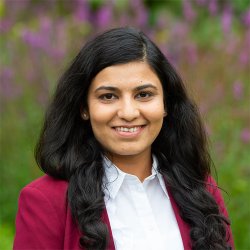
Dr Armin Mustafa
About
Biography
I am currently a Royal Academy of Engineering Research Fellow in the Centre for Vision, Speech and Signal Processing, University of Surrey working in 4D Vision for perceptive machines. The emergence of machines that interact with their environment has led to an increasing demand for automatic visual understanding of real-world scenes. My research exploits Artificial Intelligence (AI) to better understand complex scenes so that machines can efficiently model and interpret real-world for a range of socially beneficial applications including entertainment and creative industries, autonomous systems, and augmented/virtual reality. Over the past ten years, I have pioneered advances in 4D vision, NLP and Scene Understanding to understand complex real-world dynamic scenes.
I finished PhD in general dynamic scene reconstruction from multi-view videos in 2016 from the University of Surrey, supervised by Prof. Adrian Hilton, after which I became a Research Fellow at CVSSP, University of Surrey. I have previously worked at Samsung Research Institute, Bangalore, India for 3 years (2010 - 2013) in Computer Vision.
Areas of specialism
University roles and responsibilities
- Early Career Representative, CVSSP
- Surrey AI Fellow, PAI
Affiliations and memberships
Awards
2018 - Research Fellowship, The Royal Academy of Engineering , UK.
2017 - Young Researcher award, CVPR.
2016 - Doctoral Consortium grant, CVPR.
2015 - BMVA travel grant for ICCV.
2014 - Set-Squared Research to Innovator grant, Global #1 University incubator, UK.
2013 - Overseas Research Scholarship, FEPS, The University of Surrey, UK.
2010 - Cadence Silver Medal, Indian Institute of Technology, Kanpur, India.
News
In the media
ResearchResearch projects
£15 pound UKRI Prosperity Partnership with BBC - 2021 - 2026
4D Vision for Perceptive Machines5 year fellowship funded by Royal Academy of Engineering
Innovate UK project in collaboration with Figment Productions and Foundry
EU FP7 project in collaboration with Filmlight, Double Negative, AUTH, UPF and BUT
Research projects
£15 pound UKRI Prosperity Partnership with BBC - 2021 - 2026
5 year fellowship funded by Royal Academy of Engineering
Innovate UK project in collaboration with Figment Productions and Foundry
EU FP7 project in collaboration with Filmlight, Double Negative, AUTH, UPF and BUT
Supervision
Postgraduate research supervision
Current
- Sarthak Batra ”Text or Video to 3D for Scenes with Multiple Interacting People”
- Ayushi Dutta ”Multi-person Reconstruction and Rendering of Dynamic Scenes”
- Asmar Nadeem ”Audio-visual Scene Understanding from Monocular Video”
- Soon Yau on ”Automatic Storyboard Generation” from 2021 - Now
- Nikolina Kubiak on ”Computation Relighting in Video” from 2020 - Now
Completed
- Stephanie Stoll on ”Automatic Sign Language Production” from 2019 – 2022
- Mertalp Ocal on ”Self-Supervised 3D Reconstruction of Complex Dynamic Scenes” from 2018 - Now
- Akin Caliskan on ”Dynamic 3D Human Reconstruction From Video” from 2017 - 2021
Teaching
I currently contribute to teaching on the following modules:
- EEE3032 Computer Vision and Pattern Recognition
- EEEM004 MSc Projects
- EEE3017 UG Projects
Publications
Text-to-image models (T2I) such as StableDiffusion have been used to generate high quality images of people. However, due to the random nature of the generation process, the person has a different appearance e.g. pose, face, and clothing, despite using the same text prompt. The appearance inconsistency makes T2I unsuitable for pose transfer. We address this by proposing a multimodal diffusion model that accepts text, pose, and visual prompting. Our model is the first unified method to perform all person image tasks-generation, pose transfer, and mask-less edit. We also pioneer using small dimensional 3D body model parameters directly to demonstrate new capability - simultaneous pose and camera view interpolation while maintaining the person's appearance.
Leveraging machine learning techniques, in the context of object-based media production, could enable provision of personalized media experiences to diverse audiences. To fine-tune and evaluate techniques for personalization applications, as well as more broadly, datasets which bridge the gap between research and production are needed. We introduce and publicly release such a dataset, themed around a UK weather forecast and shot against a blue-screen background, of three professional actors/presenters – one male and one female (English) and one female (British Sign Language). Scenes include both production and research-oriented examples, with a range of dialogue, motions, and actions. Capture techniques consisted of a synchronized 4K resolution 16-camera array, production-typical microphones plus professional audio mix, a 16-channel microphone array with collocated Grasshopper3 camera, and a photogrammetry array. We demonstrate applications relevant to virtual production and creation of personalized media including neural radiance fields, shadow casting, action/event detection, speaker source tracking and video captioning.
