supporting robot localisation: material composition. The proposed method complements the existing visual, structural, and semantic cues utilized in the literature. However, it has a distinct advantage in its ability to differentiate structurally [23], visually [25] or categorically [1] similar objects such as different doors, by using Raman spectrometers. Such devices can identify the material of objects it probes through the bonds between the material’s molecules. Unlike similar sensors, such as mass spectroscopy, it does so without damaging the material or environment. In addition to introducing the first material-based localisation algorithm, this paper supports the future growth of the field by presenting a gazebo plugin for Raman spectrometers, material sensing demonstrations, as well as the first-ever localisation data-set with benchmarks for material-based localisation. This benchmarking shows that the proposed technique results in a significant improvement over current state-of-the-art localisation techniques, achieving 16% more accurate localisation than the leading baseline. The code and dataset will be released at: https://github.com/ThirgoodC/RaSpectLoc
We present SILT, a Self-supervised Implicit Lighting Transfer method. Unlike previous research on scene relighting, we do not seek to apply arbitrary new lighting configurations to a given scene. Instead, we wish to transfer the lighting style from a database of other scenes, to provide a uniform lighting style regardless of the input. The solution operates as a two-branch network that first aims to map input images of any arbitrary lighting style to a unified domain, with extra guidance achieved through implicit image decomposition. We then remap this unified input domain using a discriminator that is presented with the generated outputs and the style reference, i.e. images of the desired illumination conditions. Our method is shown to outperform supervised relighting solutions across two different datasets without requiring lighting supervision.
This paper discusses the results for the second edition of the Monocular Depth Estimation Challenge (MDEC). This edition was open to methods using any form of supervision, including fully-supervised, self-supervised, multi-task or proxy depth. The challenge was based around the SYNS-Patches dataset, which features a wide diversity of environments with high-quality dense ground-truth. This includes complex natural environments, e.g. forests or fields, which are greatly underrepresented in current benchmarks. The challenge received eight unique submissions that outperformed the provided SotA baseline on any of the pointcloud- or image-based metrics. The top supervised submission improved relative F-Score by 27.62%, while the top self-supervised improved it by 16.61%. Supervised submissions generally leveraged large collections of datasets to improve data diversity. Self-supervised submissions instead updated the network architecture and pretrained backbones. These results represent a significant progress in the field, while highlighting avenues for future research, such as reducing interpolation artifacts at depth boundaries, improving self-supervised indoor performance and overall natural image accuracy.
Existing shadow detection models struggle to differentiate dark image areas from shadows. In this paper, we tackle this issue by verifying that all detected shadows are real, i.e. they have paired shadow casters. We perform this step in a physically-accurate manner by dif-ferentiably re-rendering the scene and observing the changes stemming from carving out estimated shadow casters. Thanks to this approach, the RenDetNet proposed in this paper is the first learning-based shadow detection model whose supervisory signals can be computed in a self-supervised manner. The developed system compares favourably against recent models trained on our data. As part of this publication, we release our code on github.
This paper summarizes the results of the first Monocular Depth Estimation Challenge (MDEC) organized at WACV2023. This challenge evaluated the progress of selfsupervised monocular depth estimation on the challenging SYNS-Patches dataset. The challenge was organized on CodaLab and received submissions from 4 valid teams. Participants were provided a devkit containing updated reference implementations for 16 State-of-the-Art algorithms and 4 novel techniques. The threshold for acceptance for novel techniques was to outperform every one of the 16 SotA baselines. All participants outperformed the baseline in traditional metrics such as MAE or AbsRel. However, pointcloud reconstruction metrics were challenging to improve upon.We found predictions were characterized by interpolation artefacts at object boundaries and errors in relative object positioning. We hope this challenge is a valuable contribution to the community and encourage authors to participate in future editions
In this paper, we propose a novel particle filter based probabilistic forced alignment approach for training spatio-temporal deep neural networks using weak border level annotations. The proposed method jointly learns to localize and recognize isolated instances in continuous streams. This is done by drawing training volumes from a prior distribution of likely regions and training a discriminative 3D-CNN from this data. The classifier is then used to calculate the posterior distribution by scoring the training examples and using this as the prior for the next sampling stage. We apply the proposed approach to the challenging task of large-scale user-independent continuous gesture recognition. We evaluate the performance on the popular ChaLearn 2016 Continuous Gesture Recognition (ConGD) dataset. Our method surpasses state-of-the-art results by obtaining 0.3646 and 0.3744 Mean Jaccard Index Score on the validation and test sets of ConGD, respectively. Furthermore, we participated in the ChaLearn 2017 Continuous Gesture Recognition Challenge and was ranked 3rd. It should be noted that our method is learner independent, it can be easily combined with other approaches.
How do computers and intelligent agents view the world around them? Feature extraction and representation constitutes one the basic building blocks towards answering this question. Traditionally, this has been done with carefully engineered hand-crafted techniques such as HOG, SIFT or ORB. However, there is no “one size fits all” approach that satisfies all requirements. In recent years, the rising popularity of deep learning has resulted in a myriad of end-to-end solutions to many computer vision problems. These approaches, while successful, tend to lack scalability and can’t easily exploit information learned by other systems. Instead, we propose SAND features, a dedicated deep learning solution to feature extraction capable of providing hierarchical context information. This is achieved by employing sparse relative labels indicating relationships of similarity/dissimilarity between image locations. The nature of these labels results in an almost infinite set of dissimilar examples to choose from. We demonstrate how the selection of negative examples during training can be used to modify the feature space and vary it’s properties. To demonstrate the generality of this approach, we apply the proposed features to a multitude of tasks, each requiring different properties. This includes disparity estimation, semantic segmentation, self-localisation and SLAM. In all cases, we show how incorporating SAND features results in better or comparable results to the baseline, whilst requiring little to no additional training. Code can be found at: https://github.com/jspenmar/SAND_features
How many times does a human have to drive through the same area to become familiar with it? To begin with, we might first build a mental model of our surroundings. Upon revisiting this area, we can use this model to extrapolate to new unseen locations and imagine their appearance. Based on this, we propose an approach where an agent is capable of modelling new environments after a single visitation. To this end, we introduce "Deep Imagination", a combination of classical Visual-based Monte Carlo Localisation and deep learning. By making use of a feature embedded 3D map, the system can "imagine" the view from any novel location. These "imagined" views are contrasted with the current observation in order to estimate the agent's current location. In order to build the embedded map, we train a deep Siamese Fully Convolutional U-Net to perform dense feature extraction. By training these features to be generic, no additional training or fine tuning is required to adapt to new environments. Our results demonstrate the generality and transfer capability of our learnt dense features by training and evaluating on multiple datasets. Additionally, we include several visualizations of the feature representations and resulting 3D maps, as well as their application to localisation.
