CVSSP academics showcase 11 papers at leading computer vision conference
Researchers from our Centre for Vision, Speech and Signal Processing (CVSSP) have been invited to present an impressive 11 papers at the Conference for Computer Vision and Pattern Recognition (CVPR).
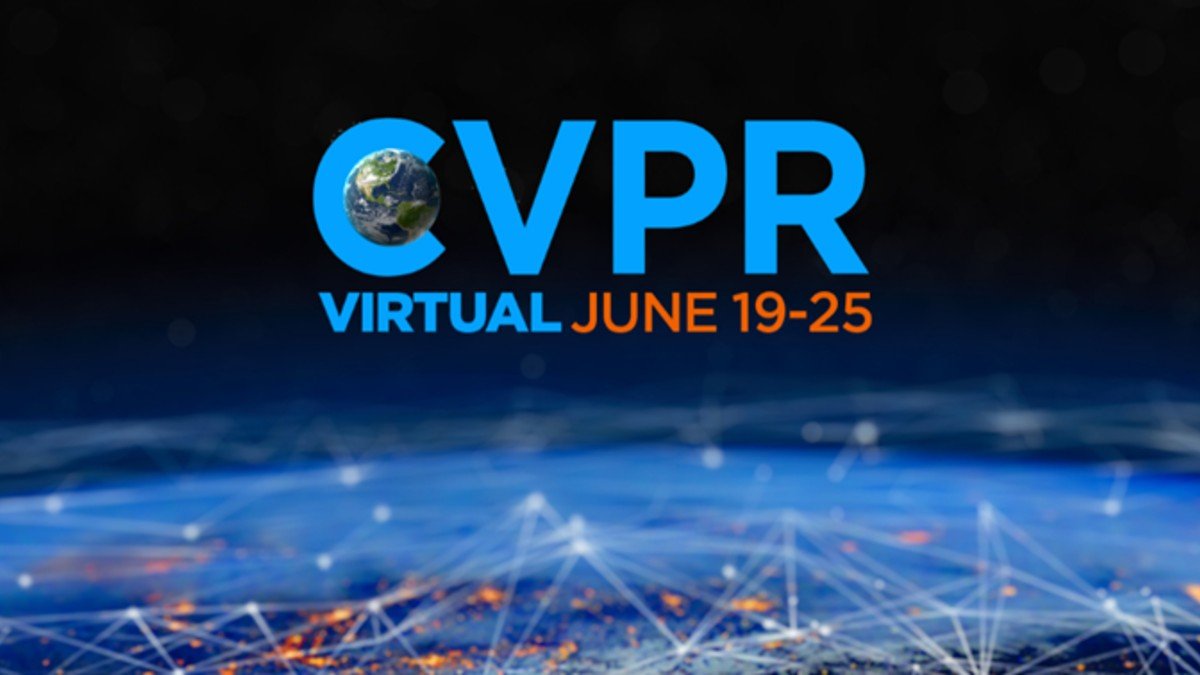
CVPR is ranked as the most important conference on computer vision in the world
CVPR runs from 19-25 June and comprises a main conference and off-shoot events. Its organising committee features names from some of the most prestigious academic institutions on the planet, with influential figures from Facebook and Google also offering behind-the-scenes support.
Global audience
Even though pandemic restrictions mean CVPR is a virtual convention in 2021, it’s still a great opportunity for CVSSP, which is ranked No.1 in the UK for computer vision research, to demonstrate its cutting-edge work to a global audience of fellow academics and figures from industry.
In terms of academic credentials, CVPR is ranked as the most important conference on computer vision in the world and it is currently the highest cited conference in the whole of computer science and engineering.
