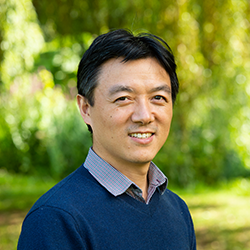
Professor Wenwu Wang
Academic and research departments
Centre for Vision, Speech and Signal Processing (CVSSP), School of Computer Science and Electronic Engineering.About
Biography
Wenwu Wang is currently a Professor of Signal Processing and Machine Learning, and a Co-Director of the Machine Audition Lab within the Centre for Vision Speech and Signal Processing. He is also an AI Fellow of Surrey Institute for People Centred Artificial Intelligence.
He was born in Anhui, China. He received the B.Sc. degree in 1997, the M.E. degree in 2000, and the Ph.D. degree in 2002, all from Harbin Engineering University, China. He then worked in King's College London (2002-2003), Cardiff University (2004-2005), Tao Group Ltd. (now Antix Labs Ltd.) (2005-2006), and Creative Labs (2006-2007), before joining University of Surrey, UK, in May 2007. He was a Visiting Scholar at the Perception and Neurodynamics Laboratory (PNL) and the Centre for Cognitive and Brain Sciences, in the Ohio State University, USA, in 2008. He is a Guest Professor at Qingdao University of Science and Technology (2018-) and at Tianjin University (2020-).
His current research interests include blind signal processing, sparse signal processing, audio-visual signal processing, machine learning and perception, artificial intelligence, machine audition (listening), and statistical anomaly detection. He has (co)-authored over 300 publications in these areas including two books: Machine Audition: Principles, Algorithms and Systems by IGI Global published in 2010 and Blind Source Separation: Advances in Theory Algorithms and Applications by Springer in 2014. His work has been funded by EPSRC, EU, Dstl, MoD, DoD, Home Office, Royal Academy of Engineering, National Physical Laboratory, BBC, and industry (including Samsung, Tencent, Huawei, Atlas, Saab, and Kaon).
He is a (co-)author or (co-)recipient of over 15 awards including the IEEE Signal Processing Society (SPS) 2022 Young Author Best Paper Award, ICAUS 2021 Best Paper Award, DCASE 2020 and 2023 Judges' Award, DCASE 2019 and 2020 Reproducible System Award, LVA/ICA 2018 Best Student Paper Award, FSDM 2016 Best Oral Presentation Award, ICASSP 2019 and LVA/ICA 2010 Best Student Paper Award Nominees, 2016 TVB Europe Award for Best Achievement in Sound, 2012 Best Solution Award on the Dstl Challenge, and the 1st place in 2020 DCASE challenge on "Urban Sound Tagging with Spatial-Temporal Context", and the 1st place in the 2017 DCASE Challenge on "Large-scale Weakly Supervised Sound Event Detection for Smart Cars". He also received the Outstanding Graduate Award in 2000, the Excellent Paper Award in 2000, the Excellent Thesis Award in 2000 and numerous Scholarships for academic excellence from Harbin Engineering University. He was on the 2021 and 2022 Stanford University list of World Top 2% Scientists.
He is an Associate Editor (2020-) for IEEE/ACM Transactions on Audio Speech and Language Processing, an Associate Editor of (Nature) Scientific Report (2022-), a Specialty Editor in Chief (2021-) of Frontier in Signal Processing, and an Associate Editor (2019-) for EURASIP Journal on Audio Speech and Music Processing. He was a Senior Area Editor (2019-2023) and an Associate Editor (2014-2018) for IEEE Transactions on Signal Processing, and Senior Area Editor of Digital Signal Processing (2021-2023). He is elected Chair (2023-2024) of IEEE SPS Machine Learning for Signal Processing Technical Committee, elected Vice Chair (2022-2024) of EURASIP Technical Area Committee on Acoustic Speech and Music Signal Processing, Board Member (2023-2024) of IEEE SPS Technical Directions Board, an elected Member (2021-) of the IEEE Signal Processing Theory and Methods Technical Committee, and an elected Member (2019-) of the International Steering Committee of Latent Variable Analysis and Signal Separation. He was a Local Arrangement Co-Chair of IEEE MLSP 2013, Publicity Co-Chair of IEEE SSP 2009, Publication Co-Chair for ICASSP 2019 and a Satellite Workshop Co-Chair for INTERSPEECH 2022 and ICASSP 2024, a Special Session Co-Chair for MLSP 2024, and a Technical/Program Committee Member of over 100 international conferences. He is a Senior Member of IEEE, and a Fellow of the Higher Education Academy.
Please visit my personal page for more information, including downloadable publications and codes.
Areas of specialism
News
[10/2023] Invited Keynote Speaker on SoRAIM 2023 Winter School, Grenoble, France, February 19th-23rd, 2024.
[10/2023] Invited Speaker on SANE 2023, Speech and Audio in the Northeast, 26th October 2023, New York.
[09/2023] Won the Judge's Award on DCASE 2023, Tampere, Finland, for the work "Text-Driven Foley Sound Generation With Latent Diffusion Model", co-authored by Y. Yuan, H. Liu, X. Liu, X. Kang, M. D. Plumbley, and W. Wang. This is an improved version of AudioLDM tuned for the DCASE challenge task 7 dataset. See the full paper, an earlier report, and the code.
[08/2023] Invited Survey Talk on the 24th INTERSPEECH Conference Interspeech 2023, 20-24 August, Dublin, Ireland. Interspeech 2023 had about 2000 attendees. [Talk ppt slides download]
[07/2023] WavJourney: a new compositional text-to-audio generation model, released in August 2023. The WavJourney system can be used to create audio content with storylines encompassing speech, music, and sound effects, guided by text instructions. WavJourney leverages large language models to connect various audio models for audio content generation, applicable across diverse real-world scenarios, including science fiction, education, and radio play.
[07/2023] Following the release of the powerful text-to-audio model AudioLDM in February 2023, here comes an even more powerful model AudioLDM 2 with paper/code/demos released in August 2023. AudioLDM 2 is a novel and versatile audio generation model capable of performing conditional audio, music, and intelligible speech generation. AudioLDM 2 achieves state-of-the-art (SoTA) performance in text-to-audio and text-to-music generation, while also delivering competitive results in text-to-speech generation, comparable to the current SoTA.
[07/2023] Satellite Workshop Co-Chair for ICASSP 2024 - the 49th IEEE International Conference on Acoustics, Speech, and Signal Processing, to be held in Seoul, South Korea.
[06/2023] Invited Talk on the Workshop of Advances in Neuromorphic AI and Electronics 2023, 26-29 June, Loughborough, UK.
[06/2023] Congratulations to Thomas Marshall for winning The BAE Systems Applied Intelligence Prize for his final-year project "Using Electroencephalogram and Machine Learning for Prosthetics", completed under my supervision.
[06/2023] Our system is ranked at the First Position in DCASE 2023 Challenge Task 7 (Foley Sound Synthesis). More details can be found from the results, paper, and the code.
[06/2023] Honored to be an invited Perspective Talks Speaker on 48th IEEE International Conference on Acoustics, Speech, and Signal Processing (ICASSP 2023), held at Rhodes, Greece, on June 4-10, 2023. This is one of the six Perspective Talks (three academic & three industrial) on the conference. ICASSP is the flagship conference in the area of signal processing, attracting 3000+ attendees each year.
[04/2023] Keynote Speaker on the 10th National Conference on Sound and Music Technology (CSMT 2023), held at Guangzhou, China, on June 2-4, 2023.
[03/2023] Our first text to audio generation method has been selected as the baseline system for Task 7 (Foley Sound Synthesis) of the DCASE 2023 Challenge. This method, presented on IEEE MLSP 2021, was, to our knowledge, among the first in the field of general audio generation with text input, e.g. dog barking, people talking, baby crying. Our earlier work on sound generation include:
[02/2023] AudioLDM: a powerful state-of-the-art method for generating speech/sound effects/music and beyond, in terms of text description, e.g. "A hammer is hitting a wooden surface". Somebody on social media called it "ChatGPT for audio". It is now one of the 25 most-liked machine learning apps on Hugging Face Spaces among 25000+ apps. It can also be downloaded from Replicate and Zenodo. Since its release in early February 2023 by Haohe Liu (our second year PhD), it has attracted significant attention in the community and on the social media. Typing "AudioLDM" in Google will pop up at least six pages of entries discussing AudioLDM. Some examples of media attention about this work include: Youtube: 1, 2, and more, MarkTechPost, Note, MachineHeart, and many twits on Twitter and LinkedIn. This tool has been integrated by others into their apps such as AI Albums, Diffuser Library, and Image to Audio Generation. Please check out the project page for the paper, code, and demos of this fantastic method. See the University Press Release here.
[01/2023] Featured Article "Automated audio captioning: an overview of recent progress and new challenges" [PDF]
[01/2023] Board Member, IEEE SPS Technical Directions Board.
[12/2022] IEEE SPS Young Author Best Paper Award given to our former PhD graduates Qiuqiang Kong and Turab Iqbal for the paper co-authored with Yin Cao, Yuxuan Wang, Wenwu Wang, and Mark D. Plumbley, "PANNs: large-scale pretrained audio neural networks for audio pattern recognition", IEEE/ACM Transactions on Audio Speech and Language Processing, 2020. [PDF] [code] [IEEE award page]
[08/2022] Invited Plenary Speaker on the 21st UK Workshop on Computational Intelligence (UKCI 2022), 7-9 September 2022, Sheffield.
[07/2022] Achieved excellent results on DCASE 2022 Challenge: the Second Place on Task 5 - Few Shot Bioacoustic Event Detection (results, paper, and code), the Second Place on Task 6b - Language based Audio Retrieval (results, paper, and code), the Third Place in DCASE 2022 Challenge Task 6a - Automated Audio Captioning (results, paper, and code).
[06/2022] Elected Vice Chair of EURASIP Technical Area Committee on Acoustic Speech and Music Signal Processing (TAC-ASMSP). Thanks the TAC-ASMSP members for voting me.
[04/2022] Appointed Associate Editor for (Nature) Scientific Reports.
[03/2022] Awarded two projects by SAAB in the area of intelligent information fusion for distributed sensor networks and sensor swarms, with Prof Pei Xiao (CI), 5G/6G Innovation Centre, Institute of Communication Systems.
[02/2022] Plenary Speaker on GHOST DAY Applied Machine Learning Conference, 24-26 March, 2022.
[01/2022] Awarded an EPSRC iCASE project, titled "Differentiable particle filters for data-driven sequential inference", with Dr Yunpeng Li (PI), and industrial partner National Physical Laboratory. The university also provides a second project to match the iCASE award.
[01/2022] Appointed AI Fellow of the newly established Surrey Institute for People Centred Artificial Intelligence.
[11/2021] Elected Vice Chair of IEEE Machine Learning for Signal Processing Technical Committe, to serve from January 2022. Thanks the TC members for voting me.
[11/2021] Proud to be on the Standford University List of World Top 2% Scientists. See more information here.
[10/2021] Plenary Speaker of the International Workshop on Neuro-engineering and Signal Processing for Diagonostics and Control, Taiyuan, China, 15-17, October, 2021.
[09/2021] Won the Best Paper Award on the 2021 International Conference on Autonomous Unmanned Systems (ICAUS 2021). See the full paper here.
[08/2021] Keynote Speaker, 8th International Conference on Signal Processing and Integrated Networks (SPIN 2021), Noida Delhi/NCR, India, August 26-27, 2021.
[07/2021] Achieved the Third Place in DCASE 2021 Challenge on Task 6 - Automated Audio Captioning. Check the results here, and the paper here.
[04/2021] Awarded a five-year EPSRC grant, under the Prosperity Partnership scheme, worth £15M (including industrial supports), titled "BBC Prosperity Partnership: AI for Future Personalised Media Experiences". (Surrey investigators: Prof Adrian Hilton (PI, project lead), CIs: Dr Philip Jackson, Dr Armin Mustafa, Dr Jean-Yves Guillemaut, Dr Marco Volina, and Prof Wenwu Wang; Project manager: Mrs Elizabeth James) [The project is led by University of Surrey, in collaboration with BBC and University of Lancaster, with supports from 10+ industrial partners.] (See project website for more information, and the press releases by Surrey, UKRI, and BBC)
[04/2021] Appointed Award Sub-Committee Chair of IEEE Machine Learning for Signal Processing Technical Committe.
[03/2021] Invited to serve as Satellite Workshop Co-chair on the organising committee of INTERSPEECH 2022, to be held in Incheon, Korea, 18-22 September, 2022. INTERSPEECH is the flagship conference in speech/language science and technology with 1000+ attendees each year.
[03/2021] Keynote Speaker, on Robotics and Artificial Intelligence Virtual Conference (V-Robot2021), 27-28 March, 2021.
[02/2021] Awarded a £250k two-year British Council grant (Newton Institutional Links Award), titled "Automated Captioning of Image and Audio for Visually and Hearing Impaired". (Surrey investigators: Prof Wenwu Wang (PI), project lead). [jointly with Izmir Katip Celebi University (IKCU) (Dr Volkan Kilic).]
[01/2021] Invited Keynote Speaker, Global Summit and Expo on "Robot Intelligence Technology and Applications" (GSERITA2021), Lisbon, Portugal, September 06-08, 2021.
[12/2020] Keynote Speaker, Workshop on Intelligent Navigation and Advanced Information Fusion Technology, Harbin, China, December 12-13, 2020. (1000+ attendees online)
[11/2020] Elected Member of two IEEE Technical Committees: IEEE Signal Processing Theory and Methods Technical Committee & IEEE Machine Learning for Signal Processing Technical Committe, both for a three-year term to start on 1st January 2021.
[11/2020] Won two awards on DCASE 2020, November, 2-4, Tokyo, Japan. The paper "Incorporating Auxiliary Data for Urban Sound Tagging", authored by Turab Iqbal, Yin Cao, Mark D. Plumbley and Wenwu Wang, was given the Judge's Award, for "the method considered by the judges to be the most interesting or innovative". The paper "Event-Independent Network for Polyphonic Sound Event Localization and Detection", authored by Yin Cao, Turab Iqbal, Qiuqiang Kong, Zhong Yue, Wenwu Wang, and Mark D. Plumbley, was given the Reproducible system award, for "the highest scoring method that is open-source and fully reproducible". Read the university newsletter here.
[10/2020] Awarded a $1.2M three-year DoD & MoD grant (UDRC phase 3 application theme on Signal and Information Processing for Decentralized Intelligence, Surveillance, and Reconnaissance), titled "SIGNetS: signal and information gathering for networked surveillance". (Surrey investigators: Prof Wenwu Wang (PI), and Prof Pei Xiao (CI)). [The project is led by University of Cambridge (Prof Simon Godsill), jointly with University of Surrey and University of Sheffield (Prof Lyudmila Mihaylova).] (project website)
[10/2020] Awarded a £500k MoD grant (DASA call on Countering Drones), titled "Acoustic surveillance", to develop AI technologies for drone detection with acoustic sensors. (Surrey investigators: Prof Wenwu Wang). [The project is led by Airspeed.]
[09/2020] Awarded a £2.3M three-year EPSRC grant (responsive mode), titled "Multimodal video search by examples". (Surrey investigators: Prof Josef Kittler (PI), Prof Miroslaw Bober (CI), Prof Wenwu Wang (CI) and Prof Mark Plumbley (CI). [The project is led by Ulster University (Prof Hui Wang), jointly with University of Surrey and University of Cambridge (Prof Mark Gales).]
[08/2020] Keynote Speaker on the 6th International Conference on Machine Vision and Machine Learning (MVML 2020), Prague, Czech Republic, August 13-15, 2020.
[07/2020] Achieved the First Place (with Turab Iqbal, Yin Cao, and Mark Plumbley) on the DCASE 2020 challenge on Task 3: "Urban Sound Tagging with Spatiotemporal Contexts".
[07/2020] Awarded a three-year industrial project by Tencent AI Lab (Seattle, US), titled "Particle flow PHD filtering for audio-visual multi-speaker speech tracking". Surrey Investigator: Prof Wenwu Wang (PI). Industry partner: Tencent (Dr Yong Xu).
[05/2020] Awarded an EPSRC impact acceleration account (IAA) project titled "audio tagging for meta data generation of media for programme recommendation". Surrey Investigators: Prof Wenwu Wang (PI) and Prof Mark Plumbley (CI). Industry partner: BBC (Dr Chris Baume and Dr Chris Pike).
[02/2020] External PhD Examiner at Nanyang Technological University, Singapore.
[01/2020] Appointed Associate Editor (2020-) for IEEE/ACM Transactions on Audio Speech and Language Processing.
[01/2020] Awarded a 2020 DUO-India Professor Fellowship, to study deep embedding techniques in audio scene classification and event detection.
[12/2019] Keynote Speaker on the IEEE International Conference on Signal, Information and Data Processing (ICSIDP 2019), Chongqing, China, 11-13 December 2019, with 1000+ attendees.
[12/2019] External PhD Examiner at University of Oxford, Imperial College London, Queen Mary University of London, and Newcastle University.
[11/2019] Elected Member of the International Steering Committee of Latent Variable Analysis and Signal Separation.
[10/2019] CVSSP audio team (Yin Cao, Turab Iqbal, Qiuqiang Kong, Miguel Blanco Galindo, Wenwu Wang and Mark Plumbley) was given the Reproducible System Award on the DCASE 2019 Workshop for their system "Sound Event Localization and Detection". The award was given to recognize the quality, innovation and reproducibility of the work. Our system is described in this paper. See here for the source codes that implement the system.
[07/2019] I gave 32 hours invited lectures in a Summer School on Machine Learning, in Beijing Post and Telecommunication University.
[07/2019] CVSSP audio team (Yin Cao, Turab Iqbal, Qiuqiang Kong, Miguel Blanco Galindo, Wenwu Wang and Mark Plumbley) did well in the DCASE 2019 Challenge Task 3 (Sound Event Localization and Detection). The team was ranked 2nd overall out of 23 teams, and the top academic team. More details can be found from here. See here for the paper that describes our proposed system, and here for source codes for implementing the system.
[05/2019] ICASSP 2024 will be held in Seoul, South Korea. I will serve as Tutorial Chair on the organising committee.
[05/2019] ICASSP 2019 was successfully held during 12-17 May, in Brighton, UK, with 3100+ attendees from all over the world. I served as Publication Chair on the organising committee.
[05/2019] Congratulations to Qiuqiang Kong for the acceptance by IJCAI 2019 of our paper "Single-Channel Signal Separation and Deconvolution with Generative Adversarial Networks". Acceptance rate this year: 850/4752 = 17.9%. IJCAI is a flagship conference in AI, along with NIPS and AAAI.
[05/2019] Congratulations to Yang Liu for being selected as a Best Student Paper Award Finalist on IEEE International Conference on Acoustics, Speech and Signal Processing (ICASSP 2019), Brighton, UK, for our paper: Y. Liu, Q. Hu, Y. Zou, and W. Wang, "Labelled non-zero particle flow for SMC-PHD filtering". [PDF]
[01/2019] Appointed Senior Area Editor (2019-) for IEEE Transactions on Signal Processing. TSP is the flagship journal in the area of signal processing.
[11/2018] Plenary Speaker on the 7th International Conference on Signal and Image Processing, November 28-30, 2018, Sanya, China.
[11/2018] Awarded a Guest Professor by Qingdao University of Science and Techonology, China.
[11/2018] Keynote Speaker on the 6th China Conference on Sound and Music Technology, November 24-26, 2018, Xiamen, China.
[11/2018] Congratulations to Turab Iqbal for being awarded the CVSSP Outstanding First Year PhD.
[10/2018] Invited Keynote Speaker on the International Conference on Digital Image and Signal Processing, April 29-30, 2019, Oxford, UK.
[08/2018] Keynote Speaker on the China Computer Federation (CCF) Workshop on Sparse Representation and Deep Learning, Shenzhen, China.
[08/2018] We finished on the 3rd place among the 558 teams worldwide who participated the Kaggle "Freesound General-Purpose Audio Tagging Challenge" (Can you automatically recognize sounds from a wide range of real-world environments?). Congratulations to Turab Iqbal and Qiuqiang Kong for this great achievement. See here for the competition results, and here for the paper that describes our proposed system. Read the university press release here.
[07/2018] Best Student Paper Award on the 14th International Conference on Latent Variable Analysis and Signal Separation (LVA/ICA 2018). Congratulations to Lucas Rencker. Paper: Consistent dictionary learning for signal declipping. The Matlab codes can be found from Lucas Rencker's Github page or personal page. Read the university news here.
[06/2018] Invited seminar at Oxford University, Machine Learning Group, "Deep learning for audio classification".
[12/2017] Plenary Speaker on 3rd Intelligent Signal Processing Conference, London.
[11/2017] Plenary Speaker in Alan Turing Institute, Workshop on Data Science and Signal Processing.
[09/2017] CVSSP audio team (Yong Xu, Qiuqiang Kong, Wenwu Wang and Mark D. Plumbley) won the 1st prize on the DCASE2017 Challenge for the task "large-scale weakly supervised sound event detection for smart cars"!. DCASE 2017 challenge is organized by TUT, CMU and INRIA, sponsored by Google and Audio Analytic. The CVSSP team submitted four systems to the audio tagging sub-task, which took all the top four places on the result table, among the 31 systems submitted by a number of organisations. CVSSP's system is also ranked at the 3rd place in the sound event detection subtask, among 17 systems. The competitors include CMU, New York University, Bosch, USC, TUT, Singapore A*Star, Korean Advanced Institute of Science and Technology, Seoul National University, National Taiwan university, etc. More details about the systems we submitted can be found from here. The competition results can be found from here. Read the university news here.
[02/2017] CVSSP awarded a £1.5M five-year EPSRC platform grant entitled "Audio-Visual Media Research". The project is led by Prof Adrian Hilton with co-investigators including Mark Plumbley, Josef Kittler, Wenwu Wang, John Collomosse, Philip Jackson, and Jean-Yves Guillemaut.
[11/2016] Congratulations to Lucas Rencker for winning the CVSSP Directors Award for Outstanding First Year PhD Performance after his PhD confirmation on "Sparse representations for audio restoration and inpainting".
[10/2016] S3A and BBC Research have won the TVB Europe Award for Best Achievement in Sound for "The Turning Forest" VR sound experience using the spatial audio Radio Drama produced in S3A integrated into an immersive audio-visual experience by the BBC. The award was made at the European TV Industry awards last night, with S3A winning against entries from Britain's Got Talent, Sky's the Five and BBC TV Programme coverage. More details can be found from here.
[10/2015] Awarded a €2.98M three-year EC Horizon 2020 grant entitled "ACE-CReAte: Audio Commons - an Ecosystem for Creative Use of Audio Contents". The project is led by Universitat Pompeu Fabra (Spain), in collaboration with University of Surrey, Queen Mary University of London, Jamendo SA (LU), AudioGaming (France), and Waves Audio Ltd (Ireland). Surrey team is composed of Prof Mark Plumbley (PI), Dr Wenwu Wang, Dr Tim Brookes (Institute of Sound Recording), and Dr David Plans (School of Business).
[09/2015] Awarded a £1.3M three-year EPSRC grant entitled "Making Sense of Sounds". The project is led by University of Surrey, in collaboration with University of Salford. Surrey team is composed of Prof Mark Plumbley (Lead and PI of the whole project), Dr Wenwu Wang, Dr Philip Jackson, and Prof David Frohlich (Digital World Research Centre).
[03/2014] Congratulations to Jing Dong for winning the IEEE Signal Processing Society Travel Grant to attend the ICASSP 2014 conference in Florence, Italy.
[01/2014] We were delighted to see that Figure 6 of our paper below was shown on the front page of IEEE Transactions on Signal Processing: Q. Liu, W. Wang, P. Jackson, M. Barnard, J. Kittler, and J.A. Chambers, "Source Separation of Convolutive and Noisy Mixtures using Audio-Visual Dictionary Learning and Probabilistic Time-Frequency Masking", IEEE Transactions on Signal Processing, vol. 61, no. 22, pp. 5520-5535, 2013. [PDF]
[10/2013] Congratulations to Volkan Kilic for winning the CVSSP Directors Award for Outstanding Performance in the First Year of his PhD. Research topic: Audio-visual tracking of multiple moving speakers.
[10/2013] Awarded an industrial project entitled "Enhancing Speech Quality Using Lip Tracking" by Samsung Electronics Research Institute (UK). Industry partner: Dr Holly Francis (Samsung).
[09/2013] Awarded a £5.4M (FEC: £6.5M) five-year EPSRC programme grant entitled "S3A: Future Spatial Audio for an Immersive Listener Experience at Home". The project is led by University of Surrey, in collaboration with University of Southampton, University of Salford and BBC. Surrey team is composed of Prof Adrian Hilton (Lead and PI of the whole program), Dr Philip Jackson, Dr Wenwu Wang and Dr Tim Brookes (Institute of Sound Recording).
[03/2013] IEEE Signal Processing Society Travel Grant. Congratulations to Volkan Kilic for winning this competitive award for attending the ICASSP 2013 conference in Vancouver, Canada.
[12/2012] Awarded a £4.4M (FEC) five-year project supported by the EPSRC and Dstl entitled "Signal Processing Solutions for the Networked Battlespace". Our consortium, as part of the Phase II of the UDRC in Signal Processing, is composed of Loughborough, Surrey, Strathclyde and Cardiff (LSSC) universities, as well as six industry partners QinetiQ, Selex-Galileo, Thales, Texas Instruments, PrismTech and Steepest Ascent. Surrey team is composed of Dr Wenwu Wang (PI), Prof Josef Kittler and Dr Philip Jackson. The project was led by Prof Jonathon Chambers.
[09/2012] Best Solution Award on the DSTL Challenge Workshop for the signal processing challenge "Undersampled Signal Recognition", announced on the SSPD 2012 conference, London, September 25-27, 2012. Congratulations to Qingju Liu for this achievement.
Selected Activities
Editorial Activities
- Senior Area Editor, IEEE Transactions on Signal Processing, 2019-2023
- Associate Editor, IEEE/ACM Transactions on Audio Speech and Language Processing, 2020-present.
- Associate Editor, (Nature) Scientific Reports
- Specialty Editor in Chief, Frontiers in Signal Processing, 2021-present.
- Associate Editor, EURASIP Journal on Audio Speech and Music Processing, 2019-present
- Guest Editor, IEEE Transactions on Circuits and Systems for Video Technology, 2023-2024
- Senior Area Editor, (Elsevier) Digital Signal Processing, 2021-2023
- Associate Editor, IEEE Transactions on Signal Processing, 2014-2018
- Associate Editor, The Scientific World Journal: Signal Processing (Hindawi), 2014-2016
Technical Committee Activities
- Board Member of IEEE SPS Technical Directions Board, 2023-2024
- Chair of IEEE SPS Machine Learning for Signal Processing Technical Committee, 2023-2024
- Vice Chair of EURASIP Technical Area Committee on Acoustic Speech and Music Signal Processing, 2022-2024
- Award Sub-Committee Chair, IEEE Machine Learning for Signal Processing Technical Committe, 2021-2022
- Elected Member, IEEE Signal Processing Theory and Methods Technical Committee, 2021-present
- Elected Member, IEEE Machine Learning for Signal Processing Technical Committe, 2021-present
- Elected Member, International Steering Committee of Latent Variable Analysis and Signal Separation (LVA/ICA), 2019-present
Selected Conference Activities
- Satellite Workshop Co-Chair, 2022 Interspeech Conference (INTERSPEECH 2022), Incheon, Korea.
- Publication Co-Chair, 2019 IEEE International Conference on Acoustics, Speech and Signal Processing (ICASSP 2019), Brighton, UK.
- Local Arrangement Co-Chair, 2013 IEEE International Conference on Machine Learning for Signal Processing (MLSP 2013), Southampton, UK.
- Publicity Co-Chair, 2009 IEEE International Workshop on Statistical Signal Processing (SSP 2009), Cardiff, UK.
- Session Chairs, for 10+ conferences such as ICASSP 2021, IJCAI 2019, DSP 2015, ISP 2015, DSP 2013, ICASSP 2012, SSPD 2012, EUSIPCO 2012, EUSIPCO 2011, and WCCI 2008.
Regular Technical/Program Committees
I am a regular (or irregular) technical and program committee members of some major signal processing and machine learning conferences, such as:
- ICASSP, Interspeech, MLSP, SSP, EUSIPCO, WASPAA, SSPD, DSP, MMSP, NeuIPS, IJCAI, ICML, AAAI, BMVC, ICPR, UKCI, WCCI, ISNN, etc.
External PhD Examination
- 2023.05.1x, Leicester University, PhD Thesis: Big Data Analytics and Machine Learning Tools for Space and Earth Observation.
- 2023.05.03, University College London, PhD Thesis: Optimal Transport for Latent Variable Models.
- 2023.04.24, Queen Mary University of London, PhD Thesis: Deep Learning Methods for Instrument Separation and Recognition.
- 2023.03.21, Sheffield University, PhD Thesis: Machine Learning Methods for Autonomous Classification and Decision Making.
- 2023.02.10, Aalborg University (Denmark), PhD Thesis: Data-driven Speech Enhancement: from Non-negative Matrix Factorization to Deep Representation Learning.
- 2023.01.11, Multimedia University (Malaysia), PhD Thesis: Automated Detection of profanities for film censorship using deep learning.
- 2022.04.20, Brunel University, PhD Thesis: Fast embedding for image classification & retrieval and its application to the hostel industry.
- 2022.01.18, Edinburgh University, PhD Thesis: Data aware sparse non-negative signal processing.
- 2021.12.10, International Islamic University (Pakistan), PhD Thesis: Optimized implementation of multi-layer convolutional sparse coding framework for high dimensional data.
- 2021.11.25, Newcastle University, PhD Thesis: Advanced informatics for event detection and temporal localization.
- 2021.09.20, Imperial College London, PhD Thesis: Super-resolved localization in multipath environments.
- 2021.06.29. Nanyang Technological University (Singapore), PhD Thesis: Audio intelligence and domain adaptation for deep learning models at the edge in smart cities.
- 2021.04.12, Leicester University, PhD Thesis: Learning and generalisation for high-dimensional data.
- 2021.03.20, National Institute of Technology Meghalaya (India), PhD Thesis: Building robust acoustic models for an automatic speech recognition system.
- 2020.02.15, Nanyang Technological University (Singapore), PhD Thesis: Complex-valued mixing matrix estimation for blind separation of acoustic convolutive mixtures
- 2019.12.02, Oxford University, PhD Thesis: Recurrent neural networks for time series prediction.
- 2019.12.05, Queen Mary University of London, PhD Thesis: Intelligent control of dynamic range compressor.
- 2019.11.20, Imperial College London, PhD Thesis: Deep dictionary learning for image enhancement.
- 2019.11.25, Newcastle University, PhD Thesis: Signal processing and machine learning techniques for automatic image-based facial expression recognition.
- 2019.03.08, University of East Anglia, PhD Thesis: Audio speech enhancement using masks derived from visual speech.
- 2017.01.12, Loughborough University, PhD Thesis: Enhanced Independent Vector Analysis for Speech Separation in Room Environments.
- 2016.10.28, Queen Mary University of London, PhD Thesis: Music transcription using NMF.
- 2016.07.29, Southampton University, PhD Thesis: Source separation in underwater acoustic problems.
- 2016.03.04, Aalborg University (Denmark), PhD Thesis: Enhancement of speech signals - with a focus on voiced speech models.
- 2015.10.02, Edinburgh University, PhD Thesis: "Acoustic source localization and tracking using microphone arrays".
- 2015.09.15, Loughborough University, PhD Thesis: "Loughborough University Spontaneous Expression Database and Baseline Results for Automatic Emotion Recognition".
- 2014.11.21, Cardiff University, MPhil Thesis: "Joint EEG-fMRI signal model for EEG separation and localization".
- 2013.11.20, Loughborough University, PhD Thesis: "Enhanced independent vector analysis for audio separation in a room environment".
- 2012.12.10, Queen Mary University of London, PhD Thesis: "Sparse approximation and dictionary learning with applications to audio signals".
- 2010.10.13, University of Edinburgh, PhD Thesis: "Acoustic source localization and tracking using microphone arrays".
ResearchResearch interests
- Unsupervised learning techniques (including independent component analysis, independent vector analysis, latent variable analysis, sparse component analysis, non-negative matrix/tensor factorisation, low-rank representation, manifold learning, and subspace clustering)
- Supervised learning techniques (including deep learning, dictionary learning, multimodal learning, and learning with priors and signal properties)
- Computational auditory scene analysis (audio scene recognition, audio event detection, audio tagging, and audio captioning)
- Audio signal separation (convolutive audio source separation, underdetermined audio source separation including monaural source separation)
- Audio feature extraction and perception (including pitch detection, onset detection, rhythm detection, music transcription and low bit-rate audio coding)
- Sound source localisation (using audio, video, depth information, with particle filtering, PHD filtering, and/or particle flow filtering)
- Multimodal speech source separation (audio/visual source separation with modelled based techniques such as Gaussian mixture model and learning-based method such as audio-visual dictionary learning)
- Sparse representation and compressed sensing (synthesis model and analysis model based dictionary learning for sparse represenation, with applications to audio source separation, speech enhancement, audio inpainiting, and image enhancement)
- Cocktail party processing (using techniques such as independent component analysis, blind source separation, computational auditory scene analysis, sparse representation/dictionary learning, Gaussian mixture modelling and expectation maximisation, and multimodal fusion)
- Non-negative sparse coding of audio signals (including sparsity constrained non-negative matrix factorisation for audio analysis)
- 3D positional audio technology (including head-related transfer functions, binaural modelling, multiple loudspeaker panning, and room geometry estimation)
- Approximate joint diagonalization for source separation (including unitary or non-unitary constrained joint diagonalization approaches)
- Robust solutions for permutation problem of frequency domain independent component analysis (including approaches using filter constraints, statistical characteristics of signals, and beamforming)
- Convex and non-convex optimisation (gradient descent, Newton methods, interior point method, ADMM, etc.)
- Psychoacoustics motivationed signal processing and machine learning methods (e.g. time-frequency masking, perceptually informed speech separation/enhancement, intelligibility adaptive speech separation algorithms)
Funded Projects (Grants)
I appreciate the financial support for my research from the following bodies (since 2008): Engineering and Physical Science Research Council (EPSRC), Ministry of Defence (MoD), Defence Science and Technology Laboratory (Dstl), Department of Defence (DoD), Home Office (HO), Royal Academy of Engineering (RAENG), European Commission (EC), National Natural Science Foundation of China (NSFC), Shenzhen Science and Technology Innovation Council (SSTIC) of China, the University Research Support Fund (URSF), and the Ohio State University (OSU), and UK/EU industries including BBC, Atlas, Kaon, Huawei, Tencent, NPL, and Samsung. [Total award to Surrey where I am a Principal Investigator (PI) or Co-Investigator (CI): £13M+ (as PI £2.2M, as CI £14M+). As PI/CI, on a total grant award portfolio: £30M+]
- 04/2022-04/2026, PI, "Uncertainty modelling and quantification for heterogeneous sensor/effector networks", SAAB (Surrey investigators: Wenwu Wang (PI), Pei Xiao (CI)) Project partner: SAAB.
- 04/2022-04/2026, PI, "Cooperative sensor fusion and management for distributed sensor swarms", SAAB (Surrey investigators: Wenwu Wang (PI), Pei Xiao (CI)) Project partner: SAAB.
- 01/2022-01/2026, CI, "Differentiable particle filters for data-driven sequential inference", EPSRC & NPL (iCASE). (Surrey investigators: Yunpeng Li (PI), Wenwu Wang (CI)) Project partner: National Physical Laboratory.
- 07/2021-07/2026, CI, "BBC Prosperity Partnership: AI for Future Personalised Media Experiences", EPSRC (Prosperity Partnership scheme). (Surrey investigators: Adrian Hilton (PI, project lead), CIs: Philip Jackson, Armin Mustafa, Jean-Yves Guillemaut, Marco Volina, and Wenwu Wang; project manager: Elizabeth James ) [The project is led by University of Surrey, jointly with BBC and University of Lancaster, with supports from 10+ industrial partners.] (project website)
- 07/2021-07/2024, CI, "Uncertainty Quantification for Robust AI through Optimal Transport", University of Surrey (project-based Doctoral College studentship competition). (Surrey investigators: Yunpeng Li (PI), CI: Wenwu Wang.
- 02/2021-02/2023, PI, "Automated Captioning of Image and Audio for Visually and Hearing Impaired", British Council (Newton Institutional Links Award). (Surrey investigators: Wenwu Wang) [The project is led by University of Surrey, jointly with Izmir Katip Celebi University (IKCU) @ Turkey (Volkan Kilic).]
- 04/2021-04/2024, CI, "Multimodal video search by examples", EPSRC (responsive mode). (Surrey investigators: PI: Josef Kittler, CIs: Miroslaw Bober, Wenwu Wang and Mark Plumbley) [The project is led by Ulster University (Hui Wang), jointly with Univ. of Surrey and Univ of Cambridge (Mark Gales).]
- 01/2021-10/2022, PI, "Acoustic surveillance", MoD (DASA call on countering drones). (Surrey investigators: Wenwu Wang) [The project is led by Airspeed Electronics.]
- 11/2020-11/2023, PI, "SIGNetS: signal and information gathering for networked surveillance", DoD & MoD (UDRC phase 3 call on the application theme Signal and Information Processing for Decentralized Intelligence, Surveillance, and Reconnaissance). (Surrey investigators: Wenwu Wang (PI) and Pei Xiao (CI)) [The project is a collaboration between University of Cambridge (Simon Godsill, project lead), University of Surrey, and University of Sheffield (Lyudmila Mihaylova).] (project website)
- 08/2020-08/2023, PI, "Particle flow PHD filtering for audio-visual multi-speaker speech tracking", Tencent (Rhino-Bird funding scheme). (Surrey investigator: Wenwu Wang) [Industry partner: Yong Xu @ Tencent AI Lab]
- 01/2020-10/2022, PI, "Deep embedding techniques for audio scene analysis and source separation", ASEM-DUO (Duo-India Professor Fellowship). [jointly with Dr Vipul Arora at Indian Institute of Technology (IIT) Kanpur] (Surrey investigator: Wenwu Wang).
03/2017-01/2023, CI, "Audio Visual Media Research", EPSRC (Platform grant). [Surrey investigators: PI: Adrian Hilton, CIs: Mark Plumbley, Josef Kittler, Wenwu Wang, John Collomosse, Philip Jackson, and Jean-Yves Guillemaut.]
------
- 08/2020-05/2021, PI, "Audio tagging for meta data generation of media for programme recommendation", EPSRC (impact acceleration account). (Surrey investigators: Wenwu Wang (PI) and Mark Plumbley (CI))
- 09/2018-03/2019, PI, "Array optimisation with sensor failure", EPSRC (impact acceleration account). [jointly with Kaon] [Surrey investigators: Wenwu Wang.]
- 02/2018-12/2018, PI, "Speech detection, separation and localisation with acoustic vector sensor", Huawei (HIRP). [Surrey investigators: Wenwu Wang.]
- 01/2017-01/2020, PI, "Improving the Robustness of UWAN Data Transmitting and Receiving Utilize Deep Learning and Statistical Model", NSFC (Youth Science Foundation). [Surrey investigators: Wenwu Wang.]
- 02/2016-02/2019, CI, "ACE-CReAte: Audio Commons", EC (Horizon 2020). [jointly wih Universitat Pompeu Fabra, Queen Mary University of London, Jamendo SA, AudioGaming, and Waves Audio Ltd.] [Surrey investigators: PI: Mark Plumbley, CIs: Wenwu Wang, Tim Brookes, and David Plans.] (project website)
- 02/2016-02/2019, PI, "Marine environment surveillance technology based on underwater acoustic signal processing", SSTIC ('international collaboration' call). [jointly wih Harbin Institute of Technology at Shenzhen] [Surrey investigators: Wenwu Wang.]
- 01/2016-01/2019, CI, "Making sense of sounds", EPSRC ('making sense from data' call). [jointly wih Salford University] [Surrey investigators: Mark Plumbley (PI), CIs: Wenwu Wang, Philip Jackson and David Frohlich.] (project website)
- 01/2015-01/2019, CI, "MacSeNet: machine sensing training network", EC (Horizon 2020, Marie Curie Actions - Innovative Training Network). [jointly with INRIA (France), University of Edinburgh (UK), Technical University of Muenchen (Germany), EPFL (Switzerland), Computer Technology Institute (Greece), Institute of Telecommunications (Portugal), Tampere University of Technology (Finland), Fraunhofer IDMT (Germany), Cedar Audio Ltd (Cambridge, UK), Audio Analytic (Cambridge, UK), VisioSafe SA (Switzerland), and Noiseless Imaging Oy (Finland)] [Surrey investigators: Mark Plumbley (PI) and Wenwu Wang (CI)] (project website)
- 10/2014-10/2018, CI, "SpaRTaN: Sparse representation and compressed sensing training network", EC (FP7, Marie Curie Actions - Initial Training Network). [jointly with University of Edinburgh (UK), EPFL (Switzerland), Institute of Telecommunications (Portugal), INRIA (France), VisioSafe SA (Switzerland), Noiseless Imaging Oy (Finland), Tampere University of Technology (Finland), Cedar Audio Ltd (Cambridge, UK), and Fraunhofer IDMT (Germany)] [Surrey investigators: Mark Plumbley (PI) and Wenwu Wang (CI).] (project website)
- 01/2014-01/2019, CI, "S3A: future spatial audio for an immersive listener experience at home", EPSRC (programme grant). [jointly with University of Southampton, University of Salford, and BBC.] [Surrey investigators: PI: Adrian Hilton, CIs: Philip Jackson, Wenwu Wang, Tim Brookes, and Russell Mason.] (project website)
- 04/2013-06/2018, PI, "Signal processing solutions for a networked battlespace", EPSRC and Dstl ('signal processing' call). [jointly with Loughborough University, University of Strathclyde, and Cardiff University.] [Surrey investigators: Wenwu Wang (PI), Josef Kittler (CI), and Philip Jackson (CI)] (project website)
- 09/2015-06/2016, PI, "Array processing exploiting sparsity for submarine hull mounted arrays", Atlas Electronik & MoD (MarCE scheme) [Surrey investigators: Wenwu Wang.]
- 03/2015-09/2015, PI, "Speech enhancement based on lip tracking", EPSRC (impact acceleration account). [jointly with SAMSUNG (UK)] [Surrey investigators: Wenwu Wang.]
- 10/2013-03/2014, PI, "Enhancing speech quality using lip tracking", SAMSUNG (industrial grant). [Surrey investigators: Wenwu Wang.]
- 12/2012-12/2013, PI, "Audio-visual cues based attention switching for machine listening", MILES and EPSRC (feasibility study). [jointly with School of Psychology and Department of Computing.] [Surrey investigators: PI: Wenwu Wang, CIs: Mandeep Dhami, Shujun Li, and Anthony Ho.]
- 11/2012-07/2013, PI, "Audio-visual blind source separation", NSFC (international collaboration scheme). [jointly with Nanchang University, China.] [Surrey investigators: Wenwu Wang.]
- 12/2011-03/2012, PI, "Enhancement of audio using video", HO (pathway to impact). [jointly with University of East Anglia.] [Surrey investigators: Wenwu Wang and Richard Bowden (CI).]
- 10/2010-10/2013, CI, "Audio and video based speech separation for multiple moving sources within a room environment", EPSRC (responsive mode). [jointly with Loughborough University.] [Surrey investigators: Josef Kittler (PI) and Wenwu Wang (CI).]
