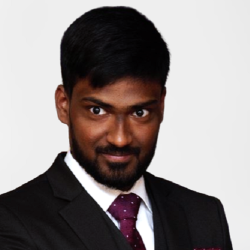
Dr Mukunthan Tharmakulasingam
Academic and research departments
Centre for Vision, Speech and Signal Processing (CVSSP), Faculty of Engineering and Physical Sciences.About
My research project
Interpretable machine learning algorithms for predicting antimicrobial resistanceThis research study develops models for predicting antimicrobial resistance (AMR) using state-of-the-art interpretable machine learning algorithms. The study tackles several practical challenges in AMR prediction, such as the complex nature of gene annotation, multiple AMRs within a single genomic sequence, and the issue of missing labels.
By effectively addressing these challenges, the proposed approach significantly enhances the accuracy and interpretability of AMR prediction. One key highlight of this research is the provision of interpretable results. Healthcare professionals and researchers gain valuable insights into the mechanisms driving antimicrobial resistance by elucidating the underlying factors and features that contribute to AMR prediction. This interpretability aspect is vital for informed decision-making, personalised treatment strategies, and optimising antimicrobial stewardship programs.
Its application in real-world scenarios can effectively support antimicrobial stewardship efforts, aiding in the development of tailored antibiotic treatments and facilitating the adoption of AMR-aware practices within various sectors, including healthcare and the food industry. Overall, this research project pioneers a sophisticated and technically advanced approach to AMR prediction by addressing missing label issues and providing interpretable results, and contributes to the advancement of precision medicine and AMR-aware food.
Supervisors
This research study develops models for predicting antimicrobial resistance (AMR) using state-of-the-art interpretable machine learning algorithms. The study tackles several practical challenges in AMR prediction, such as the complex nature of gene annotation, multiple AMRs within a single genomic sequence, and the issue of missing labels.
By effectively addressing these challenges, the proposed approach significantly enhances the accuracy and interpretability of AMR prediction. One key highlight of this research is the provision of interpretable results. Healthcare professionals and researchers gain valuable insights into the mechanisms driving antimicrobial resistance by elucidating the underlying factors and features that contribute to AMR prediction. This interpretability aspect is vital for informed decision-making, personalised treatment strategies, and optimising antimicrobial stewardship programs.
Its application in real-world scenarios can effectively support antimicrobial stewardship efforts, aiding in the development of tailored antibiotic treatments and facilitating the adoption of AMR-aware practices within various sectors, including healthcare and the food industry. Overall, this research project pioneers a sophisticated and technically advanced approach to AMR prediction by addressing missing label issues and providing interpretable results, and contributes to the advancement of precision medicine and AMR-aware food.
News
In the media
ResearchResearch interests
Explainable AI
AI for health
Digital Health
Bioinformatics
Research interests
Explainable AI
AI for health
Digital Health
Bioinformatics
Publications
Background Human, animal, and environmental health are increasingly threatened by the emergence and spread of antibiotic resistance. Inappropriate use of antibiotic treatments commonly contributes to this threat, but it is also becoming apparent that multiple, interconnected environmental factors can play a significant role. Thus, a One Health approach is required for a comprehensive understanding of the environmental dimensions of antibiotic resistance and inform science-based decisions and actions. The broad and multidisciplinary nature of the problem poses several open questions drawing upon a wide heterogeneous range of studies. Objective This study seeks to collect and catalogue the evidence of the potential effects of environmental factors on the abundance or detection of antibiotic resistance determinants in the outdoor environment, i.e., antibiotic resistant bacteria and mobile genetic elements carrying antibiotic resistance genes, and the effect on those caused by local environmental conditions of either natural or anthropogenic origin. Methods Here, we describe the protocol for a systematic evidence map to address this, which will be performed in adherence to best practice guidelines. We will search the literature from 1990 to present, using the following electronic databases: MEDLINE, Embase, and the Web of Science Core Collection as well as the grey literature. We shall include full-text, scientific articles published in English. Reviewers will work in pairs to screen title, abstract and keywords first and then full-text documents. Data extraction will adhere to a code book purposely designed. Risk of bias assessment will not be conducted as part of this SEM. We will combine tables, graphs, and other suitable visualisation techniques to compile a database i) of studies investigating the factors associated with the prevalence of antibiotic resistance in the environment and ii) map the distribution, network, cross-disciplinarity, impact and trends in the literature.