This paper presents a new information source for supporting robot localisation: material composition. The proposed method complements the existing visual, structural, and semantic cues utilized in the literature. However, it has a distinct advantage in its ability to differentiate structurally [23], visually [25] or categorically [1] similar objects such as different doors, by using Raman spectrometers. Such devices can identify the material of objects it probes through the bonds between the material's molecules. Unlike similar sensors, such as mass spectroscopy, it does so without damaging the material or environment. In addition to introducing the first material-based localisation algorithm, this paper supports the future growth of the field by presenting a gazebo plugin for Raman spectrometers, material sensing demonstrations, as well as the first-ever localisation data-set with benchmarks for material-based localisation. This benchmarking shows that the proposed technique results in a significant improvement over current state-of-the-art localisation techniques, achieving 16 % more accurate localisation than the leading baseline. The code and dataset will be released at: https://github.com/ThirgoodC/RaSpectLoc
Grasping is one of the oldest problems in robotics and is still considered challenging, especially when grasping unknown objects with unknown 3D shape. We focus on exploiting recent advances in computer vision recognition systems. Object classification problems tend to have much larger datasets to train from and have far fewer practical constraints around the size of the model and speed to train. In this paper we will investigate how to adapt Convolutional Neural Networks (CNNs), traditionally used for image classification, for planar robotic grasping. We consider the differences in the problems and how a network can be adjusted to account for this. Positional information is far more important to robotics than generic image classification tasks, where max pooling layers are used to improve translation invariance. By using a more appropriate network structure we are able to obtain improved accuracy while simultaneously improving run times and reducing memory consumption by reducing model size by up to 69%.
The use of human-level semantic information to aid robotic tasks has recently become an important area for both Computer Vision and Robotics. This has been enabled by advances in Deep Learning that allow consistent and robust semantic understanding. Leveraging this semantic vision of the world has allowed human-level understanding to naturally emerge from many different approaches. Particularly, the use of semantic information to aid in localisation and reconstruction has been at the forefront of both fields. Like robots, humans also require the ability to localise within a structure. To aid this, humans have designed highlevel semantic maps of our structures called floorplans. We are extremely good at localising in them, even with limited access to the depth information used by robots. This is because we focus on the distribution of semantic elements, rather than geometric ones. Evidence of this is that humans are normally able to localise in a floorplan that has not been scaled properly. In order to grant this ability to robots, it is necessary to use localisation approaches that leverage the same semantic information humans use. In this paper, we present a novel method for semantically enabled global localisation. Our approach relies on the semantic labels present in the floorplan. Deep Learning is leveraged to extract semantic labels from RGB images, which are compared to the floorplan for localisation. While our approach is able to use range measurements if available, we demonstrate that they are unnecessary as we can achieve results comparable to state-of-the-art without them.
This paper demonstrates a novel method for automatically discovering and recognising characters in video without any labelled examples or user intervention. Instead weak supervision is obtained via a rough script-to-subtitle alignment. The technique uses pose invariant features, extracted from detected faces and clustered to form groups of co-occurring characters. Results show that with 9 characters, 29% of the closest exemplars are correctly identified, increasing to 50% as additional exemplars are considered.
Sign languages use multiple asynchronous information channels (articulators), not just the hands but also the face and body, which computational approaches often ignore. In this paper we tackle the multiarticulatory sign language translation task and propose a novel multichannel transformer architecture. The proposed architecture allows both the inter and intra contextual relationships between different sign articulators to be modelled within the transformer network itself, while also maintaining channel specific information. We evaluate our approach on the RWTH-PHOENIX-Weather-2014T dataset and report competitive translation performance. Importantly, we overcome the reliance on gloss annotations which underpin other state-of-the-art approaches, thereby removing the need for expensive curated datasets.
Sign Language Recognition (SLR) has been an active research field for the last two decades. However, most research to date has considered SLR as a naive gesture recognition problem. SLR seeks to recognize a sequence of continuous signs but neglects the underlying rich grammatical and linguistic structures of sign language that differ from spoken language. In contrast, we introduce the Sign Language Translation (SLT) problem. Here, the objective is to generate spoken language translations from sign language videos, taking into account the different word orders and grammar. We formalize SLT in the framework of Neural Machine Translation (NMT) for both end-to-end and pretrained settings (using expert knowledge). This allows us to jointly learn the spatial representations, the underlying language model, and the mapping between sign and spoken language. To evaluate the performance of Neural SLT, we collected the first publicly available Continuous SLT dataset, RWTHPHOENIX-Weather 2014T1. It provides spoken language translations and gloss level annotations for German Sign Language videos of weather broadcasts. Our dataset contains over .95M frames with >67K signs from a sign vocabulary of >1K and >99K words from a German vocabulary of >2.8K. We report quantitative and qualitative results for various SLT setups to underpin future research in this newly established field. The upper bound for translation performance is calculated at 19.26 BLEU-4, while our end-to-end frame-level and gloss-level tokenization networks were able to achieve 9.58 and 18.13 respectively.
The Thermal Infrared Visual Object Tracking challenge 2016, VOT-TIR2016, aims at comparing short-term single-object visual trackers that work on thermal infrared (TIR) sequences and do not apply pre-learned models of object appearance. VOT-TIR2016 is the second benchmark on short-term tracking in TIR sequences. Results of 24 trackers are presented. For each participating tracker, a short description is provided in the appendix. The VOT-TIR2016 challenge is similar to the 2015 challenge, the main difference is the introduction of new, more difficult sequences into the dataset. Furthermore, VOT-TIR2016 evaluation adopted the improvements regarding overlap calculation in VOT2016. Compared to VOT-TIR2015, a significant general improvement of results has been observed, which partly compensate for the more difficult sequences. The dataset, the evaluation kit, as well as the results are publicly available at the challenge website.