- 10/2009-10/2012, PI, "Multimodal blind source separation for robot audition", EPSRC and Dstl ('signal processing' call). [Surrey investigators: PI: Wenwu Wang, CIs: Josef Kittler and Philip Jackson.] (project website)
- 05/2008-06/2008, PI, "Convolutive non-negative sparse coding", RAENG (international travel grant).[Surrey investigators: Wang.]
- 02/2008-06/2008, PI, "Convolutive non-negative matrix factorization", URSF (small grant). [Surrey investigators: Wang.]
- 02/2008-03/2008, PI, "Computational audition", OSU (visiting scholarship). [Surrey investigators: Wang.] (Collaborator: Prof Deliang Wang)
Research interests
- Unsupervised learning techniques (including independent component analysis, independent vector analysis, latent variable analysis, sparse component analysis, non-negative matrix/tensor factorisation, low-rank representation, manifold learning, and subspace clustering)
- Supervised learning techniques (including deep learning, dictionary learning, multimodal learning, and learning with priors and signal properties)
- Computational auditory scene analysis (audio scene recognition, audio event detection, audio tagging, and audio captioning)
- Audio signal separation (convolutive audio source separation, underdetermined audio source separation including monaural source separation)
- Audio feature extraction and perception (including pitch detection, onset detection, rhythm detection, music transcription and low bit-rate audio coding)
- Sound source localisation (using audio, video, depth information, with particle filtering, PHD filtering, and/or particle flow filtering)
- Multimodal speech source separation (audio/visual source separation with modelled based techniques such as Gaussian mixture model and learning-based method such as audio-visual dictionary learning)
- Sparse representation and compressed sensing (synthesis model and analysis model based dictionary learning for sparse represenation, with applications to audio source separation, speech enhancement, audio inpainiting, and image enhancement)
- Cocktail party processing (using techniques such as independent component analysis, blind source separation, computational auditory scene analysis, sparse representation/dictionary learning, Gaussian mixture modelling and expectation maximisation, and multimodal fusion)
- Non-negative sparse coding of audio signals (including sparsity constrained non-negative matrix factorisation for audio analysis)
- 3D positional audio technology (including head-related transfer functions, binaural modelling, multiple loudspeaker panning, and room geometry estimation)
- Approximate joint diagonalization for source separation (including unitary or non-unitary constrained joint diagonalization approaches)
- Robust solutions for permutation problem of frequency domain independent component analysis (including approaches using filter constraints, statistical characteristics of signals, and beamforming)
- Convex and non-convex optimisation (gradient descent, Newton methods, interior point method, ADMM, etc.)
- Psychoacoustics motivationed signal processing and machine learning methods (e.g. time-frequency masking, perceptually informed speech separation/enhancement, intelligibility adaptive speech separation algorithms)
Funded Projects (Grants)
I appreciate the financial support for my research from the following bodies (since 2008): Engineering and Physical Science Research Council (EPSRC), Ministry of Defence (MoD), Defence Science and Technology Laboratory (Dstl), Department of Defence (DoD), Home Office (HO), Royal Academy of Engineering (RAENG), European Commission (EC), National Natural Science Foundation of China (NSFC), Shenzhen Science and Technology Innovation Council (SSTIC) of China, the University Research Support Fund (URSF), and the Ohio State University (OSU), and UK/EU industries including BBC, Atlas, Kaon, Huawei, Tencent, NPL, and Samsung. [Total award to Surrey where I am a Principal Investigator (PI) or Co-Investigator (CI): £13M+ (as PI £2.2M, as CI £14M+). As PI/CI, on a total grant award portfolio: £30M+]
- 04/2022-04/2026, PI, "Uncertainty modelling and quantification for heterogeneous sensor/effector networks", SAAB (Surrey investigators: Wenwu Wang (PI), Pei Xiao (CI)) Project partner: SAAB.
- 04/2022-04/2026, PI, "Cooperative sensor fusion and management for distributed sensor swarms", SAAB (Surrey investigators: Wenwu Wang (PI), Pei Xiao (CI)) Project partner: SAAB.
- 01/2022-01/2026, CI, "Differentiable particle filters for data-driven sequential inference", EPSRC & NPL (iCASE). (Surrey investigators: Yunpeng Li (PI), Wenwu Wang (CI)) Project partner: National Physical Laboratory.
- 07/2021-07/2026, CI, "BBC Prosperity Partnership: AI for Future Personalised Media Experiences", EPSRC (Prosperity Partnership scheme). (Surrey investigators: Adrian Hilton (PI, project lead), CIs: Philip Jackson, Armin Mustafa, Jean-Yves Guillemaut, Marco Volina, and Wenwu Wang; project manager: Elizabeth James ) [The project is led by University of Surrey, jointly with BBC and University of Lancaster, with supports from 10+ industrial partners.] (project website)
- 07/2021-07/2024, CI, "Uncertainty Quantification for Robust AI through Optimal Transport", University of Surrey (project-based Doctoral College studentship competition). (Surrey investigators: Yunpeng Li (PI), CI: Wenwu Wang.
- 02/2021-02/2023, PI, "Automated Captioning of Image and Audio for Visually and Hearing Impaired", British Council (Newton Institutional Links Award). (Surrey investigators: Wenwu Wang) [The project is led by University of Surrey, jointly with Izmir Katip Celebi University (IKCU) @ Turkey (Volkan Kilic).]
- 04/2021-04/2024, CI, "Multimodal video search by examples", EPSRC (responsive mode). (Surrey investigators: PI: Josef Kittler, CIs: Miroslaw Bober, Wenwu Wang and Mark Plumbley) [The project is led by Ulster University (Hui Wang), jointly with Univ. of Surrey and Univ of Cambridge (Mark Gales).]
- 01/2021-10/2022, PI, "Acoustic surveillance", MoD (DASA call on countering drones). (Surrey investigators: Wenwu Wang) [The project is led by Airspeed Electronics.]
- 11/2020-11/2023, PI, "SIGNetS: signal and information gathering for networked surveillance", DoD & MoD (UDRC phase 3 call on the application theme Signal and Information Processing for Decentralized Intelligence, Surveillance, and Reconnaissance). (Surrey investigators: Wenwu Wang (PI) and Pei Xiao (CI)) [The project is a collaboration between University of Cambridge (Simon Godsill, project lead), University of Surrey, and University of Sheffield (Lyudmila Mihaylova).] (project website)
- 08/2020-08/2023, PI, "Particle flow PHD filtering for audio-visual multi-speaker speech tracking", Tencent (Rhino-Bird funding scheme). (Surrey investigator: Wenwu Wang) [Industry partner: Yong Xu @ Tencent AI Lab]
- 01/2020-10/2022, PI, "Deep embedding techniques for audio scene analysis and source separation", ASEM-DUO (Duo-India Professor Fellowship). [jointly with Dr Vipul Arora at Indian Institute of Technology (IIT) Kanpur] (Surrey investigator: Wenwu Wang).
03/2017-01/2023, CI, "Audio Visual Media Research", EPSRC (Platform grant). [Surrey investigators: PI: Adrian Hilton, CIs: Mark Plumbley, Josef Kittler, Wenwu Wang, John Collomosse, Philip Jackson, and Jean-Yves Guillemaut.]
------
- 08/2020-05/2021, PI, "Audio tagging for meta data generation of media for programme recommendation", EPSRC (impact acceleration account). (Surrey investigators: Wenwu Wang (PI) and Mark Plumbley (CI))
- 09/2018-03/2019, PI, "Array optimisation with sensor failure", EPSRC (impact acceleration account). [jointly with Kaon] [Surrey investigators: Wenwu Wang.]
- 02/2018-12/2018, PI, "Speech detection, separation and localisation with acoustic vector sensor", Huawei (HIRP). [Surrey investigators: Wenwu Wang.]
- 01/2017-01/2020, PI, "Improving the Robustness of UWAN Data Transmitting and Receiving Utilize Deep Learning and Statistical Model", NSFC (Youth Science Foundation). [Surrey investigators: Wenwu Wang.]
- 02/2016-02/2019, CI, "ACE-CReAte: Audio Commons", EC (Horizon 2020). [jointly wih Universitat Pompeu Fabra, Queen Mary University of London, Jamendo SA, AudioGaming, and Waves Audio Ltd.] [Surrey investigators: PI: Mark Plumbley, CIs: Wenwu Wang, Tim Brookes, and David Plans.] (project website)
- 02/2016-02/2019, PI, "Marine environment surveillance technology based on underwater acoustic signal processing", SSTIC ('international collaboration' call). [jointly wih Harbin Institute of Technology at Shenzhen] [Surrey investigators: Wenwu Wang.]
- 01/2016-01/2019, CI, "Making sense of sounds", EPSRC ('making sense from data' call). [jointly wih Salford University] [Surrey investigators: Mark Plumbley (PI), CIs: Wenwu Wang, Philip Jackson and David Frohlich.] (project website)
- 01/2015-01/2019, CI, "MacSeNet: machine sensing training network", EC (Horizon 2020, Marie Curie Actions - Innovative Training Network). [jointly with INRIA (France), University of Edinburgh (UK), Technical University of Muenchen (Germany), EPFL (Switzerland), Computer Technology Institute (Greece), Institute of Telecommunications (Portugal), Tampere University of Technology (Finland), Fraunhofer IDMT (Germany), Cedar Audio Ltd (Cambridge, UK), Audio Analytic (Cambridge, UK), VisioSafe SA (Switzerland), and Noiseless Imaging Oy (Finland)] [Surrey investigators: Mark Plumbley (PI) and Wenwu Wang (CI)] (project website)
- 10/2014-10/2018, CI, "SpaRTaN: Sparse representation and compressed sensing training network", EC (FP7, Marie Curie Actions - Initial Training Network). [jointly with University of Edinburgh (UK), EPFL (Switzerland), Institute of Telecommunications (Portugal), INRIA (France), VisioSafe SA (Switzerland), Noiseless Imaging Oy (Finland), Tampere University of Technology (Finland), Cedar Audio Ltd (Cambridge, UK), and Fraunhofer IDMT (Germany)] [Surrey investigators: Mark Plumbley (PI) and Wenwu Wang (CI).] (project website)
- 01/2014-01/2019, CI, "S3A: future spatial audio for an immersive listener experience at home", EPSRC (programme grant). [jointly with University of Southampton, University of Salford, and BBC.] [Surrey investigators: PI: Adrian Hilton, CIs: Philip Jackson, Wenwu Wang, Tim Brookes, and Russell Mason.] (project website)
- 04/2013-06/2018, PI, "Signal processing solutions for a networked battlespace", EPSRC and Dstl ('signal processing' call). [jointly with Loughborough University, University of Strathclyde, and Cardiff University.] [Surrey investigators: Wenwu Wang (PI), Josef Kittler (CI), and Philip Jackson (CI)] (project website)
- 09/2015-06/2016, PI, "Array processing exploiting sparsity for submarine hull mounted arrays", Atlas Electronik & MoD (MarCE scheme) [Surrey investigators: Wenwu Wang.]
- 03/2015-09/2015, PI, "Speech enhancement based on lip tracking", EPSRC (impact acceleration account). [jointly with SAMSUNG (UK)] [Surrey investigators: Wenwu Wang.]
- 10/2013-03/2014, PI, "Enhancing speech quality using lip tracking", SAMSUNG (industrial grant). [Surrey investigators: Wenwu Wang.]
- 12/2012-12/2013, PI, "Audio-visual cues based attention switching for machine listening", MILES and EPSRC (feasibility study). [jointly with School of Psychology and Department of Computing.] [Surrey investigators: PI: Wenwu Wang, CIs: Mandeep Dhami, Shujun Li, and Anthony Ho.]
- 11/2012-07/2013, PI, "Audio-visual blind source separation", NSFC (international collaboration scheme). [jointly with Nanchang University, China.] [Surrey investigators: Wenwu Wang.]
- 12/2011-03/2012, PI, "Enhancement of audio using video", HO (pathway to impact). [jointly with University of East Anglia.] [Surrey investigators: Wenwu Wang and Richard Bowden (CI).]
- 10/2010-10/2013, CI, "Audio and video based speech separation for multiple moving sources within a room environment", EPSRC (responsive mode). [jointly with Loughborough University.] [Surrey investigators: Josef Kittler (PI) and Wenwu Wang (CI).]
- 10/2009-10/2012, PI, "Multimodal blind source separation for robot audition", EPSRC and Dstl ('signal processing' call). [Surrey investigators: PI: Wenwu Wang, CIs: Josef Kittler and Philip Jackson.] (project website)
- 05/2008-06/2008, PI, "Convolutive non-negative sparse coding", RAENG (international travel grant).[Surrey investigators: Wang.]
- 02/2008-06/2008, PI, "Convolutive non-negative matrix factorization", URSF (small grant). [Surrey investigators: Wang.]
- 02/2008-03/2008, PI, "Computational audition", OSU (visiting scholarship). [Surrey investigators: Wang.] (Collaborator: Prof Deliang Wang)
Supervision
Completed postgraduate research projects I have supervised
Postdoc research fellows
- Dr Jianyuan Sun (09/2021 -): Deep learning for audio classfication and captioning
- Dr Syed Ahmad Soleymani (01/2023 -): Sensor fusion with autonomous sensor management
- Dr Shidrokh Goudarzi (09/2021 -01/2023): Q-learning for autonomous sensor management
- Dr Saeid Safavi (02/2021-07/2022): Machine learning for audio detection and localization
- Dr Gishantha Thantulage (09/2021 - 03/2022): Machine learning (Co-supervisor. Co-supervised with Prof Anil Fernando)
- Dr Oluwatobi Baiyekusi (09/2021 - 03/2022): Deep learning for media content analysis (Co-supervisor. Co-supervised with Prof Anil Fernando)
- Dr Tassadaq Hussain (03/2021 - 07/2021): Audio tagging for program recommendation (Main supervisor. Co-supervised with Prof Mark Plumbley)
- Dr Lam Pham (08/2020 - 02/2021): Audio tagging for meta data generation for program recommendation (Main supervisor. Co-supervised with Prof Mark Plumbley)
- Dr Yin Cao (09/2018 - 12/2020): Audio scene classification, event detection and audio tagging (Main supervisor. Co-supervised with Prof Mark Plumbley)
- Dr Saeid Safavi (03/2018 - 06/2020): Machine learning for predicting perceptual reverberation (Main supervisor. Co-supervised with Prof Mark Plumbley)
- Dr Mark Barnard (08/2018 - 03/2019): Array optimisation with sensor failure
- Dr Qingju Liu (04/2014 - 05/2019): Source separation and objectification for future spatial audio (Primary supervisor. Co-supervised with Dr Philip Jackson and Prof Adrian Hilton)
- Dr Cemre Zor (04/2013 - 02/2019): Statistical anomaly detection (Primary supervisor. Co-supervised with Prof Josef Kittler)
- Dr Qiang Huang (04/2016 - 10/2018): Semantic Audio-Visual Processing and Interaction (Co-supervisor. Co-supervised with Dr Philip Jackson and Prof Mark Plumbley)
- Dr Yong Xu (04/2016 - 05/2018): Machine Listening (Main supervisor. Co-supervised with Prof Mark Plumbley and Dr Philip Jackson)
- Dr Viet Hung Tran (08/2017 - 06/2018): Acoustic source localisation and separation
- Dr Mark Barnard (09/2014 - 08/2018): Underwater acoustic signal processing (major) / Visual tracking for future spatial audio (minor) (Main supervisor. Co-supervised with Prof Adrian Hilton and Dr Philip Jackson)
- Dr Lu Ge (03/2015 - 09/2015): Audio-visual signal processing
- Dr Swati Chandna (05/2013 - 11/2014): Bootstrapping for robust source separation (Primary supervisor. Co-supervised with Dr Philip Jackson)
- Dr Mark Barnard (10/2010 - 12/2013): Audio-visual speech separation of multiple moving sources (Primary supervisor. Co-supervised with Prof Josef Kittler. External Collaborators: Prof Jonathon Chambers, Loughborough University; Dr Sangarapillai Lambotharan, Loughborough University; Prof Christian Jutten, Grenoble, France, and Dr Rivet Bertrand, Grenoble, France)
- Dr Qingju Liu (01/2013 - 03/2014): Words spotting from noisy mixtures & Lip-tracking for voice enhancement
PhD students
- Xinran Liu: Cross-modality generation(Co-supervisor. Co-supervised with Dr Zhenhua Feng)
- John-Joseph Brady: Differentiable particle filtering (Co-supervisor. Co-supervised with Dr Yunpeng Li)
- Zhi Qin Tan: Bayesian machine learning (Co-supervisor. Co-supervised with Dr Yunpeng Li)
- Junqi Zhao: Audio restoration with generative models (Primary supervisor. Co-supervised with Prof Mark Plumbley)
- Haojie Chang: Audio-visual analysis of fish behaviour (Primary supervisor. Co-supervised with Dr Lian Liu from CPE Department)
- Jiaxi Li: Statistical machine learning (Co-supervisor. Co-supervised with Dr Yunpeng Li)
- Sindhu Vasireddy: Distributed fusion of heterogeneous sensory data (Primary supervisor. Co-supervised with Prof Pei Xiao, 5G/6G Innovation Centre)
- Yi Yuan: Deep learning for intelligent sound generation (Primary supervisor. Co-supervised with Prof Mark Plumbley)
- Yaru Chen: Multimodal learning and analysis of fish behaviour (Primary supervisor. Co-supervised with Prof Tao Chen from CPE Department)
- Haohe Liu: Audio tagging (Co-supervisor. Co-supervised with Prof Mark Plumbley)
- Meng Cui: Machine learning for multimodal analysis of fish behaviour (Primary supervisor. Co-supervised with Prof Guoping Lian from Unilever/CPE and Prof Tao Chen from CPE Department)
- Yanze Xu: Recognition of paralinguistic features for singing voice description (Co-supervisor. Co-supervised with Prof Mark Plumbley)
- James King: Information theoretic learning for sound analysis (Co-supervisor. Co-supervised with Prof Mark Plumbley)
- Jinzheng Zhao: Audio-visual multi-speaker tracking (Primary supervisor. Co-supervised with Prof Mark Plumbley, and Dr Yong Xu (Tencent AI Lab, USA)
- Xinhao Mei: Automated audio captioning (Primary supervisor. Co-supervised with Dr Yunpeng Li)
- Xubo Liu: Automated translations between audio and texts (Primary supervisor. Co-supervised with Prof Mark Plumbley)
- Peipei Wu: Multimodal multi-target tracking (Primary supervisor. Co-supervised with Dr Philip Jackson)
- Andrew Bailey: Multimodal signal processing (Co-supervisor. Co-supervised with Prof Mark Plumbley)
- Buddhiprabha Erabadda: Advanced video coding (Co-supervisor. Co-supervised with Prof Anil Fernando)
------
- Mukunthan Tharmakulasingam (PhD defended in April 2023): Interpretable Machine Learning Models to Predict Antimicrobial Resistance (Co-supervisor. Co-supervised with Prof Anil Fernando and Prof Roberto La Ragione)
- Jingshu Zhang (PhD awarded in December 2022): Phase Aware Speech Enhancement and Dereverberation (Co-supervisor. Co-supervised with Prof Mark Plumbley)
- Shuoyang Li (PhD awarded in June 2022): Sketching and Streaming based Subspace Clustering for Large-scale Data Classification (Primary supervisor. Co-supervised with Dr Philip Jackson, and Dr Yuantao Gu from Tsinghua University, China)
- Jayasingam Adhuran (PhD awarded in April 2022): QoE Aware VVC Based Omnidirectional and Screen Content Coding (Co-supervisor. Co-supervised with Prof Anil Fernando)
- Turab Iqbal (PhD awarded in December 2021): Noisy Web Supervision for Audio Classification (Primary supervisor. Co-supervised with Prof Mark Plumbley)
- Lucas Rencker (PhD awarded in August 2020): Sparse Signal Recovery From Linear and Nonlinear Compressive Measurements (Primary supervisor. Co-supervised with Prof Mark Plumbley, and Prof Francis Bach, INRIA, France) [Lucas is a Marie Curie Early Stage Researcher]
- Iwona Sobieraj (PhD awarded in June 2020): Environmental Audio Analysis by Non-negative Matrix Factorization (Co-supervisor. Co-supervised with Prof Mark Plumbley) [Iwona is a Marie Curie Early Stage Researcher]
- Alfredo Zermini (PhD awarded in April 2020): Deep learning for speech separation (Primary supervisor. Co-supervised with Prof Mark Plumbley, and Prof Francis Bach, INRIA, France) [Alfredo is a Marie Curie Early Stage Researcher]
- Yang Liu (PhD awarded in February 2020): Particle Flow PHD Filtering for Audio-Visual Multi-Speaker Tracking (Primary supervisor. Co-supervised with Prof Adrian Hilton)
- Hanne Stenzel (PhD awarded in December 2019): Influences on perceived horizontal audio-visual spatial alignment (Co-supervisor. Co-supervised with Dr Philip Jackson)
- Cian O'Brien (PhD awarded in November 2019): Low rank modelling for polyphonic music analysis (Co-supervisor. Co-supervised with Prof Mark Plumbley) [Cian is a Marie Curie Early Stage Researcher]
- Qiuqiang Kong (PhD awarded in September 2019): Sound event detection with weakly labelled data (Co-supervisor. Co-supervised with Prof Mark Plumbley)
- Luca Remaggi (PhD awarded in August 2017): Estimation of Room Reflection Parameters for a Reverberant Spatial Audio Object (Co-supervisor. Co-supervised with Dr Philip Jackson)
- Pengming Feng (PhD awarded in November 2016): Enhanced particle PHD filtering for multiple human tracking (Co-supervisor. Co-supervised with Prof Jonathon Chambers and Dr Syed Mohsen Naqvi, Newcastle University)
- Atiyeh Alinaghi (PhD awarded in October 2016): Blind convolutive stereo speech separation and dereverberation (Co-supervisor. Co-supervised with Dr Philip Jackson)
- Jing Dong (PhD awarded in July 2016): Sparse Analysis Model Based Dictionary Learning and Signal Reconstruction (Primary supervisor. Co-supervised with Dr Philip Jackson; External Collaborator: Dr Wei Dai, Imperial College London)
- Shahrzad Shapoori (PhD awarded in April 2016): Detection of medial temporal brain discharges from EEG signals using joint source separation-dictionary learning (Co-supervisor. Co-supervised with Dr Saeid Sanei, Department of Computing)
- Volkan Kilic (PhD awarded in January 2016): Audio visual tracking of multiple moving sources (Primary supervisor. Co-supervised with Prof Josef Kittler and Dr Mark Barnard)
- Marek Olik (PhD awarded in January 2015): Personal sound zone reproducation with room reflections (Co-supervisor. Co-supervised with Dr Philip Jackson)
- Syed Zubair (PhD awarded in June 2014): Dictionary learning for signal classification (Primary supervisor. Co-supervised with Dr Philip Jackson; Internal collaborator: Dr Fei Yan; External collaborator: Dr Wei Dai, Imperial College London)
- Philip Coleman (PhD awarded in May 2014): Loudspeaker array processing for personal sound zone reproduction (Co-supervisor. Co-supervised with Dr Philip Jackson)
- Qingju Liu (PhD awarded in October 2013): Multimodal blind source separation for robot audition (Primary supervisor. Co-supervised with Dr Philip Jackson, Prof Josef Kittler; External collaborator: Prof Jonathon Chambers, Loughborough University)
- Tao Xu (PhD awarded in June 2013): Dictionary learning for sparse representations with applications to blind source separation (Primary supervisor. Co-supervised with Dr Philip Jackson; External collaborator: Dr Wei Dai, Imperial College London)
- Rakkrit Duangsoithong (PhD awarded in Oct 2012): Feature selection and causal discovery for ensemble classifiers (Co-supervisor; Co-supervised with Dr Terry Windeatt)
- Tariqullah Jan (PhD awarded in Feb 2012): Blind convolutive speech separation and dereverberation (Primary Supervisor; Co-Supervised with Prof Josef Kittler; External collaborator: Prof DeLiang Wang, The Ohio State University)
Academic visitors
- Mr Xuenan Xu (09/2023-): PhD Student, Shanghai Jiaotong University, China. Topic: Audio captioning.</li> (Co-supervisor. Jointly supervised with Prof Mark Plumbley)
- Mr Jinhua Liang (11/2022-): PhD Student, Queen Mary University of London, UK. Topic: Audio classification.
------
- Dr Vipul Arora (09/2022 - ): Associate Professor, Indian Institute of Technology, India. Topic: Audio source separation and scene analysis.
- Mr Bin Lin (08/2019 - 08/2020): Senior Research Engineer, China Academy of Space Technology, China. Topic: Sparse analysis model based dictionary learning from nonlinear measurements.
- Dr Takahiro Murakami (04/2019 - 04/2020): Assistant Professor, Meiji University, Japan. Topic: Microphone array calibration.
- Dr Shiyong Lan (10/2018 -10/2019): Associate Professor, Sichuan University, Chengdu, China. Topic: Multimodal tracking.
- Prof Jinjia Wang (07/2017 - 07/2018): Professor, Yanshan University, Qinghuangdao, China. Topic: Deep sparse learning.
- Dr Ning Li (08/2017 - 08/2018): Associate Professor, Harbin Engineering University, Harbin, China. Topic: Blind sparse inverse filtering and deconvolution.
- Dr Yang Chen (08/2017 - 08/2018): Associate Professor, Changzhou University, China. Topic: Acoustic source localisation and separation.
- Dr Yina Guo (09/2016 - 02/2017): Associate Professor, Taiyuan University of Science and Technology, Taiyuan, China. Topic: Blind source separation.
- Dr Ronan Hamon (06/2016-11/2016), Postdoctoral Researcher, QARMA Team (LIF - Aix-Marseille Universite), France. Topic: Perceptual and objective measure of musical noise in audio source separation & audio impainting. (Co-supervisor. Co-supervised with Prof Mark Plumbley.)
- Dr Zongxia Xie (01/2016 - 01/2017): Associate Professor, Tianjin University, Tianjin, China. Topic: Sparse representation for big uncertain data classification.
- Mr Jian Guan (10/2014 - 01/2017): PhD student, Harbin Institute of Techonology, Shenzhen Graduate School, Shenzhen, China. Topic: Blind sparse deconvolution and dereverberation.
- Dr Jesper Rindom Jensen (04/2016 - 04/2016): Postdoctoral Research Fellow, Aalborg University, Denmark. Topic: Audio-visual speech processing.
- Dr Xiaorong Shen (02/2015 - 12/2015): Associate Professor, Beihang University, Beijing, China. Topic: Audio-visual source detection, localization and tracking.
- Mr Luc Guy (06/2015 - 09/2015): MSc student, Polytech Montpellier, France. Topic: Music audio source separation.
- Mr Hatem Deif (02/2015 - 02/2015): PhD student, Brunel University, London, UK. Topic: Single channel audio source separation.
- Dr Yang Yu (04/2014 - 04/2015): Associate Professor, Northwestern Polytechnical University, Xi'an, China. Topic: Underwater acoustic source localisation and tracking with sparse array and deep learning.
- Mr Jamie Corr (10/2014 - 10/2014): PhD student, Strathclyde Univeristy, Glasgow, UK. Topic: Underwater acoustic data processing with polynomial matrix decomposition.
- Dr Xionghu Zhong (07/2014 - 07/2014): Independent Research Fellow, Nanyang Technological University, Singapore. Topic: Acoustic source tracking.
- Xiaoyi Chen (10/2012 - 09/2013 ): PhD student, Northwestern Polytechnical University, Xi'an, China. Topic: Convolutive blind source separation of underwater acoustic mixtures.
- Dr Ye Zhang (12/2012 - 08/2013): Associate Professor, Nanchang University, Nanchang, China. Topic: Analysis dictionary learning and source separation.
- Victor Popa (04/2013 - 07/2013), PhD student, University Politehnica of Bucharest, Bucharest, Romania. Topic: Audio source separation.
- Dr Stefan Soltuz (10/2008 -07/2009), Research Scientist, Tiberiu Popoviciu Institute of Numerical Analysis, Romania. Topic: Non-negative matrix factorization for music audio separation (Primary supervisor. Co-supervised with Dr Philip Jackson)
- Yanfeng Liang (MSc, 05/2009), MSc Student: Harbin Engineering University, Harbin, China. Topic: Adaptive signal processing for clutter removal in radar images (Co-supervisor. Co-supervised with Prof Jonathon Chambers, Loughborough University)
Publications
Fish feeding intensity assessment (FFIA) aims to evaluate fish appetite changes during feeding, which is crucial in industrial aquaculture applications. Existing FFIA methods are limited by their robustness to noise, computational complexity, and the lack of public datasets for developing the models. To address these issues, we first introduce AV-FFIA, a new dataset containing 27,000 labeled audio and video clips that capture different levels of fish feeding intensity. Then, we introduce multi-modal approaches for FFIA by leveraging the models pre-trained on individual modalities and fused with data fusion methods. We perform benchmark studies of these methods on AV-FFIA, and demonstrate the advantages of the multi-modal approach over the single-modality based approach, especially in noisy environments. However, compared to the methods developed for individual modalities, the multimodal approaches may involve higher computational costs due to the need for independent encoders for each modality. To overcome this issue, we further present a novel unified mixed-modality based method for FFIA, termed as U-FFIA. U-FFIA is a single model capable of processing audio, visual, or audio-visual modalities, by leveraging modality dropout during training and knowledge distillation using the models pre-trained with data from single modality. We demonstrate that U-FFIA can achieve performance better than or on par with the state-of-the-art modality-specific FFIA models, with significantly lower computational overhead, enabling robust and efficient FFIA for improved aquaculture management. To encourage further research, we have released the AV-FFIA dataset, the pre-trained model and codes at https://github.com/FishMaster93/U-FFIA. Note to Practitioners-Feeding is one of the most important costs in aquaculture. However, current feeding machines usually operate with fixed thresholds or human experiences, lacking the ability to automatically adjust to fish feeding intensity. FFIA can evaluate the intensity changes in fish appetite during the feeding process and optimize the control strategies of the feeding machine to avoid inadequate feeding or overfeeding, thereby reducing the feeding cost and improving the well-being of fish in industrial aquaculture. The existing methods have mainly exploited single-modality data, and have a high sensitivity to input noise. Using video and audio offers improved chances to address the challenges brought by various environments. However, compared with processing data from single modalities, using multiple modalities simultaneously often involves increased computational resources, including memory, processing power, and storage. This can impact system performance and scalability. To address these issues, we focus on the efficient unified model, which is capable of processing both multimodal and single-modal input. Our proposed model achieved state-of-the-art (SOTA) performance in FFIA with high computational efficiency.
Digital aquaculture leverages advanced technologies and data‐driven methods, providing substantial benefits over traditional aquaculture practices. This article presents a comprehensive review of three interconnected digital aquaculture tasks, namely, fish tracking, counting, and behaviour analysis, using a novel and unified approach. Unlike previous reviews which focused on single modalities or individual tasks, we analyse vision‐based (i.e., image‐ and video‐based), acoustic‐based, and biosensor‐based methods across all three tasks. We examine their advantages, limitations, and applications, highlighting recent advancements and identifying critical cross‐cutting research gaps. The review also includes emerging ideas such as applying multitask learning and large language models to address various aspects of fish monitoring, an approach not previously explored in aquaculture literature. We identify the major obstacles hindering research progress in this field, including the scarcity of comprehensive fish datasets and the lack of unified evaluation standards. To overcome the current limitations, we explore the potential of using emerging technologies such as multimodal data fusion and deep learning to improve the accuracy, robustness, and efficiency of integrated fish monitoring systems. In addition, we provide a summary of existing datasets available for fish tracking, counting, and behaviour analysis. This holistic perspective offers a roadmap for future research, emphasizing the need for comprehensive datasets and evaluation standards to facilitate meaningful comparisons between technologies and to promote their practical implementations in real‐world settings.
Language-queried audio source separation (LASS) is a new paradigm for computational auditory scene analysis (CASA). LASS aims to separate a target sound from an audio mixture given a natural language query, which provides a natural and scalable interface for digital audio applications. Recent works on LASS, despite attaining promising separation performance on specific sources (e.g., musical instruments, limited classes of audio events), are unable to separate audio concepts in the open domain. In this work, we introduce AudioSep, a foundation model for open-domain audio source separation with natural language queries. We train AudioSep on large-scale multimodal datasets and extensively evaluate its capabilities on numerous tasks including audio event separation, musical instrument separation, and speech enhancement. AudioSep demonstrates strong separation performance and impressive zero-shot generalization ability using audio captions or text labels as queries, substantially outperforming previous audio-queried and language-queried sound separation models. Specifically, AudioSep achieved strong results including a Signal-to-Distortion Ratio Improvement (SDRi) of 7.74 dB across 527 sound classes of the AudioSet; 9.14 dB on the VGGSound dataset; 8.22 dB on the AudioCaps dataset; 6.85 dB on the Clotho dataset; 10.51 dB on the MUSIC dataset; 10.04 dB on the ESC-50 dataset; 8.16 dB on the DCASE 2024 Task 9 dataset; and an SSNR of 9.21 dB on the VoicebankDemand dataset.
The auditory system plays a substantial role in shaping the overall human perceptual experience. While prevailing large language models (LLMs) and visual language models (VLMs) have shown their promise in solving a wide variety of language and vision understanding tasks, only a few of them can be generalised to the audio domain without compromising their domain-specific capability. In this work, we introduce Acoustic Prompt Tuning (APT), a new adapter extending LLMs and VLMs to the audio domain by injecting audio embeddings to the input of LLMs, namely soft prompting. Specifically, APT applies an instruction-aware audio aligner to generate soft prompts, conditioned on both input text and sounds, as the inputs to the language model. To mitigate data scarcity in the audio domain, a curriculum learning strategy is proposed by formulating diverse audio tasks in a sequential manner. Moreover, we improve the audio language model by using interleaved audio-text embeddings as the input sequence. In this improved model, zero constraints are imposed on the input format, thus it is capable of tackling diverse modelling tasks, such as few-shot audio classification and audio comparison. To further evaluate the advanced ability of the audio networks, we introduce natural language audio reasoning (NLAR), a new task that analyses two audio clips by comparison and summarisation. Experiments show that APT-enhanced LLMs (namely APT-LLMs) achieve competitive results compared to the expert models (i.e., the networks trained on the target datasets) across various tasks. We finally demonstrate APT's ability in extending frozen VLMs to the audio domain without fine-tuning, achieving promising results in audiovisual question and answering. Our code and model weights will be released at https://github.com/JinhuaLiang/APT. Index Terms—Audio understanding, large language model, audio-language learning, audio recognition, automated audio captioning, natural language audio reasoning.
Language-queried audio source separation (LASS) is a new paradigm for computational auditory scene analysis (CASA). LASS aims to separate a target sound from an audio mixture given a natural language query, which provides a natural and scalable interface for digital audio applications. Recent works on LASS, despite attaining promising separation performance on specific sources (e.g., musical instruments, limited classes of audio events), are unable to separate audio concepts in the open domain. In this work, we introduce AudioSep, a foundation model for open-domain audio source separation with natural language queries. We train AudioSep on large-scale multimodal datasets and extensively evaluate its capabilities on numerous tasks including audio event separation, musical instrument separation, and speech enhancement. AudioSep demonstrates strong separation performance and impressive zero-shot generalization ability using audio captions or text labels as queries, substantially outperforming previous audio-queried and language-queried sound separation models. For reproducibility of this work, we will release the source code, evaluation benchmark and pre-trained model at: https://github.com/Audio-AGI/AudioSep.
Large language models (LLMs) have significantly advanced audio processing through audio codecs that convert audio into discrete tokens, enabling the application of language modelling techniques to audio data. However, traditional codecs often operate at high bitrates or within narrow domains such as speech and lack the semantic clues required for efficient language modelling. Addressing these challenges, we introduce SemantiCodec, a novel codec designed to compress audio into fewer than a hundred tokens per second across diverse audio types, including speech, general sound, and music, without compromising quality. SemantiCodec features a dual-encoder architecture: a semantic encoder using a self-supervised pre-trained Audio Masked Autoencoder (AudioMAE), discretized using k-means clustering on extensive audio data, and an acoustic encoder to capture the remaining details. The semantic and acoustic encoder outputs are used to reconstruct audio via a diffusion-model-based decoder. SemantiCodec is presented in three variants with token rates of 25, 50, and 100 per second, supporting a range of ultra-low bit rates between 0.31 kbps and 1.40 kbps. Experimental results demonstrate that SemantiCodec significantly outperforms the state-of-the-art Descript codec on reconstruction quality. Our results also suggest that SemantiCodec contains significantly richer semantic information than all evaluated state-of-the-art audio codecs, even at significantly lower bitrates. Our code and demos are available at https://haoheliu.github.io/SemantiCodec/ .
—In the Industrial Internet of Things (IIoT), outdoor electronic devices serve crucial roles across sectors, providing vital data for decision-making. However, their exposure to open outdoor environments makes them vulnerable to unauthorized access, physical theft, or compromise, endangering both the device and its data. Ensuring the security of outdoor devices and their data is thus critical. This study addresses data security in outdoor IIoT devices by supporting the encryption of all IIoT-related data in device memory. Accessing and retrieving this data requires operations on encrypted data. Hence, we introduce a Searchable Symmetric Encryption (SSE) scheme called MI3SE, which ensures each device's encryption key is unique and valid for a period based on the device's security sensitivity. Moreover, MI3SE meets key security requirements, including confidentiality, integrity, forward secrecy, and backward secrecy. It is specifically designed to mitigate physical compromise and query pattern analysis through a two-keyword query approach and withstand various attacks, as validated by rigorous security analysis. Comparative evaluations against benchmark schemes underscore the efficacy of MI3SE in terms of both security and performance. Moreover, comprehensive non-mathematical security analysis and simulation experiments affirm the enhanced accuracy and efficacy of MI3SE in securing sensitive data stored in outdoor IIoT devices.
Blind deconvolution is an ill-posed problem. To solve such a prob- lem, prior information, such as, the sparseness of the source (i.e. input) signal or channel impulse responses, is usually adopted. In speech deconvolution, the source signal is not naturally sparse. However, the direct impulse and early reflections of the impulse responses of an acoustic system can be considered as sparse. In this paper, we exploit the channel sparsity and present an algorithm for speech deconvolution, where the dynamic range of the convolutive speech is also used as the prior information. In this algorithm, the estimation of the impulse response and the source signal is achieved by alternating between two steps, namely, the ℓ1 regularized least squares optimization and a proximal operation. As demonstrated in our experiments, the proposed method pro- vides superior performance for deconvolution of a sparse acoustic system, as compared with two state-of-the-art methods.
Audio-visual speaker tracking has drawn increasing attention over the past few years due to its academic values and wide application. Audio and visual modalities can provide complementary information for localization and tracking. With audio and visual information, the Bayesian-based filter can solve the problem of data association, audio-visual fusion and track management. In this paper, we conduct a comprehensive overview of audio-visual speaker tracking. To our knowledge, this is the first extensive survey over the past five years. We introduce the family of Bayesian filters and summarize the methods for obtaining audio-visual measurements. In addition, the existing trackers and their performance on AV16.3 dataset are summarized. In the past few years, deep learning techniques have thrived, which also boosts the development of audio visual speaker tracking. The influence of deep learning techniques in terms of measurement extraction and state estimation is also discussed. At last, we discuss the connections between audio-visual speaker tracking and other areas such as speech separation and distributed speaker tracking.
Sound event localization and detection (SELD) combines two subtasks: sound event detection (SED) and direction of arrival (DOA) estimation. SELD is usually tackled as an audio-only problem, but visual information has been recently included. Few audio-visual (AV)-SELD works have been published and most employ vision via face/object bounding boxes, or human pose keypoints. In contrast, we explore the integration of audio and visual feature embeddings extracted with pre-trained deep networks. For the visual modality, we tested ResNet50 and Inflated 3D ConvNet (I3D). Our comparison of AV fusion methods includes the AV-Conformer and Cross-Modal Attentive Fusion (CMAF) model. Our best models outperform the DCASE 2023 Task3 audio-only and AV baselines by a wide margin on the development set of the STARSS23 dataset, making them competitive amongst state-of-the-art results of the AV challenge, without model ensembling, heavy data augmentation, or prediction post-processing. Such techniques and further pre-training could be applied as next steps to improve performance.
Antimicrobial Resistance (AMR) is a growing public and veterinary health concern, and the ability to accurately predict AMR from antibiotics administration data is crucial for effectively treating and managing infections. While genomics-based approaches can provide better results, sequencing, assembling, and applying Machine Learning (ML) methods can take several hours. Therefore, alternative approaches are required. This study focused on using ML for antimicrobial stewardship by utilising data extracted from hospital electronic health records, which can be done in real-time, and developing an interpretable 1D-Transformer model for predicting AMR. A multi-baseline Integrated Gradient pipeline was also incorporated to interpret the model, and quantitative validation metrics were introduced to validate the model. The performance of the proposed 1D-Transformer model was evaluated using a dataset of urinary tract infection (UTI) patients with four antibiotics. The proposed 1D-Transformer model achieved 10% higher area under curve (AUC) in predicting AMR and outperformed traditional ML models. The Explainable Artificial Intelligence (XAI) pipeline also provided interpretable results, identifying the signatures contributing to the predictions. This could be used as a decision support tool for personalised treatment, introducing AMR-aware food and management of AMR, and it could also be used to identify signatures for targeted interventions.
The advancement of audio-language (AL) multi-modal learning tasks has been significant in recent years, yet the limited size of existing audio-language datasets poses challenges for researchers due to the costly and time-consuming collection process. To address this data scarcity issue, we introduce WavCaps, the first large-scale weakly-labelled audio captioning dataset, comprising approximately 400k audio clips with paired captions. We sourced audio clips and their raw descriptions from web sources and a sound event detection dataset. However, the online-harvested raw descriptions are highly noisy and unsuitable for direct use in tasks such as automated audio captioning. To overcome this issue, we propose a three-stage processing pipeline for filtering noisy data and generating high-quality captions, where ChatGPT, a large language model, is leveraged to filter and transform raw descriptions automatically. We conduct a comprehensive analysis of the characteristics of WavCaps dataset and evaluate it on multiple downstream audio-language multimodal learning tasks. The systems trained on WavCaps outperform previous state-of-the-art (SOTA) models by a significant margin. Our aspiration is for the WavCaps dataset we have proposed to facilitate research in audio-language multimodal learning and demonstrate the potential of utilizing large language models (LLMs) to enhance academic research. Our dataset and codes are available at https://github.com/XinhaoMei/WavCaps.
Cross-modal content generation has become very popular in recent years. To generate high-quality and realistic content, a variety of methods have been proposed. Among these approaches, visual content generation has attracted significant attention from academia and industry due to its vast potential in various applications. This survey provides an overview of recent advances in visual content generation conditioned on other modalities, such as text, audio, speech, and music, with a focus on their key contributions to the community. In addition, we summarize the existing publicly available datasets that can be used for training and benchmarking cross-modal visual content generation models. We provide an in-depth exploration of the datasets used for audio-to-visual content generation, filling a gap in the existing literature. Various evaluation metrics are also introduced along with the datasets. Furthermore, we discuss the challenges and limitations encountered in the area, such as modality alignment and semantic coherence. Last, we outline possible future directions for synthesizing visual content from other modalities including the exploration of new modalities, and the development of multi-task multi-modal networks. This survey serves as a resource for researchers interested in quickly gaining insights into this burgeoning field.