Remote medical monitoring and consultation has become indispensable in order to enhance the availability of better health-care services to the patients in remote rural areas in the country. This paper proposes an inexpensive, easy to handle Remote Medical Consultation System (RMCS) which supports the healthcare workers to carry out their services through bi-directional video and voice communication between the remote end and doctors end as well as automated measuring of medical parameters that can be controlled from both ends. RMCS is consisted of a wearable sensors kit, a centralized hardware platform which connects to the medical sensors and devices and a software platform with database for operating and managing the system. RMCS is capable of remotely measuring patients blood pressure, heart rate, body temperature, electrocardiogram (ECG), heart sounds and the systems platform supports to add-on more medical sensors or devices. The key aspect of the system is that it reduces most of the complexity in operation and facilitates the doctors to monitor and diagnose the patients in real-time. RMCS was essentially developed to eliminate the issues of low quality healthcare services in rural areas and to assist in monitoring immobilized patients.
The timely identification of pathogens is vital in order to effectively control diseases and avoid antimicrobial resistance. Non-invasive point-of-care diagnostic tools are recently trending in identification of the pathogens and becoming a helpful tool especially for rural areas. Machine learning approaches have been widely applied on biological markers for predicting diseases and pathogens. However, there are few studies in the literature that have utilized volatile organic compounds (VOCs) as non-invasive biological markers to identify bacterial pathogens. Furthermore, there is no comprehensive study investigating the effect of different distance and similarity metrics for pathogen classification based on VOC data. In this study, we compared various non-Euclidean distance and similarity metrics with Euclidean metric to identify significantly contributing VOCs to predict pathogens. In addition, we also utilized backward feature elimination (BFE) method to accurately select the best set of features. The dataset we utilized for experiments was composed from the publications published between 1977 and 2016, and consisted of associations in between 703 VOCs and 11 pathogens. We performed extensive set of experiments with five different distance metrics in both uniform and weighted manner. Comprehensive experiments showed that it is possible to correctly predict pathogens by using 68 VOCs among 703 with 78.6% accuracy using k-nearest neighbour classifier and Sorensen distance metric.
This paper presents the application of the backward feature elimination technique on an electronic nose (E-nose) to aid the rapid detection of pathogens using Volatile Organic Compounds (VOCs). The timely identification of pathogens is vital to facilitate control of diseases. E-noses are widely used for the identification of VOCs as a non-invasive tool. However, the identification of VOC signatures associated with microbial pathogens using E-nose is currently inefficient for the timely identification of pathogens. Therefore, we proposed an E-nose system integrating the backward feature elimination. Comprehensive experiments of backward feature elimination showed that they improve the classification accuracy.
In the published article, there was an error in the Article title. Instead of “VIDIIA Hunter diagnostic platform: a low-cost, smartphone connected, artificial intelligence-assisted COVID-19 rapid diagnostics approved for medical use in the UK,” it should be “VIDIIA Hunter: a low-cost, smartphone connected, artificial intelligence-assisted COVID-19 rapid diagnostic platform approved for medical use in the UK”. The authors apologize for this error and state that this does not change the scientific conclusions of the article in any way. The original article has been updated.
Until vaccines and effective therapeutics become available, the practical solution to transit safely out of the current coronavirus disease 19 (CoVID-19) lockdown may include the implementation of an effective testing, tracing and tracking system. However, this requires a reliable and clinically validated diagnostic platform for the sensitive and specific identification of SARS-CoV-2. Here, we report on the development of a de novo, high-resolution and comparative genomics guided reverse-transcribed loop-mediated isothermal amplification (LAMP) assay. To further enhance the assay performance and to remove any subjectivity associated with operator interpretation of results, we engineered a novel hand-held smart diagnostic device. The robust diagnostic device was further furnished with automated image acquisition and processing algorithms and the collated data was processed through artificial intelligence (AI) pipelines to further reduce the assay run time and the subjectivity of the colorimetric LAMP detection. This advanced AI algorithm-implemented LAMP (ai-LAMP) assay, targeting the RNA-dependent RNA polymerase gene, showed high analytical sensitivity and specificity for SARS-CoV-2. A total of similar to 200 coronavirus disease (CoVID-19)-suspected NHS patient samples were tested using the platform and it was shown to be reliable, highly specific and significantly more sensitive than the current gold standard qRT-PCR. Therefore, this system could provide an efficient and cost-effective platform to detect SARS-CoV-2 in resource-limited laboratories.