The Visual Object Tracking challenge VOT2017 is the fifth annual tracker benchmarking activity organized by the VOT initiative. Results of 51 trackers are presented; many are state-of-the-art published at major computer vision conferences or journals in recent years. The evaluation included the standard VOT and other popular methodologies and a new "real-time" experiment simulating a situation where a tracker processes images as if provided by a continuously running sensor. Performance of the tested trackers typically by far exceeds standard baselines. The source code for most of the trackers is publicly available from the VOT page. The VOT2017 goes beyond its predecessors by (i) improving the VOT public dataset and introducing a separate VOT2017 sequestered dataset, (ii) introducing a realtime tracking experiment and (iii) releasing a redesigned toolkit that supports complex experiments. The dataset, the evaluation kit and the results are publicly available at the challenge website(1).
Although 3D reconstruction from a monocular video has been an active area of research for a long time, and the resulting models offer great realism and accuracy, strong conditions must be typically met when capturing the video to make this possible. This prevents general reconstruction of moving objects in dynamic, uncontrolled scenes. In this paper, we address this issue. We present a novel algorithm for modelling 3D shapes from unstructured, unconstrained discontinuous footage. The technique is robust against distractors in the scene, background clutter and even shot cuts. We show reconstructed models of objects, which could not be modelled by conventional Structure from Motion methods without additional input. Finally, we present results of our reconstruction in the presence of shot cuts, showing the strength of our technique at modelling from existing footage.
Despite the success of neural networks in computer vision tasks, digital 'neurons' are a very loose approximation of biological neurons. Today's learning approaches are designed to function on digital devices with digital data representations such as image frames. In contrast, biological vision systems are generally much more capable and efficient than state-of-the-art digital computer vision algorithms. Event cameras are an emerging sensor technology which imitates biological vision with asynchronously firing pixels, eschewing the concept of the image frame. To leverage modern learning techniques, many event-based algorithms are forced to accumulate events back to image frames, somewhat squandering the advantages of event cameras. We follow the opposite paradigm and develop a new type of neural network which operates closer to the original event data stream. We demonstrate state-of-the-art performance in angular velocity regression and competitive optical flow estimation, while avoiding difficulties related to training Spiking Neural Networks. Furthermore, the processing latency of our proposed approach is less than 1/10 any other implementation, while continuous inference increases this improvement by another order of magnitude. Code is available at https://gitlab.surrey.ac.uk/cw0071/edenn.
In this work, we present an end-to-end network for stereo-consistent image inpainting with the objective of inpainting large missing regions behind objects. The proposed model consists of an edge-guided UNet-like network using Partial Convolutions. We enforce multi-view stereo consistency by introducing a disparity loss. More importantly, we develop a training scheme where the model is learned from realistic stereo masks representing object occlusions, instead of the more common random masks. The technique is trained in a supervised way. Our evaluation shows competitive results compared to previous state-of-the-art techniques.
The Visual Object Tracking challenge 2014, VOT2014, aims at comparing short-term single-object visual trackers that do not apply pre-learned models of object appearance. Results of 38 trackers are presented. The number of tested trackers makes VOT 2014 the largest benchmark on short-term tracking to date. For each participating tracker, a short description is provided in the appendix. Features of the VOT2014 challenge that go beyond its VOT2013 predecessor are introduced: (i) a new VOT2014 dataset with full annotation of targets by rotated bounding boxes and per-frame attribute, (ii) extensions of the VOT2013 evaluation methodology, (iii) a new unit for tracking speed assessment less dependent on the hardware and (iv) the VOT2014 evaluation toolkit that significantly speeds up execution of experiments. The dataset, the evaluation kit as well as the results are publicly available at the challenge website.
In the context of self-driving vehicles there is strong competition between approaches based on visual localisa-tion and Light Detection And Ranging (LiDAR). While LiDAR provides important depth information, it is sparse in resolution and expensive. On the other hand, cameras are low-cost and recent developments in deep learning mean they can provide high localisation performance. However, several fundamental problems remain, particularly in the domain of uncertainty, where learning based approaches can be notoriously over-confident. Markov, or grid-based, localisation was an early solution to the localisation problem but fell out of favour due to its computational complexity. Representing the likelihood field as a grid (or volume) means there is a trade off between accuracy and memory size. Furthermore, it is necessary to perform expensive convolutions across the entire likelihood volume. Despite the benefit of simultaneously maintaining a likelihood for all possible locations, grid based approaches were superseded by more efficient particle filters and Monte Carlo sampling (MCL). However, MCL introduces its own problems e.g. particle deprivation. Recent advances in deep learning hardware allow large likelihood volumes to be stored directly on the GPU, along with the hardware necessary to efficiently perform GPU-bound 3D convolutions and this obviates many of the disadvantages of grid based methods. In this work, we present a novel CNN-based localisation approach that can leverage modern deep learning hardware. By implementing a grid-based Markov localisation approach directly on the GPU, we create a hybrid Convolutional Neural Network (CNN) that can perform image-based localisation and odometry-based likelihood propagation within a single neural network. The resulting approach is capable of outperforming direct pose regression methods as well as state-of-the-art localisation systems.
Most 3D reconstruction approaches passively optimise over all data, exhaustively matching pairs, rather than actively selecting data to process. This is costly both in terms of time and computer resources, and quickly becomes intractable for large datasets. This work proposes an approach to intelligently filter large amounts of data for 3D reconstructions of unknown scenes using monocular cameras. Our contributions are twofold: First, we present a novel approach to efficiently optimise the Next-Best View ( NBV ) in terms of accuracy and coverage using partial scene geometry. Second, we extend this to intelligently selecting stereo pairs by jointly optimising the baseline and vergence to find the NBV ’s best stereo pair to perform reconstruction. Both contributions are extremely efficient, taking 0.8ms and 0.3ms per pose, respectively. Experimental evaluation shows that the proposed method allows efficient selection of stereo pairs for reconstruction, such that a dense model can be obtained with only a small number of images. Once a complete model has been obtained, the remaining computational budget is used to intelligently refine areas of uncertainty, achieving results comparable to state-of-the-art batch approaches on the Middlebury dataset, using as little as 3.8% of the views.