Showcasing CVSSP research
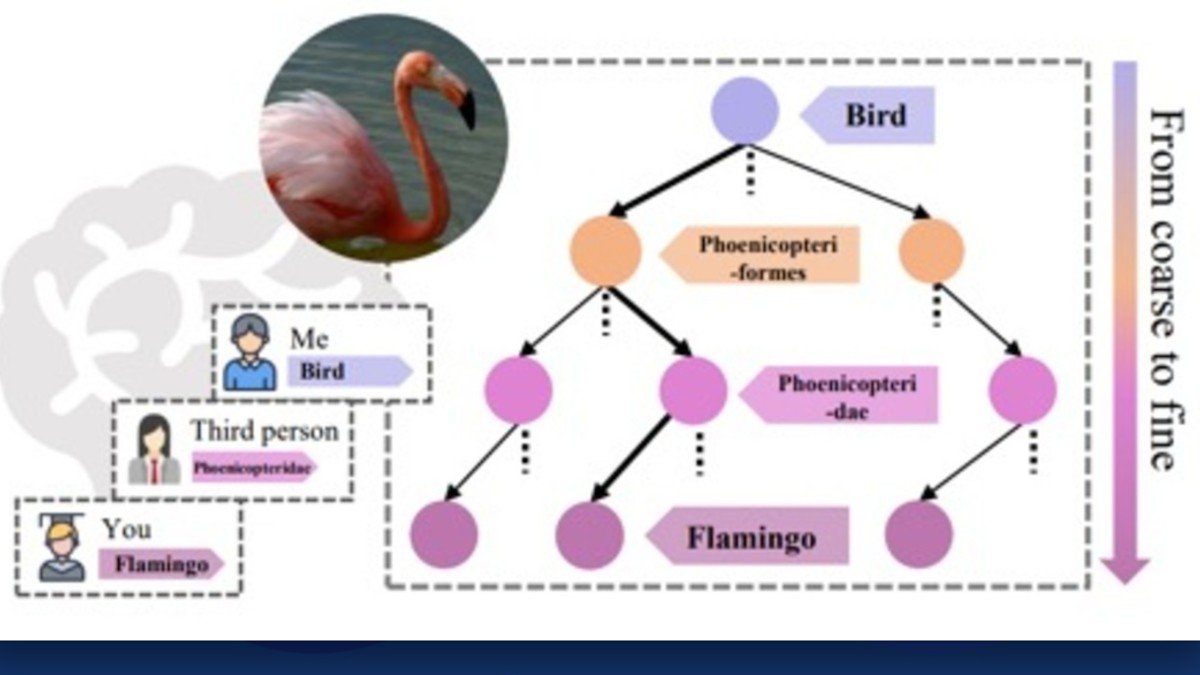
CVSSP's paper on fine-grained definitions investigates how a machine thinks about different names for the same thing
- Your “Flamingo” is My “Bird”: Fine-Grained or Not: To some of us, it’s "a labrador”. To others, it’s "a dog". But it’s the same image. So how can a machine know which term to use? In this paper, we tailor for different fine-grained definitions under divergent levels of expertise, and achieve state-of-the-art performance on fine-grained recognition by doing so
- Vectorization and Rasterization: Self-Supervised Learning for Sketch and Handwriting: This paper proposes a self-supervised method of training a machine to represent sketch and handwriting data without any prior information. It does it by combining two image recognition processes called rasterization and vectorization. This allows us to convert a dynamic drawing to a simple image and vice-versa, which makes it easier for the machine to “see” and replicate the image.
- Cloud2Curve: Generation and Vectorization of Parametric Sketches: This paper allows us to convert a raster image (an image built of blocks of pixels) into a scalable vector-graphic format (an image composed of lines with a start and an end point). We introduce a model using parametric curves. Crucially, it is able to be trained on point cloud data, thus being widely applicable to general raster images and enable the creation of more sophisticated images.
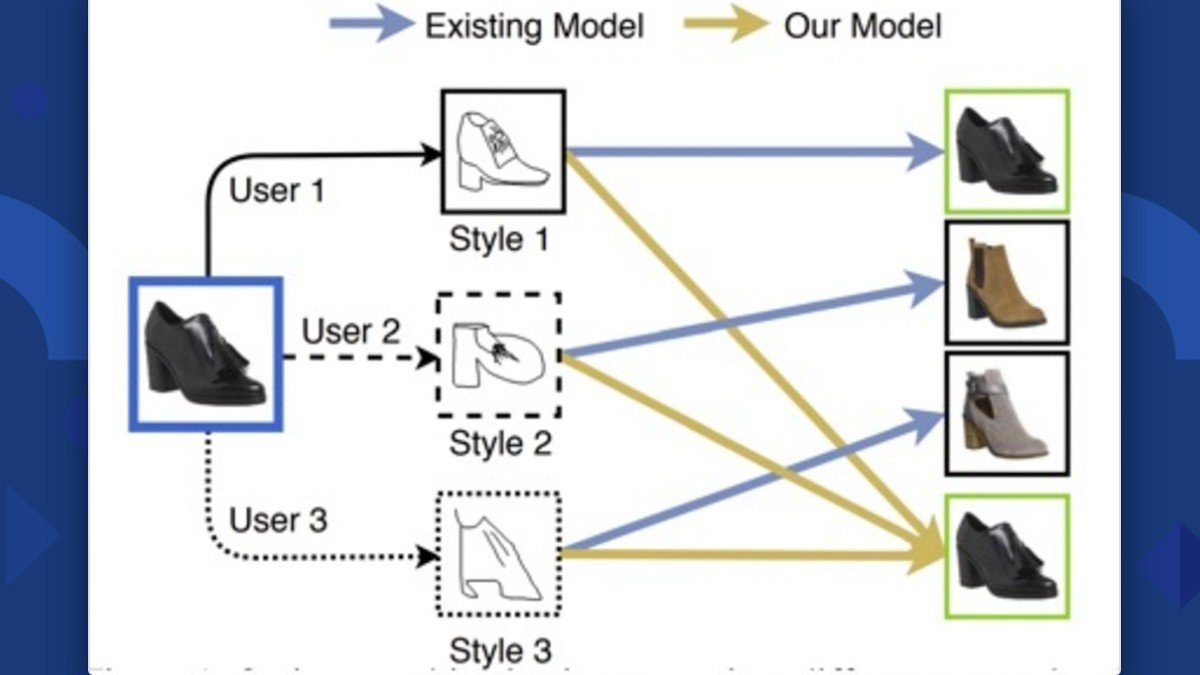
CVSSP's research helps ensure different sketching styles don't affect the accuracy of a machine's image recognition and retrieval system
