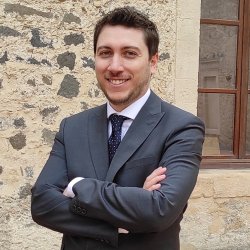
Dr Giovanni Iacobello
Academic and research departments
Turbulence, Centre for Aerodynamics and Environmental Flow, School of Mechanical Engineering Sciences, Institute for Sustainability.About
University roles and responsibilities
- Sustainability Institute Representative at Surrey Research Compute (SRC) Stakeholders Group
My qualifications
ResearchResearch interests
Research Topics:
- Wall-bounded turbulence;
- Lagrangian mixing and dispersion;
- Unsteady aerodynamics;
- Non-equilibrium processes;
- Data-driven modelling of fluid flows:
- Nonlinear time-series analysis;
- Reduced-order methods;
- Time-series estimation.
Research interests
Research Topics:
- Wall-bounded turbulence;
- Lagrangian mixing and dispersion;
- Unsteady aerodynamics;
- Non-equilibrium processes;
- Data-driven modelling of fluid flows:
- Nonlinear time-series analysis;
- Reduced-order methods;
- Time-series estimation.
Teaching
ENG3162/ENG3216 Aircraft Design - Group Design Project
ENG3207 Aerodynamics 2
ENG3215 High speed Aerodynamics
ENG3167 Aerodynamics
Sustainable development goals
My research interests are related to the following:
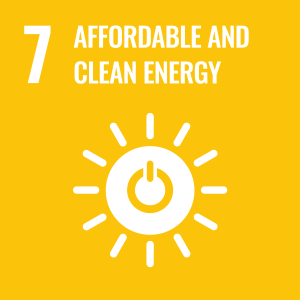
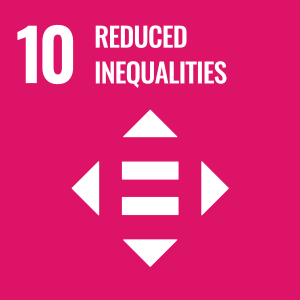
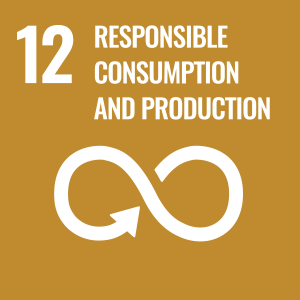
Publications
This study proposes a data-driven methodology to complement existing time-series measurement tools for turbulent flows. Specifically, a cluster-based transition network model is employed for the estimation of velocity time traces and their corresponding statistics. The method is tested on a laboratory-modelled turbulent boundary layer over a step change in surface roughness, where velocity time series are recorded for training and validation purposes via Laser Doppler Anemometry. Results show that our approach can estimate velocity and momentum flux statistics within experimental uncertainty over a rough surface through an unsupervised approach, and across the step change in roughness through a semi-supervised variant. The friction velocity across the domain is also estimated with 10% relative error compared to the measured value. The proposed methodology is interpretable robust against the main methodological parameters. A reliable data-driven framework is hence provided that can be integrated within existing laboratory setups to supplement or partially replace measurement systems, as well as to reduce wind tunnel running times.
Dataset description associated with the article “Turbulence statistics estimation across a step change in roughness via interpretable network-based modelling”, (2024), G. Iacobello, M. Placidi, S. Ding, M. Carpentieri. Files description:- ‘u_timetraces.txt’, ‘v_timetraces.txt’, and ‘w_timetraces.txt' include the instantaneous velocity values for the three velocity components u, v, and w in [m/s].- ‘XYZ_LDA_coordinatees.txt' includes the measurement coordinates (in [m[) with respect to the reference system.- ‘x_coordinatees.txt' and ‘z_coordinatees.txt' include the unique x and z coordinates (streamwise and vertical coordinates, respectively) in [m].