We present a novel approach to 3D reconstruction which is inspired by the human visual system. This system unifies standard appearance matching and triangulation techniques with higher level reasoning and scene understanding, in order to resolve ambiguities between different interpretations of the scene. The types of reasoning integrated in the approach includes recognising common configurations of surface normals and semantic edges (e.g. convex, concave and occlusion boundaries). We also recognise the coplanar, collinear and symmetric structures which are especially common in man made environments.
How many times does a human have to drive through the same area to become familiar with it? To begin with, we might first build a mental model of our surroundings. Upon revisiting this area, we can use this model to extrapolate to new unseen locations and imagine their appearance. Based on this, we propose an approach where an agent is capable of modelling new environments after a single visitation. To this end, we introduce “Deep Imagination”, a combination of classical Visual-based Monte Carlo Localisation and deep learning. By making use of a feature embedded 3D map, the system can “imagine” the view from any novel location. These “imagined” views are contrasted with the current observation in order to estimate the agent’s current location. In order to build the embedded map, we train a deep Siamese Fully Convolutional U-Net to perform dense feature extraction. By training these features to be generic, no additional training or fine tuning is required to adapt to new environments. Our results demonstrate the generality and transfer capability of our learnt dense features by training and evaluating on multiple datasets. Additionally, we include several visualizations of the feature representations and resulting 3D maps, as well as their application to localisation.
Long term tracking of an object, given only a single instance in an initial frame, remains an open problem. We propose a visual tracking algorithm, robust to many of the difficulties which often occur in real-world scenes. Correspondences of edge-based features are used, to overcome the reliance on the texture of the tracked object and improve invariance to lighting. Furthermore we address long-term stability, enabling the tracker to recover from drift and to provide redetection following object disappearance or occlusion. The two-module principle is similar to the successful state-of-the-art long-term TLD tracker, however our approach extends to cases of low-textured objects. Besides reporting our results on the VOT Challenge dataset, we perform two additional experiments. Firstly, results on short-term sequences show the performance of tracking challenging objects which represent failure cases for competing state-of-the-art approaches. Secondly, long sequences are tracked, including one of almost 30000 frames which to our knowledge is the longest tracking sequence reported to date. This tests the re-detection and drift resistance properties of the tracker. All the results are comparable to the state-of-the-art on sequences with textured objects and superior on non-textured objects. The new annotated sequences are made publicly available
Action recognition “in the wild” is extremely challenging, particularly when complex 3D actions are projected down to the image plane, losing a great deal of information. The recent growth of 3D data in broadcast content and commercial depth sensors, makes it possible to overcome this. However, there is little work examining the best way to exploit this new modality. In this paper we introduce the Hollywood 3D benchmark, which is the first dataset containing “in the wild” action footage including 3D data. This dataset consists of 650 stereo video clips across 14 action classes, taken from Hollywood movies. We provide stereo calibrations and depth reconstructions for each clip. We also provide an action recognition pipeline, and propose a number of specialised depth-aware techniques including five interest point detectors and three feature descriptors. Extensive tests allow evaluation of different appearance and depth encoding schemes. Our novel techniques exploiting this depth allow us to reach performance levels more than triple those of the best baseline algorithm using only appearance information. The benchmark data, code and calibrations are all made available to the community.
Small space robots have the potential to revolutionise space exploration by facilitating the on-orbit assembly of infrastructure, in shorter time scales, at reduced costs. Their commercial appeal will be further improved if such a system is also capable of performing on-orbit servicing missions, in line with the current drive to limit space debris and prolong the lifetime of satellites already in orbit. Whilst there have been a limited number of successful demonstrations of technologies capable of these on-orbit operations, the systems remain large and bespoke. The recent surge in small satellite technologies is changing the economics of space and in the near future, downsizing a space robot might become be a viable option with a host of benets. This industry wide shift means some of the technologies for use with a downsized space robot, such as power and communication subsystems, now exist. However, there are still dynamic and control issues that need to be overcome before a downsized space robot can be capable of undertaking useful missions. This paper rst outlines these issues, before analyzing the effect of downsizing a system on its operational capability. Therefore presenting the smallest controllable system such that the benefits of a small space robot can be achieved with current technologies. The sizing of the base spacecraft and manipulator are addressed here. The design presented consists of a 3 link, 6 degrees of freedom robotic manipulator mounted on a 12U form factor satellite. The feasibility of this 12U space robot was evaluated in simulation and the in-depth results presented here support the hypothesis that a small space robot is a viable solution for in-orbit operations. Keywords: Small Satellite; Space Robot; In-orbit Assembly and Servicing; In-orbit operations; Free-Flying; Free-Floating.
Robots need to be able to work in multiple different environments. Even when performing similar tasks, different behaviour should be deployed to best fit the current environment. In this paper, We propose a new approach to navigation, where it is treated as a multi-task learning problem. This enables the robot to learn to behave differently in visual navigation tasks for different environments while also learning shared expertise across environments. We evaluated our approach in both simulated environments as well as real-world data. Our method allows our system to converge with a 26% reduction in training time, while also increasing accuracy.
The Visual Object Tracking challenge 2015, VOT2015, aims at comparing short-term single-object visual trackers that do not apply pre-learned models of object appearance. Results of 62 trackers are presented. The number of tested trackers makes VOT 2015 the largest benchmark on short-term tracking to date. For each participating tracker, a short description is provided in the appendix. Features of the VOT2015 challenge that go beyond its VOT2014 predecessor are: (i) a new VOT2015 dataset twice as large as in VOT2014 with full annotation of targets by rotated bounding boxes and per-frame attribute, (ii) extensions of the VOT2014 evaluation methodology by introduction of a new performance measure. The dataset, the evaluation kit as well as the results are publicly available at the challenge website.
In this paper, we address the problem of tracking an unknown object in 3D space. Online 2D tracking often fails for strong outof-plane rotation which results in considerable changes in appearance beyond those that can be represented by online update strategies. However, by modelling and learning the 3D structure of the object explicitly, such effects are mitigated. To address this, a novel approach is presented, combining techniques from the fields of visual tracking, structure from motion (SfM) and simultaneous localisation and mapping (SLAM). This algorithm is referred to as TMAGIC (Tracking, Modelling And Gaussianprocess Inference Combined). At every frame, point and line features are tracked in the image plane and are used, together with their 3D correspondences, to estimate the camera pose. These features are also used to model the 3D shape of the object as a Gaussian process. Tracking determines the trajectories of the object in both the image plane and 3D space, but the approach also provides the 3D object shape. The approach is validated on several video-sequences used in the tracking literature, comparing favourably to state-of-the-art trackers for simple scenes (error reduced by 22 %) with clear advantages in the case of strong out-of-plane rotation, where 2D approaches fail (error reduction of 58 %).