Audio-text retrieval aims at retrieving a target audio clip or caption from a pool of candidates given a query in another modality. Solving such cross-modal retrieval task is challenging because it not only requires learning robust feature representations for both modalities, but also requires capturing the fine-grained alignment between these two modalities. Existing cross-modal retrieval models are mostly optimized by metric learning objectives as both of them attempt to map data to an embedding space, where similar data are close together and dissimilar data are far apart. Unlike other cross-modal retrieval tasks such as image-text and video-text retrievals, audio-text retrieval is still an unexplored task. In this work, we aim to study the impact of different metric learning objectives on the audio-text retrieval task. We present an extensive evaluation of popular metric learning objectives on the AudioCaps and Clotho datasets. We demonstrate that NT-Xent loss adapted from self-supervised learning shows stable performance across different datasets and training settings, and outperforms the popular triplet-based losses. Our code is available at https://github.com/XinhaoMei/ audio-text_retrieval.
Deep neural networks based methods dominate recent development in single channel speech enhancement. In this paper, we propose a multi-scale feature recalibration convolutional encoder-decoder with bidirectional gated recurrent unit (BGRU) architecture for end-to-end speech enhancement. More specifically, multi-scale recalibration 2-D convolutional layers are used to extract local and contextual features from the signal. In addition, a gating mechanism is used in the recalibration network to control the information flow among the layers, which enables the scaled features to be weighted in order to retain speech and suppress noise. The fully connected layer (FC) is then employed to compress the output of the multi-scale 2-D convolutional layer with a small number of neurons, thus capturing the global information and improving parameter efficiency. The BGRU layers employ forward and backward GRUs, which contain the reset, update, and output gates, to exploit the interdependency among the past, current and future frames to improve predictions. The experimental results confirm that the proposed MCGN method outperforms several state-of-the-art methods.
A block-based compressed sensing approach coupled with binary time-frequency masking is presented for the underdetermined speech separation problem. The proposed algorithm consists of multiple steps. First, the mixed signals are segmented to a number of blocks. For each block, the unknown mixing matrix is estimated in the transform domain by a clustering algorithm. Using the estimated mixing matrix, the sources are recovered by a compressed sensing approach. The coarsely separated sources are then used to estimate the time-frequency binary masks which are further applied to enhance the separation performance. The separated source components from all the blocks are concatenated to reconstruct the whole signal. Numerical experiments are provided to show the improved separation performance of the proposed algorithm, as compared with two recent approaches. The block-based operation has the advantage in improving considerably the computational efficiency of the compressed sensing algorithm without degrading its separation performance.
Universal sound separation (USS) is a task of separating mixtures of arbitrary sound sources. Typically, universal separation models are trained from scratch in a supervised manner, using labeled data. Self-supervised learning (SSL) is an emerging deep learning approach that leverages unlabeled data to obtain task-agnostic representations, which can benefit many downstream tasks. In this paper, we propose integrating a self-supervised pre-trained model, namely the audio masked autoencoder (A-MAE), into a universal sound separation system to enhance its separation performance. We employ two strategies to utilize SSL embeddings: freezing or updating the parameters of A-MAE during fine-tuning. The SSL embeddings are concate-nated with the short-time Fourier transform (STFT) to serve as input features for the separation model. We evaluate our methods on the AudioSet dataset, and the experimental results indicate that the proposed methods successfully enhance the separation performance of a state-of-the-art ResUNet-based USS model.
The problem of multiple acoustic source localization using observations from a microphone array network is investigated in this article. Multiple source signals are assumed to be window-disjoint-orthogonal (WDO) on the time-frequency (TF) domain and time delay of arrival (TDOA) measurements are extracted at each TF bin. A Bayesian network model is then proposed to jointly assign the measurements to different sources and estimate the acoustic source locations. Considering that the WDO assumption is usually violated under reverberant and noisy environments, we construct a relational network by coding the distance information between the distributed microphone arrays such that adjacent arrays have higher probabilities of observing the same acoustic source, which is able to mitigate the miss detection issues in adverse environments. A Laplace approximate variational inference method is introduced to estimate the hidden variables in the proposed Bayesian network model. Both simulations and real data experiments are performed. The results show that our proposed method is able to achieve better source localization accuracy than existing methods.
Dictionary learning aims to adapt elementary codewords directly from training data so that each training signal can be best approximated by a linear combination of only a few codewords. Following the two-stage iterative processes: sparse coding and dictionary update, that are commonly used, for example, in the algorithms of MOD and K-SVD, we propose a novel framework that allows one to update an arbitrary set of codewords and the corresponding sparse coefficients simultaneously, hence termed simultaneous codeword optimization (SimCO). Under this framework, we have developed two algorithms, namely the primitive and the regularized SimCO. Simulations are provided to show the advantages of our approach over the K-SVD algorithm in terms of both learning performance and running speed. © 2012 IEEE.
Unification of classification and regression is a major challenge in machine learning and has attracted increasing attentions from researchers. In this article, we present a new idea for this challenge, where we convert the classification problem into a regression problem, and then use the methods in regression to solve the problem in classification. To this end, we leverage the widely used maximum margin classification algorithm and its typical representative, support vector machine (SVM). More specifically, we convert SVM into a piecewise linear regression task and propose a regression-based SVM (RBSVM) hyperparameter learning algorithm, where regression methods are used to solve several key problems in classification, such as learning of hyperparameters, calculation of prediction probabilities, and measurement of model uncertainty. To analyze the uncertainty of the model, we propose a new concept of model entropy, where the leave-one-out prediction probability of each sample is converted into entropy, and then used to quantify the uncertainty of the model. The model entropy is different from the classification margin, in the sense that it considers the distribution of all samples, not just the support vectors. Therefore, it can assess the uncertainty of the model more accurately than the classification margin. In the case of the same classification margin, the farther the sample distribution is from the classification hyperplane, the lower the model entropy. Experiments show that our algorithm (RBSVM) provides higher prediction accuracy and lower model uncertainty, when compared with state-of-the-art algorithms, such as Bayesian hyperparameter search and gradient-based hyperparameter learning algorithms.
Audio and visual signals can be used jointly to provide complementary information for multi-speaker tracking. Face detectors and color histogram can provide visual measurements while Direction of Arrival (DOA) lines and global coherence field (GCF) maps can provide audio measurements. GCF, as a traditional sound source localization method, has been widely used to provide audio measurements in audio-visual speaker tracking by estimating the positions of speakers. However, GCF cannot directly deal with the scenarios of multiple speakers due to the emergence of spurious peaks on the GCF map, making it difficult to find the non-dominant speakers. To overcome this limitation, we propose a phase-aware VoiceFilter and a separation-before-localization method, which enables the audio mixture to be separated into individual speech sources while retaining their phases. This allows us to calculate the GCF map for multiple speakers, thereby their positions accurately and concurrently. Based on this method, we design an adaptive audio measurement likelihood for audio-visual multiple speaker tracking using Poisson multi-Bernoulli mixture (PMBM) filter. The experiments demonstrate that our proposed tracker achieves state-of-the-art results on the AV16.3 dataset.
Data-driven approaches hold promise for audio captioning. However, the development of audio captioning methods can be biased due to the limited availability and quality of text-audio data. This paper proposes a SynthAC framework, which leverages recent advances in audio generative models and commonly available text corpus to create synthetic text-audio pairs, thereby enhancing text-audio representation. Specifically, the text-to-audio generation model, i.e., AudioLDM, is used to generate synthetic audio signals with captions from an image captioning dataset. Our SynthAC expands the availability of well-annotated captions from the text-vision domain to audio captioning, thus enhancing text-audio representation by learning relations within synthetic text-audio pairs. Experiments demonstrate that our SynthAC framework can benefit audio captioning models by incorporating well-annotated text corpus from the text-vision domain, offering a promising solution to the challenge caused by data scarcity. Furthermore, SynthAC can be easily adapted to various state-of-the-art methods, leading to substantial performance improvements.
Acoustic scene classification (ASC) can be helpful for creating context awareness for intelligent robots. Humans naturally use the relations between acoustic scenes (AS) and audio events (AE) to understand and recognize their surrounding environments. However, in most previous works, ASC and audio event classification (AEC) are treated as independent tasks, with a focus primarily on audio features shared between scenes and events, but not their implicit relations. To address this limitation, we propose a cooperative scene-event modelling (cSEM) framework to automatically model the intricate scene-event relation by an adaptive coupling matrix to improve ASC. Compared with other scene-event modelling frameworks, the proposed cSEM offers the following advantages. First, it reduces the confusion between similar scenes by aligning the information of coarse-grained AS and fine-grained AE in the latent space, and reducing the redundant information between the AS and AE embeddings. Second, it exploits the relation information between AS and AE to improve ASC, which is shown to be beneficial, even if the information of AE is derived from unverified pseudo-labels. Third, it uses a regression-based loss function for cooperative modelling of scene-event relations, which is shown to be more effective than classification-based loss functions. Instantiated from four models based on either Transformer or convolutional neural networks, cSEM is evaluated on real-life and synthetic datasets. Experiments show that cSEM-based models work well in real-life scene-event analysis, offering competitive results on ASC as compared with other multi-feature or multi-model ensemble methods. The ASC accuracy achieved on the TUT2018, TAU2019, and JSSED datasets is 81.0%, 88.9% and 97.2%, respectively.
Unsupervised anomalous sound detection (ASD) aims to detect unknown anomalous sounds of devices when only normal sound data is available. The autoencoder (AE) and self-supervised learning based methods are two mainstream methods. However, the AE-based methods could be limited as the feature learned from normal sounds can also fit with anomalous sounds, reducing the ability of the model in detecting anomalies from sound. The self-supervised methods are not always stable and perform differently, even for machines of the same type. In addition, the anomalous sound may be short-lived, making it even harder to distinguish from normal sound. This paper proposes an ID-constrained Transformer-based autoencoder (IDC-TransAE) architecture with weighted anomaly score computation for unsupervised ASD. Machine ID is employed to constrain the latent space of the Transformer-based autoencoder (TransAE) by introducing a simple ID classifier to learn the difference in the distribution for the same machine type and enhance the ability of the model in distinguishing anomalous sound. Moreover, weighted anomaly score computation is introduced to highlight the anomaly scores of anomalous events that only appear for a short time. Experiments performed on DCASE 2020 Challenge Task2 development dataset demonstrate the effectiveness and superiority of our proposed method.
Supervised learning has been used to solve monaural speech enhancement problem, offering state-of-the-art performance. However, clean training data is difficult or expensive to obtain in real room environments, which limits the training of supervised learning-based methods. In addition, mismatch conditions e.g., noises in the testing stages may be unseen in the training stage, present a common challenge. In this paper, we propose a self-supervised learning-based monaural speech enhancement method, using two autoencoders i.e., the speech autoencoder (SAE) and mixture autoencoder (MAE), with a shared layer, which help to mitigate mismatch conditions by learning a shared latent space between speech and mixture. To further improve the enhancement performance, we also propose phase-aware training and multi-resolution spectral losses. The latent representations of the amplitude and phase are independently learned in two decoders of the proposed SAE with only a very limited set of clean speech signals. Moreover, multi-resolution spectral losses help extract rich feature information. Experimental results on a benchmark dataset demonstrate that the proposed method outperforms the state-of-the-art self-supervised and supervised approaches. The source code is available at https://github.com/Yukino-3/Complex-SSL-SE. 1
Graph neural networks (GNNs) have achieved great success in many fields due to their powerful capabilities of processing graph-structured data. However, most GNNs can only be applied to scenarios where graphs are known, but real-world data are often noisy or even do not have available graph structures. Recently, graph learning has attracted increasing attention in dealing with these problems. In this article, we develop a novel approach to improving the robustness of the GNNs, called composite GNN. Different from existing methods, our method uses composite graphs (C-graphs) to characterize both sample and feature relations. The C-graph is a unified graph that unifies these two kinds of relations, where edges between samples represent sample similarities, and each sample has a tree-based feature graph to model feature importance and combination preference. By jointly learning multiaspect C-graphs and neural network parameters, our method improves the performance of semisupervised node classification and ensures robustness. We conduct a series of experiments to evaluate the performance of our method and the variants of our method that only learn sample relations or feature relations. Extensive experimental results on nine benchmark datasets demonstrate that our proposed method achieves the best performance on almost all the datasets and is robust to feature noises.
This paper proposes a method for jointly performing blind source separation (BSS) and blind dereverberation (BD) for speech mixtures. In most of the previous studies, BSS and BD have been explored separately. It is common that the performance of the speech separation algorithms deteriorates with the increase of room reverberations. Also most of the dereverberation algorithms rely on the availability of room impulse responses (RIRs) which are not readily accessible in practice. Therefore in this work the dereverberation and separation method are combined to mitigate the effects of room reverberations on the speech mixtures and hence to improve the separation performance. As required by the dereverberation algorithm, a step for blind estimation of reverberation time (RT) is used to estimate the decay rate of reverberations directly from the reverberant speech signal (i.e., speech mixtures) by modeling the decay as a Laplacian random process modulated by a deterministic envelope. Hence the developed algorithm works in a blind manner, i.e., directly dealing with the reverberant speech signals without explicit information from the RIRs. Evaluation results in terms of signal to distortion ratio (SDR) and segmental signal to reverberation ratio (SegSRR) reveal that using this method the performance of the separation algorithm that we have developed previously can be further enhanced. © 2012 EURASIP.
Spontaneous speech in videos capturing the speaker's mouth provides bimodal information. Exploiting the relationship between the audio and visual streams, we propose a new visual voice activity detection (VAD) algorithm, to overcome the vulnerability of conventional audio VAD techniques in the presence of background interference. First, a novel lip extraction algorithm combining rotational templates and prior shape constraints with active contours is introduced. The visual features are then obtained from the extracted lip region. Second, with the audio voice activity vector used in training, adaboosting is applied to the visual features, to generate a strong final voice activity classifier by boosting a set of weak classifiers. We have tested our lip extraction algorithm on the XM2VTS database (with higher resolution) and some video clips from YouTube (with lower resolution). The visual VAD was shown to offer low error rates.
© 2015 Elsevier B.V. All rights reserved. Existing speech source separation approaches overwhelmingly rely on acoustic pressure information acquired by using a microphone array. Little attention has been devoted to the usage of B-format microphones, by which both acoustic pressure and pressure gradient can be obtained, and therefore the direction of arrival (DOA) cues can be estimated from the received signal. In this paper, such DOA cues, together with the frequency bin-wise mixing vector (MV) cues, are used to evaluate the contribution of a specific source at each time-frequency (T-F) point of the mixtures in order to separate the source from the mixture. Based on the von Mises mixture model and the complex Gaussian mixture model respectively, a source separation algorithm is developed, where the model parameters are estimated via an expectation-maximization (EM) algorithm. A T-F mask is then derived from the model parameters for recovering the sources. Moreover, we further improve the separation performance by choosing only the reliable DOA estimates at the T-F units based on thresholding. The performance of the proposed method is evaluated in both simulated room environments and a real reverberant studio in terms of signal-to-distortion ratio (SDR) and the perceptual evaluation of speech quality (PESQ). The experimental results show its advantage over four baseline algorithms including three T-F mask based approaches and one convolutive independent component analysis (ICA) based method.
Estimating the geometric properties of an indoor environment through acoustic room impulse responses (RIRs) is useful in various applications, e.g., source separation, simultaneous localization and mapping, and spatial audio. Previously, we developed an algorithm to estimate the reflector’s position by exploiting ellipses as projection of 3D spaces. In this article, we present a model for full 3D reconstruction of environments. More specifically, the three components of the previous method, respectively, MUSIC for direction of arrival (DOA) estimation, numerical search adopted for reflector estimation and the Hough transform to refine the results, are extended for 3D spaces. A variation is also proposed using RANSAC instead of the numerical search and the Hough transform wich significantly reduces the run time. Both methods are tested on simulated and measured RIR data. The proposed methods perform better than the baseline, reducing the estimation error.
Low-rank tensor completion is a recent method for estimating the values of the missing elements in tensor data by minimizing the tensor rank. However, with only the low rank prior, the local piecewise smooth structure that is important for visual data is not used effectively. To address this problem, we define a new spatial regularization S-norm for tensor completion in order to exploit the local spatial smoothness structure of visual data. More specifically, we introduce the S-norm to the tensor completion model based on a non-convex LogDet function. The S-norm helps to drive the neighborhood elements towards similar values. We utilize the Alternating Direction Method of Multiplier (ADMM) to optimize the proposed model. Experimental results in visual data demonstrate that our method outperforms the state-of-the-art tensor completion models.
—Audio pattern recognition is an important research topic in the machine learning area, and includes several tasks such as audio tagging, acoustic scene classification, music classification , speech emotion classification and sound event detection. Recently, neural networks have been applied to tackle audio pattern recognition problems. However, previous systems are built on specific datasets with limited durations. Recently, in computer vision and natural language processing, systems pretrained on large-scale datasets have generalized well to several tasks. However, there is limited research on pretraining systems on large-scale datasets for audio pattern recognition. In this paper, we propose pretrained audio neural networks (PANNs) trained on the large-scale AudioSet dataset. These PANNs are transferred to other audio related tasks. We investigate the performance and computational complexity of PANNs modeled by a variety of convolutional neural networks. We propose an architecture called Wavegram-Logmel-CNN using both log-mel spectrogram and waveform as input feature. Our best PANN system achieves a state-of-the-art mean average precision (mAP) of 0.439 on AudioSet tagging, outperforming the best previous system of 0.392. We transfer PANNs to six audio pattern recognition tasks, and demonstrate state-of-the-art performance in several of those tasks. We have released the source code and pretrained models of PANNs: https://github.com/qiuqiangkong/audioset_tagging_cnn.
Decentralized cooperative localization (DCL) is a promising method to determine accurate multirobot poses (i.e., positions and orientations) for robot teams operating in an environment without absolute navigation information. Existing DCL methods often use fixed measurement noise covariance matrices for multirobot pose estimation; however, their performance degrades when the measurement noise covariance matrices are time-varying. To address this problem, in this article, a novel adaptive recursive DCL method is proposed for multi-robot systems with time-varying measurement accuracy. Each robot estimates its pose and measurement noise covariance matrices simultaneously in a decentralized manner based on the constructed hierarchical Gaussian models using the variational Bayesian approach. Simulation and experimental results show that the proposed method has improved cooperative localization accuracy and estimation consistency but slightly heavier computational load than the existing recursive DCL method.
Circular microphone arrays have been used for multi-speaker localization in computational auditory scene analysis, for their high flexibility in sound field analysis, including the generation of frequency-invariant eigenbeams for wideband acoustic sources. However, the localization performance of existing circular harmonic approaches, such as circular harmonics beamformer (CHB) depends strongly on the physical characteristics (such as shape) of sensor arrays, and the level of uncertainties presented in acoustic environments (such as background noise, room reverberation, and the number of sources). These uncertainties may limit the performance or practical application of the speaker localization algorithms. To address these issues, in this paper, we present a new indoor multi-speaker localization method in the circular harmonic domain based on the acoustic holography beamforming (AHB) technique and the Bayesian nonparametrics (BNP) method. More specifically, we use the AHB technique, which combines the delay-and-sum beamforming with acoustic-holography-based virtual sensing, to generate direction of arrival (DOA) measurements in the time-frequency (TF) domain, and then design a BNP algorithm based on the infinite Gaussian mixture model (IGMM) to estimate the DOAs of the individual sources without the prior knowledge about the number of sources. These estimates may degrade in the presence of room reverberation and background noise. To address this issue, we develop a robust TF bin selection and permutation method on the basis of mixture weights, using power, power ratio and local variance estimated at each TF bin. Experiments performed on both simulated and real-data show that our method gives significantly better performance, than four recent baseline methods, in a variety of noise and reverberation levels, in terms of the root-mean-square error (RMSE) of the DOA estimation and the source detecting success rate.
Learning effective vocal representations from a waveform mixture is a crucial but challenging task for deep neural network (DNN)-based singing voice separation (SVS). Successful representation learning (RL) depends heavily on well-designed neural architectures and effective general priors. However, DNNs for RL in SVS are mostly built on generic architectures without general priors being systematically considered. To address these issues, we introduce deep unfolding to RL and propose two RL-based models for SVS, deep unfolded representation learning (DURL) and optimal transport DURL (OT-DURL). In both models, we formulate RL as a sequence of optimization problems for signal reconstruction, where three general priors, synthesis, non-negative, and our novel analysis, are incorporated. In DURL and OT-DURL, we take different approaches in penalizing the analysis prior. DURL uses the Euclidean distance as its penalty, while OT-DURL uses a more sophisticated penalty known as the OT distance. We address the optimization problems in DURL and OT-DURL with the first-order operator splitting algorithm and unfold the obtained iterative algorithms to novel encoders, by mapping the synthesis/analysis/non-negative priors to different interpretable sublayers of the encoders. We evaluated these DURL and OT-DURL encoders in the unsupervised informed SVS and supervised Open-Unmix frameworks. Experimental results indicate that (1) the OT-DURL encoder is better than the DURL encoder and (2) both encoders can considerably improve the vocal-signal-separation performance compared with those of the baseline model.
Accurate prediction of the traffic state has received sustained attention for its ability to provide the anticipatory traffic condition required for people's travel and traffic management. In this paper, we propose a novel short-term traffic flow prediction method based on wavelet transform (WT) and multi-dimensional Taylor network (MTN), which is named as W-MTN. Influenced by the short-term noise disturbance in traffic flow information, the WT is employed to improve prediction accuracy by decomposing the time series of traffic flow. The MTN model, which exploits polynomials to approximate the unknown nonlinear function, makes full use of periodicity and temporal feature without transcendental knowledge and mechanism of the system to be predicted. Our proposed W-MTN model is evaluated on the traffic flow information in a certain area of Shenzhen, China. The experimental results indicate that the proposed W-MTN model offers better prediction performance and temporal correlation, as compared with the corresponding models in the known literature. In addition, the proposed model shows good robustness and generalization ability, when considering data from the different days and locations.
State-of-the-art audio captioning methods typically use the encoder-decoder structure with pretrained audio neural networks (PANNs) as encoders for feature extraction. However, the convolution operation used in PANNs is limited in capturing the long-time dependencies within an audio signal, thereby leading to potential performance degradation in audio captioning. This letter presents a novel method using graph attention (GraphAC) for encoder-decoder based audio captioning. In the encoder, a graph attention module is introduced after the PANNs to learn contextual association (i.e. the dependency among the audio features over different time frames) through an adjacency graph, and a top-k mask is used to mitigate the interference from noisy nodes. The learnt contextual association leads to a more effective feature representation with feature node aggregation. As a result, the decoder can predict important semantic information about the acoustic scene and events based on the contextual associations learned from the audio signal. Experimental results show that GraphAC outperforms the state-of-the-art methods with PANNs as the encoders, thanks to the incorporation of the graph attention module into the encoder for capturing the long-time dependencies within the audio signal.
Single channel blind source separation (SCBSS) refers to separating multiple sources from a mixture collected by a single sensor. Existing methods for SCBSS have limited performance in separating multiple sources and generalization. To address these problems, an algorithm is proposed in this paper to separate multiple sources from a mixture by designing a parallel dual generative adversarial network (PDualGAN) that can build the relationship between a mixture and the corresponding multiple sources to achieve one-to-multiple cross-domain mapping. This algorithm can be applied to a variety of mixtures including both instantaneous and convolutive mixtures. In addition, new datasets for single channel source separation are created which include the mixtures and corresponding sources for this study. Experiments were performed on four different datasets including both one-dimensional and two-dimensional signals. Experimental results show that the proposed algorithm outperforms state-of-the-art algorithms, measured with peak signal-to-noise ratio (PSNR), structural similarity index (SSIM), source-to-distortion ratio (SDR), source-to-interferences ratio (SIR), relative root mean squared error (RRMSE) and correlation.
In a recent study, it was shown that, with adversarial training of an attentive generative network, it is possible to convert a raindrop degraded image into a relatively clean one. However, in real world, raindrop appearance is not only formed by individual raindrops, but also by the distant raindrops accumulation and the atmospheric veiling, namely haze. Current methods are limited in extracting accurate features from a raindrop degraded image with background scene, the blurred raindrop regions, and the haze. In this paper, we propose a new model for an image corrupted by the raindrops and the haze, and introduce an integrated multi-task algorithm to address the joint raindrop and haze removal (JRHR) problem by combining an improved estimate of the atmospheric light, a modified transmission map, a generative adversarial network (GAN) and an optimized visual attention network. The proposed algorithm can extract more accurate features for both sky and non-sky regions. Experimental evaluation has been conducted to show that the proposed algorithm significantly outperforms state-of-the-art algorithms on both synthetic and real-world images in terms of both qualitative and quantitative measures.
Acoustic scene classification has drawn much research attention where labeled data are often used for model training. However, in practice, acoustic data are often unlabeled, weakly labeled, or incorrectly labeled. To classify unlabeled data, or detect and correct wrongly labeled data, we present an unsupervised clustering method based on sparse subspace clustering. The computational cost of the sparse subspace clustering algorithm becomes prohibitively high when dealing with high dimensional acoustic features. To address this problem, we introduce a random sketching method to reduce the feature dimensionality for the sparse subspace clustering algorithm. Experimental results reveal that this method can reduce the computational cost significantly with a limited loss in clustering accuracy.
This work aims to temporally localize events that are both audible and visible in video. Previous methods mainly focused on temporal modeling of events with simple fusion of audio and visual features. In natural scenes, a video records not only the events of interest but also ambient acoustic noise and visual background, resulting in redundant information in the raw audio and visual features. Thus, direct fusion of the two features often causes false localization of the events. In this paper, we propose a co-attention model to exploit the spatial and semantic correlations between the audio and visual features, which helps guide the extraction of discriminative features for better event localization. Our assumption is that in an audiovisual event, shared semantic information between audio and visual features exists and can be extracted by attention learning. Specifically, the proposed co-attention model is composed of a co-spatial attention module and a co-semantic attention module that are used to model the spatial and semantic correlations, respectively. The proposed co-attention model can be applied to various event localization tasks, such as cross-modality localization and multimodal event localization. Experiments on the public audiovisual event (AVE) dataset demonstrate that the proposed method achieves state-of-the-art performance by learning spatial and semantic co-attention.
Accurately estimating the ocean's interior structures using sea surface data is of vital importance for understanding the complexities of dynamic ocean processes. In this study, we proposed an advanced machine-learning method, the Light Gradient Boosting Machine (LightGBM)-based Deep Forest (LGB-DF) method, to estimate the ocean subsurface salinity structure (OSSS) in the South China Sea (SCS) by using sea surface data from multiple satellite observations. We selected sea surface salinity (SSS), sea surface temperature (SST), sea surface height (SSH), sea surface wind (SSW, decomposed into eastward wind speed (USSW) and northward wind speed (VSSW) components), and the geographical information (including longitude and latitude) as input data to estimate OSSS in the SCS. Argo data were used to train and validate the LGB-DF model. The model performance was evaluated using root mean square error (RMSE), normalized root mean square error (NRMSE), and determination coefficient (R-2). The results showed that the LGB-DF model had a good performance and outperformed the traditional LightGBM model in the estimation of OSSS. The proposed LGB-DF model using sea surface data by SSS/SST/SSH and SSS/SST/SSH/SSW performed less satisfactorily than when considering the contribution of the wind speed and geographical information, indicating that these are important parameters for accurately estimating OSSS. The performance of the LGB-DF model was found to vary with season and water depth. Better estimation accuracy was obtained in winter and autumn, which was due to weaker stratification. This method provided important technical support for estimating the OSSS from satellite-derived sea surface data, which offers a novel insight into oceanic observations.
A method based on Deep Neural Networks (DNNs) and time-frequency masking has been recently developed for binaural audio source separation. In this method, the DNNs are used to predict the Direction Of Arrival (DOA) of the audio sources with respect to the listener which is then used to generate soft time-frequency masks for the recovery/estimation of the individual audio sources. In this paper, an algorithm called ‘dropout’ will be applied to the hidden layers, affecting the sparsity of hidden units activations: randomly selected neurons and their connections are dropped during the training phase, preventing feature co-adaptation. These methods are evaluated on binaural mixtures generated with Binaural Room Impulse Responses (BRIRs), accounting a certain level of room reverberation. The results show that the proposed DNNs system with randomly deleted neurons is able to achieve higher SDRs performances compared to the baseline method without the dropout algorithm.
Persona-based dialogue systems aim to generate consistent responses based on historical context and predefined persona. Unlike conventional dialogue generation, the persona-based dialogue needs to consider both dialogue context and persona, posing a challenge for coherent training. Specifically, this requires a delicate weight balance between context and persona. To achieve that, in this paper, we propose an effective framework with Persona-Adaptive Attention (PAA), which adaptively integrates the weights from the persona and context information via our designed attention. In addition, a dynamic masking mechanism is applied to the PAA to not only drop redundant information in context and persona but also serve as a regularization mechanism to avoid overfitting. Experimental results demonstrate the superiority of the proposed PAA framework compared to the strong baselines in both automatic and human evaluation. Moreover, the proposed PAA approach can perform equivalently well in a low-resource regime compared to models trained in a full-data setting, which achieve a similar result with only 20% to 30% of data compared to the larger models trained in the full-data setting. To fully exploit the effectiveness of our design, we designed several variants for handling the weighted information in different ways, showing the necessity and sufficiency of our weighting and masking designs.
Discriminative dictionary learning (DDL) aims to address pattern classification problems via learning dictionaries from training samples. Dictionary pair learning (DPL) based DDL has shown superiority as compared with most existing algorithms which only learn synthesis dictionaries or analysis dictionaries. However, in the original DPL algorithm, the discrimination capability is only promoted via the reconstruction error and the structures of the learned dictionaries, while the discrimination of coding coefficients is not considered in the process of dictionary learning. To address this issue, we propose a new DDL algorithm by introducing an additional discriminative term associated with coding coefficients. Specifically, a support vector machine (SVM) based term is employed to enhance the discrimination of coding coefficients. In this model, a structured dictionary pair and SVM classifiers are jointly learned, and an optimization method is developed to address the formulated optimization problem. A classification scheme based on both the reconstruction error and SVMs is also proposed. Simulation results on several widely used databases demonstrate that the proposed method can achieve competitive performance as compared with some state-of-the-art DDL algorithms.
Foley sound generation aims to synthesise the background sound for multimedia content. Previous models usually employ a large development set with labels as input (e.g., single numbers or one-hot vector). In this work, we propose a diffusion model based system for Foley sound generation with text conditions. To alleviate the data scarcity issue, our model is initially pre-trained with large-scale datasets and fine-tuned to this task via transfer learning using the contrastive language-audio pertaining (CLAP) technique. We have observed that the feature embedding extracted by the text encoder can significantly affect the performance of the generation model. Hence, we introduce a trainable layer after the encoder to improve the text embedding produced by the encoder. In addition, we further refine the generated waveform by generating multiple candidate audio clips simultaneously and selecting the best one, which is determined in terms of the similarity score between the embedding of the candidate clips and the embedding of the target text label. Using the proposed method, our system ranks \({1}^{st}\) among the systems submitted to DCASE Challenge 2023 Task 7. The results of the ablation studies illustrate that the proposed techniques significantly improve sound generation performance. The codes for implementing the proposed system are available online.
Humans recognize objects by combining multi-sensory information in a coordinated fashion. However, visual-based and haptic-based object recognition remain two separate research directions in robotics. Visual images and haptic time series have different properties, which can be difficult for robots to fuse for object recognition as humans do. In this work, we propose an architecture to fuse visual, haptic and kinesthetic data for object recognition, based on the multimodal Convolutional Recurrent Neural Networks with Transformer. We use Convolutional Neural Networks (CNNs) to learn spatial representation, Recurrent Neural Networks (RNNs) to model temporal relationships, and Transformer’s self-attention and cross-attention structures to focus on global and cross-modal information. We propose two fusion methods and conduct experiments on the multimodal AU dataset. The results show that our model offers higher accuracy than the latest multimodal object recognition methods. We conduct an ablation study on the individual components of the inputs to demonstrate the importance of multimodal information in object recognition. The codes will be available at https://github.com/SYLan2019/VHKOR.
Foley sound presents the background sound for multimedia content and the generation of Foley sound involves computationally modelling sound effects with specialized techniques. In this work, we proposed a system for DCASE 2023 challenge task 7: Foley Sound Synthesis. The proposed system is based on AudioLDM, which is a diffusion-based text-to-audio generation model. To alleviate the data-hungry problem, the system first trained with large-scale datasets and then downstreamed into this DCASE task via transfer learning. Through experiments, we found out that the feature extracted by the encoder can significantly affect the performance of the generation model. Hence, we improve the results by leveraging the input label with related text embedding features obtained by a significant language model, i.e., contrastive language-audio pertaining (CLAP). In addition, we utilize a filtering strategy to further refine the output, i.e. by selecting the best results from the candidate clips generated in terms of the similarity score between the sound and target labels. The overall system achieves a Frechet audio distance (FAD) score of 4.765 on average among all seven different classes, substantially outperforming the baseline system which performs a FAD score of 9.7.
Audio tagging aims to assign predefined tags to audio clips to indicate the class information of audio events. Sequential audio tagging (SAT) means detecting both the class information of audio events, and the order in which they occur within the audio clip. Most existing methods for SAT are based on connectionist temporal classification (CTC). However, CTC cannot effectively capture event connections due to the conditional independence assumption between outputs at different times. The contextual Transformer (cTransformer) addresses this issue by exploiting contextual information in SAT. Nevertheless , cTransformer is also limited in exploiting contextual information as it only uses forward information in inference. This paper proposes a gated contextual Transformer (GCT) with forward-backward inference (FBI). In addition, a gated contextual multi-layer perceptron (GCMLP) block is proposed in GCT to improve the performance of cTransformer structurally. Experiments on the two real-life audio datasets with manually annotated sequential labels show that the proposed GCT with GCMLP and FBI performs better than the CTC-based methods and cTransformer.
The probability hypothesis density (PHD) filter based on sequential Monte Carlo (SMC) approximation (also known as SMC-PHD filter) has proven to be a promising algorithm for multi-speaker tracking. However, it has a heavy computational cost as surviving, spawned and born particles need to be distributed in each frame to model the state of the speakers and to estimate jointly the variable number of speakers with their states. In particular, the computational cost is mostly caused by the born particles as they need to be propagated over the entire image in every frame to detect the new speaker presence in the view of the visual tracker. In this paper, we propose to use audio data to improve the visual SMC-PHD (VSMC- PHD) filter by using the direction of arrival (DOA) angles of the audio sources to determine when to propagate the born particles and re-allocate the surviving and spawned particles. The tracking accuracy of the AV-SMC-PHD algorithm is further improved by using a modified mean-shift algorithm to search and climb density gradients iteratively to find the peak of the probability distribution, and the extra computational complexity introduced by mean-shift is controlled with a sparse sampling technique. These improved algorithms, named as AVMS-SMCPHD and sparse-AVMS-SMC-PHD respectively, are compared systematically with AV-SMC-PHD and V-SMC-PHD based on the AV16.3, AMI and CLEAR datasets.
Humans are able to identify a large number of environmental sounds and categorise them according to high-level semantic categories, e.g. urban sounds or music. They are also capable of generalising from past experience to new sounds when applying these categories. In this paper we report on the creation of a data set that is structured according to the top-level of a taxonomy derived from human judgements and the design of an associated machine learning challenge, in which strong generalisation abilities are required to be successful. We introduce a baseline classification system, a deep convolutional network, which showed strong performance with an average accuracy on the evaluation data of 80.8%. The result is discussed in the light of two alternative explanations: An unlikely accidental category bias in the sound recordings or a more plausible true acoustic grounding of the high-level categories.
The number of sources present in a mixture is crucial information often assumed to be known or detected by source counting. The exiting methods for source counting in underdetermined blind speech separation (UBSS) suffer from the overlapping between sources with low W-disjoint orthogonality (WDO). To address this issue, we propose to fit the direction of arrival (DOA) histogram with multiple von-Mises density (VM) functions directly and form a sparse recovery problem, where all the source clusters and the sidelobes in the DOA histogram are fitted with VM functions of different spatial parameters. We also developed a formula to perform the source counting taking advantage of the values of the sparse source vector to reduce the influence of sidelobes. Experiments are carried out to evaluate the proposed source counting method and the results show that the proposed method outperforms two well-known baseline methods.
Generative Adversarial Networks (GANs) have been used recently for anomaly detection from images, where the anomaly scores are obtained by comparing the global difference between the input and generated image. However, the anomalies often appear in local areas of an image scene, and ignoring such information can lead to unreliable detection of anomalies. In this paper, we propose an efficient anomaly detection network Skip-Attention GAN (SAGAN), which adds attention modules to capture local information to improve the accuracy of latent representation of images, and uses depth-wise separable convolutions to reduce the number of parameters in the model. We evaluate the proposed method on the CIFAR-10 dataset and the LBOT dataset (built by ourselves), and show that the performance of our method in terms of area under curve (AUC) on both datasets is improved by more than 10% on average, as compared with three recent baseline methods.
As a typical problem in time series analysis, traffic flow prediction is one of the most important application fields of machine learning. However, achieving highly accurate traffic flow prediction is a challenging task, due to the presence of complex dynamic spatial-temporal dependencies within a road network. This paper proposes a novel Dynamic Spatial-Temporal Aware Graph Neural Network (DSTAGNN) to model the complex spatial-temporal interaction in road network. First, considering the fact that historical data carries intrinsic dynamic information about the spatial structure of road networks, we propose a new dynamic spatial-temporal aware graph based on a data-driven strategy to replace the pre-defined static graph usually used in traditional graph convolution. Second, we design a novel graph neural network architecture, which can not only represent dynamic spatial relevance among nodes with an improved multi-head attention mechanism, but also acquire the wide range of dynamic temporal dependency from multi-receptive field features via multi-scale gated convolution. Extensive experiments on real-world data sets demonstrate that our proposed method significantly outperforms the state-of-the-art methods.
In a recent study of auditory evoked potential (AEP) based brain-computer interface (BCI), it was shown that, with an encoder–decoder framework, it is possible to translate human neural activity to speech (T-CAS). Current encoder–decoder-based methods achieve T-CAS often with a two-step approach where the information is passed between the encoder and decoder with a shared vector of reduced dimension, which, however, may result in information loss. In this paper, we propose an end-to-end model to translate human neural activity to speech (ET-CAS) by introducing a dual-dual generative adversarial network (Dual-DualGAN) for cross-domain mapping between electroencephalogram (EEG) and speech signals. In this model, we bridge the EEG and speech signals by introducing transition signals which are obtained by cascading the corresponding EEG and speech signals in a certain proportion. We then learn the mappings between the speech/EEG signals and the transition signals. We also develop a new EEG dataset where the attention of the participants is detected before the EEG signals are recorded to ensure that the participants have good attention in listening to speech utterances. The proposed method can translate word-length and sentence-length sequences of neural activity to speech. Experimental results show that the proposed method significantly outperforms state-of-the-art methods on both words and sentences of auditory stimulus.
A block-based compressed sensing approach coupled with binary time-frequency masking is presented for the underdetermined speech separation problem. The proposed algorithm consists of multiple steps. First, the mixed signals are segmented to a number of blocks. For each block, the unknown mixing matrix is estimated in the transform domain by a clustering algorithm. Using the estimated mixing matrix, the sources are recovered by a compressed sensing approach. The coarsely separated sources are then used to estimate the time-frequency binary masks which are further applied to enhance the separation performance. The separated source components from all the blocks are concatenated to reconstruct the whole signal. Numerical experiments are provided to show the improved separation performance of the proposed algorithm, as compared with two recent approaches. The block-based operation has the advantage in improving considerably the computational efficiency of the compressed sensing algorithm without degrading its separation performance.
© 2014 International Society of Information Fusion.Acoustic source tracking in a room environment based on a number of distributed microphone pairs has been widely studied in the past. Based on the received microphone pair signals, the time-delay of arrival (TDOA) measurement is easily accessible. Bayesian tracking approaches such as extended Kalman filter (EKF) and particle filtering (PF) are subsequently applied to estimate the source position. In this paper, the Bayesian performance bound, namely posterior Cramér-Rao bound (PCRB) is derived for such a tracking scheme. Since the position estimation is indirectly related to the received signal, a two-stage approach is developed to formulate the Fisher information matrix (FIM). First, the Cramér-Rao bound (CRB) of the TDOA measurement in the noisy and reverberant environment is calculated. The CRB is then regarded as the variance of the TDOAs in the measurement function to obtain the PCRB. Also, two different TDOA measurement models are considered: 1) single TDOA corresponding to the largest peak of the generalized cross-correlation (GCC) function; and 2) multiple TDOAs from several peaks in GCC function. The later measurement model implies a higher probability of detection and heavier false alarms. The PCRB for both measurement models are derived. Simulations under different noisy and reverberant environments are organized to validate the proposed PCRB.
In this paper, we present a gated convolutional neural network and a temporal attention-based localization method for audio classification, which won the 1st place in the large-scale weakly supervised sound event detection task of Detection and Classification of Acoustic Scenes and Events (DCASE) 2017 challenge. The audio clips in this task, which are extracted from YouTube videos, are manually labelled with one or more audio tags, but without time stamps of the audio events, hence referred to as weakly labelled data. Two subtasks are defined in this challenge including audio tagging and sound event detection using this weakly labelled data. We propose a convolutional recurrent neural network (CRNN) with learnable gated linear units (GLUs) non-linearity applied on the log Mel spectrogram. In addition, we propose a temporal attention method along the frames to predict the locations of each audio event in a chunk from the weakly labelled data. The performances of our systems were ranked the 1st and the 2nd as a team in these two sub-tasks of DCASE 2017 challenge with F value 55.6% and Equal error 0.73, respectively.
The problem of underdetermined blind audio source separation is usually addressed under the framework of sparse signal representation. In this paper, we develop a novel algorithm for this problem based on compressed sensing which is an emerging technique for efficient data reconstruction. The proposed algorithm consists of two stages. The unknown mixing matrix is firstly estimated from the audio mixtures in the transform domain, as in many existing methods, by a K-means clustering algorithm. Different from conventional approaches, in the second stage, the sources are recovered by using a compressed sensing approach. This is motivated by the similarity between the mathematical models adopted in compressed sensing and source separation. Numerical experiments including the comparison with a recent sparse representation approach are provided to show the good performance of the proposed method.
Despite recent progress in text-to-audio (TTA) generation, we show that the state-of-the-art models, such as AudioLDM, trained on datasets with an imbalanced class distribution, such as AudioCaps, are biased in their generation performance. Specifically, they excel in generating common audio classes while underperforming in the rare ones, thus degrading the overall generation performance. We refer to this problem as long-tailed text-to-audio generation. To address this issue, we propose a simple retrieval-augmented approach for TTA models. Specifically, given an input text prompt, we first leverage a Contrastive Language Audio Pretraining (CLAP) model to retrieve relevant text-audio pairs. The features of the retrieved audio-text data are then used as additional conditions to guide the learning of TTA models. We enhance AudioLDM with our proposed approach and denote the resulting augmented system as Re-AudioLDM. On the AudioCaps dataset, Re-AudioLDM achieves a state-of-the-art Frechet Audio Distance (FAD) of 1.37, outperforming the existing approaches by a large margin. Furthermore, we show that Re-AudioLDM can generate realistic audio for complex scenes, rare audio classes, and even unseen audio types, indicating its potential in TTA tasks.
Polyphonic sound event localization and detection is not only detecting what sound events are happening but localizing corresponding sound sources. This series of tasks was first introduced in DCASE 2019 Task 3. In 2020, the sound event localization and detection task introduces additional challenges in moving sound sources and overlapping-event cases, which include two events of the same type with two different direction-of-arrival (DoA) angles. In this paper, a novel event-independent network for polyphonic sound event lo-calization and detection is proposed. Unlike the two-stage method we proposed in DCASE 2019 Task 3, this new network is fully end-to-end. Inputs to the network are first-order Ambisonics (FOA) time-domain signals, which are then fed into a 1-D convolutional layer to extract acoustic features. The network is then split into two parallel branches. The first branch is for sound event detection (SED), and the second branch is for DoA estimation. There are three types of predictions from the network, SED predictions, DoA predictions , and event activity detection (EAD) predictions that are used to combine the SED and DoA features for onset and offset estimation. All of these predictions have the format of two tracks indicating that there are at most two overlapping events. Within each track, there could be at most one event happening. This architecture introduces a problem of track permutation. To address this problem, a frame-level permutation invariant training method is used. Experimental results show that the proposed method can detect polyphonic sound events and their corresponding DoAs. Its performance on the Task 3 dataset is greatly increased as compared with that of the baseline method. Index Terms— Sound event localization and detection, direction of arrival, event-independent, permutation invariant training.