The dynamics of a passive scalar plume in a turbulent boundary layer is experimentally investigated via vertical turbulent transport time series. Experimental data are acquired in a rough-wall turbulent boundary layer that develops in a recirculating wind tunnel setup. Two source sizes in an elevated position are considered in order to investigate the influence of the emission conditions on the plume dynamics. The analysis is focused on the effects of the meandering motion and the relative dispersion of the plume with respect to its center of mass. First, classical statistics are investigated. We found that (in accordance with previous studies) the meandering motion is the main factor responsible for differences in the variance and intermittency, as well as the kurtosis and power spectral density, between the two source sizes. On the contrary, the mean and the skewness are slightly affected by the emission conditions. With the aim to characterize the temporal structure of the turbulent transport series, the visibility algorithm is exploited to carry out a complex network-based analysis. In particular, two network metrics-the average peak occurrence and the assortativity coefficient-are analyzed, as they are able to capture the temporal occurrence of extreme events and their relative intensity in the series. The effects of the meandering motion and the relative dispersion of the plume are discussed in view of the network metrics, revealing that a stronger meandering motion is associated with higher values of both the average peak occurrence and the assortativity coefficient. The network-based analysis advances the level of information of classical statistics by characterizing the impact of the emission conditions on the temporal structure of the signals in terms of extreme events (namely, peaks and pits) and their relative intensity. In this way, complex networks provide-through the evaluation of network metrics-an effective tool for time-series analysis of experimental data.
In this work, data of passive scalar plumes in a turbulent boundary layer are investigated. The experiments are performed in a wind tunnel where a passive scalar is injected through an L-shaped tube. Two source configurations are analysed for two different tube diameters. The passive scalar concentration is then measured at different distances from the source and wall-normal locations. By exploiting the recent advances of complex networks theory, the concentration time-series are mapped into networks, through the visibility algorithm. The resulting networks inherit the temporal features of the mapped time-series, revealing non-trivial information about the underlying transport process. This work represents an example of the great potentialities of the complex network approach for the analysis of turbulent transport and mixing.
Swimmers and flyers in nature take advantage of distributed sensor feedback to control the interaction between the surrounding fluid and their bodies, even in challenging environments such as wake flows or gusts. Inspired by this behavior, we suggest a novel data-driven method that thereby could enable effective flow control. Sparse sensor data captured on the propulsor are combined with a pre-trained algorithm to provide an estimate of the present aerodynamic state. By combining transition network theory and Bayesian statistics, a low-order model of the highly non-linear system is obtained, which is robust towards the high noise levels ubiquitous in experimental (real) pressure data. For the current study, the flow around an accelerating elliptical plate is selected as a test case. The plate is accelerated and decelerated at various (fixed) angles of attack, and the flow is captured by load and pressure measurements. The aerodynamic loads are then estimated for angle-of-attack cases that were not included in the training data, thus showing the method effectiveness under unknown (untrained) configurations.
A temporal complex network-based approach is proposed as a novel formulation to investigate turbulent mixing from a Lagrangian viewpoint. By exploiting a spatial proximity criterion, the dynamics of a set of fluid particles is geometrized into a time-varying weighted network. Specifically, a numerically solved turbulent channel flow is employed as an exemplifying case. We show that the time-varying network is able to clearly describe the particle swarm dynamics, in a parametrically robust and computationally inexpensive way. The network formalism enables us to straightforwardly identify transient and long-term flow regimes, the interplay between turbulent mixing and mean flow advection and the occurrence of proximity events among particles. Thanks to their versatility and ability to highlight significant flow features, complex networks represent a suitable tool for Lagrangian investigations of turbulent mixing. The present application of complex networks offers a powerful resource for Lagrangian analysis of turbulent flows, thus providing a further step in building bridges between turbulence research and network science.
Scale interaction is studied in wall-bounded turbulence by focusing on the frequency modulation (FM) mechanism of the large scales on small-scale velocity fluctuations. Different from the analysis of amplitude modulation (AM), FM has been less investigated owing to the difficulty of developing robust tools for broadband signals. To tackle this issue, the natural visibility graph approach is proposed in this work to map the full velocity signals into complex networks. We show that the network degree centrality is able to capture the signal structure at local scales directly from the full signal, thereby quantifying FM. Velocity signals from numerically-simulated turbulent channel flows and an experimental turbulent boundary layer are investigated at different Reynolds numbers. A correction of Taylor's hypothesis for time series is proposed to overcome the overprediction of near-wall FM obtained when local mean velocity is used as the convective velocity. Results provide network-based evidence of the large-to-small FM features for all the three velocity components in the near-wall region, with a reversal mechanism emerging far from the wall. Additionally, scaling arguments, in view of the quasi-steady quasi-homogeneous hypothesis, are discussed, and a delay time between the large and small scales is detected that is very close to the characteristic time of the near-wall cycle. Results show that the visibility graph is a parameter-free tool that turns out to be effective and robust to detect FM in different configurations of wall-bounded turbulent flows. Based on the present findings, the visibility network-based approach can represent a reliable tool to systematically investigate scale interaction mechanisms in wall-bounded turbulence.