Accurate extrinsic sensor calibration is essential for both autonomous vehicles and robots. Traditionally this is an involved process requiring calibration targets, known fiducial markers and is generally performed in a lab. Moreover, even a small change in the sensor layout requires recalibration. With the anticipated arrival of consumer autonomous vehicles, there is demand for a system which can do this automatically, after deployment and without specialist human expertise. To solve these limitations, we propose a flexible framework which can estimate extrinsic parameters without an explicit calibration stage, even for sensors with unknown scale. Our first contribution builds upon standard hand-eye calibration by jointly recovering scale. Our second contribution is that our system is made robust to imperfect and degenerate sensor data, by collecting independent sets of poses and automatically selecting those which are most ideal. We show that our approach’s robustness is essential for the target scenario. Unlike previous approaches, ours runs in real time and constantly estimates the extrinsic transform. For both an ideal experimental setup and a real use case, comparison against these approaches shows that we outperform the state-of-the-art. Furthermore, we demonstrate that the recovered scale may be applied to the full trajectory, circumventing the need for scale estimation via sensor fusion.
We present a novel approach to automatic Sign Language Production using recent developments in Neural Machine Translation (NMT), Generative Adversarial Networks, and motion generation. Our system is capable of producing sign videos from spoken language sentences. Contrary to current approaches that are dependent on heavily annotated data, our approach requires minimal gloss and skeletal level annotations for training. We achieve this by breaking down the task into dedicated sub-processes. We first translate spoken language sentences into sign pose sequences by combining an NMT network with a Motion Graph. The resulting pose information is then used to condition a generative model that produces photo realistic sign language video sequences. This is the first approach to continuous sign video generation that does not use a classical graphical avatar. We evaluate the translation abilities of our approach on the PHOENIX14T Sign Language Translation dataset. We set a baseline for text-to-gloss translation, reporting a BLEU-4 score of 16.34/15.26 on dev/test sets. We further demonstrate the video generation capabilities of our approach for both multi-signer and high-definition settings qualitatively and quantitatively using broadcast quality assessment metrics.
The Visual Object Tracking challenge VOT2016 aims at comparing short-term single-object visual trackers that do not apply pre-learned models of object appearance. Results of 70 trackers are presented, with a large number of trackers being published at major computer vision conferences and journals in the recent years. The number of tested state-of-the-art trackers makes the VOT 2016 the largest and most challenging benchmark on short-term tracking to date. For each participating tracker, a short description is provided in the Appendix. The VOT2016 goes beyond its predecessors by (i) introducing a new semi-automatic ground truth bounding box annotation methodology and (ii) extending the evaluation system with the no-reset experiment. The dataset, the evaluation kit as well as the results are publicly available at the challenge website (http:// votchallenge. net).
This paper proposes Sparse View Synthesis. This is a view synthesis problem where the number of reference views is limited, and the baseline between target and reference view is significant.Under these conditions, current radiance field methods fail catastrophically due to inescapable artifacts such 3d floating blobs, blurring and structural duplication, whenever the number of reference views is limited, or the target view diverges significantly from the reference views. Advances in network architecture and loss regularisation are unable to satisfactorily remove these artifacts. The occlusions within the scene ensure that the true contents of these regions is simply not available to the model.In this work, we instead focus on hallucinating plausible scene contents within such regions. To this end we unify radiance field models with adversarial learning and perceptual losses. The resulting system provides up to 60% improvement in perceptual accuracy compared to current state-of-the-art radiance field models on this problem.
The broad scope of obstacle avoidance has led to many kinds of computer vision-based approaches. Despite its popularity, it is not a solved problem. Traditional computer vision techniques using cameras and depth sensors often focus on static scenes, or rely on priors about the obstacles. Recent developments in bio-inspired sensors present event cameras as a compelling choice for dynamic scenes. Although these sensors have many advantages over their frame-based counterparts, such as high dynamic range and temporal resolution, event based perception has largely remained in 2D. This often leads to solutions reliant on heuristics and specific to a particular task. We show that the fusion of events and depth overcomes the failure cases of each individual modality when performing obstacle avoidance. Our proposed approach unifies event camera and lidar streams to estimate metric Time-To-Impact (TTI) without prior knowledge of the scene geometry or obstacles. In addition, we release an extensive event-based dataset with six visual streams spanning over 700 scanned scenes.
In the field of media production, video editing techniques play a pivotal role. Recent approaches have had great success at performing novel view image synthesis of static scenes. But adding temporal information adds an extra layer of complexity. Previous models have focused on implicitly representing static and dynamic scenes using NeRF. These models achieve impressive results but are costly at training and inference time. They overfit an MLP to describe the scene implicitly as a function of position. This paper proposes ZeST-NeRF, a new approach that can produce temporal NeRFs for new scenes without retraining. We can accurately reconstruct novel views using multi-view synthesis techniques and scene flow-field estimation, trained only with unrelated scenes. We demonstrate how existing state-of-the-art approaches from a range of fields cannot adequately solve this new task and demonstrate the efficacy of our solution. The resulting network improves quantitatively by 15% and produces significantly better visual results.
The successful performance of any system is dependant on the hardware of the agent, which is typically immutable during RL training. In this work, we present ORCHID (Optimisation of Robotic Control and Hardware In Design) which allows for truly simultaneous optimisation of hardware and control parameters in an RL pipeline. We show that by forming a complex differential path through a trajectory rollout we can leverage a vast amount of information from the system that was previously lost in the 'black-box' environment. Combining this with a novel hardware-conditioned critic network minimises variance during training and ensures stable updates are made. This allows for refinements to be made to both the morphology and control parameters simultaneously. The result is an efficient and versatile approach to holistic robot design, that brings the final system nearer to true optimality. We show improvements in performance across 4 different test environments with two different control algorithms - in all experiments the maximum performance achieved with ORCHID is shown to be unattainable using only policy updates with the default design. We also show how re-designing a robot using ORCHID in simulation, transfers to a vast improvement in the performance of a real-world robot.