An artificial intelligence-assisted low-cost portable device for the rapid detection of severe acute respiratory syndrome coronavirus 2 (SARS-CoV-2) is presented here. This standalone temperature-controlled device houses tubes designed for conducting reverse transcription loop-mediated isothermal amplification (RT-LAMP) assays. Moreover, the device utilises tubes illuminated by LEDs, an in-built camera, and a small onboard computer with automated image acquisition and processing algorithms. This intelligent device significantly reduces the normal assay run time and removes the subjectivity associated with operator interpretation of colourimetric RT-LAMP results. To further improve this device's usability, a mobile app has been integrated into the system to control the LAMP assay environment and to visually display the assay results by connecting the device to a smartphone via Bluetooth. This study was undertaken using similar to 5000 images produced from the similar to 200 LAMP amplification assays using the prototype device. Synthetic RNA and a small panel of positive and negative SARS-CoV-2 patient samples were assayed for this study. State-of-the-art image processing and artificial intelligence algorithms were applied to these images to analyse them and to select the most efficient algorithm. The template matching algorithm for image extraction and MobileNet CNN architecture for classification results provided 98.0% accuracy with an average run time of 20 min to confirm the endpoint result. Two working points were chosen based on the best compromise between sensitivity and specificity. The high sensitivity point has a sensitivity value of 99.12% and specificity value of 70.8%, while at the high specificity point, the sensitivity is 96.05% and specificity 93.59%. Furthermore, this device provides an efficient and cost-effective platform for non-health professionals to detect not only SARS-CoV-2 but also other pathogens in resource-limited laboratories, factories, airports, schools, universities, and homes.
Predicting Antimicrobial Resistance (AMR) from genomic sequence data has become a significant component of overcoming the AMR challenge, especially given its potential for facilitating more rapid diagnostics and personalised antibiotic treatments. With the recent advances in sequencing technologies and computing power, deep learning models for genomic sequence data have been widely adopted to predict AMR more reliably and error-free. There are more than 30 different types of AMR; therefore, any practical AMR prediction system must be able to identify multiple AMRs present in a genomic sequence. Unfortunately, most genomic sequence datasets do not have all the labels marked, thereby making a deep learning modelling approach challenging owing to its reliance on labels for reliability and accuracy. This paper addresses this issue by presenting an effective deep learning solution, Mask-Loss 1D convolution neural network (ML-ConvNet), for AMR prediction on datasets with many missing labels. The core component of ML-ConvNet utilises a masked loss function that overcomes the effect of missing labels in predicting AMR. The proposed ML-ConvNet is demonstrated to outperform state-of-the-art methods in the literature by 10.5%, according to the F1 score. The proposed model's performance is evaluated using different degrees of the missing label and is found to outperform the conventional approach by 76% in the F1 score when 86.68% of labels are missing. Furthermore, the proposed ML-ConvNet is established with an explainable artificial intelligence (XAI) pipeline, thereby making it ideally suited for hospitals and healthcare settings where model interpretability is an essential requirement.
Predicting Antimicrobial Resistance (AMR) from genomic data has important implications for human and animal healthcare, and especially given its potential for more rapid diagnostics and informed treatment choices. With the recent advances in sequencing technologies, applying machine learning techniques for AMR prediction have indicated promising results. Despite this, there are shortcomings in the literature concerning methodologies suitable for multi-drug AMR prediction and especially where samples with missing labels exist. To address this shortcoming, we introduce a Rectified Classifier Chain (RCC) method for predicting multi-drug resistance. This RCC method was tested using annotated features of genomics sequences and compared with similar multi-label classification methodologies. We found that applying the eXtreme Gradient Boosting (XGBoost) base model to our RCC model outperformed the second-best model, XGBoost based binary relevance model, by 3.3% in Hamming accuracy and 7.8% in F1-score. Additionally, we note that in the literature machine learning models applied to AMR prediction typically are unsuitable for identifying biomarkers informative of their decisions; in this study, we show that biomarkers contributing to AMR prediction can also be identified using the proposed RCC method. We expect this can facilitate genome annotation and pave the path towards identifying new biomarkers indicative of AMR.