In this paper, we compare different deep neural networks (DNN) in extracting speech signals from competing speakers in room environments, including the conventional fullyconnected multilayer perception (MLP) network, convolutional neural network (CNN), recurrent neural network (RNN), and the recently proposed capsule network (CapsNet). Each DNN takes input of both spectral features and converted spatial features that are robust to position mismatch, and outputs the separation mask for target source estimation. In addition, a psychacoustically-motivated objective function is integrated in each DNN, which explores perceptual importance of each TF unit in the training process. Objective evaluations are performed on the separated sounds using the converged models, in terms of PESQ, SDR as well as STOI. Overall, all the implemented DNNs have greatly improved the quality and speech intelligibility of the embedded target source as compared to the original recordings. In particular, bidirectional RNN, either along the temporal direction or along the frequency bins, outperforms the other DNN structures with consistent improvement.
The intelligibility of speech in noise can be improved by modifying the speech. But with object-based audio, there is the possibility of altering the background sound while leaving the speech unaltered. This may prove a less intrusive approach, affording good speech intelligibility without overly compromising the perceived sound quality. In this study, the technique of spectral weighting was applied to the background. The frequency-dependent weightings for adaptation were learnt by maximising a weighted combination of two perceptual objective metrics for speech intelligibility and audio quality. The balance between the two objective metrics was determined by the perceptual relationship between intelligibility and quality. A neural network was trained to provide a fast solution for real-time processing. Tested in a variety of background sounds and speech-to-background ratios (SBRs), the proposed method led to a large intelligibility gain over the unprocessed baseline. Compared to an approach using constant weightings, the proposed method was able to dynamically preserve the overall audio quality better with respect to SBR changes.
Sparse deep networks have been widely used in many linear inverse problems, such as image super-resolution and signal recovery. Its performance is as good as deep learning at the same time its parameters are much less than deep learning. However, when the linear inverse problems involve several linear transformations or the ratio of input dimension to output dimension is large, the performance of a single sparse deep network is poor. In this paper, we propose a cascade sparse deep network to address the above problem. In our model, we trained two cascade sparse networks based on Gregor and LeCun’s “learned ISTA” and “learned CoD”. The cascade structure can effectively improve the performance as compared to the noncascade model. We use the proposed methods in image sparse code prediction and signal recovery. The experimental results show that both algorithms perform favorably against a single sparse network.
As a multi-label classification task, audio tagging aims to predict the presence or absence of certain sound events in an audio recording. Existing works in audio tagging do not explicitly consider the probabilities of the co-occurrences between sound events, which is termed as the label dependencies in this study. To address this issue, we propose to model the label dependencies via a graph-based method, where each node of the graph represents a label. An adjacency matrix is constructed by mining the statistical relations between labels to represent the graph structure information, and a graph convolutional network (GCN) is employed to learn node representations by propagating information between neighboring nodes based on the adjacency matrix, which implicitly models the label dependencies. The generated node representations are then applied to the acoustic representations for classification. Experiments on Audioset show that our method achieves a state-of-the-art mean average precision (mAP) of 0:434.
A sequential algorithm for the blind separation of a class of periodic source signals is introduced in this paper. The algorithm is based only on second-order statistical information and exploits the assumption that the source signals have distinct periods. Separation is performed by sequentially converging to a solution which in effect diagonalizes the output covariance matrix constructed at a lag corresponding to the fundamental period of the source we select, the one with the smallest period. Simulation results for synthetic signals and real electrocardiogram recordings show that the proposed algorithm has the ability to restore statistical independence, and its performance is comparable to that of the equivariant adaptive source separation (EASI) algorithm, a benchmark high-order statistics-based sequential algorithm with similar computational complexity. The proposed algorithm is also shown to mitigate the limitation that the EASI algorithm can separate at most one Gaussian distributed source. Furthermore, the steady-state performance of the proposed algorithm is compared with that of EASI and the block-based second-order blind identification (SOBI) method. © 2006 IEEE.
In object-based spatial audio system, positions of the audio objects (e.g. speakers/talkers or voices) presented in the sound scene are required as important metadata attributes for object acquisition and reproduction. Binaural microphones are often used as a physical device to mimic human hearing and to monitor and analyse the scene, including localisation and tracking of multiple speakers. The binaural audio tracker, however, is usually prone to the errors caused by room reverberation and background noise. To address this limitation, we present a multimodal tracking method by fusing the binaural audio with depth information (from a depth sensor, e.g., Kinect). More specifically, the PHD filtering framework is first applied to the depth stream, and a novel clutter intensity model is proposed to improve the robustness of the PHD filter when an object is occluded either by other objects or due to the limited field of view of the depth sensor. To compensate mis-detections in the depth stream, a novel gap filling technique is presented to map audio azimuths obtained from the binaural audio tracker to 3D positions, using speaker-dependent spatial constraints learned from the depth stream. With our proposed method, both the errors in the binaural tracker and the mis-detections in the depth tracker can be significantly reduced. Real-room recordings are used to show the improved performance of the proposed method in removing outliers and reducing mis-detections.
Scans of double-sided documents often suffer from show-through distortions, where contents of the reverse side (verso) may appear in the front-side page (recto). Several algorithms employed for show-through removal from the scanned images, are based on linear mixing models, including blind source separation (BSS), non-negative matrix factorization (NMF), and adaptive filtering. However, a recent study shows that a non-linear model may provide better performance for resolving the overlapping front-reverse contents, especially in grayscale scans. In this paper, we propose a new non-linear NMF algorithm based on projected gradient adaptation. An adaptive filtering process is also incorporated to further eliminate the blurring effect caused by non-perfect calibration of the scans. Our numerical tests show that the proposed algorithm offers better results than the baseline methods. © 2013 IEEE.
Dictionary learning has found broad applications in signal and image processing. By adding constraints to the traditional dictionary learning model, dictionaries with discriminative capability can be obtained which can deal with the task of image classification. The Discriminative Convolutional Analysis Dictionary Learning (DCADL) algorithm proposed recently has achieved promising results with low computational complexity. However, DCADL is still limited in classification performance because of the lack of constraints on dictionary structures. To solve this problem, this study introduces an adaptively ordinal locality preserving (AOLP) term to the original model of DCADL to further improve the classification performance. With the AOLP term, the distance ranking in the neighborhood of each atom can be preserved, which can improve the discrimination of coding coefficients. In addition, a linear classifier for the classification of coding coefficients is trained along with the dictionary. A new method is designed specifically to solve the optimization problem corresponding to the proposed model. Experiments are performed on several commonly used datasets to show the promising results of the proposed algorithm in classification performance and computational efficiency.
Clipping, or saturation, is a common nonlinear distortion in signal processing. Recently, declipping techniques have been proposed based on sparse decomposition of the clipped signals on a fixed dictionary, with additional constraints on the amplitude of the clipped samples. Here we propose a dictionary learning approach, where the dictionary is directly learned from the clipped measurements. We propose a soft-consistency metric that minimizes the distance to a convex feasibility set, and takes into account our knowledge about the clipping process. We then propose a gradient descent-based dictionary learning algorithm that minimizes the proposed metric, and is thus consistent with the clipping measurement. Experiments show that the proposed algorithm outperforms other dictionary learning algorithms applied to clipped signals. We also show that learning the dictionary directly from the clipped signals outperforms consistent sparse coding with a fixed dictionary.
Sparse coding and dictionary learning are popular techniques for linear inverse problems such as denoising or inpainting. However in many cases, the measurement process is nonlinear, for example for clipped, quantized or 1-bit measurements. These problems have often been addressed by solving constrained sparse coding problems, which can be difficult to solve, and assuming that the sparsifying dictionary is known and fixed. Here we propose a simple and unified framework to deal with nonlinear measurements. We propose a cost function that minimizes the distance to a convex feasibility set, which models our knowledge about the nonlinear measurement. This provides an unconstrained, convex, and differentiable cost function that is simple to optimize, and generalizes the linear least squares cost commonly used in sparse coding. We then propose proximal based sparse coding and dictionary learning algorithms, that are able to learn directly from nonlinearly corrupted signals. We show how the proposed framework and algorithms can be applied to clipped, quantized and 1-bit data.
Text-to-audio (TTA) system has recently gained attention for its ability to synthesize general audio based on text descriptions. However, previous studies in TTA have limited generation quality with high computational costs. In this study, we propose AudioLDM, a TTA system that is built on a latent space to learn the continuous audio representations from contrastive language-audio pretraining (CLAP) latents. The pretrained CLAP models enable us to train LDMs with audio embedding while providing text embedding as a condition during sampling. By learning the latent representations of audio signals and their compositions without modeling the cross-modal relationship, AudioLDM is advantageous in both generation quality and computational efficiency. Trained on AudioCaps with a single GPU, AudioLDM achieves state-of-the-art TTA performance measured by both objective and subjective metrics (e.g., frechet distance). Moreover, AudioLDM is the first TTA system that enables various text-guided audio manipulations (e.g., style transfer) in a zero-shot fashion. Our implementation and demos are available at https://audioldm.github.io.
Audio tagging aims to assign one or several tags to an audio clip. Most of the datasets are weakly labelled, which means only the tags of the clip are known, without knowing the occurrence time of the tags. The labeling of an audio clip is often based on the audio events in the clip and no event level label is provided to the user. Previous works have used the bag of frames model assume the tags occur all the time, which is not the case in practice. We propose a joint detection-classification (JDC) model to detect and classify the audio clip simultaneously. The JDC model has the ability to attend to informative and ignore uninformative sounds. Then only informative regions are used for classification. Experimental results on the “CHiME Home” dataset show that the JDC model reduces the equal error rate (EER) from 19.0% to 16.9%. More interestingly, the audio event detector is trained successfully without needing the event level label.
In this paper, we consider the problem of recovering the phase information of multiple sources from a mixed phaseless Short-Time Fourier Transform (STFT) measurement, which is called multiple input single output (MISO) phase retrieval problem. It is an inherently ill-posed problem due to the lack of the phase and mixing information, and the existing phase retrieval algorithms are not explicitly designed for this case. To address the MISO phase retrieval problem, a least squares (LS) method coupled with an independent component analysis (ICA) algorithm is proposed for the case of sufficiently long window length. When these conditions are not met, an integrated algorithm is presented, which combines a gradient descent (GD) algorithm by minimizing a non-convex loss function with an ICA algorithm. Experimental evaluation has been conducted to show that under appropriate conditions the proposed algorithms can explicitly recover the signals, the phases of the signals and the mixing matrix. In addition, the algorithm is robust to noise
© 2014 IEEE.We consider the source number estimation problem in the presence of unknown spatially nonuniform noise and underdetermined mixtures. We develop a new attractive source number estimator by replacing the covariance with the Fourth-Order (FO) cumulant of the observations. Furthermore, a Modified Minimum Description Length (MMDL) principle is proposed to reduce the divergence of the singular values corresponding to noise thereby improving the performance of the estimator. Simulation experiments validate the superiority of the proposed FO cumulant MMDL method to the conventional Minimum Description Length (MDL) method.
Automated Audio captioning (AAC) is a cross-modal translation task that aims to use natural language to describe the content of an audio clip. As shown in the submissions received for Task 6 of the DCASE 2021 Challenges, this problem has received increasing interest in the community. The existing AAC systems are usually based on an encoder-decoder architecture, where the audio signal is encoded into a latent representation, and aligned with its corresponding text descriptions, then a decoder is used to generate the captions. However, training of an AAC system often encounters the problem of data scarcity, which may lead to inaccurate representation and audio-text alignment. To address this problem, we propose a novel encoder-decoder framework called Contrastive Loss for Audio Captioning (CL4AC). In CL4AC, the self-supervision signals derived from the original audio-text paired data are used to exploit the correspondences between audio and texts by contrasting samples, which can improve the quality of latent representation and the alignment between audio and texts, while trained with limited data. Experiments are performed on the Clotho dataset to show the effectiveness of our proposed approach.
Head pose is an important cue in many applications such as, speech recognition and face recognition. Most approaches to head pose estimation to date have focussed on the use of visual information of a subject’s head. These visual approaches have a number of limitations such as, an inability to cope with occlusions, changes in the appearance of the head, and low resolution images. We present here a novel method for determining coarse head pose orientation purely from audio information, exploiting the direct to reverberant speech energy ratio (DRR) within a reverberant room environment. Our hypothesis is that a speaker facing towards a microphone will have a higher DRR and a speaker facing away from the microphone will have a lower DRR. This method has the advantage of actually exploiting the reverberations within a room rather than trying to suppress them. This also has the practical advantage that most enclosed living spaces, such as meeting rooms or offices are highly reverberant environments. In order to test this hypothesis we also present a new data set featuring 56 subjects recorded in three different rooms, with different acoustic properties, adopting 8 different head poses in 4 different room positions captured with a 16 element microphone array. As far as the authors are aware this data set is unique and will make a significant contribution to further work in the area of audio head pose estimation. Using this data set we demonstrate that our proposed method of using the DRR for audio head pose estimation provides a significant improvement over previous methods.
The existing convolutional neural network (CNN) based methods still have limitations in model accuracy, latency and computational cost for single channel speech enhancement. In order to address these limitations, we propose a multi-scale convolutional bidirectional long short-term memory (BLSTM) recurrent neural network, which is named as McbNet, a deep learning framework for end-to-end single channel speech enhancement. The proposed McbNet enlarges the receptive fields in two aspects. Firstly, every convolutional layer employs filters with varied dimensions to capture local and global information. Secondly, the BLSTM is applied to evaluate the interdependency of past, current and future temporal frames. The experimental results confirm the proposed McbNet offers consistent improvement over the state-of-the-art methods and public datasets.
Differentiable particle filters are an emerging class of particle filtering methods that use neural networks to construct and learn parametric state-space models. In real-world applications, both the state dynamics and measurements can switch between a set of candidate models. For instance, in target tracking, vehicles can idle, move through traffic, or cruise on motorways, and measurements are collected in different geographical or weather conditions. This paper proposes a new differentiable particle filter for regime-switching state-space models. The method can learn a set of unknown candidate dynamic and measurement models and track the state posteriors. We evaluate the performance of the novel algorithm in relevant models, showing its great performance compared to other competitive algorithms.
Increased use of social media platforms has resulted in a vast amount of user-generated video content being released to the internet daily. Measuring and monitoring the perceptual quality of these videos is vital for efficient network and storage management. However, these videos do not have a pristine reference, posing challenges for accurate quality monitoring. In this paper, we introduce a hybrid metric to measure the perceptual quality of user-generated video content using both pixel-level and compression-level features. Our experiments on large-scale databases of user-generated content show that the proposed method performs comparably in predicting the perceptual quality when compared with state-of-the-art metrics.
In this paper, we introduce the task of language-queried audio source separation (LASS), which aims to separate a target source from an audio mixture based on a natural language query of the target source (e.g., “a man tells a joke followed by people laughing”). A unique challenge in LASS is associated with the complexity of natural language description and its relation with the audio sources. To address this issue, we proposed LASSNet, an end-to-end neural network that is learned to jointly process acoustic and linguistic information, and separate the target source that is consistent with the language query from an audio mixture. We evaluate the performance of our proposed system with a dataset created from the AudioCaps dataset. Experimental results show that LASS-Net achieves considerable improvements over baseline methods. Furthermore, we observe that LASS-Net achieves promising generalization results when using diverse human-annotated descriptions as queries, indicating its potential use in real-world scenarios. The separated audio samples and source code are available at https://liuxubo717.github.io/LASS-demopage.
Although audio generation shares commonalities across different types of audio, such as speech, music, and sound effects, designing models for each type requires careful consideration of specific objectives and biases that can significantly differ from those of other types. To bring us closer to a unified perspective of audio generation, this paper proposes a holistic framework that utilizes the same learning method for speech, music, and sound effect generation. Our framework utilizes a general representation of audio, called “language of audio” (LOA). Any audio can be translated into LOA based on AudioMAE, a self-supervised pre-trained representation learning model. In the generation process, we translate other modalities into LOA by using a GPT-2 model, and we perform self-supervised audio generation learning with a latent diffusion model conditioned on the LOA of audio in our training set. The proposed framework naturally brings advantages such as reusable self-supervised pretrained latent diffusion models. Experiments on the major benchmarks of text-to-audio, text-to-music, and text-to-speech with three AudioLDM 2 variants demonstrate competitive performance of the AudioLDM 2 framework against previous approaches.
This survey paper provides a comprehensive overview of the recent advancements and challenges in applying large language models to the field of audio signal processing. Audio processing, with its diverse signal representations and a wide range of sources--from human voices to musical instruments and environmental sounds--poses challenges distinct from those found in traditional Natural Language Processing scenarios. Nevertheless, \textit{Large Audio Models}, epitomized by transformer-based architectures, have shown marked efficacy in this sphere. By leveraging massive amount of data, these models have demonstrated prowess in a variety of audio tasks, spanning from Automatic Speech Recognition and Text-To-Speech to Music Generation, among others. Notably, recently these Foundational Audio Models, like SeamlessM4T, have started showing abilities to act as universal translators, supporting multiple speech tasks for up to 100 languages without any reliance on separate task-specific systems. This paper presents an in-depth analysis of state-of-the-art methodologies regarding \textit{Foundational Large Audio Models}, their performance benchmarks, and their applicability to real-world scenarios. We also highlight current limitations and provide insights into potential future research directions in the realm of \textit{Large Audio Models} with the intent to spark further discussion, thereby fostering innovation in the next generation of audio-processing systems. Furthermore, to cope with the rapid development in this area, we will consistently update the relevant repository with relevant recent articles and their open-source implementations at https://github.com/EmulationAI/awesome-large-audio-models.
Spatiotemporal regularized Discriminative Correlation Filters (DCF) have been proposed recently for visual tracking, achieving state-of-the-art performance. However, the tracking performance of the online learning model used in this kind methods is highly dependent on the quality of the appearance feature of the target, and the target feature appearance could be heavily deformed due to the occlusion by other objects or the variations in their dynamic self-appearance. In this paper, we propose a new approach to mitigate these two kinds of appearance deformation. Firstly, we embed the occlusion perception block into the model update stage, then we adaptively adjust the model update according to the situation of occlusion. Secondly, we use the relatively stable colour statistics to deal with the appearance shape changes in large targets, and compute the histogram response scores as a complementary part of final correlation response. Extensive experiments are performed on four well-known datasets, i.e. OTB100, VOT-2018, UAV123, and TC128. The results show that the proposed approach outperforms the baseline DCF method, especially, on the TC128/UAV123 datasets, with a gain of over 4.05 %/2.43% in mean overlap precision. We will release our code at https://github.com/SYLan2019/STD0D.
Biomedical image segmentation of organs, tissues and lesions has gained increasing attention in clinical treatment planning and navigation, which involves the exploration of two-dimensional (2D) and three-dimensional (3D) contexts in the biomedical image. Compared to 2D methods, 3D methods pay more attention to inter-slice correlations, which offer additional spatial information for image segmentation. An organ or tumor has a 3D structure that can be observed from three directions. Previous studies focus only on the vertical axis, limiting the understanding of the relationship between a tumor and its surrounding tissues. Important information can also be obtained from sagittal and coronal axes. Therefore, spatial information of organs and tumors can be obtained from three directions, i.e. the sagittal, coronal and vertical axes, to understand better the invasion depth of tumor and its relationship with the surrounding tissues. Moreover, the edges of organs and tumors in biomedical image may be blurred. To address these problems, we propose a three-direction fusion volumetric segmentation (TFVS) model for segmenting 3D biomedical images from three perspectives in sagittal, coronal and transverse planes, respectively. We use the dataset of the liver task provided by the Medical Segmentation Decathlon challenge to train our model. The TFVS method demonstrates a competitive performance on the 3D-IRCADB dataset. In addition, the t-test and Wilcoxon signed-rank test are also performed to show the statistical significance of the improvement by the proposed method as compared with the baseline methods. The proposed method is expected to be beneficial in guiding and facilitating clinical diagnosis and treatment.
This paper presents a distributed multi-class Gaussian process (MCGP) algorithm for ground vehicle classification using acoustic data. In this algorithm, the harmonic structure analysis is used to extract features for GP classifier training. The predictions from local classifiers are then aggregated into a high-level prediction to achieve the decision-level fusion, following the idea of divide-and-conquer. Simulations based on the acoustic-seismic classification identification data set (ACIDS) confirm that the proposed algorithm provides competitive performance in terms of classification error and negative log-likelihood (NLL), as compared to an MCGP based on the data-level fusion where only one global MCGP is trained using data from all the sensors.
Visual object tracking is an important prerequisite in many applications. However, the performance of the tracking system is often affected by the quality of the visual object’s feature representation and whether it can identify the best match of the target template in the search area. To alleviate these challenges, we propose a new method based on Multi-Layer Perceptron (MLP) and multi-head cross attention. First, a new MLP-based module is designed to enhance the input features, by refining the internal association between the spatial and channel dimensions of these features. Second, an improved head network is constructed for predicting the location of the target, in which the multi-head cross attention mechanism is used to find the optimal matching between the template and the search area. Experiments on four datasets show that the proposed method offers competitive tracking performance as compared with several recent baseline methods. The codes will be available at https://github.com/SYLan2019/MLP-MHCA.
We investigate the problem of visual tracking of multiple human speakers in an office environment. In particular, we propose novel solutions to the following challenges: (1) robust and computationally efficient modeling and classification of the changing appearance of the speakers in a variety of different lighting conditions and camera resolutions; (2) dealing with full or partial occlusions when multiple speakers cross or come into very close proximity; (3) automatic initialization of the trackers, or re-initialization when the trackers have lost lock caused by e.g. the limited camera views. First, we develop new algorithms for appearance modeling of the moving speakers based on dictionary learning (DL), using an off-line training process. In the tracking phase, the histograms (coding coefficients) of the image patches derived from the learned dictionaries are used to generate the likelihood functions based on Support Vector Machine (SVM) classification. This likelihood function is then used in the measurement step of the classical particle filtering (PF) algorithm. To improve the computational efficiency of generating the histograms, a soft voting technique based on approximate Locality-constrained Soft Assignment (LcSA) is proposed to reduce the number of dictionary atoms (codewords) used for histogram encoding. Second, an adaptive identity model is proposed to track multiple speakers whilst dealing with occlusions. This model is updated online using Maximum a Posteriori (MAP) adaptation, where we control the adaptation rate using the spatial relationship between the subjects. Third, to enable automatic initialization of the visual trackers, we exploit audio information, the Direction of Arrival (DOA) angle, derived from microphone array recordings. Such information provides, a priori, the number of speakers and constrains the search space for the speaker's faces. The proposed system is tested on a number of sequences from three publicly available and challenging data corpora (AV16.3, EPFL pedestrian data set and CLEAR) with up to five moving subjects. © 2014 IEEE.
Monaural singing voice separation (MSVS) is a challenging task and has been extensively studied. Deep neural networks (DNNs) are current state-of-the-art methods for MSVS. However, they are often designed manually, which is time-consuming and error-prone. They are also pre-defined, thus cannot adapt their structures to the training data. To address these issues, we first designed a multi-resolution convolutional neural network (CNN) for MSVS called multi-resolution pooling CNN (MRP-CNN), which uses various-sized pooling operators to extract multi-resolution features. We then introduced Neural Architecture Search (NAS) to extend the MRP-CNN to the evolving MRP-CNN (E-MRP-CNN) to automatically search for effective MRP-CNN structures using genetic algorithms optimized in terms of a single objective taking into account only separation performance and multiple objectives taking into account both separation performance and model complexity. The E-MRP-CNN using the multi-objective algorithm gives a set of Pareto-optimal solutions, each providing a trade-off between separation performance and model complexity. Evaluations on the MIR-1 K, DSD100, and MUSDB18 datasets were used to demonstrate the advantages of the E-MRP-CNN over several recent baselines.
In recent research, deep neural network (DNN) has been used to solve the monaural source separation problem. According to the training objectives, DNN-based monaural speech separation is categorized into three aspects, namely masking, mapping and signal approximation (SA) based techniques. However, the performance of the traditional methods is not robust due to variations in real-world environments. Besides, in the vanilla DNN-based methods, the temporal information cannot be fully utilized. Therefore, in this paper, the long short-term memory (LSTM) neural network is applied to exploit the long-term speech contexts. Then, we propose the complex signal approximation (cSA) which is operated in the complex domain to utilize the phase information of the desired speech signal to improve the separation performance. The IEEE and the TIMIT corpora are used to generate mixtures with noise and speech interferences to evaluate the efficacy of the proposed method. The experimental results demonstrate the advantages of the proposed cSA-based LSTM RNN method in terms of different objective performance measures.
The detection of acoustic scenes is a challenging problem in which environmental sound events must be detected from a given audio signal. This includes classifying the events as well as estimating their onset and offset times. We approach this problem with a neural network architecture that uses the recentlyproposed capsule routing mechanism. A capsule is a group of activation units representing a set of properties for an entity of interest, and the purpose of routing is to identify part-whole relationships between capsules. That is, a capsule in one layer is assumed to belong to a capsule in the layer above in terms of the entity being represented. Using capsule routing, we wish to train a network that can learn global coherence implicitly, thereby improving generalization performance. Our proposed method is evaluated on Task 4 of the DCASE 2017 challenge. Results show that classification performance is state-of-the-art, achieving an Fscore of 58.6%. In addition, overfitting is reduced considerably compared to other architectures.
Heterogeneous feature representations are widely used in machine learning and pattern recognition, especially for multimedia analysis. The multi-modal, often also highdimensional, features may contain redundant and irrelevant information that can deteriorate the performance of modeling in classification. It is a challenging problem to select the informative features for a given task from the redundant and heterogeneous feature groups. In this paper, we propose a novel framework to address this problem. This framework is composed of two modules, namely, multi-modal deep neural networks and feature selection with sparse group LASSO. Given diverse groups of discriminative features, the proposed technique first converts the multi-modal data into a unified representation with different branches of the multi-modal deep neural networks. Then, through solving a sparse group LASSO problem, the feature selection component is used to derive a weight vector to indicate the importance of the feature groups. Finally, the feature groups with large weights are considered more relevant and hence are selected. We evaluate our framework on three image classification datasets. Experimental results show that the proposed approach is effective in selecting the relevant feature groups and achieves competitive classification performance as compared with several recent baseline methods.
Dictionary learning algorithms are typically derived for dealing with one or two dimensional signals using vector-matrix operations. Little attention has been paid to the problem of dictionary learning over high dimensional tensor data. We propose a new algorithm for dictionary learning based on tensor factorization using a TUCKER model. In this algorithm, sparseness constraints are applied to the core tensor, of which the n-mode factors are learned from the input data in an alternate minimization manner using gradient descent. Simulations are provided to show the convergence and the reconstruction performance of the proposed algorithm. We also apply our algorithm to the speaker identification problem and compare the discriminative ability of the dictionaries learned with those of TUCKER and K-SVD algorithms. The results show that the classification performance of the dictionaries learned by our proposed algorithm is considerably better as compared to the two state of the art algorithms. © 2013 IEEE.
The concept of sensing-as-a-service is proposed to enable a unified way of accessing and controlling sensing devices for many Internet of Things based applications. Existing techniques for Web service computing are not sufficient for this class of services that are exposed by resource-constrained devices. The vast number of distributed and redundantly deployed sensors necessitate specialised techniques for their discovery and ranking. Current research in this line mostly focuses on discovery, e.g., designing efficient searching methods by exploiting the geographical properties of sensing devices. The problem of ranking, which aims to prioritise semantically equivalent sensor services returned by the discovery process, has not been adequately studied. Existing methods mostly leverage the information directly associated with sensor services, such as detailed service descriptions or quality of service information. However, assuming the availability of such information for sensor services is often unrealistic. We propose a ranking strategy by estimating the cost of accessing sensor services. The computation is based on properties of the sensor nodes as well as the relevant contextual information extracted from the service access process. The evaluation results demonstrate not only the superior performance of the proposed method in terms of ranking quality measure, but also the potential for preserving the energy of the sensor nodes.
Sequential Monte Carlo probability hypothesis density (SMC-PHD) filtering is a popular method used recently for audio-visual (AV) multi-speaker tracking. However, due to the weight degeneracy problem, the posterior distribution can be represented poorly by the estimated probability, when only a few particles are present around the peak of the likelihood density function. To address this issue, we propose a new framework where particle flow (PF) is used to migrate particles smoothly from the prior to the posterior probability density. We consider both zero and non-zero diffusion particle flows (ZPF/NPF), and developed two new algorithms, AV-ZPF-SMC-PHD and AV-NPFSMC- PHD, where the speaker states from the previous frames are also considered for particle relocation. The proposed algorithms are compared systematically with several baseline tracking methods using the AV16.3, AVDIAR and CLEAR datasets, and are shown to offer improved tracking accuracy and average effective sample size (ESS).
Clipping is a common type of distortion in which the amplitude of a signal is truncated if it exceeds a certain threshold. Sparse representation has underpinned several algorithms developed recently for reconstruction of the original signal from clipped observations. However, these declipping algorithms are often built on a synthesis model, where the signal is represented by a dictionary weighted by sparse coding coefficients. In contrast to these works, we propose a sparse analysis-model-based declipping (SAD) method, where the declipping model is formulated on an analysis (i.e. transform) dictionary, and additional constraints characterizing the clipping process. The analysis dictionary is updated using the Analysis SimCO algorithm, and the signal is recovered by using a least-squares based method or a projected gradient descent method, incorporating the observable signal set. Numerical experiments on speech and music are used to demonstrate improved performance in signal to distortion ratio (SDR) compared to recent state-of-the-art methods including A-SPADE and ConsDL.
Semantic modeling for the Internet of Things has become fundamental to resolve the problem of interoperability given the distributed and heterogeneous nature of the “Things”. Most of the current research has primarily focused on devices and resources modeling while paid less attention on access and utilisation of the information generated by the things. The idea that things are able to expose standard service interfaces coincides with the service oriented computing and more importantly, represents a scalable means for business services and applications that need context awareness and intelligence to access and consume the physical world information. We present the design of a comprehensive description ontology for knowledge representation in the domain of Internet of Things and discuss how it can be used to support tasks such as service discovery, testing and dynamic composition.
The audio spectrogram is a time-frequency representation that has been widely used for audio classification. One of the key attributes of the audio spectrogram is the temporal resolution, which depends on the hop size used in the Short-Time Fourier Transform (STFT). Previous works generally assume the hop size should be a constant value (e.g., 10 ms). However, a fixed temporal resolution is not always optimal for different types of sound. The temporal resolution affects not only classification accuracy but also computational cost. This paper proposes a novel method, DiffRes, that enables differentiable temporal resolution modeling for audio classification. Given a spectrogram calculated with a fixed hop size, DiffRes merges non-essential time frames while preserving important frames. DiffRes acts as a "drop-in" module between an audio spectrogram and a classifier and can be jointly optimized with the classification task. We evaluate DiffRes on five audio classification tasks, using mel-spectrograms as the acoustic features, followed by off-the-shelf classifier backbones. Compared with previous methods using the fixed temporal resolution, the DiffRes-based method can achieve the equivalent or better classification accuracy with at least 25% computational cost reduction. We further show that DiffRes can improve classification accuracy by increasing the temporal resolution of input acoustic features, without adding to the computational cost.
The purpose of blind speech deconvolution is to recover both the original speech source and the room impulse response (RIR) from the observed reverberant speech. This can be beneficial for speech intelligibility and speech perception. However, the problem is ill-posed, which often requires additional knowledge to solve. In order to address this problem, prior informations (such as the sparseness of signal or acoustic channel) are often exploited. In this paper, we propose a joint L1 − L2 regularisation based blind speech deconvolution method for a single-input and single-output (SISO) acoustic system with a high level of reverberation, where both the sparsity and density of the room impulse responses (RIR) are considered, by imposing an L1 and L2 norm constraint on their early and late part respectively. By employing an alternating strategy, both the source signal and early part in the RIR can be well reconstructed while the late part of the RIR can be suppressed.
The information generated from the Internet of Things (IoT) potentially enables a better understanding of the physical world for humans and supports creation of ambient intelligence for a wide range of applications in different domains. A semantics-enabled service layer is a promising approach to facilitate seamless access and management of the information from the large, distributed and heterogeneous sources. This paper presents the efforts of the IoT.est project towards developing a framework for service creation and testing in an IoT environment. The architecture design extends the existing IoT reference architecture and enables a test-driven, semantics-based management of the entire service lifecycle. The validation of the architecture is shown though a dynamic test case generation and execution scenario.
The Web of Things aims to make physical world objects and their data accessible through standard Web technologies to enable intelligent applications and sophisticated data analytics. Due to the amount and heterogeneity of the data, it is challenging to perform data analysis directly; especially when the data is captured from a large number of distributed sources. However, the size and scope of the data can be reduced and narrowed down with search techniques, so that only the most relevant and useful data items are selected according to the application requirements. Search is fundamental to the Web of Things while challenging by nature in this context, e.g., mobility of the objects, opportunistic presence and sensing, continuous data streams with changing spatial and temporal properties, efficient indexing for historical and real time data. The research community has developed numerous techniques and methods to tackle these problems as reported by a large body of literature in the last few years. A comprehensive investigation of the current and past studies is necessary to gain a clear view of the research landscape and to identify promising future directions. This survey reviews the state-of-the-art search methods for the Web of Things, which are classified according to three different viewpoints: basic principles, data/knowledge representation, and contents being searched. Experiences and lessons learned from the existing work and some EU research projects related to Web of Things are discussed, and an outlook to the future research is presented.
In this paper, we consider the problem of recovering the phase information of the multiple images from the multiple mixed phaseless Short-Time Fourier Transform (STFT) image measurements, which is called the blind multiple input multiple output image phase retrieval (BMIPR) problem. It is an inherently ill-posed problem due to the lack of the phase and mixing information, and the existing phase retrieval algorithms are not explicitly designed for this case. To address the BMIPR phase retrieval problem, an integrated algorithm is presented, which combines a gradient descent (GD) algorithm by minimizing a nonconvex loss function with an independent component analysis (ICA) algorithm and a non-local means (NM) algorithm. Experimental evaluation has been conducted to show that under appropriate conditions the proposed algorithms can explicitly recover the images, the phases of the images and the mixing matrix. In addition, the algorithm is robust to noise.
The sequential Monte Carlo probability hypothesis density (SMC-PHD) filter has been shown to be promising for audio-visual multi-speaker tracking. Recently, the zero diffusion particle flow (ZPF) has been used to mitigate the weight degeneracy problem in the SMC-PHD filter. However, this leads to a substantial increase in the computational cost due to the migration of particles from prior to posterior distribution with a partial differential equation. This paper proposes an alternative method based on the non-zero diffusion particle flow (NPF) to adjust the particle states by fitting the particle distribution with the posterior probability density using the nonzero diffusion. This property allows efficient computation of the migration of particles. Results from the AV16.3 dataset demonstrate that we can significantly mitigate the weight degeneracy problem with a smaller computational cost as compared with the ZPF based SMC-PHD filter.
A novel algorithm for convolutive non-negative matrix factorization (NMF) with multiplicative rules is presented in this paper. In contrast to the standard NMF, the low rank approximation is represented by a convolutive model which has an advantage of revealing the temporal structure possessed by many realistic signals. The convolutive basis decomposition is obtained by the minimization of the conventional squared Euclidean distance, rather than the Kullback-Leibler divergence. The algorithm is applied to the audio pattern separation problem in the magnitude spectrum domain. Numerical experiments suggest that the proposed algorithm has both less computational loads and better separation performance for auditory pattern extraction, as compared with an existing method developed by Smaragdis. ©2007 IEEE.
The availability of audio data on sound sharing platforms such as Freesound gives users access to large amounts of annotated audio. Utilising such data for training is becoming increasingly popular, but the problem of label noise that is often prevalent in such datasets requires further investigation. This paper introduces ARCA23K, an Automatically Retrieved and Curated Audio dataset comprised of over 23 000 labelled Freesound clips. Unlike past datasets such as FSDKaggle2018 and FSDnoisy18K, ARCA23K facilitates the study of label noise in a more controlled manner. We describe the entire process of creating the dataset such that it is fully reproducible, meaning researchers can extend our work with little effort. We show that the majority of labelling errors in ARCA23K are due to out-of-vocabulary audio clips, and we refer to this type of label noise as open-set label noise. Experiments are carried out in which we study the impact of label noise in terms of classification performance and representation learning.
Dictionary learning has been extensively studied in sparse representations. However, existing dictionary learning algorithms are developed mainly for standard matrices (i.e. matrices with scalar elements), and little attention has been paid to polynomial matrices, despite their wide use for describing convolutive signals or for modeling acoustic channels in room and underwater acoustics. In this paper, we propose a polynomial dictionary learning technique to deal with signals with time delays. We present two types of polynomial dictionary learning methods based on the fact that a polynomial matrix can be represented either as a polynomial of matrices (i.e. the coefficient in the polynomial corresponding to each time lag is a scalar matrix) or equally as a matrix of polynomial elements (i.e. each element of the matrix is a polynomial). The first method allows one to extend any state-of-the-art dictionary learning method to the polynomial case; and the second method allows one to directly process the polynomial matrix without having to access its coefficient matrices. A sparse coding method is also presented for reconstructing convolutive signals based on a polynomial dictionary. Simulations are provided to demonstrate the performance of the proposed algorithms, e.g. for polynomial signal reconstruction from noisy measurements.
In this paper the mixing vector (MV) in the statistical mixing model is compared to the binaural cues represented by interaural level and phase differences (ILD and IPD). It is shown that the MV distributions are quite distinct while binaural models overlap when the sources are close to each other. On the other hand, the binaural cues are more robust to high reverberation than MV models. According to this complementary behavior we introduce a new robust algorithm for stereo speech separation which considers both additive and convolutive noise signals to model the MV and binaural cues in parallel and estimate probabilistic time-frequency masks. The contribution of each cue to the final decision is also adjusted by weighting the log-likelihoods of the cues empirically. Furthermore, the permutation problem of the frequency domain blind source separation (BSS) is addressed by initializing the MVs based on binaural cues. Experiments are performed systematically on determined and underdetermined speech mixtures in five rooms with various acoustic properties including anechoic, highly reverberant, and spatially-diffuse noise conditions. The results in terms of signal-to-distortion-ratio (SDR) confirm the benefits of integrating the MV and binaural cues, as compared with two state-of-the-art baseline algorithms which only use MV or the binaural cues.
Multiple instance learning (MIL) with convolutional neural networks (CNNs) has been proposed recently for weakly labelled audio tagging. However, features from the various CNN filtering channels and spatial regions are often treated equally, which may limit its performance in event prediction. In this paper, we propose a novel attention mechanism, namely, spatial and channel-wise attention (SCA). For spatial attention, we divide it into global and local submodules with the former to capture the event-related spatial regions and the latter to estimate the onset and offset of the events. Considering the variations in CNN channels, channel-wise attention is also exploited to recognize different sound scenes. The proposed SCA can be employed into any CNNs seamlessly with affordable overheads and is end-to-end trainable fashion. Extensive experiments on weakly labelled dataset Audioset show that the proposed SCA with CNNs achieves a state-of-the-art mean average precision (mAP) of 0.390.
Algorithms aiming at solving dictionary learning problem usually involve iteratively performing two stage operations: sparse coding and dictionary update. In the dictionary update stage, codewords are updated based on a given sparsity pattern. In the ideal case where there is no noise and the true sparsity pattern is known a priori, dictionary update should produce a dictionary that precisely represent the training samples. However, we analytically show that benchmark algorithms, including MOD, K-SVD and regularized SimCO, could not always guarantee this property: they may fail to converge to a global minimum. The key behind the failure is the singularity in the objective function. To address this problem, we propose a weighted technique based on the SimCO optimization framework, hence the term weighted SimCO. Decompose the overall objective function as a sum of atomic functions. The crux of weighted SimCO is to apply weighting coefficients to atomic functions so that singular points are zeroed out. A second order method is implemented to solve the corresponding optimization problem. We numerically compare the proposed algorithm with the benchmark algorithms for noiseless and noisy scenarios. The empirical results demonstrate the significant improvement in the performance.
In pervasive environments, availability and reliability of a service cannot always be guaranteed. In such environments, automatic and dynamic mechanisms are required to compose services or compensate for a service that becomes unavailable during the runtime. Most of the existing works on services composition do not provide sufficient support for automatic service provisioning in pervasive environments. We propose a Divide and Conquer algorithm that can be used at the service runtime to repeatedly divide a service composition request into several simpler sub-requests. The algorithm repeats until for each sub-request we find at least one atomic service that meets the requirements of that sub-request. The identified atomic services can then be used to create a composite service. We discuss the technical details of our approach and show evaluation results based on a set of composite service requests. The results show that our proposed method performs effectively in decomposing a composite service requests to a number of sub-requests and finding and matching service components that can fulfill the service composition request.
Sound event detection (SED) is a task to detect sound events in an audio recording. One challenge of the SED task is that many datasets such as the Detection and Classification of Acoustic Scenes and Events (DCASE) datasets are weakly labelled. That is, there are only audio tags for each audio clip without the onset and offset times of sound events. We compare segment-wise and clip-wise training for SED that is lacking in previous works. We propose a convolutional neural network transformer (CNN-Transfomer) for audio tagging and SED, and show that CNN-Transformer performs similarly to a convolutional recurrent neural network (CRNN). Another challenge of SED is that thresholds are required for detecting sound events. Previous works set thresholds empirically, and are not an optimal approaches. To solve this problem, we propose an automatic threshold optimization method. The first stage is to optimize the system with respect to metrics that do not depend on thresholds, such as mean average precision (mAP). The second stage is to optimize the thresholds with respect to metrics that depends on those thresholds. Our proposed automatic threshold optimization system achieves a state-of-the-art audio tagging F1 of 0.646, outperforming that without threshold optimization of 0.629, and a sound event detection F1 of 0.584, outperforming that without threshold optimization of 0.564.
Deep neural networks (DNNs) have been used for dereverberation and separation in the monaural source separation problem. However, the performance of current state-ofthe-art methods is limited, particularly when applied in highly reverberant room environments. In this paper, we propose a twostage approach with two DNN-based methods to address this problem. In the first stage, the dereverberation of the speech mixture is achieved with the proposed dereverberation mask (DM). In the second stage, the dereverberant speech mixture is separated with the ideal ratio mask (IRM). To realize this two-stage approach, in the first DNN-based method, the DM is integrated with the IRM to generate the enhanced time-frequency (T-F) mask, namely the ideal enhanced mask (IEM), as the training target for the single DNN. In the second DNN-based method, the DM and the IRM are predicted with two individual DNNs. The IEEE and the TIMIT corpora with real room impulse responses (RIRs) and noise from the NOISEX dataset are used to generate speech mixtures for evaluations. The proposed methods outperform the state-of-the-art specifically in highly reverberant room environments.