The development of industrial automation is closely related to the evolution of mobile robot positioning and navigation mode. In this paper, we introduce ASL-SLAM, the first line-based SLAM system operating directly on robots using the event sensor only. This approach maximizes the advantages of the event information generated by a bio-inspired sensor. We estimate the local Surface of Active Events (SAE) to get the planes for each incoming event in the event stream. Then the edges and their motion are recovered by our line extraction algorithm. We show how the inclusion of event-based line tracking significantly improves performance compared to state-of-the-art frame-based SLAM systems. The approach is evaluated on publicly available datasets. The results show that our approach is particularly effective with poorly textured frames when the robot faces simple or low texture environments. We also experimented with challenging illumination situations to order to be suitable for various industrial environments, including low-light and high motion blur scenarios. We show that our approach with the event-based camera has natural advantages and provides up to 85% reduction in error when performing SLAM under these conditions compared to the traditional approach.
How do computers and intelligent agents view the world around them? Feature extraction and representation constitutes one the basic building blocks towards answering this question. Traditionally, this has been done with carefully engineered hand-crafted techniques such as HOG, SIFT or ORB. However, there is no "one size fits all" approach that satisfies all requirements. In recent years, the rising popularity of deep learning has resulted in a myriad of end-to-end solutions to many computer vision problems. These approaches, while successful, tend to lack scalability and can't easily exploit information learned by other systems. Instead, we propose SAND features, a dedicated deep learning solution to feature extraction capable of providing hierarchical context information. This is achieved by employing sparse relative labels indicating relationships of similarity/dissimilarity between image locations. The nature of these labels results in an almost infinite set of dissimilar examples to choose from. We demonstrate how the selection of negative examples during training can be used to modify the feature space and vary it's properties. To demonstrate the generality of this approach, we apply the proposed features to a multitude of tasks, each requiring different properties. This includes disparity estimation, semantic segmentation, self-localisation and SLAM. In all cases, we show how incorporating SAND features results in better or comparable results to the baseline, whilst requiring little to no additional training. Code can be found at:https:github.com.jspenmar/SAND_features
We present a framework which allows standard stereo reconstruction to be unified with a wide range of classic top-down cues from urban scene understanding. The resulting algorithm is analogous to the human visual system where conflicting interpretations of the scene due to ambiguous data can be resolved based on a higher level understanding of urban environments. The cues which are reformulated within the framework include: recognising common arrangements of surface normals and semantic edges (e.g. concave, convex and occlusion boundaries), recognising connected or coplanar structures such as walls, and recognising collinear edges (which are common on repetitive structures such as windows). Recognition of these common configurations has only recently become feasible, thanks to the emergence of large-scale reconstruction datasets. To demonstrate the importance and generality of scene understanding during stereo-reconstruction, the proposed approach is integrated with 3 different state-of-the-art techniques for bottom-up stereo reconstruction. The use of high-level cues is shown to improve performance by up to 15 % on the Middlebury 2014 and KITTI datasets. We further evaluate the technique using the recently proposed HCI stereo metrics, finding significant improvements in the quality of depth discontinuities, planar surfaces and thin structures.
Interest is increasing in the use of neural networks and deep-learning for on-board processing tasks in the space industry [1]. However development has lagged behind terrestrial applications for several reasons: space qualified computers have significantly less processing power than their terrestrial equivalents, reliability requirements are more stringent than the majority of applications deep-learning is being used for. The long requirements, design and qualification cycles in much of the space industry slows adoption of recent developments. GPUs are the first hardware choice for implementing neural networks on terrestrial computers, however no radiation hardened equivalent parts are currently available. Field Programmable Gate Array devices are capable of efficiently implementing neural networks and radiation hardened parts are available, however the process to deploy and validate an inference network is non-trivial and robust tools that automate the process are not available. We present an open source tool chain that can automatically deploy a trained inference network from the TensorFlow framework directly to the LEON 3, and an industrial case study of the design process used to train and optimise a deep-learning model for this processor. This does not directly change the three challenges described above however it greatly accelerates prototyping and analysis of neural network solutions, allowing these options to be more easily considered than is currently possible. Future improvements to the tools are identified along with a summary of some of the obstacles to using neural networks and potential solutions to these in the future.
In this paper, we propose a novel particle filter based probabilistic forced alignment approach for training spatiotemporal deep neural networks using weak border level annotations. The proposed method jointly learns to localize and recognize isolated instances in continuous streams. This is done by drawing training volumes from a prior distribution of likely regions and training a discriminative 3D-CNN from this data. The classifier is then used to calculate the posterior distribution by scoring the training examples and using this as the prior for the next sampling stage. We apply the proposed approach to the challenging task of large-scale user-independent continuous gesture recognition. We evaluate the performance on the popular ChaLearn 2016 Continuous Gesture Recognition (ConGD) dataset. Our method surpasses state-of-the-art results by obtaining 0:3646 and 0:3744 Mean Jaccard Index Score on the validation and test sets of ConGD, respectively. Furthermore, we participated in the ChaLearn 2017 Continuous Gesture Recognition Challenge and was ranked 3rd. It should be noted that our method is learner independent, it can be easily combined with other approaches.
In this work, we introduce a new perspective for learning transferable content in multi-task imitation learning. Humans are able to transfer skills and knowledge. If we can cycle to work and drive to the store, we can also cycle to the store and drive to work. We take inspiration from this and hypothesize the latent memory of a policy network can be disentangled into two partitions. These contain either the knowledge of the environmental context for the task or the generalizable skill needed to solve the task. This allows improved training efficiency and better generalization over previously unseen combinations of skills in the same environment, and the same task in unseen environments. We used the proposed approach to train a disentangled agent for two different multi-task IL environments. In both cases we out-performed the SOTA by 30% in task success rate. We also demonstrated this for navigation on a real robot.
We present SignSynth, a fully automatic and holistic approach to generating sign language video. Traditionally, Sign Language Production (SLP) relies on animating 3D avatars using expensively annotated data, but so far this approach has not been able to simultaneously provide a realistic, and scalable solution. We introduce a gloss2pose network architecture that is capable of generating human pose sequences conditioned on glosses.1 Combined with a generative adversarial pose2video network, we are able to produce natural-looking, high definition sign language video. For sign pose sequence generation, we outperform the SotA by a factor of 18, with a Mean Square Error of 1.0673 in pixels. For video generation we report superior results on three broadcast quality assessment metrics. To evaluate our full gloss-to-video pipeline we introduce two novel error metrics, to assess the perceptual quality and sign representativeness of generated videos. We present promising results, significantly outperforming the SotA in both metrics. Finally we evaluate our approach qualitatively by analysing example sequences.