Introduction: Accurate and rapid diagnostics paired with effective tracking and tracing systems are key to halting the spread of infectious diseases, limiting the emergence of new variants and to monitor vaccine efficacy. The current gold standard test (RT-qPCR) for COVID-19 is highly accurate and sensitive, but is time-consuming, and requires expensive specialised, lab-based equipment. Methods: Herein, we report on the development of a SARS-CoV-2 (COVID-19) rapid and inexpensive diagnostic platform that relies on a reverse-transcription loop-mediated isothermal amplification (RT-LAMP) assay and a portable smart diagnostic device. Automated image acquisition and an Artificial Intelligence (AI) deep learning model embedded in the Virus Hunter 6 (VH6) device allow to remove any subjectivity in the interpretation of results. The VH6 device is also linked to a smartphone companion application that registers patients for swab collection and manages the entire process, thus ensuring tests are traced and data securely stored. Results: Our designed AI-implemented diagnostic platform recognises the nucleocapsid protein gene of SARS-CoV-2 with high analytical sensitivity and specificity. A total of 752 NHS patient samples, 367 confirmed positives for coronavirus disease (COVID-19) and 385 negatives, were used for the development and validation of the test and the AI-assisted platform. The smart diagnostic platform was then used to test 150 positive clinical samples covering a dynamic range of clinically meaningful viral loads and 250 negative samples. When compared to RT-qPCR, our AI-assisted diagnostics platform was shown to be reliable, highly specific (100%) and sensitive (98–100% depending on viral load) with a limit of detection of 1.4 copies of RNA per µL in 30 min. Using this data, our CE-IVD and MHRA approved test and associated diagnostic platform has been approved for medical use in the United Kingdom under the UK Health Security Agency’s Medical Devices (Coronavirus Test Device Approvals, CTDA) Regulations 2022. Laboratory and in-silico data presented here also indicates that the VIDIIA diagnostic platform is able to detect the main variants of concern in the United Kingdom (September 2023). Discussion: This system could provide an efficient, time and cost-effective platform to diagnose SARS-CoV-2 and other infectious diseases in resource-limited settings.
Background Drug repurposing provides an opportunity to redeploy drugs, which are already tested for safety or approved for use in humans, for the treatment of diseases distinct from their primary indication. For example, the repurposing of dexamethasone and baricitinib has played a crucial role in saving patient lives during the SARS-CoV-2 pandemic. There remains a need to expand therapeutic approaches to prevent life-threatening complications in vulnerable patients with COVID-19. Method Using an in silico approach based on structural similarity to drugs already in clinical trials for COVID-19, potential drugs were predicted for repurposing. For a subset of identified drugs with different targets to their corresponding COVID-19 clinical trial drug, a mechanism of action analysis based on affected pathways in the context of the first 24 h of infection was applied to establish whether they might have a role in inhibiting the replication of SARS-CoV-2. Results Twenty-nine structurally similar drugs with potential for repurposing against COVID-19 were predicted. Two of these with the potential to inhibit SARS-CoV-2 replication were identified using mechanism of action analysis. Triamcinolone is a corticosteroid that is structurally similar to dexamethasone; gallopamil is a calcium channel blocker that is structurally similar to verapamil. Conclusion The identification of these drugs as potentially useful for patients with COVID-19 who are at a higher risk of developing severe disease supports the use of in silico approaches to facilitate quick and cost-effective identification of drugs for repurposing. Such drugs could expand the number of treatments available to the subset of patients who are not fully protected by vaccination.
Antimicrobial Resistance (AMR) is a growing public and veterinary health concern, and the ability to accurately predict AMR from antibiotics administration data is crucial for effectively treating and managing infections. While genomics-based approaches can provide better results, sequencing, assembling, and applying Machine Learning (ML) methods can take several hours. Therefore, alternative approaches are required. This study focused on using ML for antimicrobial stewardship by utilising data extracted from hospital electronic health records, which can be done in real-time, and developing an interpretable 1D-Transformer model for predicting AMR. A multi-baseline Integrated Gradient pipeline was also incorporated to interpret the model, and quantitative validation metrics were introduced to validate the model. The performance of the proposed 1D-Transformer model was evaluated using a dataset of urinary tract infection (UTI) patients with four antibiotics. The proposed 1D-Transformer model achieved 10% higher area under curve (AUC) in predicting AMR and outperformed traditional ML models. The Explainable Artificial Intelligence (XAI) pipeline also provided interpretable results, identifying the signatures contributing to the predictions. This could be used as a decision support tool for personalised treatment, introducing AMR-aware food and management of AMR, and it could also be used to identify signatures for targeted interventions.
The software and hardware applications are clearly on the way of becoming an integral tool of business, communication and popular culture in many parts of the world. People are interacting with the environment via the Internet to perform physical activities remotely. These applications are hosted in the public or private servers under the control of the server admin. The users' online usage data can be stored in public or private cloud platforms, used for processing and monitoring users' online behaviour and emotional factors and shared with third parties to facilitate making their business decisions. When users allow their data to be collected via software applications and mobile devices, users need to have some level of trust and control over their data. But, software applications or mobile devices connected to the cloud server using client-server architecture does not ensure the reliability, security and integrity among their data. To get over these kinds of limitations, we propose a database management system using blockchain technology that can be used by any software applications. The blockchain database connected to the cloud server can be used to increase the trustfulness of the application. Blockchain has the capability to provide decentralization, immutability and owner-controlled digital assets among software applications. Since users can save their data in a shared transaction repository with tamper-resistant records, it enables related parties to access and control users' data without the need for a central control system.