The problem of tracking multiple moving speakers in indoor environments has received much attention. Earlier techniques were based purely on a single modality, e.g., vision. Recently, the fusion of multi-modal information has been shown to be instrumental in improving tracking performance, as well as robustness in the case of challenging situations like occlusions (by the limited field of view of cameras or by other speakers). However, data fusion algorithms often suffer from noise corrupting the sensor measurements which cause non-negligible detection errors. Here, a novel approach to combining audio and visual data is proposed. We employ the direction of arrival angles of the audio sources to reshape the typical Gaussian noise distribution of particles in the propagation step and to weight the observation model in the measurement step. This approach is further improved by solving a typical problem associated with the PF, whose efficiency and accuracy usually depend on the number of particles and noise variance used in state estimation and particle propagation. Both parameters are specified beforehand and kept fixed in the regular PF implementation which makes the tracker unstable in practice. To address these problems, we design an algorithm which adapts both the number of particles and noise variance based on tracking error and the area occupied by the particles in the image. Experiments on the AV16.3 dataset show the advantage of our proposed methods over the baseline PF method and an existing adaptive PF algorithm for tracking occluded speakers with a significantly reduced number of particles.
Audio source separation aims to extract individual sources from mixtures of multiple sound sources. Many techniques have been developed such as independent compo- nent analysis, computational auditory scene analysis, and non-negative matrix factorisa- tion. A method based on Deep Neural Networks (DNNs) and time-frequency (T-F) mask- ing has been recently developed for binaural audio source separation. In this method, the DNNs are used to predict the Direction Of Arrival (DOA) of the audio sources with respect to the listener which is then used to generate soft T-F masks for the recovery/estimation of the individual audio sources.
A novel multimodal (audio-visual) approach to the problem of blind source separation (BSS) is evaluated in room environments. The main challenges of BSS in realistic environments are: 1) sources are moving in complex motions and 2) the room impulse responses are long. For moving sources the unmixing filters to separate the audio signals are difficult to calculate from only statistical information available from a limited number of audio samples. For physically stationary sources measured in rooms with long impulse responses, the performance of audio only BSS methods is limited. Therefore, visual modality is utilized to facilitate the separation. The movement of the sources is detected with a 3-D tracker based on a Markov Chain Monte Carlo particle filter (MCMC-PF), and the direction of arrival information of the sources to the microphone array is estimated. A robust least squares frequency invariant data independent (RLSFIDI) beamformer is implemented to perform real time speech enhancement. The uncertainties in source localization and direction of arrival information are also controlled by using a convex optimization approach in the beamformer design. A 16 element circular array configuration is used. Simulation studies based on objective and subjective measures confirm the advantage of beamforming based processing over conventional BSS methods. © 2011 EURASIP.
Blind source separation (BSS) has attracted dramatic research interests in the past decade due to its potential applications in signal processing, telecommunications, and medical imaging. Among the open issues in BSS is how to recover the source signals from the linear convolutive mixtures which are observed by an array of sensors, and this remains a challenging problem. An effective solution is to transform the convolutive model into the frequency domain so that a series of complex-valued instantaneous BSS can be applied independently to each frequency bin. This has simplified the separation problem with a better convergence performance. However, a crucial problem, called the permutation problem, should be solved before gaining a good separation performance. This talk gives an outline of our approach to the frequency domain BSS with emphasis on the solutions to the permutation problem. Some recent results, together with a comparative discussion of the state-of-the-art approaches will be presented.
Edge computing is a viable paradigm for supporting the Industrial Internet of Things deployment by shifting computationally demanding tasks from resource-constrained devices to powerful edge servers. In this study, mobile edge computing (MEC) services are provided for multiple ground mobile nodes (MNs) through a time-division multiple access protocol using the unmanned aerial vehicle (UAV)-enabled edge servers. Remotely controlled UAVs can serve as MEC servers due to their adaptability and flexibility. However, the current MEC approaches have proven ineffective in situations where the number of MNs rapidly increases, or network resources are sparsely distributed. Furthermore, suitable accessibility across wireless networks via MNs with an acceptable quality of service is a fundamental problem for conventional UAV-assisted communications. To tackle this issue, we present an optimized computation resource allocation model using cooperative evolutionary computation to solve the joint optimization problem of queuebased computation offloading and adaptive computing resource allocation. The developed method ensures the task computation delay of all MNs within a time block, optimizes the sum of MN’s accessibility rates, and reduces the energy consumption of the UAV and MNs while meeting task computation restrictions. Moreover, we propose a multilayer data flow processing system to make full use of the computational capability across the system. The top layer of the system contains the cloud centre, the middle layer contains the UAV-assisted MEC (U-MEC) servers, and the bottom layer contains the mobile devices. Our numerical analysis and simulation results prove that the proposed scheme outperforms conventional techniques such as equal offloading time allocation and straight-line flight.
Generative adversarial networks (GANs) and Conditional GANs (cGANs) have recently been applied for singing voice extraction (SVE), since they can accurately model the vocal distributions and effectively utilize a large amount of unlabelled datasets. However, current GANs/cGANs based SVE frameworks have no explicit mechanism to eliminate the mutual interferences between different sources. In this work, we introduce a novel 'crossfire' criterion into GANs to complement its standard adversarial training, which forms a dual-objective GANs, namely Crossfire GANs (Cr-GANs). In addition, we design a Generalized Projection Method (GPM) for cGANs based frameworks to extract more effective conditional information for SVE. Using the proposed GPM, we extend our Cr-GANs to conditional version, i.e., Crossfire Conditional GANs (Cr-cGANs). The proposed methods were evaluated on the DSD100 and CCMixter datasets. The numerical results have shown that the 'crossfire' criterion and GPM are beneficial to each other and considerably improve the separation performance of existing GANs/cGANs based SVE methods.
Audio captioning aims to automatically generate a natural language description of an audio clip. Most captioning models follow an encoder-decoder architecture, where the decoder predicts words based on the audio features extracted by the encoder. Convolutional neural networks (CNNs) and recurrent neural networks (RNNs) are often used as the audio encoder. However, CNNs can be limited in modelling temporal relationships among the time frames in an audio signal, while RNNs can be limited in modelling the long-range dependencies among the time frames. In this paper, we propose an Audio Captioning Transformer (ACT), which is a full Transformer network based on an encoder-decoder architecture and is totally convolution-free. The proposed method has a better ability to model the global information within an audio signal as well as capture temporal relationships between audio events. We evaluate our model on AudioCaps, which is the largest audio captioning dataset publicly available. Our model shows competitive performance compared to other state-of-the-art approaches.
The transformer based model (e.g., FusingTF) has been employed recently for Electrocardiogram (ECG) signal classification. However, the high-dimensional embedding obtained via 1-D convolution and positional encoding can lead to the loss of the signal’s own temporal information and a large amount of training parameters. In this paper, we propose a new method for ECG classification, called low-dimensional denoising embedding transformer (LDTF), which contains two components, i.e., low-dimensional denoising embedding (LDE) and transformer learning. In the LDE component, a low-dimensional representation of the signal is obtained in the time-frequency domain while preserving its own temporal information. And with the low-dimensional embedding, the transformer learning is then used to obtain a deeper and narrower structure with fewer training parameters than that of the FusingTF. Experiments conducted on the MIT-BIH dataset demonstrates the effectiveness and the superior performance of our proposed method, as compared with state-of-the-art methods.
Weakly labelled audio tagging aims to predict the classes of sound events within an audio clip, where the onset and offset times of the sound events are not provided. Previous works have used the multiple instance learning (MIL) framework, and exploited the information of the whole audio clip by MIL pooling functions. However, the detailed information of sound events such as their durations may not be considered under this framework. To address this issue, we propose a novel two-stream framework for audio tagging by exploiting the global and local information of sound events. The global stream aims to analyze the whole audio clip in order to capture the local clips that need to be attended using a class-wise selection module. These clips are then fed to the local stream to exploit the detailed information for a better decision. Experimental results on the AudioSet show that our proposed method can significantly improve the performance of audio tagging under different baseline network architectures.
Image captioning aims to generate a description of visual contents with natural language automatically. This is useful in several potential applications, such as image understanding and virtual assistants. With recent advances in deep neural networks, natural and semantic text generation has been improved in image captioning. However, maintaining the gradient flow between neurons in consecutive layers becomes challenging as the network gets deeper. In this paper, we propose to integrate an auxiliary classifier in the residual recurrent neural network, which enables the gradient flow to reach the bottom layers for enhanced caption generation. Experiments on the MSCOCO and VizWiz datasets demonstrate the advantage of our proposed approach over the state-of-the-art approaches in several performance metrics.
Large Language Models (LLMs) have shown great promise in integrating diverse expert models to tackle intricate language and vision tasks. Despite their significance in advancing the field of Artificial Intelligence Generated Content (AIGC), their potential in intelligent audio content creation remains unexplored. In this work, we tackle the problem of creating audio content with storylines encompassing speech, music, and sound effects, guided by text instructions. We present WavJourney, a system that leverages LLMs to connect various audio models for audio content generation. Given a text description of an auditory scene, WavJourney first prompts LLMs to generate a structured script dedicated to audio storytelling. The audio script incorporates diverse audio elements, organized based on their spatio-temporal relationships. As a conceptual representation of audio, the audio script provides an interactive and interpretable rationale for human engagement. Afterward, the audio script is fed into a script compiler, converting it into a computer program. Each line of the program calls a task-specific audio generation model or computational operation function (e.g., concatenate, mix). The computer program is then executed to obtain an explainable solution for audio generation. We demonstrate the practicality of WavJourney across diverse real-world scenarios, including science fiction, education, and radio play. The explainable and interactive design of WavJourney fosters human-machine co-creation in multi-round dialogues, enhancing creative control and adaptability in audio production. WavJourney audiolizes the human imagination, opening up new avenues for creativity in multimedia content creation.
Sound events in daily life carry rich information about the objective world. The composition of these sounds affects the mood of people in a soundscape. Most previous approaches only focus on classifying and detecting audio events and scenes, but may ignore their perceptual quality that may impact humans' listening mood for the environment, e.g. annoyance. To this end, this paper proposes a novel hierarchical graph representation learning (HGRL) approach which links objective audio events (AE) with subjective annoyance ratings (AR) of the soundscape perceived by humans. The hierarchical graph consists of fine-grained event (fAE) embeddings with single-class event semantics, coarse-grained event (cAE) embeddings with multi-class event semantics, and AR embeddings. Experiments show the proposed HGRL successfully integrates AE with AR for AEC and ARP tasks, while coordinating the relations between cAE and fAE and further aligning the two different grains of AE information with the AR.
Contrastive language-audio pretraining (CLAP) has been developed to align the representations of audio and language, achieving remarkable performance in retrieval and classification tasks. However, current CLAP struggles to capture temporal information within audio and text features, presenting substantial limitations for tasks such as audio retrieval and generation. To address this gap, we introduce T-CLAP, a temporal-enhanced CLAP model. We use Large Language Models (LLMs) and mixed-up strategies to generate temporal-contrastive captions for audio clips from extensive audio-text datasets. Subsequently, a new temporal-focused contrastive loss is designed to fine-tune the CLAP model by incorporating these synthetic data. We conduct comprehensive experiments and analysis in multiple downstream tasks. T-CLAP shows improved capability in capturing the temporal relationship of sound events and outperforms state-of-the-art models by a significant margin. Our code and models will be released soon.
"This book covers advances in algorithmic developments, theoretical frameworks, andexperimental research findings to assist professionals who want an improved ...
Transformers, which were originally developed for natural language processing, have recently generated significant interest in the computer vision and audio communities due to their flexibility in learning long-range relationships. Constrained by the data hungry nature of transformers and the limited amount of labelled data, most transformer-based models for audio tasks are finetuned from ImageNet pretrained models, despite the huge gap between the domain of natural images and audio. This has motivated the research in self-supervised pretraining of audio transformers, which reduces the dependency on large amounts of labeled data and focuses on extracting concise representations of audio spectrograms. In this paper, we propose L ocal- G lobal A udio S pectrogram v I sion T ransformer, namely ASiT, a novel self-supervised learning framework that captures local and global contextual information by employing group masked model learning and self-distillation. We evaluate our pretrained models on both audio and speech classification tasks, including audio event classification, keyword spotting, and speaker identification. We further conduct comprehensive ablation studies, including evaluations of different pretraining strategies. The proposed ASiT framework significantly boosts the performance on all tasks and sets a new state-of-the-art performance in five audio and speech classification tasks, outperforming recent methods, including the approaches that use additional datasets for pretraining.
Audio captioning aims to generate text descriptions of audio clips. In the real world, many objects produce similar sounds. How to accurately recognize ambiguous sounds is a major challenge for audio captioning. In this work, inspired by inherent human multimodal perception, we propose visually-aware audio captioning, which makes use of visual information to help the description of ambiguous sounding objects. Specifically , we introduce an off-the-shelf visual encoder to extract video features and incorporate the visual features into an audio captioning system. Furthermore, to better exploit complementary audiovisual contexts, we propose an audiovisual attention mechanism that adaptively integrates audio and visual context and removes the redundant information in the latent space. Experimental results on AudioCaps, the largest audio captioning dataset, show that our proposed method achieves state-of-the-art results on machine translation metrics.
Automated audio captioning (AAC) which generates textual descriptions of audio content. Existing AAC models achieve good results but only use the high-dimensional representation of the encoder. There is always insufficient information learning of high-dimensional methods owing to high-dimensional representations having a large amount of information. In this paper, a new encoder-decoder model called the Low-and High-Dimensional Feature Fusion (LHDFF) is proposed. LHDFF uses a new PANNs encoder called Residual PANNs (RPANNs) to fuse low-and high-dimensional features. Low-dimensional features contain limited information about specific audio scenes. The fusion of low-and high-dimensional features can improve model performance by repeatedly emphasizing specific audio scene information. To fully exploit the fused features, LHDFF uses a dual transformer decoder structure to generate captions in parallel. Experimental results show that LHDFF outperforms existing audio captioning models.
"This book covers advances in algorithmic developments, theoretical frameworks, andexperimental research findings to assist professionals who want an improved ...
Blind speech deconvolution aims to estimate both the source speech and acoustic channel from the convolutive reverberant speech. The problem is ill-posed and underdetermined, which often requires prior knowledge for the estimation of the source and channel. In this paper, we propose a blind speech deconvolution method via a pretrained polynomial dictionary and sparse representation. A polynomial dictionary learning technique is employed to learn the dictionary from room impulse responses, which is then used as prior information to estimate the source and the acoustic impulse responses via an alternating optimization strategy. Simulations are provided to demonstrate the performance of the proposed method.
This paper investigates the Audio Set classification. Audio Set is a large scale weakly labelled dataset (WLD) of audio clips. In WLD only the presence of a label is known, without knowing the happening time of the labels. We propose an attention model to solve this WLD problem and explain the attention model from a novel probabilistic perspective. Each audio clip in Audio Set consists of a collection of features. We call each feature as an instance and the collection as a bag following the terminology in multiple instance learning. In the attention model, each instance in the bag has a trainable probability measure for each class. The classification of the bag is the expectation of the classification output of the instances in the bag with respect to the learned probability measure. Experiments show that the proposed attention model achieves a mAP of 0.327 on Audio Set, outperforming the Google’s baseline of 0.314.
In this paper, we propose a novel algorithm for the separation of convolutive speech mixtures using two-microphone recordings, based on the combination of independent component analysis (ICA) and ideal binary mask (IBM), together with a post-filtering process in the cepstral domain. Essentially, the proposed algorithm consists of three steps. First, a constrained convolutive ICA algorithm is applied to separate the source signals from two-microphone recordings. In the second step, we estimate the IBM by comparing the energy of corresponding time-frequency (T-F) units from the separated sources obtained with the convolutive ICA algorithm. The last step is to reduce musical noise caused typically by T-F masking using cepstral smoothing. The performance of the proposed approach is evaluated based on both reverberant mixtures generated using a simulated room model and real recordings. The proposed algorithm offers considerably higher efficiency, together with improved speech quality while producing similar separation performance as compared with a recent approach.
Blind source separation (BSS) aims to estimate unknown sources from their mixtures. Methods to address this include the benchmark ICA, SCA, MMCA, and more recently, a dictionary learning based algorithm BMMCA. In this paper, we solve the separation problem by using the recently proposed SimCO optimization framework. Our approach not only allows to unify the two sub-problems emerging in the separation problem, but also mitigates the singularity issue which was reported in the dictionary learning literature. Another unique feature is that only one dictionary is used to sparsely represent the source signals while in the literature typically multiple dictionaries are assumed (one dictionary per source). Numerical experiments are performed and the results show that our scheme significantly improves the performance, especially in terms of the accuracy of the mixing matrix estimation. © 2013 IEEE.
Sequential Monte Carlo probability hypothesis density (SMC- PHD) filtering has been recently exploited for audio-visual (AV) based tracking of multiple speakers, where audio data are used to inform the particle distribution and propagation in the visual SMC-PHD filter. However, the performance of the AV-SMC-PHD filter can be affected by the mismatch between the proposal and the posterior distribution. In this paper, we present a new method to improve the particle distribution where audio information (i.e. DOA angles derived from microphone array measurements) is used to detect new born particles and visual information (i.e. histograms) is used to modify the particles with particle flow (PF). Using particle flow has the benefit of migrating particles smoothly from the prior to the posterior distribution. We compare the proposed algorithm with the baseline AV-SMC-PHD algorithm using experiments on the AV16.3 dataset with multi-speaker sequences.
In-phase and quadrature-phase (I/Q) imbalance is a critical issue limit the achievable operating signal-to-noise ratio (SNR) at the receiver in direct conversion architecture. In recent literatures, the second-and fourth-order circularity property of communication signals have been used for designing compensator to eliminate the I/Q imbalance. In this paper, we investigate whether moment circularity of an order higher than four can be used in receiver I/Q imbalance compensation. It is shown that the sixth-order moment E[z4z*2] is a suitable statistic for measuring the sixth-order circularity of representative communication signals such as M-QAM and M-PSK with M > 2. Two blind algorithms are then proposed to update the coefficients of I/Q imbalance compensator by restoring the sixth-order circularity of the compensator output signal. Simulation results show that the new proposed methods based on sixth-order statistic converges faster or gives lower steady-state variance than the reference methods that are based on second-and fourth-order statistics.
We study the problem of dictionary learning for signals that can be represented as polynomials or polynomial matrices, such as convolutive signals with time delays or acoustic impulse responses. Recently, we developed a method for polynomial dictionary learning based on the fact that a polynomial matrix can be expressed as a polynomial with matrix coefficients, where the coefficient of the polynomial at each time lag is a scalar matrix. However, a polynomial matrix can be also equally represented as a matrix with polynomial elements. In this paper, we develop an alternative method for learning a polynomial dictionary and a sparse representation method for polynomial signal reconstruction based on this model. The proposed methods can be used directly to operate on the polynomial matrix without having to access its coefficients matrices. We demonstrate the performance of the proposed method for acoustic impulse response modeling.
Perceptual measurements have typically been recognized as the most reliable measurements in assessing perceived levels of reverberation. In this paper, a combination of blind RT60 estimation method and a binaural, nonlinear auditory model is employed to derive signal-based measures (features) that are then utilized in predicting the perceived level of reverberation. Such measures lack the excess of effort necessary for calculating perceptual measures; not to mention the variations in either stimuli or assessors that may cause such measures to be statistically insignificant. As a result, the automatic extraction of objective measurements that can be applied to predict the perceived level of reverberation become of vital significance. Consequently, this work is aimed at discovering measurements such as clarity, reverberance, and RT60 which can automatically be derived directly from audio data. These measurements along with labels from human listening tests are then forwarded to a machine learning system seeking to build a model to estimate the perceived level of reverberation, which is labeled by an expert, autonomously. The data has been labeled by an expert human listener for a unilateral set of files from arbitrary audio source types. By examining the results, it can be observed that the automatically extracted features can aid in estimating the perceptual rates.
Over the last decade, the explosive increase in demand of high-data-rate video services and massive access machine type communication (MTC) requests have become the main challenges for the future 5G wireless network. The hybrid satellite terrestrial network based on the control and user plane (C/U) separation concept is expected to support flexible and customized resource scheduling and management toward global ubiquitous networking and unified service architecture. In this paper, centralized and distributed resource management strategies (CRMS and DRMS) are proposed and compared com- prehensively in terms of throughput, power consumption, spectral and energy efficiency (SE and EE) and coverage probability, utilizing the mature stochastic geometry. Numerical results show that, compared with DRMS strategy, the U-plane cooperation between satellite and terrestrial network under CRMS strategy could improve the throughput and EE by nearly 136% and 60% respectively in ultra-sparse networks and greatly enhance the U-plane coverage probability (approximately 77%). Efficient resource management mechanism is suggested for the hybrid network according to the network deployment for the future 5G wireless network.
Automatic and fast tagging of natural sounds in audio collections is a very challenging task due to wide acoustic variations, the large number of possible tags, the incomplete and ambiguous tags provided by different labellers. To handle these problems, we use a co-regularization approach to learn a pair of classifiers on sound and text. The first classifier maps low-level audio features to a true tag list. The second classifier maps actively corrupted tags to the true tags, reducing incorrect mappings caused by low-level acoustic variations in the first classifier, and to augment the tags with additional relevant tags. Training the classifiers is implemented using marginal co-regularization, pair of which draws the two classifiers into agreement by a joint optimization. We evaluate this approach on two sound datasets, Freefield1010 and Task4 of DCASE2016. The results obtained show that marginal co-regularization outperforms the baseline GMM in both ef- ficiency and effectiveness.
Situated in the domain of urban sound scene classification by humans and machines, this research is the first step towards mapping urban noise pollution experienced indoors and finding ways to reduce its negative impact in peoples’ homes. We have recorded a sound dataset, called Open-Window, which contains recordings from three different locations and four different window states; two stationary states (open and close) and two transitional states (open to close and close to open). We have then built our machine recognition base lines for different scenarios (open set versus closed set) using a deep learning framework. The human listening test is also performed to be able to compare the human and machine performance for detecting the window state just using the acoustic cues. Our experimental results reveal that when using a simple machine baseline system, humans and machines are achieving similar average performance for closed set experiments.
Deep neural networks have recently achieved break-throughs in sound generation. Despite the outstanding sample quality, current sound generation models face issues on small-scale datasets (e.g., overfitting), significantly limiting performance. In this paper, we make the first attempt to investigate the benefits of pre-training on sound generation with AudioLDM, the cutting-edge model for audio generation, as the backbone. Our study demonstrates the advantages of the pre-trained AudioLDM, especially in data-scarcity scenarios. In addition, the baselines and evaluation protocol for sound generation systems are not consistent enough to compare different studies directly. Aiming to facilitate further study on sound generation tasks, we benchmark the sound generation task on various frequently-used datasets. We hope our results on transfer learning and benchmarks can provide references for further research on conditional sound generation.
Despite being studied extensively, the performance of blind source separation (BSS) is still limited especially for the sensor data collected in adverse environments. Recent studies show that such an issue can be mitigated by incorporating multimodal information into the BSS process. In this paper, we propose a method for the enhancement of the target speech separated by a BSS algorithm from sound mixtures, using visual voice activity detection (VAD) and spectral subtraction. First, a classifier for visual VAD is formed in the off-line training stage, using labelled features extracted from the visual stimuli. Then we use this visual VAD classifier to detect the voice activity of the target speech. Finally we apply a multi-band spectral subtraction algorithm to enhance the BSS-separated speech signal based on the detected voice activity. We have tested our algorithm on the mixtures generated artificially by the mixing filters with different reverberation times, and the results show that our algorithm improves the quality of the separated target signal. © 2011 IEEE.
Sound event detection (SED) and localization refer to recognizing sound events and estimating their spatial and temporal locations. Using neural networks has become the prevailing method for SED. In the area of sound localization, which is usually performed by estimating the direction of arrival (DOA), learning-based methods have recently been developed. In this paper, it is experimentally shown that the trained SED model is able to contribute to the direction of arrival estimation (DOAE). However, joint training of SED and DOAE degrades the performance of both. Based on these results, a two-stage polyphonic sound event detection and localization method is proposed. The method learns SED first, after which the learned feature layers are transferred for DOAE. It then uses the SED ground truth as a mask to train DOAE. The proposed method is evaluated on the DCASE 2019 Task 3 dataset, which contains different overlapping sound events in different environments. Experimental results show that the proposed method is able to improve the performance of both SED and DOAE, and also performs significantly better than the baseline method.
Audio super-resolution is a fundamental task that predicts high-frequency components for low-resolution audio, enhancing audio quality in digital applications. Previous methods have limitations such as the limited scope of audio types (e.g., music, speech) and specific bandwidth settings they can handle (e.g., 4kHz to 8kHz). In this paper, we introduce a diffusion-based generative model, AudioSR, that is capable of performing robust audio super-resolution on versatile audio types, including sound effects, music, and speech. Specifically, AudioSR can upsample any input audio signal within the bandwidth range of 2kHz to 16kHz to a high-resolution audio signal at 24kHz bandwidth with a sampling rate of 48kHz. Extensive objective evaluation on various audio super-resolution benchmarks demonstrates the strong result achieved by the proposed model. In addition, our subjective evaluation shows that AudioSR can acts as a plug-and-play module to enhance the generation quality of a wide range of audio generative models, including AudioLDM, Fastspeech2, and MusicGen. Our code and demo are available at https://audioldm.github.io/audiosr.
Audio tagging is the task of predicting the presence or absence of sound classes within an audio clip. Previous work in audio tagging focused on relatively small datasets limited to recognising a small number of sound classes. We investigate audio tagging on AudioSet, which is a dataset consisting of over 2 million audio clips and 527 classes. AudioSet is weakly labelled, in that only the presence or absence of sound classes is known for each clip, while the onset and offset times are unknown. To address the weakly-labelled audio tagging problem, we propose attention neural networks as a way to attend the most salient parts of an audio clip. We bridge the connection between attention neural networks and multiple instance learning (MIL) methods, and propose decision-level and feature-level attention neural networks for audio tagging. We investigate attention neural networks modelled by different functions, depths and widths. Experiments on AudioSet show that the feature-level attention neural network achieves a state-of-the-art mean average precision (mAP) of 0.369, outperforming the best multiple instance learning (MIL) method of 0.317 and Google’s deep neural network baseline of 0.314. In addition, we discover that the audio tagging performance on AudioSet embedding features has a weak correlation with the number of training examples and the quality of labels of each sound class.
Source separation (SS) aims to separate individual sources from an audio recording. Sound event detection (SED) aims to detect sound events from an audio recording. We propose a joint separation-classification (JSC) model trained only on weakly labelled audio data, that is, only the tags of an audio recording are known but the time of the events are unknown. First, we propose a separation mapping from the time-frequency (T-F) representation of an audio to the T-F segmentation masks of the audio events. Second, a classification mapping is built from each T-F segmentation mask to the presence probability of each audio event. In the source separation stage, sources of audio events and time of sound events can be obtained from the T-F segmentation masks. The proposed method achieves an equal error rate (EER) of 0.14 in SED, outperforming deep neural network baseline of 0.29. Source separation SDR of 8.08 dB is obtained by using global weighted rank pooling (GWRP) as probability mapping, outperforming the global max pooling (GMP) based probability mapping giving SDR at 0.03 dB. Source code of our work is published.
Semantic modelling provides a potential basis for interoperating among different systems and applications in the Internet of Things (IoT). However, current work has mostly focused on IoT resource management while not on the access and utilisation of information generated by the “Things”. We present the design of a comprehensive and lightweight semantic description model for knowledge representation in the IoT domain. The design follows the widely recognised best practices in knowledge engineering and ontology modelling. Users are allowed to extend the model by linking to external ontologies, knowledge bases or existing linked data. Scalable access to IoT services and resources is achieved through a distributed, semantic storage design. The usefulness of the model is also illustrated through an IoT service discovery method.
In this paper, we consider the dictionary learning problem for the sparse analysis model. A novel algorithm is proposed by adapting the simultaneous codeword optimization (SimCO) algorithm, based on the sparse synthesis model, to the sparse analysis model. This algorithm assumes that the analysis dictionary contains unit ℓ2-norm atoms and learns the dictionary by optimization on manifolds. This framework allows multiple dictionary atoms to be updated simultaneously in each iteration. However, similar to several existing analysis dictionary learning algorithms, dictionaries learned by the proposed algorithm may contain similar atoms, leading to a degenerate (coherent) dictionary. To address this problem, we also consider restricting the coherence of the learned dictionary and propose Incoherent Analysis SimCO by introducing an atom decorrelation step following the update of the dictionary. We demonstrate the competitive performance of the proposed algorithms using experiments with synthetic data
Separating multiple music sources from a single channel mixture is a challenging problem. We present a new approach to this problem based on non-negative matrix factorization (NMF) and note classification, assuming that the instruments used to play the sound signals are known a priori. The spectrogram of the mixture signal is first decomposed into building components (musical notes) using an NMF algorithm. The Mel frequency cepstrum coefficients (MFCCs) of both the decomposed components and the signals in the training dataset are extracted. The mean squared errors (MSEs) between the MFCC feature space of the decomposed music component and those of the training signals are used as the similarity measures for the decomposed music notes. The notes are then labelled to the corresponding type of instruments by the K nearest neighbors (K-NN) classification algorithm based on the MSEs. Finally, the source signals are reconstructed from the classified notes and the weighting matrices obtained from the NMF algorithm. Simulations are provided to show the performance of the proposed system. © 2011 Springer-Verlag Berlin Heidelberg.
Perceptual measures are usually considered more reliable than instrumental measures for evaluating the perceived level of reverberation. However, such measures are time consuming and expensive, and, due to variations in stimuli or assessors, the resulting data is not always statistically significant. Therefore, an (objective) measure of the perceived level of reverberation becomes desirable. In this paper, we develop a new method to predict the level of reverberation from audio signals by relating the perceptual listening test results with those obtained from a machine learned model. More specifically, we compare the use of a multiple stimuli test for within and between class architectures to evaluate the perceived level of reverberation. An expert set of 16 human listeners rated the perceived level of reverberation for a same set of files from different audio source types. We then train a machine learning model using the training data gathered for the same set of files and a variety of reverberation related features extracted from the data such as reverberation time, and direct to reverberation ratio. The results suggest that the machine learned model offers an accurate prediction of the perceptual scores.
Acoustic reflector localization is an important issue in audio signal processing, with direct applications in spatial audio, scene reconstruction, and source separation. Several methods have recently been proposed to estimate the 3D positions of acoustic reflectors given room impulse responses (RIRs). In this article, we categorize these methods as “image-source reversion”, which localizes the image source before finding the reflector position, and “direct localization”, which localizes the reflector without intermediate steps. We present five new contributions. First, an onset detector, called the clustered dynamic programming projected phase-slope algorithm, is proposed to automatically extract the time of arrival for early reflections within the RIRs of a compact microphone array. Second, we propose an image-source reversion method that uses the RIRs from a single loudspeaker. It is constructed by combining an image source locator (the image source direction and range (ISDAR) algorithm), and a reflector locator (using the loudspeaker-image bisection (LIB) algorithm). Third, two variants of it, exploiting multiple loudspeakers, are proposed. Fourth, we present a direct localization method, the ellipsoid tangent sample consensus (ETSAC), exploiting ellipsoid properties to localize the reflector. Finally, systematic experiments on simulated and measured RIRs are presented, comparing the proposed methods with the state-of-the-art. ETSAC generates errors lower than the alternative methods compared through our datasets. Nevertheless, the ISDAR-LIB combination performs well and has a run time 200 times faster than ETSAC.
In this technique report, we present a bunch of methods for the task 4 of Detection and Classification of Acoustic Scenes and Events 2017 (DCASE2017) challenge. This task evaluates systems for the large-scale detection of sound events using weakly labeled training data. The data are YouTube video excerpts focusing on transportation and warnings due to their industry applications. There are two tasks, audio tagging and sound event detection from weakly labeled data. Convolutional neural network (CNN) and gated recurrent unit (GRU) based recurrent neural network (RNN) are adopted as our basic framework. We proposed a learnable gating activation function for selecting informative local features. Attention-based scheme is used for localizing the specific events in a weakly-supervised mode. A new batch-level balancing strategy is also proposed to tackle the data unbalancing problem. Fusion of posteriors from different systems are found effective to improve the performance. In a summary, we get 61% F-value for the audio tagging subtask and 0.73 error rate (ER) for the sound event detection subtask on the development set. While the official multilayer perceptron (MLP) based baseline just obtained 13.1% F-value for the audio tagging and 1.02 for the sound event detection.
We present a robust and efficient audio-visual (AV) approach to speaker tracking in a room environment. A challenging problem with visual tracking is to deal with occlusions (caused by the limited field of view of cameras or by other speakers). Another challenge is associated with the particle filtering (PF) algorithm, commonly used for visual tracking, which requires a large number of particles to ensure the distribution is well modelled. In this paper, we propose a new method of fusing audio into the PF based visual tracking. We use the direction of arrival angles (DOAs) of the audio sources to reshape the typical Gaussian noise distribution of particles in the propagation step and to weight the observation model in the measurement step. Experiments on AV16.3 datasets show the advantage of our proposed method over the baseline PF method for tracking occluded speakers with a significantly reduced number of particles. © 2013 IEEE.
Class imbalance is an important factor that affects the performance of deep learning models used for remote sensing scene classification. In this paper, we propose a random finetuning meta metric learning model (RF-MML) to address this problem. Derived from episodic training in meta metric learning, a novel strategy is proposed to train the model, which consists of two phases, i.e., random episodic training and all classes fine-tuning. By introducing randomness into the episodic training and integrating it with fine-tuning for all classes, the few-shot meta-learning paradigm can be successfully applied to class imbalanced data to improve the classification performance. Experiments are conducted to demonstrate the effectiveness of the proposed model on class imbalanced datasets, and the results show the superiority of our model, as compared with other state-of-the-art methods.
Pretrained audio neural networks (PANNs) has been successful in a range of machine audition applications. But its limitation in recognising relationships between acoustic scenes and events impacts its performance in language-based audio retrieval, which retrieves audio signals from a dataset based on natural language textual queries. This paper proposes the attention-based audio encoder to exploit contextual associations between acoustic scenes/events, using self-attention or graph attention with different loss functions for language-based audio retrieval. Our experimental results show that the proposed attention-based method outperforms most of state-of-the-art methods, with self-attention performing better than graph attention. In addition, the selection of different loss functions (i.e., NT-Xent loss or supervised contrastive loss) does not have as significant an impact on the results as the selection of the attention strategy.
In this paper, we propose a new method for underdetermined blind source separation of reverberant speech mixtures by classifying each time-frequency (T-F) point of the mixtures according to a combined variational Bayesian model of spatial cues, under sparse signal representation assumption. We model the T-F observations by a variational mixture of circularly-symmetric complex-Gaussians. The spatial cues, e.g. interaural level difference (ILD), interaural phase difference (IPD) and mixing vector cues, are modelled by a variational mixture of Gaussians. We then establish appropriate conjugate prior distributions for the parameters of all the mixtures to create a variational Bayesian framework. Using the Bayesian approach we then iteratively estimate the hyper-parameters for the prior distributions by optimizing the variational posterior distribution. The main advantage of this approach is that no prior knowledge of the number of sources is needed, and it will be automatically determined by the algorithm. The proposed approach does not suffer from overfitting problem, as opposed to the Expectation-Maximization (EM) algorithm, therefore it is not sensitive to initializations.
In the past, both theoretical work and practical implementation of particle filtering (PF) method have been extensively studied. However, its application in underwater signal processing has received much less attention. This paper intends to introduce PF approach for underwater acoustic signal processing. Particularly, we are interested in direction of arrival (DOA) estimation using PF. A detailed introduction along with this perspective is presented in this paper. Since the noise usually spreads the mainlobe of likelihood function and causes problem in subsequent particle resampling step, an exponential weighted likelihood model is developed to emphasize particles at more relevant area. Hence, the the effect due to background noise can be reduced. Real underwater acoustic data collected in SWELLEx-96 experiment are employed to demonstrate the performance of the proposed PF approaches for underwater DOA tracking. © 2013 IEEE.
The Detection and Classification of Acoustic Scenes and Events (DCASE) consists of five audio classification and sound event detectiontasks: 1)Acousticsceneclassification,2)General-purposeaudio tagging of Freesound, 3) Bird audio detection, 4) Weakly-labeled semi-supervised sound event detection and 5) Multi-channel audio classification. In this paper, we create a cross-task baseline system for all five tasks based on a convlutional neural network (CNN): a “CNN Baseline” system. We implemented CNNs with 4 layers and 8 layers originating from AlexNet and VGG from computer vision. We investigated how the performance varies from task to task with the same configuration of neural networks. Experiments show that deeper CNN with 8 layers performs better than CNN with 4 layers on all tasks except Task 1. Using CNN with 8 layers, we achieve an accuracy of 0.680 on Task 1, an accuracy of 0.895 and a mean average precision (MAP) of 0.928 on Task 2, an accuracy of 0.751 andanareaunderthecurve(AUC)of0.854onTask3,asoundevent detectionF1scoreof20.8%onTask4,andanF1scoreof87.75%on Task 5. We released the Python source code of the baseline systems under the MIT license for further research.
Significant amounts of user-generated audio content, such as sound effects, musical samples and music pieces, are uploaded to online repositories and made available under open licenses. Moreover, a constantly increasing amount of multimedia content, originally released with traditional licenses, is becoming public domain as its license expires. Nevertheless, the creative industries are not yet using much of all this content in their media productions. There is still a lack of familiarity and understanding of the legal context of all this open content, but there are also problems related with its accessibility. A big percentage of this content remains unreachable either because it is not published online or because it is not well organised and annotated. In this paper we present the Audio Commons Initiative, which is aimed at promoting the use of open audio content and at developing technologies with which to support the ecosystem composed by content repositories, production tools and users. These technologies should enable the reuse of this audio material, facilitating its integration in the production workflows used by the creative industries. This is a position paper in which we describe the core ideas behind this initiative and outline the ways in which we plan to address the challenges it poses.
A novel variable step-size sign natural gradient algorithm (VS-S-NGA) for online blind separation of independent sources is presented. A sign operator for the adaptation of the separation model is obtained from the derivation of a generalized dynamic separation model. A variable step size is also derived to better match the dynamics of the input signals and unmixing matrix. The proposed sign algorithm is appealing in practice due to its computational simplicity. Experimental results verify the superior convergence performance over conventional NGA in both stationary and nonstationary environments. © 2005 IEEE.
Automated audio captioning aims to describe audio data with captions using natural language. Existing methods often employ an encoder-decoder structure, where the attention-based decoder (e.g., Transformer decoder) is widely used and achieves state-of-the-art performance. Although this method effectively captures global information within audio data via the self-attention mechanism, it may ignore the event with short time duration, due to its limitation in capturing local information in an audio signal, leading to inaccurate prediction of captions. To address this issue, we propose a method using the pretrained audio neural networks (PANNs) as the encoder and local information assisted attention-free Transformer (LocalAFT) as the decoder. The novelty of our method is in the proposal of the LocalAFT decoder, which allows local information within an audio signal to be captured while retaining the global information. This enables the events of different duration, including short duration, to be captured for more precise caption generation. Experiments show that our method outperforms the state-of-the-art methods in Task 6 of the DCASE 2021 Challenge with the standard attention-based decoder for caption generation.
The use of audio and visual modality for speaker localization has been well studied in the literature by exploiting their complementary characteristics. However, most previous works employ the setting of static sensors mounted at fixed positions. Unlike them, in this work, we explore the ego-centric setting, where the heterogeneous sensors are embodied and could be moving with a human to facilitate speaker localization. Compared to the static scenario, the ego-centric setting is more realistic for smart-home applications e.g., a service robot. However, this also brings new challenges such as blurred images, frequent speaker disappearance from the field of view of the wearer, and occlusions. In this paper, we study egocentric audio-visual speaker DOA estimation and deal with the challenges mentioned above. Specifically, we propose a transformer-based audio-visual fusion method to estimate the relative DOA of the speaker to the wearer, and design a training strategy to mitigate the problem of the speaker disappearing from the camera's view. We also develop a new dataset for simulating the out-of-view scenarios, by creating a scene with a camera wearer walking around while a speaker is moving at the same time. The experimental results show that our proposed method offers promising performance in this new dataset in terms of tracking accuracy. Finally, we adapt the proposed method for the multi-speaker scenario. Experiments on EasyCom show the effectiveness of the proposed model for multiple speakers in real scenarios, which achieves state-of-the-art results in the sphere active speaker detection task and the wearer activity prediction task. The simulated dataset and related code are available at https://github.com/KawhiZhao/Egocentric-Audio-Visual-Speaker-Localization.
Particle flow (PF) is a method originally proposed for single target tracking, and used recently to address the weight degeneracy problem of the sequential Monte Carlo probability hypothesis density (SMC-PHD) filter for audio-visual (AV) multi-speaker tracking, where the particle flow is calculated by using only the measurements near the particle, assuming that the target is detected, as in a recent method based on non-zero particle flow (NPF), i.e. the AV-NPF-SMC-PHD filter. This, however, can be problematic when occlusion happens and the occluded speaker may not be detected. To address this issue, we propose a new method where the labels of the particles are estimated using the likelihood function, and the particle flow is calculated in terms of the selected particles with the same labels. As a result, the particles associated with detected speakers and undetected speakers are distinguished based on the particle labels. With this novel method, named as AV-LPF-SMC-PHD, the speaker states can be estimated as the weighted mean of the labelled particles, which is computationally more efficient than using a clustering method as in the AV-NPF-SMC-PHD filter. The proposed algorithm is compared systematically with several baseline tracking methods using the AV16.3, AVDIAR and CLEAR datasets, and is shown to offer improved tracking accuracy with a lower computational cost.
Although audio generation shares commonalities across different types of audio, such as speech, music, and sound effects, designing models for each type requires careful consideration of specific objectives and biases that can significantly differ from those of other types. To bring us closer to a unified perspective of audio generation, this paper proposes a framework that utilizes the same learning method for speech, music, and sound effect generation. Our framework introduces a general representation of audio, called "language of audio" (LOA). Any audio can be translated into LOA based on AudioMAE, a self-supervised pre-trained representation learning model. In the generation process, we translate any modalities into LOA by using a GPT-2 model, and we perform self-supervised audio generation learning with a latent diffusion model conditioned on LOA. The proposed framework naturally brings advantages such as in-context learning abilities and reusable self-supervised pretrained AudioMAE and latent diffusion models. Experiments on the major benchmarks of text-to-audio, text-to-music, and text-to-speech demonstrate state-of-the-art or competitive performance against previous approaches. Our code, pretrained model, and demo are available at https://audioldm.github.io/audioldm2.
The non-contact monitoring of vital signs by radar has great prospects in clinical monitoring. However, the accuracy of separated respiratory and heartbeat signals has not satisfied the clinical limits of agreement. This paper presents a study for automated separation of respiratory and heartbeat signals based on empirical wavelet transform (EWT) for multiple people. The initial boundary of the EWT was set according to the limited prior information of vital signs. Using the initial boundary, empirical wavelets with a tight frame were constructed to adaptively separate the respiratory signal, the heartbeat signal and interference due to unconscious body movement. To verify the validity of the proposed method, the vital signs of three volunteers were simultaneously measured by a stepped-frequency continuous wave ultra-wideband (UWB) radar and contact physiological sensors. Compared with the vital signs from contact sensors, the proposed method can separate the respiratory and heartbeat signals among multiple people and obtain the precise rate that satisfies clinical monitoring requirements using a UWB radar. The detection errors of respiratory and heartbeat rates by the proposed method were within ±0.3 bpm and ±2 bpm, respectively, which are much smaller than those obtained by the bandpass filtering, empirical mode decomposition (EMD) and wavelet transform (WT) methods. The proposed method is unsupervised and does not require reference signals. Moreover, the proposed method can obtain accurate respiratory and heartbeat signal rates even when the persons unconsciously move their bodies.