Tracking hands and estimating their trajectories is useful in a number of tasks, including sign language recognition and human computer interaction. Hands are extremely difficult objects to track, their deformability, frequent self occlusions and motion blur cause appearance variations too great for most standard object trackers to deal with robustly. In this paper, the 3D motion field of a scene (known as the Scene Flow, in contrast to Optical Flow, which is it's projection onto the image plane) is estimated using a recently proposed algorithm, inspired by particle filtering. Unlike previous techniques, this scene flow algorithm does not introduce blurring across discontinuities, making it far more suitable for object segmentation and tracking. Additionally the algorithm operates several orders of magnitude faster than previous scene flow estimation systems, enabling the use of Scene Flow in real-time, and near real-time applications. A novel approach to trajectory estimation is then introduced, based on clustering the estimated scene flow field in both space and velocity dimensions. This allows estimation of object motions in the true 3D scene, rather than the traditional approach of estimating 2D image plane motions. By working in the scene space rather than the image plane, the constant velocity assumption, commonly used in the prediction stage of trackers, is far more valid, and the resulting motion estimate is richer, providing information on out of plane motions. To evaluate the performance of the system, 3D trajectories are estimated on a multi-view sign-language dataset, and compared to a traditional high accuracy 2D system, with excellent results.
The motion field of a scene can be used for object segmentation and to provide features for classification tasks like action recognition. Scene flow is the full 3D motion field of the scene, and is more difficult to estimate than it's 2D counterpart, optical flow. Current approaches use a smoothness cost for regularisation, which tends to over-smooth at object boundaries. This paper presents a novel formulation for scene flow estimation, a collection of moving points in 3D space, modelled using a particle filter that supports multiple hypotheses and does not oversmooth the motion field. In addition, this paper is the first to address scene flow estimation, while making use of modern depth sensors and monocular appearance images, rather than traditional multi-viewpoint rigs. The algorithm is applied to an existing scene flow dataset, where it achieves comparable results to approaches utilising multiple views, while taking a fraction of the time.
Recent approaches to multi-task learning (MTL) have fo-cused on modelling connections between tasks at the de-coder level. This leads to a tight coupling between tasks, which need retraining if a new task is inserted or removed. We argue that MTL is a stepping stone towards universal feature learning (UFL), which is the ability to learn generic features that can be applied to new tasks without retraining. We propose Medusa to realize this goal, designing task heads with dual attention mechanisms. The shared feature attention masks relevant backbone features for each task, allowing it to learn a generic representation. Meanwhile, a novel Multi-Scale Attention head allows the network to better combine per-task features from different scales when making the final prediction. We show the effectiveness of Medusa in UFL (+13.18% improvement), while maintaining MTL performance and being 25% more efficient than previous approaches.
Motion estimation algorithms are typically based upon the assumption of brightness constancy or related assumptions such as gradient constancy. This manuscript evaluates several common cost functions from the motion estimation literature, which embody these assumptions. We demonstrate that such assumptions break for real world data, and the functions are therefore unsuitable. We propose a simple solution, which significantly increases the discriminatory ability of the metric, by learning a nonlinear relationship using techniques from machine learning. Furthermore, we demonstrate how context and a nonlinear combination of metrics, can provide additional gains, and demonstrating a 44% improvement in the performance of a state of the art scene flow estimation technique. In addition, smaller gains of 20% are demonstrated in optical flow estimation tasks.
“Like night and day” is a commonly used expression to imply that two things are completely different. Unfortunately, this tends to be the case for current visual feature representations of the same scene across varying seasons or times of day. The aim of this paper is to provide a dense feature representation that can be used to perform localization, sparse matching or image retrieval,regardless of the current seasonal or temporal appearance. Recently, there have been several proposed methodologies for deep learning dense feature representations. These methods make use of ground truth pixel-wise correspondences between pairs of images and focus on the spatial properties of the features. As such, they don’t address temporal or seasonal variation. Furthermore, obtaining the required pixel-wise correspondence data to train in cross-seasonal environments is highly complex in most scenarios. We propose Deja-Vu, a weakly supervised approach to learning season invariant features that does not require pixel-wise ground truth data. The proposed system only requires coarse labels indicating if two images correspond to the same location or not. From these labels, the network is trained to produce “similar” dense feature maps for corresponding locations despite environmental changes. Code will be made available at: https://github.com/ jspenmar/DejaVu_Features
This paper proposes a Hybrid Approximate Representation (HAR) based on unifying several efficient approximations of the generalized reprojection error (which is known as the gold standard for multiview geometry). The HAR is an over-parameterization scheme where the approximation is applied simultaneously in multiple parameter spaces. A joint minimization scheme “HAR-Descent” can then solve the PnP problem efficiently, while remaining robust to approximation errors and local minima. The technique is evaluated extensively, including numerous synthetic benchmark protocols and the real-world data evaluations used in previous works. The proposed technique was found to have runtime complexity comparable to the fastest O(n) techniques, and up to 10 times faster than current state of the art minimization approaches. In addition, the accuracy exceeds that of all 9 previous techniques tested, providing definitive state of the art performance on the benchmarks, across all 90 of the experiments in the paper and supplementary material.
Reconstruction of 3D environments is a problem that has been widely addressed in the literature. While many approaches exist to perform reconstruction, few of them take an active role in deciding where the next observations should come from. Furthermore, the problem of travelling from the camera’s current position to the next, known as pathplanning, usually focuses on minimising path length. This approach is ill-suited for reconstruction applications, where learning about the environment is more valuable than speed of traversal. We present a novel Scenic Route Planner that selects paths which maximise information gain, both in terms of total map coverage and reconstruction accuracy. We also introduce a new type of collaborative behaviour into the planning stage called opportunistic collaboration, which allows sensors to switch between acting as independent Structure from Motion (SfM) agents or as a variable baseline stereo pair. We show that Scenic Planning enables similar performance to state-of-the-art batch approaches using less than 0.00027% of the possible stereo pairs (3% of the views). Comparison against length-based pathplanning approaches show that our approach produces more complete and more accurate maps with fewer frames. Finally, we demonstrate the Scenic Pathplanner’s ability to generalise to live scenarios by mounting cameras on autonomous ground-based sensor platforms and exploring an environment.