The spatio-temporal features of the velocity field of a fully-developed turbulent channel flow are investigated through the natural visibility graph (NVG) method, which is able to fully map the intrinsic structure of the time-series into complex networks. Time-series of the three velocity components, , are analyzed at fixed grid-points of the whole three-dimensional domain. Each time-series was mapped into a network by means of the NVG algorithm, so that each network corresponds to a grid-point of the simulation. The degree centrality, the transitivity and the here proposed mean link-length were evaluated as indicators of the global visibility, inter-visibility, and mean temporal distance among nodes, respectively. The metrics were averaged along the directions of homogeneity () of the flow, so they only depend on the wall-normal coordinate, . The visibility-based networks, inheriting the flow field features, unveil key temporal properties of the turbulent time-series and their changes moving along . Although intrinsically simple to be implemented, the visibility graph-based approach offers a promising and effective support to the classical methods for accurate time-series analyses of inhomogeneous turbulent flows.
A network-based analysis of a turbulent channel flow numerically solved at Re τ = 180 is proposed as an innovative perspective for the spatial characterization of the flow field. Two spatial networks corresponding to the streamwise and wall-normal velocity components are built, where nodes represent portions of volume of the physical domain. For each network, links are active if the correlation coefficient of the corresponding velocity component between pairs of nodes is sufficiently high, thus unveiling the strongest kinematic relations. Several network measures are studied in order to explore the interrelations between nodes and their neighbors. Specifically, long-range links are localized between near-wall regions and associated with the temporal persistence of coherent patterns, namely high and low speed streaks. Furthermore, long-range links play a crucial role as intermediary for the kinematic information flow, as emerges from the analysis of indirect connections between nodes. The proposed approach provides a framework to investigate spatial structures of the turbulent dynamics, showing the full potential of complex networks. Although the network analysis is based on the two-point correlation, it is able to advance the level of information, by exploiting the texture created by active links in all directions. Based on the observed findings, the current approach can pave the way for an enhanced spatial interpretation of the turbulence dynamics.
Turbulent and vortical flows are ubiquitous and their characterization is crucial for the understanding of several natural and industrial processes. Among different techniques to study spatio-temporal flow fields, complex networks represent a recent and promising tool to deal with the large amount of data on turbulent flows and shed light on their physical mechanisms. The aim of this review is to bring together the main findings achieved so far from the application of network-based techniques to study turbulent and vortical flows. A critical discussion on the potentialities and limitations of the network approach is provided, thus giving an ordered portray of the current diversified literature. The present review can boost future network-based research on turbulent and vortical flows, promoting the establishment of complex networks as a widespread tool for turbulence analysis.
Numerical and experimental turbulence simulations are nowadays reaching the size of the so-called big data, thus requiring refined investigative tools for appropriate statistical analyses and data mining. We present a new approach based on the complex network theory, offering a powerful framework to explore complex systems with a huge number of interacting elements. Although interest in complex networks has been increasing in the past years, few recent studies have been applied to turbulence. We propose an investigation starting from a two-point correlation for the kinetic energy of a forced isotropic field numerically solved. Among all the metrics analyzed, the degree centrality is the most significant, suggesting the formation of spatial patterns which coherently move with similar vorticity over the large eddy turnover time scale. Pattern size can be quantified through a newly-introduced parameter (i.e. average physical distance) and varies from small to intermediate scales. The network analysis allows a systematic identification of different spatial regions, providing new insights into the spatial characterization of turbulent flows. Based on present findings, the application to highly inhomogeneous flows seems promising and deserves additional future investigation.