- StyleMeUp: Towards Style-Agnostic Sketch-Based Image Retrieval: This paper addresses a key challenge for sketch-based image retrieval – every person sketches the same object differently. A novel style-agnostic model is proposed to explicitly account for the style diversity, so that unseen sketching styles will not affect the accuracy of image retrieval upon system deployment.
- PQA: Perceptual Question Answering: This paper rejuvenates research on perceptual organisation when applied to machine learning. For the first time, we frame a recognition problem as a perceptual question-answering (PQA) challenge. This requires an agent to infer an implied Gestalt law (a set of principles defining how humans perceive the world). The question answers gradually amass enough data to more accurately perceive the image.
- More Photos are All You Need: Semi-Supervised Learning for Fine-Grained Sketch-Based Image Retrieval: In this paper, we show how unlabelled photos alone (of which they are many) can be cultivated for training fine-grained sketch-based image retrieval systems. The answer lies with a semi-supervision design where a sequential photo-to-sketch generation model generates paired sketches for unlabelled photos.
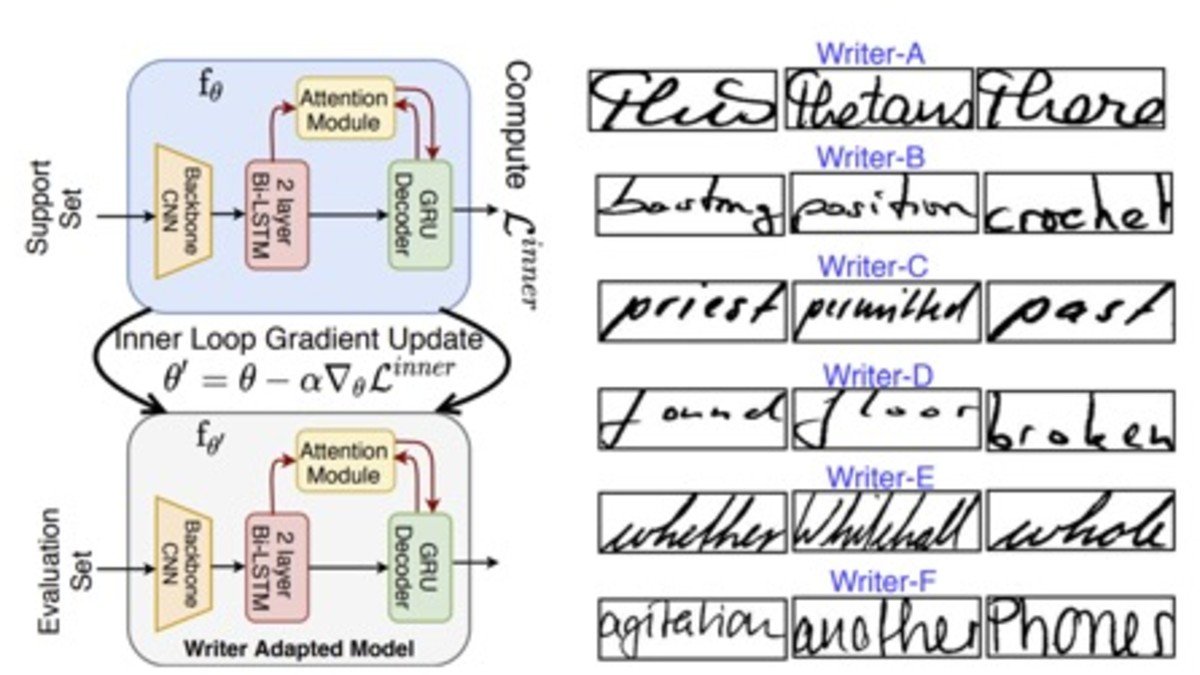
CVSSP's research means that different handwriting styles won't affect a machine's ability to recognise handwritten text
- Towards Writer-Adaptive Handwritten Text Recognition: Prior works on handwriting recognition operate with the assumption that there are a limited number of writing styles. This paper proposes a writer-adaptive recognition framework, which aims to utilise additional writer specific information at testing, resulting in a system that can work with any number of styles.