Transformer-based models (i.e., Fusing-TF and LDTF) have achieved state-of-the-art performance for electrocardiogram (ECG) classification. However, these models may suffer from low training efficiency due to the high model complexity associated with the attention mechanism. In this paper, we present a multi-layer perceptron (MLP) model for ECG classification by incorporating a multi-scale sampling strategy for signal embedding, namely, MS-MLP. In this method, a novel multi-scale sampling strategy is first proposed to exploit the multi-scale characteristics while maintaining the temporal information in the corresponding dimensions. Then, an MLP-Mixer structure with token-mixer and channel-mixer is employed to capture the multi-scale feature and temporal feature from the multi-scale embedding result, respectively. Because of the mixing operation and attention-free MLP structure, our proposed MS- MLP method not only provides better classification performance, but also has a lower model complexity, as compared with transformer-based methods, in terms of experiments performed on the MIT-BIH dataset.
Automated audio captioning (AAC) aims to generate textual descriptions for a given audio clip. Despite the existing AAC models obtaining promising performance, they struggle to capture intricate audio patterns due to only using a high-dimensional representation. In this paper, we propose a new encoder-decoder model for AAC, called the Pyramid Feature Fusion and Cross Context Attention Network (PFCA-Net). In PFCA-Net, the encoder is constructed using a pyramid network, facilitating the extraction of audio features across multiple scales. It achieves this by combining top-down and bottom-up connections to fuse features across scales, resulting in feature maps at various scales. In the decoder, cross-content attention is designed to fuse the different scale features which allows the propagation of information from a low-scale to a high-scale. Experimental results show that PFCA-Net achieves considerable improvement over existing models.
Automated audio captioning aims to use natural language to describe the content of audio data. This paper presents an audio captioning system with an encoder-decoder architecture, where the decoder predicts words based on audio features extracted by the encoder. To improve the proposed system, transfer learning from either an upstream audio-related task or a large in-domain dataset is introduced to mitigate the problem induced by data scarcity. Moreover, evaluation metrics are incorporated into the optimization of the model with reinforcement learning, which helps address the problem of " exposure bias " induced by " teacher forcing " training strategy and the mismatch between the evaluation metrics and the loss function. The resulting system was ranked 3rd in DCASE 2021 Task 6. Abla-tion studies are carried out to investigate how much each component in the proposed system can contribute to final performance. The results show that the proposed techniques significantly improve the scores of the evaluation metrics, however, reinforcement learning may impact adversely on the quality of the generated captions.
We consider the data-driven dictionary learning problem. The goal is to seek an over-complete dictionary from which every training signal can be best approximated by a linear combination of only a few codewords. This task is often achieved by iteratively executing two operations: sparse coding and dictionary update. The focus of this paper is on the dictionary update step, where the dictionary is optimized with a given sparsity pattern. We propose a novel framework where an arbitrary set of codewords and the corresponding sparse coefficients are simultaneously updated, hence the term simultaneous codeword optimization (SimCO). The SimCO formulation not only generalizes benchmark mechanisms MOD and K-SVD, but also allows the discovery that singular points, rather than local minima, are the major bottleneck of dictionary update. To mitigate the problem caused by the singular points, regularized SimCO is proposed. First and second order optimization procedures are designed to solve regularized SimCO. Simulations show that regularization substantially improves the performance of dictionary learning. © 1991-2012 IEEE.
Environmental Sound Classification (ESC) plays a vital role in machine auditory scene perception. Deep learning based ESC methods, such as the Dilated Convolutional Neural Network (D-CNN), have achieved the state-of-art results on public datasets. However, the D-CNN ESC model size is often larger than 100MB and is only suitable for the systems with powerful GPUs, which prevent their applications in handheld devices. In this study, we take the D-CNN ESC framework and focus on reducing the model size while maintaining the ESC performance. As a result, a lightweight D-CNN (termed as LDCNN) ESC system is developed. Our work lies on twofold. First, we propose to reduce the number of parameters in the convolution layers by factorizing a two-dimensional convolution filters (L ×W) to two separable one-dimensional convolution filters (L×1 and 1×W). Second, we propose to replace the first fully connection layer (FCL) by a Feature Sum layer (FSL) to further reduce the number of parameters. This is motivated by our finding that the features of the environmental sounds have weak absolute locality property and a global sum operation can be applied to compress the feature map. Experiments on three public datasets (ESC50, UrbanSound8K, and CICESE) show that the proposed system offers comparable classification performance but with a much smaller model size. For example, the model size of our proposed system is about 2.05MB, which is 50 times smaller than the original D-CNN model, but at a loss of only 1%-2% classification accuracy.
The multiplicative noise removal problem for a corrupted image has recently been considered under the framework of regularization based approaches, where the regularizations are typically de ned on sparse dictionaries and/or total va- riation (TV). This framework was demonstrated to be e ective. However, the sparse regularizers used so far are based overwhelmingly on the synthesis model, and the TV based regularizer may induce the stair-casing e ect in the recon- structed image. In this paper, we propose a new method using a sparse analysis model. Our formulation contains a data delity term derived from the distri- bution of the noise and two regularizers. One regularizer employs a learned analysis dictionary, and the other regularizer is an enhanced TV by introducing a parameter to control the smoothness constraint de ned on pixel-wise di er- ences. To address the resulting optimization problem, we adapt the alternating direction method of multipliers (ADMM) framework, and present a new method where a relaxation technique is developed to update the variables exibly with either image patches or the whole image, as required by the learned dictionary and the enhanced TV regularizers, respectively. Experimental results demon- strate the improved performance of the proposed method as compared with several recent baseline methods, especially for relatively high noise levels.
Convolutional neural networks (CNN) are one of the best-performing neural network architectures for environmental sound classification (ESC). Recently, temporal attention mechanisms have been used in CNN to capture the useful information from the relevant time frames for audio classification, especially for weakly labelled data where the onset and offset times of the sound events are not applied. In these methods, however, the inherent spectral characteristics and variations are not explicitly exploited when obtaining the deep features. In this paper, we propose a novel parallel temporal-spectral attention mechanism for CNN to learn discriminative sound representations, which enhances the temporal and spectral features by capturing the importance of different time frames and frequency bands. Parallel branches are constructed to allow temporal attention and spectral attention to be applied respectively in order to mitigate interference from the segments without the presence of sound events. The experiments on three environmental sound classification (ESC) datasets and two acoustic scene classification (ASC) datasets show that our method improves the classification performance and also exhibits robustness to noise.
In this paper, we propose a divide-and-conquer approach using two generative adversarial networks (GANs) to explore how a machine can draw colorful pictures (bird) using a small amount of training data. In our work, we simulate the procedure of an artist drawing a picture, where one begins with drawing objects’ contours and edges and then paints them different colors. We adopt two GAN models to process basic visual features including shape, texture and color. We use the first GAN model to generate object shape, and then paint the black and white image based on the knowledge learned using the second GAN model. We run our experiments on 600 color images. The experimental results show that the use of our approach can generate good quality synthetic images, comparable to real ones.
Target tracking is a challenging task and generally no analytical solution is available, especially for the multi-target tracking systems. To address this problem, probability hypothesis density (PHD) filter is used by propagating the PHD instead of the full multi-target posterior. Recently, the particle flow filter based on the log homotopy provides a new way for state estimation. In this paper, we propose a novel sequential Monte Carlo (SMC) implementation for the PHD filter assisted by the particle flow (PF), which is called PF-SMCPHD filter. Experimental results show that our proposed filter has higher accuracy than the SMC-PHD filter and is computationally cheaper than the Gaussian mixture PHD (GM-PHD) filter.
Panning techniques, such as vector base amplitude panning (VBAP) are a widely-used practical approach for spatial sound reproduction using multiple loudspeakers. Although limited to a relatively small listening area, they are very efficient and offer good localisation accuracy, timbral quality as well as a graceful degradation of quality outside the sweet spot. The aim of this paper is to investigate optimal sound reproduction techniques that adopt some of the advantageous properties of VBAP, such as the sparsity and the locality of the active loudspeakers for the reproduction of a single audio object. To this end, we state the task of multi-loudspeaker panning as an `1 optimization problem. We demonstrate and prove that the resulting solutions are exactly sparse. Moreover, we show the effect of adding a nonnegativity constraint on the loudspeaker gains in order to preserve the locality of the panning solution. Adding this constraint, `1- optimal panning can be formulated as a linear program. Using this representation, we prove that unique `1-optimal panning solutions incorporating a nonnegativity constraint are identical to VBAP using a Delaunay triangulation for the loudspeaker setup. Using results from linear programming and duality theory, we describe properties and special cases, such as solution ambiguity, of the VBAP solution.
Contrastive language-audio pretraining (CLAP) has become a new paradigm to learn audio concepts with audio-text pairs. CLAP models have shown unprecedented performance as zero-shot classifiers on downstream tasks. To further adapt CLAP with domain-specific knowledge, a popular method is to fine-tune its audio encoder with available labelled examples. However, this is challenging in low-shot scenarios, as the amount of annotations is limited compared to the model size. In this work, we introduce a Training-efficient (Treff) adapter to rapidly learn with a small set of examples while maintaining the capacity for zero-shot classification. First, we propose a crossattention linear model (CALM) to map a set of labelled examples and test audio to test labels. Second, we find initialising CALM as a cosine measurement improves our Treff adapter even without training. The Treff adapter outperforms metricbased methods in few-shot settings and yields competitive results to fully-supervised methods.
First-shot (FS) unsupervised anomalous sound detection (ASD) is a brand-new task introduced in DCASE 2023 Challenge Task 2, where the anomalous sounds for the target machine types are unseen in training. Existing methods often rely on the availability of normal and abnormal sound data from the target machines. However, due to the lack of anomalous sound data for the target machine types, it becomes challenging when adapting the existing ASD methods to the first-shot task. In this paper, we propose a new framework for the first-shot unsupervised ASD, where metadata-assisted audio generation is used to estimate unknown anomalies, by utilising the available machine information (i.e., metadata and sound data) to fine-tune a text-to-audio generation model for generating the anomalous sounds that contain unique acoustic characteristics accounting for each different machine types. We then use the method of Time-Weighted Frequency domain audio Representation with Gaussian Mixture Model (TWFR-GMM) as the backbone to achieve the first-shot unsupervised ASD. Our proposed FS-TWFR-GMM method achieves competitive performance amongst top systems in DCASE 2023 Challenge Task 2, while requiring only 1% model parameters for detection, as validated in our experiments.
In this paper, a novel probabilistic Bayesian tracking scheme is proposed and applied to bimodal measurements consisting of tracking results from the depth sensor and audio recordings collected using binaural microphones. We use random finite sets to cope with varying number of tracking targets. A measurement-driven birth process is integrated to quickly localize any emerging person. A new bimodal fusion method that prioritizes the most confident modality is employed. The approach was tested on real room recordings and experimental results show that the proposed combination of audio and depth outperforms individual modalities, particularly when there are multiple people talking simultaneously and when occlusions are frequent.
Humans are able to identify a large number of environmental sounds and categorise them according to high-level semantic categories, e.g. urban sounds or music. They are also capable of generalising from past experience to new sounds when applying these categories. In this paper we report on the creation of a data set that is structured according to the top-level of a taxonomy derived from human judgements and the design of an associated machine learning challenge, in which strong generalisation abilities are required to be successful. We introduce a baseline classification system, a deep convolutional network, which showed strong performance with an average accuracy on the evaluation data of 80.8%. The result is discussed in the light of two alternative explanations: An unlikely accidental category bias in the sound recordings or a more plausible true acoustic grounding of the high-level categories.
Mutual coupling, which is caused by a tight intersensor spacing in uniform linear arrays (ULAs), will, to a certain extent, affect the estimation result for source localisation. To address the problem, sparse arrays such as coprime array and nested array are considered to achieve less mutual coupling and more uniform degrees-of-freedom (DoFs) than ULAs. However, there are holes in coprime arrays leading to a decrease of uniform DoFs and in a nested array, some sensors may still be located so closely that the influence of mutual coupling between sensors remains significant. This paper proposes a new Loosely Distributed Nested Array (LoDiNA), which is designed in a three-level nested configuration and the three layers are linked end-to-end with a longer inter-element separation. It is proved that LoDiNA can generate a higher number of uniform DoFs with greater robustness against mutual coupling interference and simpler configurations, as compared to existing nested arrays. The feasibility of the proposed LoDiNA structure is demonstrated for Direction-of-Arrival (DoA) estimation for multiple stationary sources with noise.
Existing contrastive learning methods for anomalous sound detection refine the audio representation of each audio sample by using the contrast between the samples' augmentations (e.g., with time or frequency masking). However, they might be biased by the augmented data, due to the lack of physical properties of machine sound, thereby limiting the detection performance. This paper uses contrastive learning to refine audio representations for each machine ID, rather than for each audio sample. The proposed two-stage method uses contrastive learning to pretrain the audio representation model by incorporating machine ID and a self-supervised ID classifier to fine-tune the learnt model, while enhancing the relation between audio features from the same ID. Experiments show that our method outperforms the state-of-the-art methods using contrastive learning or self-supervised classification in overall anomaly detection performance and stability on DCASE 2020 Challenge Task2 dataset.
Sequential Monte Carlo probability hypothesis density (SMC- PHD) ltering has been recently exploited for audio-visual (AV) based tracking of multiple speakers, where audio data are used to inform the particle distribution and propagation in the visual SMC-PHD lter. How- ever, the performance of the AV-SMC-PHD lter can be a ected by the mismatch between the proposal and the posterior distribution. In this pa- per, we present a new method to improve the particle distribution where audio information (i.e. DOA angles derived from microphone array mea- surements) is used to detect new born particles and visual information (i.e. histograms) is used to modify the particles with particle ow (PF). Using particle ow has the bene t of migrating particles smoothly from the prior to the posterior distribution. We compare the proposed algo- rithm with the baseline AV-SMC-PHD algorithm using experiments on the AV16.3 dataset with multi-speaker sequences.
Typical methods for binaural source separation consider only the direct sound as the target signal in a mixture. However, in most scenarios, this assumption limits the source separation performance. It is well known that the early reflections interact with the direct sound, producing acoustic effects at the listening position, e.g. the so-called comb filter effect. In this article, we propose a novel source separation model, that utilizes both the direct sound and the first early reflection information to model the comb filter effect. This is done by observing the interaural phase difference obtained from the timefrequency representation of binaural mixtures. Furthermore, a method is proposed to model the interaural coherence of the signals. Including information related to the sound multipath propagation, the performance of the proposed separation method is improved with respect to the baselines that did not use such information, as illustrated by using binaural recordings made in four rooms, having different sizes and reverberation times.
This paper considers acoustic source tracking in a room environment using a distributed microphone pair network. Existing time-delay of arrival (TDOA) based approaches usually require all received signals to be transmitted to central processor and synchronized to extract the TDOA measurements. The source positions are then obtained by using a subsequent localization or tracking approach. In this paper, we propose a distributed particle filtering (PF) approach to track the source using a microphone pair network. Each node is constructed by a microphone pair and TDOA measurements are extracted at local nodes. An extended Kalman filter based PF is developed to estimate the first order and the second order statistics of the source state. A consensus filter is then applied to fuse these local statistics between neighboring nodes to achieve a global estimation. Under such an approach, only the state statistics need to be transmitted and the received signals need only to be pairwise synchronized. Consequently, both communication and computational cost can be significantly reduced. Simulations under different reverberant environments demonstrate that the proposed approach outperforms the centralized sequential importance sampling based PF approach in single source tracking as well as in non-concurrent multiple source tracking. © 2013 ISIF ( Intl Society of Information Fusi.
Convolutional neural network (CNN) based methods, such as the convolutional encoder-decoder network , offer state-of-the-art results in monaural speech enhancement. In the conventional encoder-decoder network, large kernel size is often used to enhance the model capacity, which, however, results in low parameter efficiency. This could be addressed by using group convolution, as in AlexNet, where group convolutions are performed in parallel in each layer, before their outputs are concatenated. However, with the simple concatenation, the inter-channel dependency information may be lost. To address this, the Shuffle network rearranges the outputs of each group before concatenating them, by taking part of the whole input sequence as the input to each group of convolution. In this work, we propose a new convolutional fusion network (CFN) for monaural speech enhancement by improving model performance, inter-channel dependency, information reuse and parameter efficiency. First, a new group convolutional fusion unit (GCFU) consisting of the standard and depth-wise separable CNN is used to reconstruct the signal. Second, the whole input sequence (full information) is fed simultaneously to two convolution networks in parallel, and their outputs are rearranged (shuffled) and then concatenated, in order to exploit the inter-channel dependency within the network. Third, the intra skip connection mechanism is used to connect different layers inside the encoder as well as decoder to further improve the model performance. Extensive experiments are performed to show the improved performance of the proposed method as compared with three recent baseline methods.
Recent studies show that visual information contained in visual speech can be helpful for the performance enhancement of audio-only blind source separation (BSS) algorithms. Such information is exploited through the statistical characterisation of the coherence between the audio and visual speech using, e.g. a Gaussian mixture model (GMM). In this paper, we present two new contributions. An adapted expectation maximization (AEM) algorithm is proposed in the training process to model the audio-visual coherence upon the extracted features. The coherence is exploited to solve the permutation problem in the frequency domain using a new sorting scheme. We test our algorithm on the XM2VTS multimodal database. The experimental results show that our proposed algorithm outperforms traditional audio-only BSS.
Audio-visual video parsing is the task of categorizing a video at the segment level with weak labels, and predicting them as audible or visible events. Recent methods for this task leverage the attention mechanism to capture the semantic correlations among the whole video across the audio-visual modalities. However, these approaches have overlooked the importance of individual segments within a video and the relationship among them, and tend to rely on a single modality when learning features. In this paper, we propose a novel interactive-enhanced cross-modal perception method~(CM-PIE), which can learn fine-grained features by applying a segment-based attention module. Furthermore, a cross-modal aggregation block is introduced to jointly optimize the semantic representation of audio and visual signals by enhancing inter-modal interactions. The experimental results show that our model offers improved parsing performance on the Look, Listen, and Parse dataset compared to other methods.
We address the problem of decomposing several consecutive sparse signals, such as audio time frames or image patches. A typical approach is to process each signal sequentially and independently, with an arbitrary sparsity level fixed for each signal. Here, we propose to process several frames simultaneously, allowing for more flexible sparsity patterns to be considered. We propose a multivariate sparse coding approach, where sparsity is enforced on average across several frames. We propose a Multivariate Iterative Hard Thresholding to solve this problem. The usefulness of the proposed approach is demonstrated on audio coding and denoising tasks. Experiments show that the proposed approach leads to better results when the signal contains both transients and tonal components.
Underdetermined reverberant speech separation is a challenging problem in source separation that has received considerable attention in both computational auditory scene analysis (CASA) and blind source separation (BSS). Recent studies suggest that, in general, the performance of frequency domain BSS methods suffer from the permutation problem across frequencies which degrades in high reverberation, meanwhile, CASA methods perform less effectively for closely spaced sources. This paper presents a method to address these limitations, based on the combination of binaural and BSS cues for the automatic classification of time-frequency (T-F) units of the speech mixture spectrogram. By modeling the interaural phase difference, the interaural level difference and frequency-bin mixing vectors, we integrate the coherent information for each source within a probabilistic framework. The Expectation Maximization (EM) algorithm is then used iteratively to refine the soft assignment of T-F regions to sources and re-estimate their model parameters. The coherence between the left and right recordings is also calculated to model the precedence effect which is then incorporated to the algorithm to reduce the effect of reverberation. Binaural room impulse responses for 5 different rooms with various acoustic properties have been used to generate the source images and the mixtures. The proposed method compares favorably with state-of-the-art baseline algorithms by Mandel et al. and Sawada et al., in terms of signal-to-distortion ratio (SDR) of the separated source signals.
Reverberant speech source separation has been of great interest for over a decade, leading to two major approaches. One of them is based on statistical properties of the signals and mixing process known as blind source separation (BSS). The other approach named as computational auditory scene analysis (CASA) is inspired by human auditory system and exploits monaural and binaural cues. In this paper these two approaches are studied and compared in more depth.
Continuously learning new classes without catastrophic forgetting is a challenging problem for on-device environmental sound classification given the restrictions on computation resources (e.g., model size, running memory). To address this issue, we propose a simple and efficient continual learning method. Our method selects the historical data for the training by measuring the per-sample classification uncertainty. Specifically, we measure the uncertainty by observing how the classification probability of data fluctuates against the parallel perturbations added to the classifier embedding. In this way, the computation cost can be significantly reduced compared with adding perturbation to the raw data. Experimental results on the DCASE 2019 Task 1 and ESC-50 dataset show that our proposed method outperforms baseline continual learning methods on classification accuracy and computational efficiency, indicating our method can efficiently and incrementally learn new classes without the catastrophic forgetting problem for on-device environmental sound classification.
Existing contrastive learning methods for anomalous sound detection refine the audio representation of each audio sample by using the contrast between the samples' augmentations (e.g., with time or frequency masking). However, they might be biased by the augmented data, due to the lack of physical properties of machine sound, thereby limiting the detection performance. This paper uses contrastive learning to refine audio representations for each machine ID, rather than for each audio sample. The proposed two-stage method uses contrastive learning to pretrain the audio representation model by incorporating machine ID and a self-supervised ID classi-fier to fine-tune the learnt model, while enhancing the relation between audio features from the same ID. Experiments show that our method outperforms the state-of-the-art methods using contrastive learning or self-supervised classification in overall anomaly detection performance and stability on DCASE 2020 Challenge Task2 dataset.
In real rooms, recorded speech usually contains reverberation, which degrades the quality and intelligibility of the speech. It has proven effective to use neural networks to estimate complex ideal ratio masks (cIRMs) using mean square error (MSE) loss for speech dereverberation. However, in some cases, when using MSE loss to estimate complex-valued masks, phase may have a disproportionate effect compared to magnitude. We propose a new weighted magnitude-phase loss function, which is divided into a magnitude component and a phase component, to train a neural network to estimate complex ideal ratio masks. A weight parameter is introduced to adjust the relative contribution of magnitude and phase to the overall loss. We find that our proposed loss function outperforms the regular MSE loss function for speech dereverberation.
We present a novel method for extracting target speech from auditory mixtures using bimodal coherence, which is statistically characterised by a Gaussian mixture modal (GMM) in the offline training process, using the robust features obtained from the audio-visual speech. We then adjust the ICA-separated spectral components using the bimodal coherence in the time-frequency domain, to mitigate the scale ambiguities in different frequency bins. We tested our algorithm on the XM2VTS database, and the results show the performance improvement with our proposed algorithm in terms of SIR measurements.
This study defines a new evaluation metric for audio tagging tasks to alleviate the limitation of the mean average precision (mAP) metric. The mAP metric treats different kinds of sound as independent classes without considering their relations. The proposed metric, ontology-aware mean average precision (OmAP), addresses the weaknesses of mAP by utilizing additional on-tology during evaluation. Specifically, we reweight the false positive events in the model prediction based on the AudioSet ontology graph distance to the target classes. The OmAP also provides insights into model performance by evaluating different coarse-grained levels in the ontology graph. We conduct a human assessment and show that OmAP is more consistent with human perception than mAP. We also propose an ontology-based loss function (OBCE) that reweights binary cross entropy (BCE) loss based on the ontology distance. Our experiment shows that OBCE can improve both mAP and OmAP metrics on the AudioSet tagging task.
Automated audio captioning (AAC) aims to describe the content of an audio clip using simple sentences. Existing AAC methods are developed based on an encoder-decoder architecture that success is attributed to the use of a pre-trained CNN10 called PANNs as the encoder to learn rich audio representations. AAC is a highly challenging task due to its high-dimensional talent space involves audio of various scenarios. Existing methods only use the high-dimensional representation of the PANNs as the input of the decoder. However, the low-dimension representation may retain as much audio information as the high-dimensional representation may be neglected. In addition, although the high-dimensional approach may predict the audio captions by learning from existing audio captions, which lacks robustness and efficiency. To deal with these challenges, a fusion model which integrates low- and high-dimensional features AAC framework is proposed. In this paper, a new encoder-decoder framework is proposed called the Low- and High-Dimensional Feature Fusion (LHDFF) model for AAC. Moreover, in LHDFF, a new PANNs encoder is proposed called Residual PANNs (RPANNs) by fusing the low-dimensional feature from the intermediate convolution layer output and the high-dimensional feature from the final layer output of PANNs. To fully explore the information of the low- and high-dimensional fusion feature and high-dimensional feature respectively, we proposed dual transformer decoder structures to generate the captions in parallel. Especially, a probabilistic fusion approach is proposed that can ensure the overall performance of the system is improved by concentrating on the respective advantages of the two transformer decoders. Experimental results show that LHDFF achieves the best performance on the Clotho and AudioCaps datasets compared with other existing models
Convolutional neural network (CNN) is a popular choice for visual object detection where two sub-nets are often used to achieve object classification and localization separately. However, the intrinsic relation between the localization and classification sub-nets was not exploited explicitly for object detection. In this letter, we propose a novel association loss, namely, the proxy squared error (PSE) loss, to entangle the two sub-nets, thus use the dependency between the classification and localization scores obtained from these two sub-nets to improve the detection performance. We evaluate our proposed loss on the MS-COCO dataset and compare it with the loss in a recent baseline, i.e. the fully convolutional one-stage (FCOS) detector. The results show that our method can improve the AP from 33:8 to 35:4 and AP75 from 35:4 to 37:8, as compared with the FCOS baseline.
Fractional adaptive algorithms have given rise to new dimensions in parameter estimation of control and signal processing systems. In this paper, we present novel fractional calculus based LMS algorithm with fast convergence properties and potential ability to avoid being trapped into local minima. We test our proposed algorithm for parameter estimation of power signals and compare it with other state-of-the-art fractional and standard LMS algorithms under different noisy conditions. Our proposed algorithm outperforms other LMS algorithms in terms of convergence rate and accuracy.
Automated audio captioning is a cross-modal translation task for describing the content of audio clips with natural language sentences. This task has attracted increasing attention and substantial progress has been made in recent years. Captions generated by existing models are generally faithful to the content of audio clips, however, these machine-generated captions are often deterministic (e.g., generating a fixed caption for a given audio clip), simple (e.g., using common words and simple grammar), and generic (e.g., generating the same caption for similar audio clips). When people are asked to describe the content of an audio clip, different people tend to focus on different sound events and describe an audio clip diversely from various aspects using distinct words and grammar. We believe that an audio captioning system should have the ability to generate diverse captions, either for a fixed audio clip, or across similar audio clips. To this end, we propose an adversarial training framework based on a conditional generative adversarial network (C-GAN) to improve diversity of audio captioning systems. A caption generator and two hybrid discriminators compete and are learned jointly, where the caption generator can be any standard encoder-decoder captioning model used to generate captions, and the hybrid discriminators assess the generated captions from different criteria, such as their naturalness and semantics. We conduct experiments on the Clotho dataset. The results show that our proposed model can generate captions with better diversity as compared to state-of-the-art methods.
In this paper, we present SpecAugment++, a novel data augmentation method for deep neural networks based acoustic scene classification (ASC). Different from other popular data augmentation methods such as SpecAugment and mixup that only work on the input space, SpecAugment++ is applied to both the input space and the hidden space of the deep neural networks to enhance the input and the intermediate feature representations. For an intermediate hidden state, the augmentation techniques consist of masking blocks of frequency channels and masking blocks of time frames, which improve generalization by enabling a model to attend not only to the most discrimina-tive parts of the feature, but also the entire parts. Apart from using zeros for masking, we also examine two approaches for masking based on the use of other samples within the mini-batch, which helps introduce noises to the networks to make them more discriminative for classification. The experimental results on the DCASE 2018 Task1 dataset and DCASE 2019 Task1 dataset show that our proposed method can obtain 3.6% and 4.7% accuracy gains over a strong baseline without augmentation (i.e. CP-ResNet) respectively, and outperforms other previous data augmentation methods.
Deep learning models have been used recently for target recognition from synthetic aperture radar (SAR) images. However, the performance of these models tends to deteriorate when only a small number of training samples are available due to the problem of overfitting. To address this problem, we propose a two-stage multiscale densely connected convolutional neural networks (TMDC-CNNs). In the proposed TMDC-CNNs, the overfitting issue is addressed with a novel multiscale densely connected network architecture and a two-stage loss function, which integrated the cosine similarity with the prevailing softmax cross-entropy loss. Experiments were conducted on the MSTAR data set, and the results show that our model offers significant recognition accuracy improvements as compared with other state-of-the-art methods, with severely limited training data. The source codes are available at https://github.com/Stubsx/TMDC-CNNs .
The medical domain is home to many critical challenges that stand to be overcome with the use of data-driven clinical decision support systems (CDSS), and there is a growing set of examples of automated diagnosis, prognosis, drug design, and testing. However, the current state of AI in medicine has been summarized as “high on promise and relatively low on data and proof.” If such problems can be addressed, a data-driven approach will be very important to the future of CDSSs as it simplifies the knowledge acquisition and maintenance process, a process that is time-consuming and requires considerable human effort. Diverse Perspectives and State-of-the-Art Approaches to the Utilization of Data-Driven Clinical Decision Support Systems critically reflects on the challenges that data-driven CDSSs must address to become mainstream healthcare systems rather than a small set of exemplars of what might be possible. It further identifies evidence-based, successful data-driven CDSSs. Covering topics such as automated planning, diagnostic systems, and explainable artificial intelligence, this premier reference source is an excellent resource for medical professionals, healthcare administrators, IT managers, pharmacists, students and faculty of higher education, librarians, researchers, and academicians. The medical domain is home to many critical challenges that stand to be overcome with the use of data-driven clinical decision support systems (CDSS), and there is a growing set of examples of automated diagnosis, prognosis, drug design, and testing. However, the current state of AI in medicine has been summarized as “high on promise and relatively low on data and proof.” If such problems can be addressed, a data-driven approach will be very important to the future of CDSSs as it simplifies the knowledge acquisition and maintenance process, a process that is time-consuming and requires considerable human effort. Diverse Perspectives and State-of-the-Art Approaches to the Utilization of Data-Driven Clinical Decision Support Systems critically reflects on the challenges that data-driven CDSSs must address to become mainstream healthcare systems rather than a small set of exemplars of what might be possible. It further identifies evidence-based, successful data-driven CDSSs. Covering topics such as automated planning, diagnostic systems, and explainable artificial intelligence, this premier reference source is an excellent resource for medical professionals, healthcare administrators, IT managers, pharmacists, students and faculty of higher education, librarians, researchers, and academicians.
Anomaly detection in computer vision aims to detect outliers from input image data. Examples include texture defect detection and semantic discrepancy detection. However, existing methods are limited in detecting both types of anomalies, especially for the latter. In this work, we propose a novel semantics-aware normalizing flow model to address the above challenges. First, we employ the semantic features extracted from a backbone network as the initial input of the normalizing flow model, which learns the mapping from the normal data to a normal distribution according to semantic attributes, thus enhances the discrimination of semantic anomaly detection. Second, we design a new feature fusion module in the normalizing flow model to integrate texture features and semantic features, which can substantially improve the fitting of the distribution function with input data, thus achieving improved performance for the detection of both types of anomalies. Extensive experiments on five well-known datasets for semantic anomaly detection show that the proposed method outperforms the state-of-the-art baselines. The codes will be available at https://github.com/SYLan2019/SANF-AD.
Deep generative models have recently achieved impressive performance in speech and music synthesis. However, compared to the generation of those domain-specific sounds, generating general sounds (such as siren, gunshots) has received less attention , despite their wide applications. In previous work, the SampleRNN method was considered for sound generation in the time domain. However, SampleRNN is potentially limited in capturing long-range dependencies within sounds as it only back-propagates through a limited number of samples. In this work, we propose a method for generating sounds via neural discrete time-frequency representation learning, conditioned on sound classes. This offers an advantage in efficiently modelling long-range dependencies and retaining local fine-grained structures within sound clips. We evaluate our approach on the UrbanSound8K dataset, compared to SampleRNN, with the performance metrics measuring the quality and diversity of generated sounds. Experimental results show that our method offers comparable performance in quality and significantly better performance in diversity.
Acoustic scene generation (ASG) is a task to generate waveforms for acoustic scenes. ASG can be used to generate audio scenes for movies and computer games. Recently, neural networks such as SampleRNN have been used for speech and music generation. However, ASG is more challenging due to its wide variety. In addition, evaluating a generative model is also difficult. In this paper, we propose to use a conditional SampleRNN model to generate acoustic scenes conditioned on the input classes. We also propose objective criteria to evaluate the quality and diversity of the generated samples based on classification accuracy. The experiments on the DCASE 2016 Task 1 acoustic scene data show that with the generated audio samples, a classification accuracy of 65:5% can be achieved compared to samples generated by a random model of 6:7% and samples from real recording of 83:1%. The performance of a classifier trained only on generated samples achieves an accuracy of 51:3%, as opposed to an accuracy of 6:7% with samples generated by a random model.
Approximate message passing (AMP) algorithms have shown great promise in sparse signal reconstruction due to their low computational requirements and fast convergence to an exact solution. Moreover, they provide a probabilistic framework that is often more intuitive than alternatives such as convex optimisation. In this paper, AMP is used for audio source separation from underdetermined instantaneous mixtures. In the time-frequency domain, it is typical to assume a priori that the sources are sparse, so we solve the corresponding sparse linear inverse problem using AMP. We present a block-based approach that uses AMP to process multiple time-frequency points simultaneously. Two algorithms known as AMP and vector AMP (VAMP) are evaluated in particular. Results show that they are promising in terms of artefact suppression.
We consider the robust principal component analysis (RPCA) problem where the observed data is decomposed to a low-rank component and a sparse component. Conventionally, the matrix rank in RPCA is often approximated using a nuclear norm. Recently, RPCA has been formulated using the nonconvex ` -norm, which provides a closer approximation to the matrix rank than the traditional nuclear norm. However, the low-rank component generally has sparse property, especially in the transform domain. In this paper, a sparsity-based regularization term modeled with `1-norm is introduced to the formulation. An iterative optimization algorithm is developed to solve the obtained optimization problem. Experiments using synthetic and real data are utilized to validate the performance of the proposed method.
© 2014 IEEE.The visual modality, deemed to be complementary to the audio modality, has recently been exploited to improve the performance of blind source separation (BSS) of speech mixtures, especially in adverse environments where the performance of audio-domain methods deteriorates steadily. In this paper, we present an enhancement method to audio-domain BSS with the integration of voice activity information, obtained via a visual voice activity detection (VAD) algorithm. Mimicking aspects of human hearing, binaural speech mixtures are considered in our two-stage system. Firstly, in the off-line training stage, a speaker-independent voice activity detector is formed using the visual stimuli via the adaboosting algorithm. In the on-line separation stage, interaural phase difference (IPD) and interaural level difference (ILD) cues are statistically analyzed to assign probabilistically each time-frequency (TF) point of the audio mixtures to the source signals. Next, the detected voice activity cues (found via the visual VAD) are integrated to reduce the interference residual. Detection of the interference residual takes place gradually, with two layers of boundaries in the correlation and energy ratio map. We have tested our algorithm on speech mixtures generated using room impulse responses at different reverberation times and noise levels. Simulation results show performance improvement of the proposed method for target speech extraction in noisy and reverberant environments, in terms of signal-to-interference ratio (SIR) and perceptual evaluation of speech quality (PESQ).
We propose a novel algorithm for the enhancement of noisy reverberant speech using empirical-mode-decomposition (EMD) based subband processing. The proposed algorithm is a one-microphone multistage algorithm. In the first step, noisy reverberant speech is decomposed adaptively into oscillatory components called intrinsic mode functions (IMFs) via an EMD algorithm. Denoising is then applied to selected high frequency IMFs using EMD-based minimum mean-squared error (MMSE) filter, followed by spectral subtraction of the resulting denoised high-frequency IMFs and low-frequency IMFs. Finally, the enhanced speech signal is reconstructed from the processed IMFs. The method was motivated by our observation that the noise and reverberations are disproportionally distributed across the IMF components. Therefore, different levels of suppression can be applied to the additive noise and reverberation in each IMF. This leads to an improved enhancement performance as shown in comparison to a related recent approach, based on the measurements by the signal-to-noise ratio (SNR). © 2011 EURASIP.
A block-based approach coupled with adaptive dictionary learning is presented for underdetermined blind speech separation. The proposed algorithm, derived as a multi-stage method, is established by reformulating the underdetermined blind source separation problem as a sparse coding problem. First, the mixing matrix is estimated in the transform domain by a clustering algorithm. Then a dictionary is learned by an adaptive learning algorithm for which three algorithms have been tested, including the simultaneous codeword optimization (SimCO) technique that we have proposed recently. Using the estimated mixing matrix and the learned dictionary, the sources are recovered from the blocked mixtures by a signal recovery approach. The separated source components from all the blocks are concatenated to reconstruct the whole signal. The block-based operation has the advantage of improving considerably the computational efficiency of the source recovery process without degrading its separation performance. Numerical experiments are provided to show the competitive separation performance of the proposed algorithm, as compared with the state-of-the-art approaches. Using mutual coherence and sparsity index, the performance of a variety of dictionaries that are applied in underdetermined speech separation is compared and analyzed, such as the dictionaries learned from speech mixtures and ground truth speech sources, as well as those predefined by mathematical transforms such as discrete cosine transform (DCT) and short time Fourier transform (STFT). © 2012 Elsevier B.V. All rights reserved.
Data augmentation is an inexpensive way to increase training data diversity and is commonly achieved via transformations of existing data. For tasks such as classification, there is a good case for learning representations of the data that are invariant to such transformations, yet this is not explicitly enforced by classification losses such as the cross-entropy loss. This paper investigates the use of training objectives that explicitly impose this consistency constraint and how it can impact downstream audio classification tasks. In the context of deep convolutional neural networks in the supervised setting, we show empirically that certain measures of consistency are not implicitly captured by the cross-entropy loss and that incorporating such measures into the loss function can improve the performance of audio classification systems. Put another way, we demonstrate how existing augmentation methods can further improve learning by enforcing consistency.
Given binaural features as input, such as interaural level difference and interaural phase difference, Deep Neural Networks (DNNs) have been recently used to localize sound sources in a mixture of speech signals and/or noise, and to create time-frequency masks for the estimation of the sound sources in reverberant rooms. Here, we explore a more advanced system, where feed-forward DNNs are replaced by Convolutional Neural Networks (CNNs). In addition, the adjacent frames of each time frame (occurring before and after this frame) are used to exploit contextual information, thus improving the localization and separation for each source. The quality of the separation results is evaluated in terms of Signal to Distortion Ratio (SDR).
Acoustic event detection for content analysis in most cases relies on lots of labeled data. However, manually annotating data is a time-consuming task, which thus makes few annotated resources available so far. Unlike audio event detection, automatic audio tagging, a multi-label acoustic event classification task, only relies on weakly labeled data. This is highly desirable to some practical applications using audio analysis. In this paper we propose to use a fully deep neural network (DNN) framework to handle the multi-label classification task in a regression way. Considering that only chunk-level rather than frame-level labels are available, the whole or almost whole frames of the chunk were fed into the DNN to perform a multi-label regression for the expected tags. The fully DNN, which is regarded as an encoding function, can well map the audio features sequence to a multi-tag vector. A deep pyramid structure was also designed to extract more robust high-level features related to the target tags. Further improved methods were adopted, such as the Dropout and background noise aware training, to enhance its generalization capability for new audio recordings in mismatched environments. Compared with the conventional Gaussian Mixture Model (GMM) and support vector machine (SVM) methods, the proposed fully DNN-based method could well utilize the long-term temporal information with the whole chunk as the input. The results show that our approach obtained a 15% relative improvement compared with the official GMM-based method of DCASE 2016 challenge.
Significant improvement has been achieved in automated audio captioning (AAC) with recent models. However, these models have become increasingly large as their performance is enhanced. In this work, we propose a knowledge distillation (KD) framework for AAC. Our analysis shows that in the encoder-decoder based AAC models, it is more effective to dis-till knowledge into the encoder as compared with the decoder. To this end, we incorporate encoder-level KD loss into training, in addition to the standard supervised loss and sequence-level KD loss. We investigate two encoder-level KD methods, based on mean squared error (MSE) loss and contrastive loss, respectively. Experimental results demonstrate that contrastive KD is more robust than MSE KD, exhibiting superior performance in data-scarce situations. By leveraging audio-only data into training in the KD framework, our student model achieves competitive performance, with an inference speed that is 19 times faster.
Self-supervised learning methods have achieved promising performance for anomalous sound detection (ASD) under domain shift, where the type of domain shift is considered in feature learning by incorporating section IDs. However, the attributes accompanying audio files under each section, such as machine operating conditions and noise types, have not been considered, although they are also crucial for characterizing domain shifts. In this paper, we present a hierarchical metadata information constrained self-supervised (HMIC) ASD method, where the hierarchical relation between section IDs and attributes is constructed, and used as constraints to obtain finer feature representation. In addition, we propose an attribute-group-center (AGC)-based method for calculating the anomaly score under the domain shift condition. Experiments are performed to demonstrate its improved performance over the state-of-the-art self-supervised methods in DCASE 2022 challenge Task 2.
Deep learning-based methods have achieved significant performance for image defogging. However, existing methods are mainly developed for land scenes and perform poorly when dealing with overwater foggy images, since overwater scenes typically contain large expanses of sky and water. In this work, we propose a Prior map Guided CycleGAN (PG-CycleGAN) for defogging of images with overwater scenes. To promote the recovery of the objects on water in the image, two loss functions are exploited for the network where a prior map is designed to invert the dark channel and the min-max normalization is used to suppress the sky and emphasize objects. However, due to the unpaired training set, the network may learn an under-constrained domain mapping from foggy to fog-free image, leading to artifacts and loss of details. Thus, we propose an intuitive Upscaling Inception Module (UIM) and a Long-range Residual Coarse-to-fine framework (LRC) to mitigate this issue. Extensive experiments on qualitative and quantitative comparisons demonstrate that the proposed method outperforms the state-of-the-art supervised, semi-supervised, and unsupervised defogging approaches.
Deep neural networks (DNN) have recently been shown to give state-of-the-art performance in monaural speech enhancement. However in the DNN training process, the perceptual difference between different components of the DNN output is not fully exploited, where equal importance is often assumed. To address this limitation, we have proposed a new perceptually-weighted objective function within a feedforward DNN framework, aiming to minimize the perceptual difference between the enhanced speech and the target speech. A perceptual weight is integrated into the proposed objective function, and has been tested on two types of output features: spectra and ideal ratio masks. Objective evaluations for both speech quality and speech intelligibility have been performed. Integration of our perceptual weight shows consistent improvement on several noise levels and a variety of different noise types.
The problem of background clutter remains as a major challenge in radar-based navigation, particularly due to its time-varying statistical properties. Adaptive solutions for clutter removal are therefore sought which meet the demanding convergence and accuracy requirements of the navigation application. In this paper, a new structure which combines blind source separation (BSS) and adaptive interference cancellation (AIC) is proposed to solve the problem more accurately without prior statistical knowledge of the sea clutter. The new algorithms are confirmed to outperform previously proposed adaptive schemes for such processing through simulation studies.