Time irreversibility is a distinctive feature of non-equilibrium phenomena such as turbulent flows, where irreversibility is mainly associated with an energy cascade process. The connection between time irreversibility and coherent motions in wall turbulence, however, has not been investigated yet. An Eulerian, multiscale analysis of time irreversibility in wall-bounded turbulence is proposed in this study, which differs from previous works relying on a Lagrangian approach and mainly focusing on homogeneous turbulence. Outcomes reveal a strong connection between irreversibility levels and coherent structures in both turbulent channel and boundary layer flows. In the near-wall region, irreversibility is directly related to the inner spectral peak originating from small-scale turbulent structures in the buffer layer. Conversely, stronger irreversibility is found in correspondence to the outer spectral peak originating from larger turbulent flow scales far from the wall. Our results represent a first effort to characterize Eulerian TI in wall-bounded turbulent flows, thus paving the way for further developments in wall-turbulence modeling and control accounting for broken temporal symmetry.
This study presents a cluster-based Bayesian methodology for state estimation under realistic conditions including noisy data from sparse sensors. The proposed approach is interpretable and, building upon previous work on transition networks, explicitly accounts for experimental noise within the data-driven framework by means of data clustering. Experimental measurements are exploited, beyond model training, to quantify the degree of uncertainty(noise) for each trained state. Such noise levels are eventually associated with probability distributions that, when combined with Bayes’ theorem, allow us to perform real-time state estimation. The proposed methodology is tested on two cases of challenging flows generated by an accelerating elliptical plate and also a delta wing experiencing gusts. Resultsspecifically indicate that the proposed approach is robust against the number of clusters, enabling state estimation with a significant order reduction, notably decreasing the computational cost while preserving estimation accuracy. Based on the present findings, the proposed data-driven approach can be employed for realistic state estimation in nonlinear systems where noise, sensor sparsity and nonlinearities represent a challenging scenario.
Realistic fluid flow problems often require that Lagrangian tracers are deployed in a sparse or very-sparse manner, such as for oceanic and atmospheric flows where large-scale motion needs characterisation. Data sparsity represents a significant issue in Lagrangian analysis, especially for data-driven methods that rely heavily on large datasets. We propose a multiscale spatial recurrence network (MSRN) methodology for characterising very-sparse Lagrangian data, which exploits individual tracks and a spatial recurrence criterion to identify the spatio-temporal complexity of tracer trajectories. The MSRN is an unsupervised modelling framework that does not require a priori parameter setting, and—through the quantification of persistent link activation at specific trajectory intervals—can reveal the presence of dominant looping scales in a variety of salient fluid flows. This new paradigm is shown to be successful for the study of Lagrangian tracers seeded in complex (realistic) flows, including unsteady and advection-dominated problems. This makes MSRNs an effective and versatile tool to characterise sensor trajectories in key problems such as environmental processes critical to understanding and mitigating climate change.
Large-scale intermittency is a widely observed phenomenon in convective surface layer turbulence that induces non-Gaussian temperature statistics, while such a signature is not observed for velocity signals. Although approaches based on probability density functions have been used so far, those are not able to explain to what extent the signals’ temporal structure impacts the statistical characteristics of the velocity and temperature fluctuations. To tackle this issue, a visibility network analysis is carried out on a field-experimental dataset from a convective atmospheric surface layer flow. Through surrogate data and network-based measures, we demonstrate that the temperature intermittency is related to strong nonlinear dependencies in the temperature signals. Conversely, a competition between linear and nonlinear effects tends to inhibit the temperature-like intermittency behaviour in streamwise and vertical velocities. Based on present findings, new research avenues are likely to be opened up in studying large-scale intermittency in convective turbulence.
Inspired by biological swimming and flying with distributed sensing, we propose a data-driven approach for load estimation that relies on complex networks. We exploit sparse, real-time pressure inputs, combined with pre-trained transition networks, to estimate aerodynamic loads in unsteady and highly separated flows. The transition networks contain the aerodynamic states of the system as nodes along with the underlying dynamics as links. A weighted average-based (WAB) strategy is proposed and tested on realistic experimental data on the flow around an accelerating elliptical plate at various angles of attack. Aerodynamic loads are then estimated for angles-of-attack cases not included in the training dataset so as to simulate the estimation process. An optimization process is also included to account for the system's temporal dynamics. Performance and limitations of the WAB approach are discussed, showing that transition networks can represent a versatile and effective data-driven tool for real-time signal estimation using sparse and noisy signals (such as surface pressure) in realistic flows.