- VDSM: Unsupervised Video Disentanglement with State-Space Modeling and Deep Mixtures of Experts: Can machines independently reason about identity and pose without being explicitly told which is which? We propose a method to separate identity and pose information in video. This allows us to generate novel video sequences combining different identities and poses.
- Multi-person Implicit Reconstruction from a Single Image: This work is the first to create spatially coherent accurate 3D models of multiple interacting people in complex scenes from a single image using deep learning.
- Magic Layouts: Structural Prior for Component Detection in User Interface Designs: In this paper, we convert a sketch of a mobile app or a screenshot of an existing app into an editable user interface to help developers rapidly prototype new apps.
- Deep Image Comparator: Learning to Visualize Editorial Change: This research helps fight fake news by tracing images to their origins, showing where they have been manipulated.
The full schedule of talks and workshops where CVSSP is taking part can be found here.
International standing
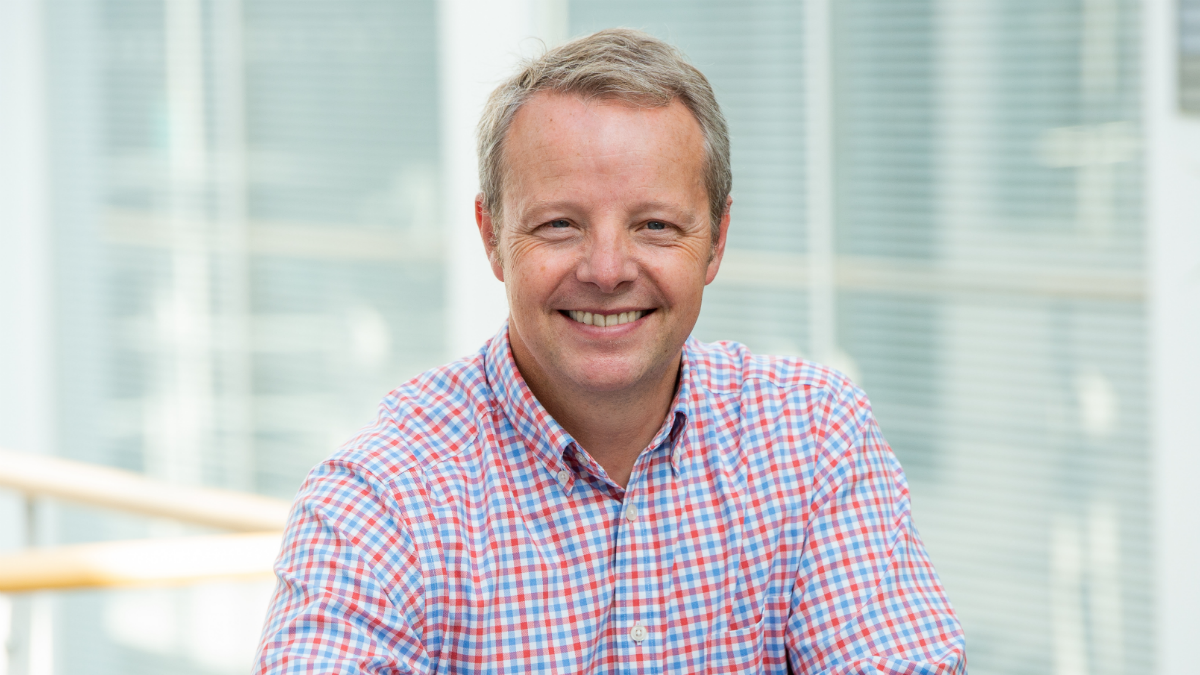
"Publication of 11 papers at CVPR underlines CVSSP's standing at the forefront of international audio-visual AI," says Professor Adrian Hilton
“Publication of 11 papers at CVPR the world’s leading conference on Computer Vision is a great achievement by CVSSP researchers and underlines our standing at the forefront of Audio-Visual AI research internationally,” comments Professor Adrian Hilton, Director of CVSSP.
Click here for the full schedule of CVSSP's presentations at CVPR.
Learn more about studying in our Centre for Vision, Speech and Signal Processing.