Personalized dialogue generation, focusing on generating highly tailored responses by lever-aging persona profiles and dialogue context, has gained significant attention in conversational AI applications. However, persona profiles , a prevalent setting in current personal-ized dialogue datasets, typically composed of merely four to five sentences, may not offer comprehensive descriptions of the persona about the agent, posing a challenge to generate truly personalized dialogues. To handle this problem, we propose Learning Retrieval Augmentation for Personalized DialOgue Generation (LAPDOG), which studies the potential of leveraging external knowledge for persona dialogue generation. Specifically, the proposed LAPDOG model consists of a story retriever and a dialogue generator. The story retriever uses a given persona profile as queries to retrieve relevant information from the story document, which serves as a supplementary context to augment the persona profile. The dialogue generator utilizes both the dialogue history and the augmented persona profile to generate personalized responses. For optimization , we adopt a joint training framework that collaboratively learns the story retriever and dialogue generator, where the story retriever is optimized towards desired ultimate metrics (e.g., BLEU) to retrieve content for the dialogue generator to generate personalized responses. Experiments conducted on the CONVAI2 dataset with ROCStory as a supplementary data source show that the proposed LAPDOG method substantially outperforms the baselines, indicating the effectiveness of the proposed method. The LAPDOG model code is publicly available for further exploration. 1
Non-negative sparse coding (NSC) is a powerful technique for low-rank data approximation, and has found several successful applications in signal processing. However, the temporal dependency, which is a vital clue for many realistic signals, has not been taken into account in its conventional model. In this paper, we propose a general framework, i.e., convolutive non-negative sparse coding (CNSC), by considering a convolutive model for the low-rank approximation of the original data. Using this model, we have developed an effective learning algorithm based on the multiplicative adaptation of the reconstruction error function defined by the squared Euclidean distance. The proposed algorithm is applied to the separation of music audio objects in the magnitude spectrum domain. Interesting numerical results are provided to demonstrate its advantages over both the conventional NSC and an existing convolutive coding method.
Environmental audio tagging is a newly proposed task to predict the presence or absence of a specific audio event in a chunk. Deep neural network (DNN) based methods have been successfully adopted for predicting the audio tags in the domestic audio scene. In this paper, we propose to use a convolutional neural network (CNN) to extract robust features from mel-filter banks (MFBs), spectrograms or even raw waveforms for audio tagging. Gated recurrent unit (GRU) based recurrent neural networks (RNNs) are then cascaded to model the long-term temporal structure of the audio signal. To complement the input information, an auxiliary CNN is designed to learn on the spatial features of stereo recordings. We evaluate our proposed methods on Task 4 (audio tagging) of the Detection and Classification of Acoustic Scenes and Events 2016 (DCASE 2016) challenge. Compared with our recent DNN-based method, the proposed structure can reduce the equal error rate (EER) from 0.13 to 0.11 on the development set. The spatial features can further reduce the EER to 0.10. The performance of the end-to-end learning on raw waveforms is also comparable. Finally, on the evaluation set, we get the state-of-the-art performance with 0.12 EER while the performance of the best existing system is 0.15 EER.
The DCASE Challenge 2016 contains tasks for Acoustic Scene Classification (ASC), Acoustic Event Detection (AED), and audio tagging. Since 2006, Deep Neural Networks (DNNs) have been widely applied to computer visions, speech recognition and natural language processing tasks. In this paper, we provide DNN baselines for the DCASE Challenge 2016. In Task 1 we obtained accuracy of 81.0% using Mel + DNN against 77.2% by using Mel Frequency Cepstral Coefficients (MFCCs) + Gaussian Mixture Model (GMM). In Task 2 we obtained F value of 12.6% using Mel + DNN against 37.0% by using Constant Q Transform (CQT) + Nonnegative Matrix Factorization (NMF). In Task 3 we obtained F value of 36.3% using Mel + DNN against 23.7% by using MFCCs + GMM. In Task 4 we obtained Equal Error Rate (ERR) of 18.9% using Mel + DNN against 20.9% by using MFCCs + GMM. Therefore the DNN improves the baseline in Task 1, 3, and 4, although it is worse than the baseline in Task 2. This indicates that DNNs can be successful in many of these tasks, but may not always perform better than the baselines.
Automatically describing audio-visual content with texts, namely video captioning, has received significant attention due to its potential applications across diverse fields. Deep neural networks are the dominant methods, offering state-of-the-art performance. However, these methods are often undeployable in low-power devices like smartphones due to the large size of the model parameters. In this paper, we propose to exploit simple pooling front-end and down-sampling algorithms with knowledge distillation for audio and visual attributes using a reduced number of audio-visual frames. With the help of knowledge distillation from the teacher model, our proposed method greatly reduces the redundant information in audio-visual streams without losing critical contexts for caption generation. Extensive experimental evaluations on the MSR-VTT dataset demonstrate that our proposed approach significantly reduces the inference time by about 80% with a small sacrifice (less than 0.02%) in captioning accuracy.
Most of the binaural source separation algorithms only consider the dissimilarities between the recorded mixtures such as interaural phase and level differences (IPD, ILD) to classify and assign the time-frequency (T-F) regions of the mixture spectrograms to each source. However, in this paper we show that the coherence between the left and right recordings can provide extra information to label the T-F units from the sources. This also reduces the effect of reverberation which contains random reflections from different directions showing low correlation between the sensors. Our algorithm assigns the T-F regions into original sources based on weighted combination of IPD, ILD, the observation vectors models and the estimated interaural coherence (IC) between the left and right recordings. The binaural room impulse responses measured in four rooms with various acoustic conditions have been used to evaluate the performance of the proposed method which shows an improvement of more than 1:4 dB in signal-to-distortion ratio (SDR) in room D with T60 = 0:89 s over the state-of-the-art algorithms.
Recent studies show that facial information contained in visual speech can be helpful for the performance enhancement of audio-only blind source separation (BSS) algorithms. Such information is exploited through the statistical characterization of the coherence between the audio and visual speech using, e.g., a Gaussian mixture model (GMM). In this paper, we present three contributions. With the synchronized features, we propose an adapted expectation maximization (AEM) algorithm to model the audiovisual coherence in the off-line training process. To improve the accuracy of this coherence model, we use a frame selection scheme to discard nonstationary features. Then with the coherence maximization technique, we develop a new sorting method to solve the permutation problem in the frequency domain. We test our algorithm on a multimodal speech database composed of different combinations of vowels and consonants. The experimental results show that our proposed algorithm outperforms traditional audio-only BSS, which confirms the benefit of using visual speech to assist in separation of the audio. © 2011 Elsevier B.V. All rights reserved.
Audio tagging aims to perform multi-label classification on audio chunks and it is a newly proposed task in the Detection and Classification of Acoustic Scenes and Events 2016 (DCASE 2016) challenge. This task encourages research efforts to better analyze and understand the content of the huge amounts of audio data on the web. The difficulty in audio tagging is that it only has a chunk-level label without a frame-level label. This paper presents a weakly supervised method to not only predict the tags but also indicate the temporal locations of the occurred acoustic events. The attention scheme is found to be effective in identifying the important frames while ignoring the unrelated frames. The proposed framework is a deep convolutional recurrent model with two auxiliary modules: an attention module and a localization module. The proposed algorithm was evaluated on the Task 4 of DCASE 2016 challenge. State-of-the-art performance was achieved on the evaluation set with equal error rate (EER) reduced from 0.13 to 0.11, compared with the convolutional recurrent baseline system.
Accurate sea surface temperature (SST) prediction is vital for disaster prevention, ocean circulation, and climate change. Traditional SST prediction methods, predominantly reliant on time-intensive numerical models, face challenges in terms of speed and efficiency. In this study, we developed a novel deep learning approach using a 3D U-Net structure with multi-source data to forecast SST in the South China Sea (SCS). SST, sea surface height anomaly (SSHA), and sea surface wind (SSW) were used as input variables. Compared with the convolutional long short-term memory (ConvLSTM) model, the 3D U-Net model achieved more accurate predictions at all lead times (from 1 to 30 days) and performed better in different seasons. Spatially, the 3D U-Net model's SST predictions exhibited low errors (RMSE < 0.5 degrees C) and high correlation (R > 0.9) across most of the SCS. The spatially averaged time series of SST, both predicted by the 3D U-Net and observed in 2021, showed remarkable consistency. A noteworthy application of the 3D U-Net model in this research was the successful detection of marine heat wave (MHW) events in the SCS in 2021. The model accurately captured the occurrence frequency, total duration, average duration, and average cumulative intensity of MHW events, aligning closely with the observed data. Sensitive experiments showed that SSHA and SSW have significant impacts on the prediction of the 3D U-Net model, which can improve the accuracy and play different roles in different forecast periods. The combination of the 3D U-Net model with multi-source sea surface variables, not only rapidly predicted SST in the SCS but also presented a novel method for forecasting MHW events, highlighting its significant potential and advantages.
Sound event detection (SED) is a widely studied field that has achieved considerable success. The dynamic routing mechanism of capsule networks has been used for SED, but its performance in capturing global information of audio is still limited. In this paper, we propose a method for SED that by combining the capsule network with transformer leverages the strength of transformer in capturing global features with that of capsule network in capturing local features. The proposed method was evaluated on the DCASE 2017 Task 4 weakly labeled dataset. The obtained F-score and Equal Error Rate are 60.6% and 0.75, respectively. Compared to other baseline systems, our method achieves significantly improved performance. Keywords: Sound event detection, audio tagging, gated convolution, transformer, capsule network.
In this paper, an event-triggered adaptive tracking control strategy is proposed for strict-feedback stochastic nonlinear systems with predetermined finite-time performance. Firstly, a finite-time performance function (FTPF) is introduced to describe the predetermined tracking performance. With the help of the error transformation technique, the original constrained tracking error is transformed into an equivalent unconstrained variable. Then, the unknown nonlinear functions are approximated by using the multi-dimensional Taylor networks (MTNs) in the backstepping design process. Meanwhile, an event-triggered mechanism with a relative threshold is introduced to reduce the communication burden between actuators and controllers. Furthermore, the proposed control strategy can ensure that all signals of the closed-loop system are bounded in probability and the tracking error is within a predefined range in a finite time. In the end, the effectiveness of the proposed control strategy is verified by two simulation examples.
In the field of computer vision, anomaly detection is a binary classification task used to identify exceptional instances within image datasets. Typically, it can be divided into two aspects: texture defect detection and semantic anomaly detection. Existing methods often use pre-trained feature extractors to singly capture semantic or spatial features of images, and then employ different classifiers to handle these two types of anomaly detection tasks. However, these methods fail to fully utilize the synergistic relationship between these two types of features, resulting in algorithms that excel in one type of anomaly detection task but perform poorly in the other type. Therefore, we propose a novel approach that successfully combines these two types of features into a normalizing flow learning module to address both types of anomaly detection tasks. Specifically, we first adopt a pre-trained Vision Transformer (ViT) model to capture both texture and semantic features of input images. Subsequently, using the semantic features as input, we design a novel normalizing flow model to fit the semantic distribution of normal data. In addition, we introduce a feature fusion module based on attention mechanisms to integrate the most relevant texture and semantic information between these two types of features, significantly enhancing the model’s ability to simultaneously represent the spatial texture and semantic features of the input image. Finally, We conduct comprehensive experiments on well-known semantic and texture anomaly detection datasets, namely Cifar10 and MVTec, to evaluate the performance of our proposed method. The results demonstrate that our model achieves outstanding performance in both semantic and texture anomaly detection tasks, particularly achieving state-of-the-art results in semantic anomaly detection.
The effective communications range for aerial acoustic communication in indoor environment is a critical challenge for applications on smartphones. An inaudible acoustic communication system on Android phones that breaks the range limit is proposed in this paper, owing to an innovative design of the receiver by considering the time–frequency features of the received signals, using a fractional Fourier transform and 2-D mask filter to denoise the signals, and a hard detection and a soft detection approach to detect the symbols so as to tackle the severe multipath and Doppler effects in large indoor environments. Onsite test results show that an error-free communication distance of 70 m can be achieved at 20 bps, and the estimated maximum communication range could reach at least 85 m.
Automatically describing audio-visual content with texts, namely video captioning, has received significant attention due to its potential applications across diverse fields. Deep neural networks are the dominant methods, offering state-of-the-art performance. However, these methods are often undeployable in low-power devices like smartphones due to the large size of the model parameters. In this paper, we propose to exploit simple pooling front-end and down-sampling algorithms with knowledge distillation for audio and visual attributes using a reduced number of audio-visual frames. With the help of knowledge distillation from the teacher model, our proposed method greatly reduces the redundant information in audio-visual streams without losing critical contexts for caption generation. Extensive experimental evaluations on the MSR-VTT dataset demonstrate that our proposed approach significantly reduces the inference time by about 80% with a small sacrifice (less than 0.02%) in captioning accuracy.
Recently, Transformer shows the potential to exploit the long-range sequence dependency in speech with self-attention. It has been introduced in single channel speech enhancement to improve the accuracy of speech estimation from a noise mixture. However, the amount of information represented across attention-heads is often huge, which leads to increased computational complexity. To address this issue, the axial attention is proposed i.e., to split a 2D attention into two 1-D attentions. In this paper, we develop a new method for speech enhancement by leveraging the axial attention, where we generate time and frequency sub-attention maps by calculating the attention map along time- and frequency-axis. Different from the conventional axial attention, the proposed method provides two parallel multi-head attentions for time- and frequency-axis, respectively. Moreover, the frequency-band aware attention is proposed i.e., high frequency-band attention (HFA), and low frequency-band attention (LFA), which facilitates the exploitation of the information related to speech and noise in different frequency bands in the noisy mixture. To re-use high-resolution feature maps from the encoder, we design a U-shaped Transformer, which helps recover lost information from the high-level representations to further improve the speech estimation accuracy. Extensive experiments on four public datasets are used to demonstrate the efficacy of the proposed method.
Smartphone ownership has increased rapidly over the past decade, and the smartphone has become a popular technological product in modern life. The universal wireless communication scheme on smartphones leverages electromagnetic wave transmission, where the spectrum resource becomes scarce in some scenarios. As a supplement to some face-to-face transmission scenarios, we design an aerial ultrasonic communication scheme. The scheme uses chirp-like signal and BPSK modulation, convolutional code encoding with ID-classified interleaving, and pilot method to estimate room impulse response. Through experiments, the error rate of the ultrasonic communication system designed for mobile phones can be within 0.001%\documentclass[12pt]{minimal} \usepackage{amsmath} \usepackage{wasysym} \usepackage{amsfonts} \usepackage{amssymb} \usepackage{amsbsy} \usepackage{mathrsfs} \usepackage{upgreek} \setlength{\oddsidemargin}{-69pt} \begin{document}$$0.001\%$$\end{document} in 1 m range. The limitations of this scheme and further research work are discussed as well.
We study the problem of wideband direction of arrival (DoA) estimation by joint optimisation of array and spatial sparsity. Two-step iterative process is proposed. In the first step, the wideband signal is reshaped and used as the input to derive the weight coefficients using a sparse array optimisation method. The weights are then used to scale the observed signal model for which a compressive sensing based spatial sparsity optimisation method is used for DoA estimation. Simulations are provided to demonstrate the performance of the proposed method for both stationary and moving sources.
Polyphonic sound event localization and detection (SELD), which jointly performs sound event detection (SED) and direction-of-arrival (DoA) estimation, detects the type and occurrence time of sound events as well as their corresponding DoA angles simultaneously. We study the SELD task from a multi-task learning perspective. Two open problems are addressed in this paper. Firstly, to detect overlapping sound events of the same type but with different DoAs, we propose to use a trackwise output format and solve the accompanying track permutation problem with permutation-invariant training. Multi-head self-attention is further used to separate tracks. Secondly, a previous finding is that, by using hard parameter-sharing, SELD suffers from a performance loss compared with learning the subtasks separately. This is solved by a soft parameter-sharing scheme. We term the proposed method as Event Independent Network V2 (EINV2), which is an improved version of our previously-proposed method and an end-to-end network for SELD. We show that our proposed EINV2 for joint SED and DoA estimation outperforms previous methods by a large margin, and has comparable performance to state-of-the-art ensemble models. Index Terms— Sound event localization and detection, direction of arrival, event-independent, permutation-invariant training, multi-task learning.
The use of semantic Web technologies and service oriented computing paradigm in Internet of Things research has recently received significant attention to create a semantic service layer that supports virtualisation of and interaction among "Things". Using service-based solutions will produce a deluge of services that provide access to different data and capabilities exposed by different resources. The heterogeneity of the resources and their service attributes, and dynamicity of mobile environments require efficient solutions that can discover services and match them to the data and capability requirements of different users. Semantic service matchmaking process is the fundamental construct for providing higher level service-oriented functionalities such as service recommendation, composition, and provisioning in Internet of Things. However, scalability of the current approaches in dealing with large number of services and efficiency of logical inference mechanisms in processing huge number of heterogeneous service attributes and metadata are limited. We propose a hybrid semantic service matchmaking method that combines our previous work on probabilistic service matchmaking using latent semantic analysis with a weighted-link analysis based on logical signature matching. The hybrid method can overcome most cases of semantic synonymy in semantic service description which usually presents the biggest challenge for semantic service matchmakers. The results show that the proposed method performs better than existing solutions in terms of precision (P@n) and normalised discounted cumulative gain (NDCG) measurement values. © 2012 IEEE.
In supervised machine learning, the assumption that training data is labelled correctly is not always satisfied. In this paper, we investigate an instance of labelling error for classification tasks in which the dataset is corrupted with out-of-distribution (OOD) instances: data that does not belong to any of the target classes, but is labelled as such. We show that detecting and relabelling certain OOD instances, rather than discarding them, can have a positive effect on learning. The proposed method uses an auxiliary classifier, trained on data that is known to be in-distribution, for detection and relabelling. The amount of data required for this is shown to be small. Experiments are carried out on the FSDnoisy18k audio dataset, where OOD instances are very prevalent. The proposed method is shown to improve the performance of convolutional neural networks by a significant margin. Comparisons with other noise-robust techniques are similarly encouraging.
General-purpose audio tagging refers to classifying sounds that are of a diverse nature, and is relevant in many applications where domain-specific information cannot be exploited. The DCASE 2018 challenge introduces Task 2 for this very problem. In this task, there are a large number of classes and the audio clips vary in duration. Moreover, a subset of the labels are noisy. In this paper, we propose a system to address these challenges. The basis of our system is an ensemble of convolutional neural networks trained on log-scaled mel spectrograms. We use preprocessing and data augmentation methods to improve the performance further. To reduce the effects of label noise, two techniques are proposed: loss function weighting and pseudo-labeling. Experiments on the private test set of this task show that our system achieves state-of-the-art performance with a mean average precision score of 0.951
A robust constrained blind source separation (CBSS) algorithm has been proposed for separation and localization of the P300 sources in schizophrenia patients. The algorithm is an extension of the Infomax algorithm, based on minimization of mutual information, for which a reference P300 signal is used as a constraint. The reference signal forces the unmixing matrix to separate the sources of both auditory and visual P300 resulted from the corresponding stimulations. The constrained problem is then converted to an unconstrained problem by means of a set of nonlinear penalty functions. This leads to the modification of the overall cost function, based on the natural gradient algorithm (NGA). The P300 sources are then localized based on electrode - source correlations. © Springer-Verlag 2004.
Single-channel signal separation and deconvolution aims to separate and deconvolve individual sources from a single-channel mixture and is a challenging problem in which no prior knowledge of the mixing filters is available. Both individual sources and mixing filters need to be estimated. In addition, a mixture may contain non-stationary noise which is unseen in the training set. We propose a synthesizing-decomposition (S-D) approach to solve the single-channel separation and deconvolution problem. In synthesizing, a generative model for sources is built using a generative adversarial network (GAN). In decomposition, both mixing filters and sources are optimized to minimize the reconstruction error of the mixture. The proposed S-D approach achieves a peak-to-noise-ratio (PSNR) of 18.9 dB and 15.4 dB in image inpainting and completion, outperforming a baseline convolutional neural network PSNR of 15.3 dB and 12.2 dB, respectively and achieves a PSNR of 13.2 dB in source separation together with deconvolution, outperforming a convolutive non-negative matrix factorization (NMF) baseline of 10.1 dB.
Source separation is the task of separating an audio recording into individual sound sources. Source separation is fundamental for computational auditory scene analysis. Previous work on source separation has focused on separating particular sound classes such as speech and music. Much previous work requires mixtures and clean source pairs for training. In this work, we propose a source separation framework trained with weakly labelled data. Weakly labelled data only contains the tags of an audio clip, without the occurrence time of sound events. We first train a sound event detection system with AudioSet. The trained sound event detection system is used to detect segments that are most likely to contain a target sound event. Then a regression is learnt from a mixture of two randomly selected segments to a target segment conditioned on the audio tagging prediction of the target segment. Our proposed system can separate 527 kinds of sound classes from AudioSet within a single system. A U-Net is adopted for the separation system and achieves an average SDR of 5.67 dB over 527 sound classes in AudioSet.
Sound Event Localization and Detection (SELD) is a task that involves detecting different types of sound events along with their temporal and spatial information, specifically, detecting the classes of events and estimating their corresponding direction of arrivals at each frame. In practice, real-world sound scenes might be complex as they may contain multiple overlapping events. For instance, in DCASE challenges task 3, each clip may involve simultaneous occurrences of up to five events. To handle multiple overlapping sound events, current methods prefer multiple output branches to estimate each event, which increases the size of the models. Therefore, current methods are often difficult to be deployed on the edge of sensor networks. In this paper, we propose a method called Probabilistic Localization and Detection of Independent Sound Events with Transformers (PLDISET), which estimates numerous events by using one output branch. The method has three stages. First, we introduce the track generation module to obtain various tracks from extracted features. Then, these tracks are fed into two transformers for sound event detection (SED) and localization, respectively. Finally, one output system, including a linear Gaussian system and regression network, is used to estimate each track. We give the evaluation resn results of our model on DCASE 2023 Task 3 development dataset.
Effcient training of support vector machines (SVMs) with large-scale samples is of crucial importance in the era of big data. Sequential minimal optimization (SMO) is considered as an effective solution to this challenging task, and the working set selection is one of the key steps in SMO. Various strategies have been developed and implemented for working set selection in LibSVM and Shark. In this work we point out that the algorithm used in LibSVM does not maintain the box-constraints which, nevertheless, are very important for evaluating the final gain of the selection operation. Here, we propose a new algorithm to address this challenge. The proposed algorithm maintains the box-constraints within a selection procedure using a feasible optional step-size. We systematically study and compare several related algorithms, and derive new theoretical results. Experiments on benchmark data sets show that our algorithm effectively improves the training speed without loss of accuracy.
In existing audio-visual blind source separation (AV-BSS) algorithms, the AV coherence is usually established through statistical modelling, using e.g. Gaussian mixture models (GMMs). These methods often operate in a lowdimensional feature space, rendering an effective global representation of the data. The local information, which is important in capturing the temporal structure of the data, however, has not been explicitly exploited. In this paper, we propose a new method for capturing such local information, based on audio-visual dictionary learning (AVDL). We address several challenges associated with AVDL, including cross-modality differences in size, dimension and sampling rate, as well as the issues of scalability and computational complexity. Following a commonly employed bootstrap coding-learning process, we have developed a new AVDL algorithm which features, a bimodality balanced and scalable matching criterion, a size and dimension adaptive dictionary, a fast search index for efficient coding, and cross-modality diverse sparsity. We also show how the proposed AVDL can be incorporated into a BSS algorithm. As an example, we consider binaural mixtures, mimicking aspects of human binaural hearing, and derive a new noise-robust AV-BSS algorithm by combining the proposed AVDL algorithm with Mandel’s BSS method, which is a state-of-the-art audio-domain method using time-frequency masking. We have systematically evaluated the proposed AVDL and AV-BSS algorithms, and show their advantages over the corresponding baseline methods, using both synthetic data and visual speech data from the multimodal LILiR Twotalk corpus.
Most deep learning-based acoustic scene classification (ASC) approaches identify scenes based on acoustic features converted from audio clips containing mixed information entangled by polyphonic audio events (AEs). However, these approaches have difficulties in explaining what cues they use to identify scenes. This paper conducts the first study on disclosing the relationship between real-life acoustic scenes and semantic embeddings from the most relevant AEs. Specifically, we propose an event-relational graph representation learning (ERGL) framework for ASC to classify scenes, and simultaneously answer clearly and straightly which cues are used in classifying. In the event-relational graph, embeddings of each event are treated as nodes, while relationship cues derived from each pair of nodes are described by multi-dimensional edge features. Experiments on a real-life ASC dataset show that the proposed ERGL achieves competitive performance on ASC by learning embeddings of only a limited number of AEs. The results show the feasibility of recognizing diverse acoustic scenes based on the audio event-relational graph. Visualizations of graph representations learned by ERGL are available here (https://github.com/Yuanbo2020/ERGL) .
State-of-the-art binaural objective intelligibility measures (OIMs) require individual source signals for making intelligibility predictions, limiting their usability in real-time online operations. This limitation may be addressed by a blind source separation (BSS) process, which is able to extract the underlying sources from a mixture. In this study, a speech source is presented with either a stationary noise masker or a fluctuating noise masker whose azimuth varies in a horizontal plane, at two speech-to-noise ratios (SNRs). Three binaural OIMs are used to predict speech intelligibility from the signals separated by a BSS algorithm. The model predictions are compared with listeners' word identification rate in a perceptual listening experiment. The results suggest that with SNR compensation to the BSS-separated speech signal, the OIMs can maintain their predictive power for individual maskers compared to their performance measured from the direct signals. It also reveals that the errors in SNR between the estimated signals are not the only factors that decrease the predictive accuracy of the OIMs with the separated signals. Artefacts or distortions on the estimated signals caused by the BSS algorithm may also be concerns.
In GPS-aided strap-down inertial navigation system, in-motion initial alignment is crucial and can be solved with a closed-loop scheme based on state estimation. With this method, the noise covariance matrices need to be estimated, which, however, can be inaccurate in practice. In this paper, a novel adaptive Kalman filter is proposed to address the above problem. The state and measurement noise covariance matrices are jointly estimated based on a variational Bayesian approach, in which the prior and posterior probability density functions of the state noise covariance matrix and one-step prediction error covariance matrix are assumed to have the same form. Simulation results demonstrate that the proposed algorithm can improve the initial alignment accuracy of the in-motion initial alignment based on a closed-loop scheme as compared with an existing baseline adaptive Kalman filter.
This paper proposes a deep learning framework for classification of BBC television programmes using audio. The audio is firstly transformed into spectrograms, which are fed into a pre-trained Convolutional Neural Network (CNN), obtaining predicted probabilities of sound events occurring in the audio recording. Statistics for the predicted probabilities and detected sound events are then calculated to extract discriminative features representing the television programmes. Finally, the embedded features extracted are fed into a classifier for classifying the programmes into different genres. Our experiments are conducted over a dataset of 6,160 programmes belonging to nine genres labelled by the BBC. We achieve an average classification accuracy of 93.7% over 14-fold cross validation. This demonstrates the efficacy of the proposed framework for the task of audio-based classification of television programmes.
Subspace clustering is a popular method for clustering unlabelled data. However, the computational cost of the subspace clustering algorithm can be unaffordable when dealing with a large data set. Using a set of dimension sketched data instead of the original data set can be helpful for mitigating the computational burden. Thus, finding a way for dimension sketching becomes an important problem. In this paper, a new dimension sketching algorithm is proposed, which aims to select informative dimensions that have significant effects on the clustering results. Experimental results reveal that this method can significantly improve subspace clustering performance on both synthetic and real-world datasets, in comparison with two baseline methods.
Multiple instance learning (MIL) has recently been used for weakly labelled audio tagging, where the spectrogram of an audio signal is divided into segments to form instances in a bag, and then the low-dimensional features of these segments are pooled for tagging. The choice of a pooling scheme is the key to exploiting the weakly labelled data. However, the traditional pooling schemes are usually fixed and unable to distinguish the contributions, making it difficult to adapt to the characteristics of the sound events. In this paper, a novel pooling algorithm is proposed for MIL, named gated multi-head attention pooling (GMAP), which is able to attend to the information of events from different heads at different positions. Each head allows the model to learn information from different representation subspaces. Furthermore, in order to avoid the redundancy of multi-head information, a gating mechanism is used to fuse individual head features. The proposed GMAP increases the modeling power of the single-head attention with no computational overhead. Experiments are carried out on Audioset, which is a large-scale weakly labelled dataset, and show superior results to the non-adaptive pooling and the vanilla attention pooling schemes.
Head pose is an important cue in many applications such as, speech recognition and face recognition. Most approaches to head pose estimation to date have used visual information to model and recognise a subject's head in different configurations. These approaches have a number of limitations such as, inability to cope with occlusions, changes in the appearance of the head, and low resolution images. We present here a novel method for determining coarse head pose orientation purely from audio information, exploiting the direct to reverberant speech energy ratio (DRR) within a highly reverberant meeting room environment. Our hypothesis is that a speaker facing towards a microphone will have a higher DRR and a speaker facing away from the microphone will have a lower DRR. This hypothesis is confirmed by experiments conducted on the publicly available AV16.3 database. © 2013 IEEE.
Describing the semantic content of an image via natural language, known as image captioning, has recently attracted substantial interest in computer vision and language processing communities. Current image captioning approaches are mainly based on an encoder-decoder framework in which visual information is extracted by an image encoder and captions are generated by a text decoder, using convolution neural networks (CNN) and recurrent neural networks (RNN), respectively. Although this framework is promising for image captioning, it has limitations in utilizing the encoded visual information for generating grammatically and semantically correct captions in the RNN decoder. More specifically, the RNN decoder is ineffective in using the contextual information from the encoded data due to its limited ability in capturing long-term complex dependencies. Inspired by the advantage of gated recurrent unit (GRU), in this paper, we propose an extension of conventional RNN by introducing a multi-layer GRU that modulates the most relevant information inside the unit to enhance the semantic coherence of captions. Experimental results on the MSCOCO dataset show the superiority of our proposed approach over the state-of-the-art approaches in several performance metrics.
Anomaly detection refers to the process of detecting anomalies from data that do not follow its distribution. In recent years, Transformer-based methods utilizing generative adversarial networks (GANs) have shown remarkable performance in this field. Unlike traditional convolutional architectures, Transformer structures have advantages in capturing long-range dependencies, leading to a substantial improvement in detection performance. However, transformer-based models may be limited in capturing fine-grained details as well as the inference speed. In this paper, we propose a scalable convolutional Generative Adversarial Network (GAN) called GanNeXt. Our design incorporates a new convolutional architecture that utilizes depthwise convolutional layers and pointwise convolutional layers as extension layers. In addition, we introduce skip connections to capture multi-scale local details. Experiments demonstrate that our proposed method achieves a 58% reduction in floating-point operations per second (FLOPs), while outperforming state-of-the-art Transformer-based GAN baselines on CIFAR10 and STL10 datasets. The codes will be available at https://github.com/SYLan2019/GanNeXt.
Visual object counting (VOC) is an emerging area in computer vision which aims to estimate the number of objects of interest in a given image or video. Recently, object density based estimation method is shown to be promising for object counting as well as rough instance localization. However, the performance of this method tends to degrade when dealing with new objects and scenes. To address this limitation, we propose a manifold-based method for visual object counting (M-VOC), based on the manifold assumption that similar image patches share similar object densities. Firstly, the local geometry of a given image patch is represented linearly by its neighbors using a predefined patch training set, and the object density of this given image patch is reconstructed by preserving the local geometry using locally linear embedding. To improve the characterization of local geometry, additional constraints such as sparsity and non-negativity are also considered via regularization, nonlinear mapping, as well as kernel trick. Compared with the state-of-the-art VOC methods, our proposed M-VOC methods achieve competitive performance on seven benchmark datasets. Experiments verify that the proposed M-VOC methods have several favorable properties, such as robustness to the variation in the size of training dataset and image resolution, as often encountered in real-world VOC applications.
Information from video has been used recently to address the issue of scaling ambiguity in convolutive blind source separation (BSS) in the frequency domain, based on statistical modeling of the audio-visual coherence with Gaussian mixture models (GMMs) in the feature space. However, outliers in the feature space may greatly degrade the system performance in both training and separation stages. In this paper, a new feature selection scheme is proposed to discard non-stationary features, which improves the robustness of the coherence model and reduces its computational complexity. The scaling parameters obtained by coherence maximization and non-linear interpolation from the selected features are applied to the separated frequency components to mitigate the scaling ambiguity. A multimodal database composed of different combinations of vowels and consonants was used to test our algorithm. Experimental results show the performance improvement with our proposed algorithm.
We address the problem of recovering a sparse signal from clipped or quantized measurements. We show how these two problems can be formulated as minimizing the distance to a convex feasibility set, which provides a convex and differentiable cost function. We then propose a fast iterative shrinkage/thresholding algorithm that minimizes the proposed cost, which provides a fast and efficient algorithm to recover sparse signals from clipped and quantized measurements.
In this paper, we propose a novel robust multiple human tracking approach based upon processing a video signal by utilizing a social force model to enhance the particle probability hypothesis density (PHD) filter. In traditional dynamic models, the states of targets are only predicted by their own history; however, in multiple human tracking, the information from interaction between targets and the intentions of each target can be employed to obtain more robust prediction. Furthermore, such information can mitigate the problems of collision and occlusion. The cardinality of variable number of targets can also be estimated by using the PHD filter, hence improving the overall accuracy of the multiple human tracker. In this work, a background subtraction step has also been employed to identify the new born targets and provide the measurement set for the PHD filter. To evaluate tracking performance, sequences from both the CAVIAR and PETS2009 datasets are employed for evaluation, which shows clear improvement of the proposed method over the conventional particle PHD filter.
A constrained blind source separation (BSS) approach for separation of intracranial spikes from scalp electroencephalogram (EEG) has been proposed in this paper. This method is based on creating a template from intracranial data, which is then used in the form of a constraint in a BSS algorithm. To generate a suitable template, the segments during which the brain discharges are labelled are used to generate the necessary templates. Approximate entropy followed by peak detection and thresholding is used for this purpose. Constrained BSS is then applied to scalp data to extract the desired source and to evaluate its effect on scalp electrodes. The effectiveness of such a constrained approach has been demonstrated by comparing its outcome with that of the unconstrained method. © 2013 IEEE.
Recently, there has been increasing interest in building efficient audio neural networks for on-device scenarios. Most existing approaches are designed to reduce the size of audio neural networks using methods such as model pruning. In this work, we show that instead of reducing model size using complex methods, eliminating the temporal redundancy in the input audio features (e.g., mel-spectrogram) could be an effective approach for efficient audio classification. To do so, we proposed a family of simple pooling front-ends (SimPFs) which use simple non-parametric pooling operations to reduce the redundant information within the mel-spectrogram. We perform extensive experiments on four audio classification tasks to evaluate the performance of SimPFs. Experimental results show that SimPFs can achieve a reduction in more than half of the number of floating point operations (FLOPs) for off-the-shelf audio neural networks, with negligible degradation or even some improvements in audio classification performance.
Sound event detection (SED) aims to detect when and recognize what sound events happen in an audio clip. Many supervised SED algorithms rely on strongly labelled data which contains the onset and offset annotations of sound events. However, many audio tagging datasets are weakly labelled, that is, only the presence of the sound events is known, without knowing their onset and offset annotations. In this paper, we propose a time-frequency (T-F) segmentation framework trained on weakly labelled data to tackle the sound event detection and separation problem. In training, a segmentation mapping is applied on a T-F representation, such as log mel spectrogram of an audio clip to obtain T-F segmentation masks of sound events. The T-F segmentation masks can be used for separating the sound events from the background scenes in the time-frequency domain. Then a classification mapping is applied on the T-F segmentation masks to estimate the presence probabilities of the sound events. We model the segmentation mapping using a convolutional neural network and the classification mapping using a global weighted rank pooling (GWRP). In SED, predicted onset and offset times can be obtained from the T-F segmentation masks. As a byproduct, separated waveforms of sound events can be obtained from the T-F segmentation masks. We remixed the DCASE 2018 Task 1 acoustic scene data with the DCASE 2018 Task 2 sound events data. When mixing under 0 dB, the proposed method achieved F1 scores of 0.534, 0.398 and 0.167 in audio tagging, frame-wise SED and event-wise SED, outperforming the fully connected deep neural network baseline of 0.331, 0.237 and 0.120, respectively. In T-F segmentation, we achieved an F1 score of 0.218, where previous methods were not able to do T-F segmentation.
Fish feeding intensity assessment (FFIA) aims to evaluate the intensity change of fish appetite during the feeding process, which is vital in industrial aquaculture applications. The main challenges surrounding FFIA are two-fold. 1) robustness: existing work has mainly leveraged single-modality (e.g., vision, audio) methods, which have a high sensitivity to input noise. 2) efficiency: FFIA models are generally expected to be employed on devices. This presents a challenge in terms of computational efficiency. In this work, we first introduce an audio-visual dataset, called AV-FFIA. AV-FFIA consists of 27,000 labeled audio and video clips that capture different levels of fish feeding intensity. To our knowledge, AV-FFIA is the first large-scale multimodal dataset for FFIA research. Then, we introduce a multi-modal approach for FFIA by leveraging single-modality pre-trained models and modality-fusion methods, with benchmark studies on AV-FFIA. Our experimental results indicate that the multi-modal approach substantially outperforms the single-modality based approach, especially in noisy environments. While multimodal approaches provide a performance gain for FFIA, it inherently increase the computational cost. To overcome this issue, we further present a novel unified model, termed as U-FFIA. U-FFIA is a single model capable of processing audio, visual, or audio-visual modalities, by leveraging modality dropout during training and knowledge distillation from single-modality pre-trained models. We demonstrate that U-FFIA can achieve performance better than or on par with the state-of-the-art modality-specific FFIA models, with significantly lower computational overhead. Our proposed U-FFIA approach enables a more robust and efficient method for FFIA, with the potential to contribute to improved management practices and sustainability in aquaculture.
Underdetermined speech separation is a challenging problem that has been studied extensively in recent years. A promising method to this problem is based on the so-called sparse signal representation. Using this technique, we have recently developed a multi-stage algorithm, where the source signals are recovered using a pre-defined dictionary obtained by e.g. the discrete cosine transform (DCT). In this paper, instead of using the pre-defined dictionary, we present three methods for learning adaptive dictionaries for the reconstruction of source signals, and compare their performance with several state-of-the-art speech separation methods. © 2011 IEEE.
Suppression of late reverberations is a challenging problem in reverberant speech enhancement. A promising recent approach to this problem is to apply a spectral subtraction mask to the spectrum of the reverberant speech, where the spectral variance of the late reverberations was estimated based on a frequency independent statistical model of the decay rate of the late reverberations. In this paper, we develop a dereverberation algorithm by following a similar process. Instead of using the frequency independent model, however, we estimate the frequency dependent reverberation time and decay rate, and use them for the estimation of the spectral subtraction mask. In order to remove the processing artifacts, the mask is further filtered by a smoothing function, and then applied to reduce the late reverberations from the reverberant speech. The performance of the proposed algorithm, measured by the segmental signal to reverberation ratio (SegSRR) and the signal to distortion ratio (SDR), is evaluated for both simulated and real data. As compared with the related frequency indepenent algorithm, the proposed algorithm offers considerable performance improvement.
Underdetermined reverberant speech separation is a challenging problem in source sep- aration that has received considerable attention in both computational auditory scene analysis (CASA) and blind source separation (BSS). Recent studies suggest that, in general, the performance of frequency domain BSS methods suffer from the permuta- tion problem across frequencies which degrades in high reverberation, meanwhile, CASA methods perform less effectively for closely spaced sources. This paper presents a method to address these limitations, based on the combination of monaural, binaural and BSS cues for the automatic classification of time-frequency (T-F) units of the speech mixture spectrogram. By modeling the interaural phase difference, the interaural level difference and frequency-bin mixing vectors, we integrate the coherence information for each source within a probabilistic framework. The Expectation-Maximization (EM) algorithm is then used iteratively to refine the soft assignment of TF regions to sources and re-estimate their model parameters. It is observed that the reliability of the cues affects the accu- racy of the estimates and varies with respect to cue type and frequency. As such, the contribution of each cue to the assignment decision is adjusted by weighting the log- likelihoods of the cues empirically, which significantly improves the performance. Results are reported for binaural speech mixtures in five rooms covering a range of reverberation times and direct-to-reverberant ratios. The proposed method compares favorably with state-of-the-art baseline algorithms by Mandel et al. and Sawada et al., in terms of signal- to-distortion ratio (SDR) of the separated source signals. The paper also investigates the effect of introducing spectral cues for integration within the same framework. Analysis of the experimental outcomes will include a comparison of the contribution of individual cues under varying conditions and discussion of the implications for system optimization.
The Internet of Things (IoT) paradigm connects everyday objects to the Internet and enables a multitude of applications with the real world data collected from those objects. In the city environment, real world data sources include fixed installations of sensor networks by city authorities as well as mobile sources, such as citizens’ smartphones,¬ taxis and buses equipped with sensors. This kind of data varies not only along the temporal but also the spatial axis. For handling such frequently updated, time-stamped and structured data from a large number of heterogeneous sources, this paper presents a data-centric framework that offers a structured substrate for abstracting heterogeneous sensing sources. More importantly, it enables the collection, storage and discovery of observation and measurement data from both static and mobile sensing sources.
The Semantic Web is an extension to the current Web in which information is provided in machine-processable format. It allows interoperable data representation and expression of meaningful relationships between the information resources. In other words, it is envisaged with the supremacy of deduction capabilities on the Web, that being one of the limitations of the current Web. In a Semantic Web framework, an ontology provides a knowledge sharing structure. The research on Semantic Web in the past few years has offered an opportunity for conventional information search and retrieval systems to migrate from keyword to semantics-based methods. The fundamental difference is that the Semantic Web is not a Web of interlinked documents; rather, it is a Web of relations between resources denoting real world objects, together with well-defined metadata attached to those resources. In this chapter, we first investigate various approaches towards ontology development, ontology population from heterogeneous data sources, semantic association discovery, semantic association ranking and presentation, and social network analysis, and then we present our methodology for an ontology-based information search and retrieval. In particular, we are interested in developing efficient algorithms to resolve the semantic association discovery and analysis issues.
We consider the problem of anomaly detection in an audio-visual analysis system designed to interpret sequences of actions from visual and audio cues. The scene activity recognition is based on a generative framework, with a high-level inference model for contextual recognition of sequences of actions. The system is endowed with anomaly detection mechanisms, which facilitate differentiation of various types of anomalies. This is accomplished using intelligence provided by a classifier incongruence detector, classifier confidence module and data quality assessment system, in addition to the classical outlier detection module. The paper focuses on one of the mechanisms, the classifier incongruence detector, the purpose of which is to flag situations when the video and audio modalities disagree in action interpretation. We demonstrate the merit of using the Delta divergence measure for this purpose. We show that this measure significantly enhances the incongruence detection rate in the Human Action Manipulation complex activity recognition data set.
Although deep learning is the mainstream method in unsupervised anomalous sound detection, Gaussian Mixture Model (GMM) with statistical audio frequency representation as input can achieve comparable results with much lower model complexity and fewer parameters. Existing statistical frequency representations, e.g, the log-Mel spectrogram's average or maximum over time, do not always work well for different machines. This paper presents Time-Weighted Frequency Domain Representation (TWFR) with the GMM method (TWFR-GMM) for anomalous sound detection. The TWFR is a generalized statistical frequency domain representation that can adapt to different machine types, using the global weighted ranking pooling over time-domain. This allows GMM estimator to recognize anomalies, even under domain-shift conditions, as visualized with a Mahalanobis distance-based metric. Experiments on DCASE 2022 Challenge Task2 dataset show that our method has better detection performance than recent deep learning methods. TWFR-GMM is the core of our submission that achieved the 3rd place in DCASE 2022 Challenge Task2.