Prior work on Sign Language Translation has shown that having a mid-level sign gloss representation(effectively recognizing the individual signs) improves the translation performance drastically. In fact, the current state-of-theart in translation requires gloss level tokenization in order to work. We introduce a novel transformer based architecture that jointly learns Continuous Sign Language Recognition and Translation whilebeing trainable in an end-to-end manner. This is achieved by using a Connectionist Temporal Classification(CTC)loss to bind the recognition and translation problems into a single unified architecture. This joint approach does not require any ground-truth timing information,simultaneously solving two co-dependant sequence to sequence learning problems and leads to significant performance gains. We evaluate the recognition and translation performances of our approaches on the challenging RWTHPHOENIX-Weather-2014T(PHOENIX14T)dataset. Wereport state-of-the-art sign language recognition and translation results achieved by our Sign Language Transformers. Our translation net works out perform both sign video to spoken language and gloss to spoken language translation models, in some cases more than doubling the performance (9.58vs. 21.80BLEU-4Score). We also share new baseline translation results using transformer networks for several other text-to-text sign language translation tasks.
Estimating the pose of an object, be it articulated, deformable or rigid, is an important task, with applications ranging from Human-Computer Interaction to environmental understanding. The idea of a general pose estimation framework, capable of being rapidly retrained to suit a variety of tasks, is appealing. In this paper a solution is proposed requiring only a set of labelled training images in order to be applied to many pose estimation tasks. This is achieved by treating pose estimation as a classification problem, with particle filtering used to provide non-discretised estimates. Depth information extracted from a calibrated stereo sequence, is used for background suppression and object scale estimation. The appearance and shape channels are then transformed to Local Binary Pattern histograms, and pose classification is performed via a randomised decision forest. To demonstrate flexibility, the approach is applied to two different situations, articulated hand pose and rigid head orientation, achieving 97% and 84% accurate estimation rates, respectively.
Significant effort has been devoted within the visual tracking community to rapid learning of object properties on the fly. However, state-of-the-art approaches still often fail in cases such as rapid out-of-plane rotation, when the appearance changes suddenly. One of the major contributions of this work is a radical rethinking of the traditional wisdom of modelling 3D motion as appearance change during tracking. Instead, 3D motion is modelled as 3D motion. This intuitive but previously unexplored approach provides new possibilities in visual tracking research. Firstly, 3D tracking is more general, as large out-of-plane motion is often fatal for 2D trackers, but helps 3D trackers to build better models. Secondly, the tracker’s internal model of the object can be used in many different applications and it could even become the main motivation, with tracking supporting reconstruction rather than vice versa. This effectively bridges the gap between visual tracking and Structure from Motion. A new benchmark dataset of sequences with extreme out-ofplane rotation is presented and an online leader-board offered to stimulate new research in the relatively underdeveloped area of 3D tracking. The proposed method, provided as a baseline, is capable of successfully tracking these sequences, all of which pose a considerable challenge to 2D trackers (error reduced by 46 %).
Long term tracking of an object, given only a single instance in an initial frame, remains an open problem. We propose a visual tracking algorithm, robust to many of the difficulties which often occur in real-world scenes. Correspondences of edge-based features are used, to overcome the reliance on the texture of the tracked object and improve invariance to lighting. Furthermore we address long-term stability, enabling the tracker to recover from drift and to provide redetection following object disappearance or occlusion. The two-module principle is similar to the successful state-of-the-art long-term TLD tracker, however our approach offers better performance in benchmarks and extends to cases of low-textured objects. This becomes obvious in cases of plain objects with no texture at all, where the edge-based approach proves the most beneficial. We perform several different experiments to validate the proposed method. Firstly, results on short-term sequences show the performance of tracking challenging (low-textured and/or transparent) objects which represent failure cases for competing state-of-the-art approaches. Secondly, long sequences are tracked, including one of almost 30 000 frames which to our knowledge is the longest tracking sequence reported to date. This tests the redetection and drift resistance properties of the tracker. Finally, we report results of the proposed tracker on the VOT Challenge 2013 and 2014 datasets as well as on the VTB1.0 benchmark and we show relative performance of the tracker compared to its competitors. All the results are comparable to the state-ofthe-art on sequences with textured objects and superior on nontextured objects. The new annotated sequences are made publicly available.
Sign language recognition (SLR) involves identifying the form and meaning of isolated signs or sequences of signs. To our knowledge, the combination of SLR and sign language assessment is novel. The goal of an ongoing three-year project in Switzerland is to pioneer an assessment system for lexical signs of Swiss German Sign Language (Deutschschweizerische Geb¨ardensprache, DSGS) that relies on SLR. The assessment system aims to give adult L2 learners of DSGS feedback on the correctness of the manual parameters (handshape, hand position, location, and movement) of isolated signs they produce. In its initial version, the system will include automatic feedback for a subset of a DSGS vocabulary production test consisting of 100 lexical items. To provide the SLR component of the assessment system with sufficient training samples, a large-scale dataset containing videotaped repeated productions of the 100 items of the vocabulary test with associated transcriptions and annotations was created, consisting of data from 11 adult L1 signers and 19 adult L2 learners of DSGS. This paper introduces the dataset, which will be made available to the research community.
In the current monocular depth research, the dominant approach is to employ unsupervised training on large datasets, driven by warped photometric consistency. Such approaches lack robustness and are unable to generalize to challenging domains such as nighttime scenes or adverse weather conditions where assumptions about photometric consistency break down. We propose DeFeat-Net (Depth & Feature network), an approach to simultaneously learn a cross-domain dense feature representation, alongside a robust depth-estimation framework based on warped feature consistency. The resulting feature representation is learned in an unsupervised manner with no explicit ground-truth correspondences required. We show that within a single domain, our technique is comparable to both the current state of the art in monocular depth estimation and supervised feature representation learning. However, by simultaneously learning features, depth and motion, our technique is able to generalize to challenging domains, allowing DeFeat-Net to outperform the current state-of-the-art with around 10% reduction in all error measures on more challenging sequences such as nighttime driving.