For learning-based sound event localization and detection (SELD) methods, different acoustic environments in the training and test sets may result in large performance differences in the validation and evaluation stages. Different environments, such as different sizes of rooms, different reverberation times, and different background noise, may be reasons for a learning-based system to fail. On the other hand, acquiring annotated spatial sound event samples, which include onset and offset time stamps, class types of sound events, and direction-of-arrival (DOA) of sound sources is very expensive. In addition, deploying a SELD system in a new environment often poses challenges due to time-consuming training and fine-tuning processes. To address these issues, we propose Meta-SELD, which applies meta-learning methods to achieve fast adaptation to new environments. More specifically, based on Model Agnostic Meta-Learning (MAML), the proposed Meta-SELD aims to find good meta-initialized parameters to adapt to new environments with only a small number of samples and parameter updating iterations. We can then quickly adapt the meta-trained SELD model to unseen environments. Our experiments compare fine-tuning methods from pre-trained SELD models with our Meta-SELD on the Sony-TAU Realistic Spatial Soundscapes 2023 (STARSSS23) dataset. The evaluation results demonstrate the effectiveness of Meta-SELD when adapting to new environments.
The work on 3D human pose estimation has seen a significant amount of progress in recent years, particularly due to the widespread availability of commodity depth sensors. However, most pose estimation methods follow a tracking-as-detection approach which does not explicitly handle occlusions, thus introducing outliers and identity association issues when multiple targets are involved. To address these issues, we propose a new method based on Probability Hypothesis Density (PHD) filter. In this method, the PHD filter with a novel clutter intensity model is used to remove outliers in the 3D head detection results, followed by an identity association scheme with occlusion detection for the targets. Experimental results show that our proposed method greatly mitigates the outliers, and correctly associates identities to individual detections with low computational cost.
This work proposes a simple but effective attention mechanism, namely Skip Attention (SA), for monaural singing voice separation (MSVS). First, the SA, embedded in the convolutional encoder-decoder network (CEDN), realizes an attention-driven and dependency modeling for the repetitive structures of the music source. Second, the SA, replacing the popular skip connection in the CEDN, effectively controls the flow of the low-level (vocal and musical) features to the output and improves the feature sensitivity and accuracy for MSVS. Finally, we implement the proposed SA on the Stacked Hourglass Network (SHN), namely Skip Attention SHN (SA-SHN). Quantitative and qualitative evaluation results have shown that the proposed SA-SHN achieves significant performance improvement on the MIR-1K dataset (compared to the state-of-the-art SHN) and competitive MSVS performance on the DSD100 dataset (compared to the state-of-the-art DenseNet), even without using any data augmentation methods.
In this paper, we propose an iterative deep neural network (DNN)-based binaural source separation scheme, for recovering two concurrent speech signals in a room environment. Besides the commonly-used spectral features, the DNN also takes non-linearly wrapped binaural spatial features as input, which are refined iteratively using parameters estimated from the DNN output via a feedback loop. Different DNN structures have been tested, including a classic multilayer perception regression architecture as well as a new hybrid network with both convolutional and densely-connected layers. Objective evaluations in terms of PESQ and STOI showed consistent improvement over baseline methods using traditional binaural features, especially when the hybrid DNN architecture was employed. In addition, our proposed scheme is robust to mismatches between the training and testing data.
Anomaly detection is the task of detecting outliers from normal data. Numerous methods have been proposed to address this problem , including recent methods based on generative adversarial network (GAN). However, these methods are limited in capturing the long-range information in data due to the limited receptive field obtained by the con-volution operation. The long-range information is crucial for producing distinctive representation for normal data belonging to different classes, while the local information is important for distinguishing normal data from abnormal data, if they belong to the same class. In this paper, we propose a novel Transformer-based architecture for anomaly detection which has advantages in extracting features with global information representing different classes as well as the local details useful for capturing anomalies. In our design, we introduce self-attention mechanism into the generator of GAN to extract global semantic information, and also modify the skip-connection to capture local details in multi-scale from input data. The experiments on CIFAR10 and STL10 show that our method provides better performance on representing different classes as compared with the state-of-the-art CNN-based GAN methods. Experiments performed on MVTecAD and LBOT datasets show that the proposed method offers state-of-the-art results, outperforming the baseline method SAGAN by over 3% in terms of the AUC metric.
Face super-resolution (FSR) is dedicated to the restoration of high-resolution (HR) face images from their low-resolution (LR) counterparts. Many deep FSR methods exploit facial prior knowledge (e.g., facial landmark and parsing map) related to facial structure information to generate HR face images. However, directly training a facial prior estimation network with deep FSR model requires manually labeled data, and is often computationally expensive. In addition, inaccurate facial priors may degrade super-resolution performance. In this paper, we propose a residual FSR method with spatial attention mechanism guided by mul-tiscale receptive-field features (MRF) for converting LR face images (i.e., 16 × 16) to HR face images (i.e., 128 × 128). With our spatial attention mechanism, we can recover local details in face images without explicitly learning the prior knowledge. Quantitative and qualitative experiments show that our method outperforms state-of-the-art FSR methods.
Target sound detection (TSD) aims to detect the target sound from a mixture audio given the reference information. Previous methods use a conditional network to extract a sound-discriminative embedding from the reference audio, and then use it to detect the target sound from the mixture audio. However , the network performs much differently when using different reference audios (e.g. performs poorly for noisy and short-duration reference audios), and tends to make wrong decisions for transient events (i.e. shorter than 1 second). To overcome these problems, in this paper, we present a reference-aware and duration-robust network (RaDur) for TSD. More specifically, in order to make the network more aware of the reference information , we propose an embedding enhancement module to take into account the mixture audio while generating the embedding , and apply the attention pooling to enhance the features of target sound-related frames and weaken the features of noisy frames. In addition, a duration-robust focal loss is proposed to help model different-duration events. To evaluate our method, we build two TSD datasets based on UrbanSound and Audioset. Extensive experiments show the effectiveness of our methods.
A non-intrusive method is introduced to predict binaural speech intelligibility in noise directly from signals captured using a pair of microphones. The approach combines signal processing techniques in blind source separation and localisation, with an intrusive objective intelligibility measure (OIM). Therefore, unlike classic intrusive OIMs, this method does not require a clean reference speech signal and knowing the location of the sources to operate. The proposed approach is able to estimate intelligibility in stationary and fluctuating noises, when the noise masker is presented as a point or diffused source, and is spatially separated from the target speech source on a horizontal plane. The performance of the proposed method was evaluated in two rooms. When predicting subjective intelligibility measured as word recognition rate, this method showed reasonable predictive accuracy with correlation coefficients above 0.82, which is comparable to that of a reference intrusive OIM in most of the conditions. The proposed approach offers a solution for fast binaural intelligibility prediction, and therefore has practical potential to be deployed in situations where on-site speech intelligibility is a concern.
This paper proposes a scheme for multiple un-manned aerial vehicles (UAVs) to track multiple targets in challenging 3-D environments while avoiding obstacle collisions. The scheme relies on Received-Signal-Strength-Indicator (RSSI) measurements to estimate and track target positions and uses a Q-Learning (QL) algorithm to enhance the intelligence of UAVs for autonomous navigation and obstacle avoidance. Considering the limitation of UAVs in their power and computing capacity, a global reward function is used to determine the optimal actions for the joint control of energy consumption, computation time, and tracking accuracy. Extensive simulations demonstrate the effectiveness of the proposed scheme, achieving accurate and efficient target tracking with low energy consumption.
Particle filters (PFs) have been widely used in speaker tracking due to their capability in modeling a non-linear process or a non-Gaussian environment. However, particle filters are limited by several issues. For example, pre-defined handcrafted measurements are often used which can limit the model performance. In addition, the transition and update models are often preset which make PF less flexible to be adapted to different scenarios. To address these issues, we propose an end-to-end differentiable particle filter framework by employing the multi-head attention to model the long-range dependencies. The proposed model employs the self-attention as the learned transition model and the cross-attention as the learned update model. To our knowledge, this is the first proposal of combining particle filter and transformer for speaker tracking, where the measurement extraction, transition and update steps are integrated into an end-to-end architecture. Experimental results show that the proposed model achieves superior performance over the recurrent baseline models.
Interpolated discrete Fourier transform (DFT) is a well-known method for frequency estimation of complex sinusoids. For signals without windowing (or with rectangular-windowing), this has been well investigated and a large number of estimators have been developed. However, very few algorithms have been developed for windowed signals so far. In this paper, we extend the well-known Jacobsen estimator to windowed signals. The extension is deduced from the fact that an arbitrary cosine-sum window functions are composed of complex sinusoids. Consequently, the Jacobsen estimator for windowed signals can be formulated as an algebraic equation with no approximation and thus an analytical solution to the estimator can be obtained. Simulation results show that our approach improves the performance in comparison with the conventional interpolated DFT algorithms for windowed signals.
The Internet of Things enables human beings to better interact with and understand their surrounding environments by extending computational capabilities to the physical world. A critical driving force behind this is the rapid development and wide deployment of wireless sensor networks, which continuously produce a large amount of real-world data for many application domains. Similar to many other large-scale distributed technologies, interoperability and scalability are the prominent and persistent challenges. The proposal of sensor-as-a-service aims to address these challenges; however, to our knowledge, there are no concrete implementations of techniques to support the idea, in particular, large-scale, distributed sensor service discovery. Based on the distinctive characteristics of the sensor services, we develop a scalable discovery architecture using geospatial indexing techniques and semantic service technologies. We perform extensive experimental studies to verify the performance of the proposed method and its applicability to large-scale, distributed sensor service discovery.
The probability hypothesis density (PHD) filter is well known for addressing the problem of multiple human tracking for a variable number of targets, and the sequential Monte Carlo (SMC) implementation of the PHD filter, known as the particle PHD filter, can give state estimates with nonlinear and non-Gaussian models. Recently, Mahler et al. have introduced a PHD smoother to gain more accurate estimates for both target states and number. However, as highlighted by Psiaki in the context of a backward-smoothing extended Kalman filter, with a non-linear state evolution model the approximation error in the backward filtering requires careful consideration. Psiaki suggests to minimise the aggregated least-squares error over a batch of data. We instead use the term retrodiction PHD (Retro-PHD) filter to describe the backward filtering algorithm in recognition of the approximation error proposed in the original PHD smoother, and we propose an adaptive recursion step to improve the approximation accuracy. This step combines forward and backward processing through the measurement set and thereby mitigates the problems with the original PHD smoother when the target number changes significantly and the targets appear and disappear randomly. Simulation results show the improved performance of the proposed algorithm and its capability in handling a variable number of targets.
We consider the dictionary learning problem for the analysis model based sparse representation. A novel algorithm is proposed by adapting the synthesis model based simultaneous codeword optimisation (SimCO) algorithm to the analysis model. This algorithm assumes that the analysis dictionary contains unit Ł-norm atoms and trains the dictionary by the optimisation on manifolds. This framework allows one to update multiple dictionary atoms in each iteration, leading to a computationally efficient optimisation process. We demonstrate the competitive performance of the proposed algorithm using experiments on both synthetic and real data, as compared with three baseline algorithms, Analysis K-SVD, analysis operator learning (AOL) and learning overcomplete sparsifying transforms (LOST), respectively. © 2014 IEEE.
Recently proposed model-based methods use timefrequency (T-F) masking for source separation, where the T-F masks are derived from various cues described by a frequency domain Gaussian Mixture Model (GMM). These methods work well for separating mixtures recorded in low-to-medium level of reverberation, however, their performance degrades as the level of reverberation is increased. We note that the relatively poor performance of these methods under reverberant conditions can be attributed to the high variance of the frequency-dependent GMM parameter estimates. To address this limitation, a novel bootstrap-based approach is proposed to improve the accuracy of expectation maximization (EM) estimates of a frequencydependent GMM based on an a priori chosen initialization scheme. It is shown how the proposed technique allows us to construct time-frequency masks which lead to improved model-based source separation for reverberant speech mixtures. Experiments and analysis are performed on speech mixtures formed using real room-recorded impulse responses.
We focus on the dictionary learning problem for the analysis model. A simple but effective algorithm based on Nesterov's gradient is proposed. This algorithm assumes that the analysis dictionary contains unit ℓ norm atoms and trains the dictionary iteratively with Nesterov's gradient. We show that our proposed algorithm is able to learn the dictionary effectively with experiments on synthetic data. We also present examples demonstrating the promising performance of our algorithm in despeckling synthetic aperture radar (SAR) images. © 2014 IEEE.
Using an acoustic vector sensor (AVS), an efficient method has been presented recently for direction-of-arrival (DOA) estimation of multiple speech sources via the clustering of the inter-sensor data ratio (AVS-ISDR). Through extensive experiments on simulated and recorded data, we observed that the performance of the AVS-DOA method is largely dependent on the reliable extraction of the target speech dominated time-frequency points (TD-TFPs) which, however, may be degraded with the increase in the level of additive noise and room reverberation in the background. In this paper, inspired by the great success of deep learning in speech recognition, we design two new soft mask learners, namely deep neural network (DNN) and DNN cascaded with a support vector machine (DNN-SVM), for multi-source DOA estimation, where a novel feature, namely, the tandem local spectrogram block (TLSB) is used as the input to the system. Using our proposed soft mask learners, the TD-TFPs can be accurately extracted under different noisy and reverberant conditions. Additionally, the generated soft masks can be used to calculate the weighted centers of the ISDR-clusters for better DOA estimation as compared with the original center used in our previously proposed AVS-ISDR. Extensive experiments on simulated and recorded data have been presented to show the improved performance of our proposed methods over two baseline AVS-DOA methods in presence of noise and reverberation.
Particle filtering has emerged as a useful tool for tracking problems. However, the efficiency and accuracy of the filter usually depend on the number of particles and noise variance used in the estimation and propagation functions for re-allocating these particles at each iteration. Both of these parameters are specified beforehand and are kept fixed in the regular implementation of the filter which makes the tracker unstable in practice. In this paper we are interested in the design of a particle filtering algorithm which is able to adapt the number of particles and noise variance. The new filter, which is based on audio-visual (AV) tracking, uses information from the tracking errors to modify the number of particles and noise variance used. Its performance is compared with a previously proposed audio-visual particle filtering algorithm with a fixed number of particles and an existing adaptive particle filtering algorithm, using the AV 16.3 dataset with single and multi-speaker sequences. Our proposed approach demonstrates good tracking performance with a significantly reduced number of particles. © 2013 EURASIP.
Metric learning plays a fundamental role in the fields of multimedia retrieval and pattern recognition. Recently, an online multi-kernel similarity (OMKS) learning method has been presented for content-based image retrieval (CBIR), which was shown to be promising for capturing the intrinsic nonlinear relations within multimodal features from large-scale data. However, the similarity function in this method is learned only from labeled images. In this paper, we present a new framework to exploit unlabeled images and develop a semi-supervised OMKS algorithm. The proposed method is a multi-stage algorithm consisting of feature selection, selective ensemble learning, active sample selection and triplet generation. The novel aspects of our work are the introduction of classification confidence to evaluate the labeling process and select the reliably labeled images to train the metric function, and a method for reliable triplet generation, where a new criterion for sample selection is used to improve the accuracy of label prediction for unlabelled images. Our proposed method offers advantages in challenging scenarios, in particular, for a small set of labeled images with high-dimensional features. Experimental results demonstrate the effectiveness of the proposed method as compared with several baseline methods.
Binaural features of interaural level difference and interaural phase difference have proved to be very effective in training deep neural networks (DNNs), to generate timefrequency masks for target speech extraction in speech-speech mixtures. However, effectiveness of binaural features is reduced in more common speech-noise scenarios, since the noise may over-shadow the speech in adverse conditions. In addition, the reverberation also decreases the sparsity of binaural features and therefore adds difficulties to the separation task. To address the above limitations, we highlight the spectral difference between speech and noise spectra and incorporate the log-power spectra features to extend the DNN input. Tested on two different reverberant rooms at different signal to noise ratios (SNR), our proposed method shows advantages over the baseline method using only binaural features in terms of signal to distortion ratio (SDR) and Short-Time Perceptual Intelligibility (STOI).
Boundary estimation from an acoustic room impulse response (RIR), exploiting known sound propagation behavior, yields useful information for various applications: e.g., source separation, simultaneous localization and mapping, and spatial audio. The baseline method, an algorithm proposed by Antonacci et al., uses reflection times of arrival (TOAs) to hypothesize reflector ellipses. Here, we modify the algorithm for 3-D environments and for enhanced noise robustness: DYPSA and MUSIC for epoch detection and direction of arrival (DOA) respectively are combined for source localization, and numerical search is adopted for reflector estimation. Both methods, and other variants, are tested on measured RIR data; the proposed method performs best, reducing the estimation error by 30%.
Audio captioning aims at using language to describe the content of an audio clip. Existing audio captioning systems are generally based on an encoder-decoder architecture, in which acoustic information is extracted by an audio encoder and then a language decoder is used to generate the captions. Training an audio captioning system often encounters the problem of data scarcity. Transferring knowledge from pre-trained audio models such as Pre-trained Audio Neural Networks (PANNs) have recently emerged as a useful method to mitigate this issue. However, there is less attention on exploiting pre-trained language models for the decoder, compared with the encoder. BERT is a pre-trained language model that has been extensively used in natural language processing tasks. Nevertheless, the potential of using BERT as the language decoder for audio captioning has not been investigated. In this study, we demonstrate the efficacy of the pre-trained BERT model for audio captioning. Specifically, we apply PANNs as the encoder and initialize the decoder from the publicly available pre-trained BERT models. We conduct an empirical study on the use of these BERT models for the decoder in the audio captioning model. Our models achieve competitive results with the existing audio captioning methods on the AudioCaps dataset.
—Training a robust tracker of objects (such as vehicles and people) using audio and visual information often needs a large amount of labelled data, which is difficult to obtain as manual annotation is expensive and time-consuming. The natural synchronization of the audio and visual modalities enables the object tracker to be trained in a self-supervised manner. In this work, we propose to localize an audio source (i.e., speaker) using a teacher-student paradigm, where the visual network teaches the audio network by knowledge distillation to localize speakers. The introduction of multi-task learning, by training the audio network to perform source localization and semantic segmentation jointly, further improves the model performance. Experimental results show that the audio localization network can learn from visual information and achieve competitive tracking performance as compared to the baseline methods that are based on the audio-only measurements. The proposed method can provide more reliable measurements for tracking than the traditional sound source localization methods, and the generated audio features aid in visual tracking.
—Human emotions can be presented in data with multiple modalities, e.g. video, audio and text. An automated system for emotion recognition needs to consider a number of challenging issues, including feature extraction, and dealing with variations and noise in data. Deep learning have been extensively used recently, offering excellent performance in emotion recognition. This work presents a new method based on audio and visual modalities, where visual cues facilitate the detection of the speech or non-speech frames and the emotional state of the speaker. Different from previous works, we propose the use of novel speech features, e.g. the Wavegram, which is extracted with a one-dimensional Convolutional Neural Network (CNN) learned directly from time-domain waveforms, and Wavegram-Logmel features which combines the Wavegram with the log mel spectrogram. The system is then trained in an end-to-end fashion on the SAVEE database by also taking advantage of the correlations among each of the streams. It is shown that the proposed approach outperforms the traditional and state-of-the art deep learning based approaches, built separately on auditory and visual handcrafted features for the prediction of spontaneous and natural emotions.
Environmental audio tagging aims to predict only the presence or absence of certain acoustic events in the interested acoustic scene. In this paper we make contributions to audio tagging in two parts, respectively, acoustic modeling and feature learning. We propose to use a shrinking deep neural network (DNN) framework incorporating unsupervised feature learning to handle the multi-label classification task. For the acoustic modeling, a large set of contextual frames of the chunk are fed into the DNN to perform a multi-label classification for the expected tags, considering that only chunk (or utterance) level rather than frame-level labels are available. Dropout and background noise aware training are also adopted to improve the generalization capability of the DNNs. For the unsupervised feature learning, we propose to use a symmetric or asymmetric deep de-noising auto-encoder (syDAE or asyDAE) to generate new data-driven features from the logarithmic Mel-Filter Banks (MFBs) features. The new features, which are smoothed against background noise and more compact with contextual information, can further improve the performance of the DNN baseline. Compared with the standard Gaussian Mixture Model (GMM) baseline of the DCASE 2016 audio tagging challenge, our proposed method obtains a significant equal error rate (EER) reduction from 0.21 to 0.13 on the development set. The proposed asyDAE system can get a relative 6.7% EER reduction compared with the strong DNN baseline on the development set. Finally, the results also show that our approach obtains the state-of-the-art performance with 0.15 EER on the evaluation set of the DCASE 2016 audio tagging task while EER of the first prize of this challenge is 0.17.
One of the obstacles in developing speech emotion recognition (SER) systems is the data scarcity problem, i.e., the lack of labeled data for training these systems. Data augmentation is an effective method for increasing the amount of training data. In this paper, we propose a cycle-generative adversarial network (cycle-GAN) for data augmentation in the SER systems. For each of the five emotions considered, an adversarial network is designed to generate data that have a similar distribution to the main data in that class but have a different distribution to those of other classes. These networks are trained in an adversarial way to produce feature vectors similar to those in the training set, which are then added to the original training sets. Instead of using the common cross-entropy loss to train cycle-GANs, we use the Wasserstein divergence to mitigate the gradient vanishing problem and to generate high-quality samples. The proposed network has been applied to SER using the EMO-DB dataset. The quality of the generated data is evaluated using two classifiers based on support vector machine and deep neural network. The results showed that the recognition accuracy in unweighted average recall was about 83.33%, which is better than the baseline methods compared.
Polyphonic sound event detection aims to detect the types of sound events that occur in given audio clips, and their onset and offset times, in which multiple sound events may occur simultaneously. Deep learning-based methods such as convolutional neural networks (CNN) achieved state-of-the-art results in polyphonic sound event detection. However, two open challenges still remain: overlap between events and prone to overfitting problem. To solve the above two problems, we proposed a capsule network-based method for polyphonic sound event detection. With so-called dynamic routing, capsule networks have the advantage of handling overlapping objects and the generalization ability to reduce overfitting. However, dynamic routing also greatly slows down the training process. In order to speed up the training process, we propose a weakly labeled polyphonic sound event detection model based on the improved capsule routing. Our proposed method is evaluated on task 4 of the DCASE 2017 challenge and compared with several baselines, demonstrating competitive results in terms of F-score and computational efficiency.
Unsupervised anomalous sound detection aims to detect unknown abnormal sounds of machines from normal sounds. However, the state-of-the-art approaches are not always stable and perform dramatically differently even for machines of the same type, making it impractical for general applications. This paper proposes a spectral-temporal fusion based self-supervised method to model the feature of the normal sound, which improves the stability and performance consistency in detection of anomalous sounds from individual machines, even of the same type. Experiments on the DCASE 2020 Challenge Task 2 dataset show that the proposed method achieved 81.39%, 83.48%, 98.22% and 98.83% in terms of the minimum AUC (worst-case detection performance amongst individuals) in four types of real machines (fan, pump, slider and valve), respectively, giving 31.79%, 17.78%, 10.42% and 21.13% improvement compared to the state-of-the-art method, i.e., Glow_Aff. Moreover, the proposed method has improved AUC (average performance of individuals) for all the types of machines in the dataset. The source codes are available at https://github.com/liuyoude/STgram_MFN
Audio signal classification is usually done using conventional signal features such as mel-frequency cepstrum coefficients (MFCC), line spectral frequencies (LSF), and short time energy (STM). Learned dictionaries have been shown to have promising capability for creating sparse representation of a signal and hence have a potential to be used for the extraction of signal features. In this paper, we consider to use sparse features for audio classification from music and speech data. We use the K-SVD algorithm to learn separate dictionaries for the speech and music signals to represent their respective subspaces and use them to extract sparse features for each class of signals using Orthogonal Matching Pursuit (OMP). Based on these sparse features, Support Vector Machines (SVM) are used for speech and music classification. The same signals were also classified using SVM based on the conventional MFCC coefficients and the classification results were compared to those of sparse coefficients. It was found that at lower signal to noise ratio (SNR), sparse coefficients give far better signal classification results as compared to the MFCC based classification.
Musical noise is a recurrent issue that appears in spectral techniques for denoising or blind source separation. Due to localised errors of estimation, isolated peaks may appear in the processed spectrograms, resulting in annoying tonal sounds after synthesis known as “musical noise”. In this paper, we propose a method to assess the amount of musical noise in an audio signal, by characterising the impact of these artificial isolated peaks on the processed sound. It turns out that because of the constraints between STFT coefficients, the isolated peaks are described as time-frequency “spots” in the spectrogram of the processed audio signal. The quantification of these “spots”, achieved through the adaptation of a method for localisation of significant STFT regions, allows for an evaluation of the amount of musical noise. We believe that this will pave the way to an objective measure and a better understanding of this phenomenon.
The state of classfier incongruence in decision making systems incorporating multiple classifiers is often an indicator of anomaly caused by an unexpected observation or an unusual situation. Its assessment is important as one of the key mechanisms for domain anomaly detection. In this paper, we investigate the sensitivity of Delta divergence, a novel measure of classifier incongruence, to estimation errors. Statistical properties of Delta divergence are analysed both theoretically and experimentally. The results of the analysis provide guidelines on the selection of threshold for classifier incongruence detection based on this measure.
—Acoustic scene classification (ASC) aims to classify an audio clip based on the characteristic of the recording environment. In this regard, deep learning based approaches have emerged as a useful tool for ASC problems. Conventional approaches to improving the classification accuracy include integrating auxiliary methods such as attention mechanism, pre-trained models and ensemble multiple sub-networks. However, due to the complexity of audio clips captured from different environments, it is difficult to distinguish their categories without using any auxiliary methods for existing deep learning models using only a single classifier. In this paper, we propose a novel approach for ASC using deep neural decision forest (DNDF). DNDF combines a fixed number of convolutional layers and a decision forest as the final classifier. The decision forest consists of a fixed number of decision tree classifiers, which have been shown to offer better classification performance than a single classifier in some datasets. In particular, the decision forest differs substantially from traditional random forests as it is stochastic, differentiable, and capable of using the back-propagation to update and learn feature representations in neural network. Experimental results on the DCASE2019 and ESC-50 datasets demonstrate that our proposed DNDF method improves the ASC performance in terms of classification accuracy and shows competitive performance as compared with state-of-the-art baselines.
—Unmanned aerial vehicles (UAVs) are useful devices due to their great manoeuvrability for long-range outdoor target tracking. However, these tracking tasks can lead to sub-optimal performance due to high computation requirements and power constraints. To cope with these challenges, we design a UAV-based target tracking algorithm where computationally intensive tasks are offloaded to Edge Computing (EC) servers. We perform joint optimization by considering the trade-off between transmission energy consumption and execution time to determine optimal edge nodes for task processing and reliable tracking. The simulation results demonstrate the superiority of the proposed UAV-based target tracking on the predefined trajectory over several existing techniques. Index Terms—Edge computing (EC), task offloading, un-manned aerial vehicle (UAV)
Most existing analysis dictionary learning (ADL) algorithms, such as the Analysis K-SVD, assume that the original signals are known or can be correctly estimated. Usually the signals are unknown and need to be estimated from its noisy versions with some computational efforts. When the noise level is high, estimation of the signals becomes unreliable. In this paper, a simple but effective ADL algorithm is proposed, where we directly employ the observed data to compute the approximate analysis sparse representation of the original signals. This eliminates the need for estimating the original signals as otherwise required in the Analysis K-SVD. The analysis sparse representation can be exploited to assign the observed data into multiple subsets, which are then used for updating the analysis dictionary. Experiments on synthetic data and natural image denoising demonstrate its advantage over the baseline algorithm, Analysis K-SVD. © 2013 EURASIP.
An OMP-like Covariance-Assisted Matching Pursuit (CAMP) method has recently been proposed. Given a priorknowledge of the covariance and mean of the sparse coefficients, CAMP balances the least squares estimator and the priorknowledge by leveraging the Gauss-Markov theorem. In this letter, we study the performance of CAMP in the framework of restricted isometry property (RIP). It is shown that under some conditions on RIP and the minimum magnitude of the nonzero elements of the sparse signal, CAMP with sparse level K can recover the exact support of the sparse signal from noisy measurements. l2 bounded noise and Gaussian noise are considered in our analysis.We also discuss the extreme conditions of noise (e.g. the noise power is infinite) to simply show the stability of CAMP.
Intensity Particle Flow (IPF) SMC-PHD has been proposed recently for multi-target tracking. In this paper, we extend IPF-SMC-PHD filter to distributed setting, and develop a novel consensus method for fusing the estimates from individual sensors, based on Arithmetic Average (AA) fusion. Different from conventional AA method which may be degraded when unreliable estimates are presented, we develop a novel arithmetic consensus method to fuse estimates from each individual IPF-SMC-PHD filter with partial consensus. The proposed method contains a scheme for evaluating the reliability of the sensor nodes and preventing unreliable sensor information to be used in fusion and communication in sensor network, which help improve fusion accuracy and reduce sensor communication costs. Numerical simulations are performed to demonstrate the advantages of the proposed algorithm over the uncooperative IPF-SMC-PHD and distributed particle-PHD with AA fusion.
Audio captioning aims at generating natural language descriptions for audio clips automatically. Existing audio captioning models have shown promising improvement in recent years. However, these models are mostly trained via maximum likelihood estimation (MLE), which tends to make captions generic, simple and deterministic. As different people may describe an audio clip from different aspects using distinct words and grammars, we argue that an audio captioning system should have the ability to generate diverse captions for a fixed audio clip and across similar audio clips. To address this problem, we propose an adversarial training framework for audio captioning based on a conditional generative adversarial network (C-GAN), which aims at improving the naturalness and diversity of generated captions. Unlike processing data of continuous values in a classical GAN, a sentence is composed of discrete tokens and the discrete sampling process is non-differentiable. To address this issue, policy gradient, a reinforcement learning technique, is used to back-propagate the reward to the generator. The results show that our proposed model can generate more diverse captions, as compared to state-of-the-art methods.
We address the problem of sparse signal reconstruction from a few noisy samples. Recently, a Covariance-Assisted Matching Pursuit (CAMP) algorithm has been proposed, improving the sparse coefficient update step of the classic Orthogonal Matching Pursuit (OMP) algorithm. CAMP allows the a-priori mean and covariance of the non-zero coefficients to be considered in the coefficient update step. In this paper, we analyze CAMP, which leads to a new interpretation of the update step as a maximum-a-posteriori (MAP) estimation of the non-zero coefficients at each step. We then propose to leverage this idea, by finding a MAP estimate of the sparse reconstruction problem, in a greedy OMP-like way. Our approach allows the statistical dependencies between sparse coefficients to be modelled, while keeping the practicality of OMP. Experiments show improved performance when reconstructing the signal from a few noisy samples.
Representing a complex acoustic scene with audio objects is desirable but challenging in object-based spatial audio production and reproduction, especially when concurrent sound signals are present in the scene. Source separation (SS) provides a potentially useful and enabling tool for audio object extraction. These extracted objects are often remixed to reconstruct a sound field in the reproduction stage. A suitable SS method is expected to produce audio objects that ultimately deliver high quality audio after remix. The performance of these SS algorithms therefore needs to be evaluated in this context. Existing metrics for SS performance evaluation, however, do not take into account the essential sound field reconstruction process. To address this problem, here we propose a new SS evaluation method which employs a remixing strategy similar to the panning law, and provides a framework to incorporate the conventional SS metrics. We have tested our proposed method on real-room recordings processed with four SS methods, including two state-of-the art blind source separation (BSS) methods and two classic beamforming algorithms. The evaluation results based on three conventional SS metrics are analysed.
In this paper, we present a deep neural network (DNN)-based acoustic scene classification framework. Two hierarchical learning methods are proposed to improve the DNN baseline performance by incorporating the hierarchical taxonomy information of environmental sounds. Firstly, the parameters of the DNN are initialized by the proposed hierarchical pre-training. Multi-level objective function is then adopted to add more constraint on the cross-entropy based loss function. A series of experiments were conducted on the Task1 of the Detection and Classification of Acoustic Scenes and Events (DCASE) 2016 challenge. The final DNN-based system achieved a 22.9% relative improvement on average scene classification error as compared with the Gaussian Mixture Model (GMM)-based benchmark system across four standard folds.
At the University of Surrey (Guildford, UK), we have brought together research groups in different disciplines, with a shared interest in audio, to work on a range of collaborative research projects. In the Centre for Vision, Speech and Signal Processing (CVSSP) we focus on technologies for machine perception of audio scenes; in the Institute of Sound Recording (IoSR) we focus on research into human perception of audio quality; the Digital World Research Centre (DWRC) focusses on the design of digital technologies; while the Centre for Digital Economy (CoDE) focusses on new business models enabled by digital technology. This interdisciplinary view, across different traditional academic departments and faculties, allows us to undertake projects which would be impossible for a single research group. In this poster we will present an overview of some of these interdisciplinary projects, including projects in spatial audio, sound scene and event analysis, and creative commons audio.
We propose a new method for source separation by synthesizing the source from a speech mixture corrupted by various environmental noise. Unlike traditional source separation methods which estimate the source from the mixture as a replica of the original source (e.g. by solving an inverse problem), our proposed method is a synthesis-based approach which aims to generate a new signal (i.e. “fake” source) that sounds similar to the original source. The proposed system has an encoder-decoder topology, where the encoder predicts intermediate-level features from the mixture, i.e. Mel-spectrum of the target source, using a hybrid recurrent and hourglass network, while the decoder is a state-of-the-art WaveNet speech synthesis network conditioned on the Mel-spectrum, which directly generates time-domain samples of the sources. Both objective and subjective evaluations were performed on the synthesized sources, and show great advantages of our proposed method for high-quality speech source separation and generation.
A new approach for convolutive blind source separation (BSS) by explicitly exploiting the second-order nonstationarity of signals and operating in the frequency domain is proposed. The algorithm accommodates a penalty function within the cross-power spectrum-based cost function and thereby converts the separation problem into a joint diagonalization problem with unconstrained optimization. This leads to a new member of the family of joint diagonalization criteria and a modification of the search direction of the gradient-based descent algorithm. Using this approach, not only can the degenerate solution induced by a null unmixing matrix and the effect of large errors within the elements of covariance matrices at low-frequency bins be automatically removed, but in addition, a unifying view to joint diagonalization with unitary or nonunitary constraint is provided. Numerical experiments are presented to verify the performance of the new method, which show that a suitable penalty function may lead the algorithm to a faster convergence and a better performance for the separation of convolved speech signals, in particular, in terms of shape preservation and amplitude ambiguity reduction, as compared with the conventional second-order based algorithms for convolutive mixtures that exploit signal nonstationarity. © 2005 IEEE.
Probabilistic models of binaural cues, such as the interaural phase difference (IPD) and the interaural level difference (ILD), can be used to obtain the audio mask in the time-frequency (TF) domain, for source separation of binaural mixtures. Those models are, however, often degraded by acoustic noise. In contrast, the video stream contains relevant information about the synchronous audio stream that is not affected by acoustic noise. In this paper, we present a novel method for modeling the audio-visual (AV) coherence based on dictionary learning. A visual mask is constructed from the video signal based on the learnt AV dictionary, and incorporated with the audio mask to obtain a noise-robust audio-visual mask, which is then applied to the binaural signal for source separation. We tested our algorithm on the XM2VTS database, and observed considerable performance improvement for noise corrupted signals.
This paper describes a semantic modelling scheme, a naming convention and a data distribution mechanism for sensor streams. The proposed solutions address important challenges to deal with large-scale sensor data emerging from the Internet of Things resources. While there are significant numbers of recent work on semantic sensor networks, semantic annotation and representation frameworks, there has been less focus on creating efficient and flexible schemes to describe the sensor streams and the observation and measurement data provided via these streams and to name and resolve the requests to these data. We present our semantic model to describe the sensor streams, demonstrate an annotation and data distribution framework and evaluate our solutions with a set of sample datasets. The results show that our proposed solutions can scale for large number of sensor streams with different types of data and various attributes.
In a recent study, it was shown that, given only the magnitude of the short-time Fourier transform (STFT) of a signal, it is possible to recover the phase information of its STFT under certain conditions. However, this is only investigated for the single-source scenario. In this paper , we extend this work and formulate a multi-source phase re- trieval problem where multi-channel phaseless STFT measurements are given as input . We then present a robust multi-source phase retrieval (RMSPR) algorithm based on a gradient descent (GD) algorithm by minimizing a non-convex loss function and inde- pendent component analysis (ICA). An improved least squares (LS) loss function is presented to find the initialization of the GD algorithm. Experimental evaluation has been conducted to show that under appropriate conditions the proposed algorithm can explicitly recover the phase of the sources, the mixing matrix, and the sources simulta- neously, from noisy measurements.
The problem of blind source separation (BSS) is investigated. Following the assumption that the time-frequency (TF) distributions of the input sources do not overlap, quadratic TF representation is used to exploit the sparsity of the statistically nonstationary sources. However, separation performance is shown to be limited by the selection of a certain threshold in classifying the eigenvectors of the TF matrices drawn from the observation mixtures. Two methods are, therefore, proposed based on recently introduced advanced clustering techniques, namely Gap statistics and self-splitting competitive learning (SSCL), to mitigate the problem of eigenvector classification. The novel integration of these two approaches successfully overcomes the problem of artificial sources induced by insufficient knowledge of the threshold and enables automatic determination of the number of active sources over the observation. The separation performance is thereby greatly improved. Practical consequences of violating the TF orthogonality assumption in the current approach are also studied, which motivates the proposal of a new solution robust to violation of orthogonality. In this new method, the TF plane is partitioned into appropriate blocks and source separation is thereby carried out in a block-by-block manner. Numerical experiments with linear chirp signals and Gaussian minimum shift keying (GMSK) signals are included which support the improved performance of the proposed approaches. © 2006 IEEE.
Automated audio captioning is a cross-modal translation task that aims to generate natural language descriptions for given audio clips. This task has received increasing attention with the release of freely available datasets in recent years. The problem has been addressed predominantly with deep learning techniques. Numerous approaches have been proposed, such as investigating different neural network architectures, exploiting auxiliary information such as keywords or sentence information to guide caption generation, and employing different training strategies, which have greatly facilitated the development of this field. In this paper, we present a comprehensive review of the published contributions in automated audio captioning, from a variety of existing approaches to evaluation metrics and datasets. We also discuss open challenges and envisage possible future research directions.
Most existing speech source separation algorithms have been developed for separating sound mixtures acquired by using a conventional microphone array. In contrast, little attention has been paid to the problem of source separation using an acoustic vector sensor (AVS). We propose a new method for the separation of convolutive mixtures by incorporating the intensity vector of the acoustic field, obtained using spatially co-located microphones which carry the direction of arrival (DOA) information. The DOA cues from the intensity vector, together with the frequency bin-wise mixing vector cues, are then used to determine the probability of each time-frequency (T-F) point of the mixture being dominated by a specific source, based on the Gaussian mixture models (GMM), whose parameters are evaluated and refined iteratively using an expectation-maximization (EM) algorithm. Finally, the probability is used to derive the T-F masks for recovering the sources. The proposed method is evaluated in simulated reverberant environments in terms of signal-to-distortion ratio (SDR), giving an average improvement of approximately 1:5 dB as compared with a related T-F mask approach based on a conventional microphone setting. © 2013 EURASIP.
With the fast development of information acquisition, there is a rapid growth of multimodality data, e.g., text, audio, image and even video, in fields of health care, multimedia retrieval and scientific research. Confronted with the challenges of clustering, classification or regression with multi-modality information, it is essential to effectively measure the distance or similarity between objects described with heterogeneous features. Metric learning, aimed at finding a task-oriented distance function, is a hot topic in machine learning. However, most existing algorithms lack efficiency for highdimensional multi-modality tasks. In this work, we develop an effective and efficient metric learning algorithm for multi-modality data, i.e., Efficient Multi-modal Geometric Mean Metric Learning (EMGMML). The proposed algorithm learns a distinctive distance metric for each view by minimizing the distance between similar pairs while maximizing the distance between dissimilar pairs. To avoid overfitting, the optimization objective is regularized by symmetrized LogDet divergence. EMGMML is very efficient in that there is a closed-formsolution for each distance metric. Experiment results show that the proposed algorithm outperforms the state-of-the-art metric learning methods in terms of both accuracy and efficiency.
The sequential Monte Carlo probability hypothesis density (SMC-PHD) filter assisted by particle flows (PF) has been shown to be promising for audio-visual multi-speaker tracking. A clustering step is often employed for calculating the particle flow, which leads to a substantial increase in the computational cost. To address this issue, we propose an alternative method based on the labelled non-zero particle flow (LNPF) to adjust the particle states. Results obtained from the AV16.3 dataset show improved performance by the proposed method in terms of computational efficiency and tracking accuracy as compared with baseline AV-NPF-SMC-PHD methods.
We propose an algorithm for the estimation of reverberation time (RT) from the reverberant speech signal by using a maximum likelihood (ML) estimator. Based on the analysis of an existing RT estimation method, which models the reverberation decay as a Gaussian random process modulated by a deterministic envelope, a Laplacian distribution based decay model is proposed in which an efficient procedure for locating free decay from reverberant speech is also incorporated. Then the RT is estimated from the free decays by the ML estimator. The method was motivated by our observation that the distribution pattern for temporal decay of the reverberant hand clap is much closer to the Laplace distribution. The estimation accuracy of the proposed method is evaluated using the experimental results and is in good agreement with the RT values measured from room impulse responses. © 2012 EURASIP.
Acoustic vector sensor (AVS) based convolutive blind source separation problem has been recently addressed under the framework of probabilistic time-frequency (T-F) masking, where both the DOA and the mixing vector cues are modelled by Gaussian distributions. In this paper, we show that the distributions of these cues vary with room acoustics, such as reverberation. Motivated by this observation, we propose a mixed model of Laplacian and Gaussian distributions to provide a better fit for these cues. The parameters of the mixed model are estimated and refined iteratively by an expectation-maximization (EM) algorithm. Experiments performed on the speech mixtures in simulated room environments show that the mixed model offers an average of about 0.68 dB and 1.18 dB improvements in signal-to-distotion (SDR) over the Gaussian and Laplacian model, respectively. © 2013 IEEE.
This paper presents a new method for reverberant speech separation, based on the combination of binaural cues and blind source separation (BSS) for the automatic classification of the time-frequency (T-F) units of the speech mixture spectrogram. The main idea is to model interaural phase difference, interaural level difference and frequency bin-wise mixing vectors by Gaussian mixture models for each source and then evaluate that model at each T-F point and assign the units with high probability to that source. The model parameters and the assigned regions are refined iteratively using the Expectation-Maximization (EM) algorithm. The proposed method also addresses the permutation problem of the frequency domain BSS by initializing the mixing vectors for each frequency channel. The EM algorithm starts with binaural cues and after a few iterations the estimated probabilistic mask is used to initialize and re-estimate the mix- ing vector model parameters. We performed experiments on speech mixtures, and showed an average of about 0.8 dB improvement in signal-to-distortion (SDR) over the binaural-only baseline
Although prototypical network (ProtoNet) has proved to be an effective method for few-shot sound event detection, two problems still exist. Firstly, the small-scaled support set is insufficient so that the class prototypes may not represent the class center accurately. Secondly, the feature extractor is task-agnostic (or class-agnostic): the feature extractor is trained with base-class data and directly applied to unseen-class data. To address these issues, we present a novel mutual learning framework with transductive learning, which aims at iteratively updating the class prototypes and feature extractor. More specifically, we propose to update class prototypes with transductive inference to make the class prototypes as close to the true class center as possible. To make the feature extractor to be task-specific, we propose to use the updated class prototypes to fine-tune the feature extractor. After that, a fine-tuned feature extractor further helps produce better class prototypes. Our method achieves the F-score of 38.4% on the DCASE 2021 Task 5 evaluation set, which won the first place in the few-shot bioacoustic event detection task of Detection and Classification of Acoustic Scenes and Events (DCASE) 2021 Challenge.
Additional publications
#publications