In the context of Audio Visual Question Answering (AVQA) tasks, the audio and visual modalities could be learnt on three levels: 1) Spatial, 2) Temporal, and 3) Semantic. Existing AVQA methods suffer from two major shortcomings; the audiovisual (AV) information passing through the network isn't aligned on Spatial and Temporal levels; and, intermodal (audio and visual) Semantic information is often not balanced within a context; this results in poor performance. In this paper, we propose a novel end-to-end Contextual Multi-modal Alignment (CAD) network that addresses the challenges in AVQA methods by i) introducing a parameter-free stochastic Contextual block that ensures robust audio and visual alignment on the Spatial level; ii) proposing a pre-training technique for dynamic audio and visual alignment on Temporal level in a self-supervised setting , and iii) introducing a cross-attention mechanism to balance audio and visual information on Semantic level. The proposed novel CAD network improves the overall performance over the state-of-the-art methods on average by 9.4% on the MUSIC-AVQA dataset. We also demonstrate that our proposed contributions to AVQA can be added to the existing methods to improve their performance without additional complexity requirements.
Exploiting both audio and visual modalities for video classification is a challenging task, as the existing methods require large model architectures, leading to high computational complexity and resource requirements. Smaller architectures, on the other hand, struggle to achieve optimal performance. In this paper, we propose Attend Fusion, an audio-visual (AV) fusion approach that introduces a compact model architecture specifically designed to capture intricate audio-visual relationships in video data. Through extensive experiments on the challenging YouTube-8M dataset, we demonstrate that Attend-Fusion achieves an F1 score of 75.64% with only 72M parameters, which is comparable to the performance of larger baseline models such as Fully-Connected Late Fusion (75.96% F1 score, 341M parameters). Attend-Fusion achieves similar performance to the larger baseline model while reducing the model size by nearly 80%, highlighting its efficiency in terms of model complexity. Our work demonstrates that the Attend-Fusion model effectively combines audio and visual information for video classification, achieving competitive performance with significantly reduced model size. This approach opens new possibilities for deploying high-performance video understanding systems in resource-constrained environments across various applications.
In this paper we present a method for single-view illumination estimation of indoor scenes, using image-based lighting, that incorporates state-of-the-art outpainting methods. Recent advancements in illumination estimation have focused on improving the detail of the generated environment map so it can realistically light mirror reflective surfaces. These generated maps often include artefacts at the borders of the image where the panorama wraps around. In this work we make the key observation that inferring the panoramic HDR illumination of a scene from a limited field of view LDR input can be framed as an outpainting problem (whereby the original image must be expanded beyond its original borders). We incorporate two key techniques used in outpainting tasks: i) separating the generation into multiple networks (a diffuse lighting network and a high-frequency detail network) to reduce the amount to be learnt by a single network, ii) utilising an inside-out method of processing the input image to reduce the border artefacts. Further to incorporating these outpainting methods we also introduce circular padding before the network to help remove the border artefacts. Results show the proposed approach is able to relight diffuse, specular and mirror surfaces more accurately than existing methods in terms of the position of the light sources and pixelwise accuracy, whilst also reducing the artefacts produced at the borders of the panorama.
Light fields are becoming an increasingly popular method of digital content production for visual effects and virtual/augmented reality as they capture a view dependent representation enabling photo realistic rendering over a range of viewpoints. Light field video is generally captured using arrays of cameras resulting in tens to hundreds of images of a scene at each time instance. An open problem is how to efficiently represent the data preserving the view-dependent detail of the surface in such a way that is compact to store and efficient to render. In this paper we show that constructing an Eigen texture basis representation from the light field using an approximate 3D surface reconstruction as a geometric proxy provides a compact representation that maintains view-dependent realism. We demonstrate that the proposed method is able to reduce storage requirements by > 95% while maintaining the visual quality of the captured data. An efficient view-dependent rendering technique is also proposed which is performed in eigen space allowing smooth continuous viewpoint interpolation through the light field.
This paper introduces ViscoNet, a novel method that enhances text-to-image human generation models with visual prompting. Unlike existing methods that rely on lengthy text descriptions to control the image structure, ViscoNet allows users to specify the visual appearance of the target object with a reference image. ViscoNet disentangles the object's appearance from the image background and injects it into a pre-trained latent diffusion model (LDM) model via a ControlNet branch. This way, ViscoNet mitigates the style mode collapse problem and enables precise and flexible visual control. We demonstrate the effectiveness of ViscoNet on human image generation, where it can manipulate visual attributes and artistic styles with text and image prompts. We also show that ViscoNet can learn visual conditioning from small and specific object domains while preserving the generative power of the LDM backbone.
Simultaneous semantically coherent object-based long-term 4D scene flow estimation, co-segmentation and reconstruction is proposed exploiting the coherence in semantic class labels both spatially, between views at a single time instant, and temporally, between widely spaced time instants of dynamic objects with similar shape and appearance. In this paper we propose a framework for spatially and temporally coherent semantic 4D scene flow of general dynamic scenes from multiple view videos captured with a network of static or moving cameras. Semantic coherence results in improved 4D scene flow estimation, segmentation and reconstruction for complex dynamic scenes. Semantic tracklets are introduced to robustly initialize the scene flow in the joint estimation and enforce temporal coherence in 4D flow, semantic labelling and reconstruction between widely spaced instances of dynamic objects. Tracklets of dynamic objects enable unsupervised learning of long-term flow, appearance and shape priors that are exploited in semantically coherent 4D scene flow estimation, co-segmentation and reconstruction. Comprehensive performance evaluation against state-of-the-art techniques on challenging indoor and outdoor sequences with hand-held moving cameras shows improved accuracy in 4D scene flow, segmentation, temporally coherent semantic labelling, and reconstruction of dynamic scenes.
In this paper we propose a framework for spatially and temporally coherent semantic co-segmentation and reconstruction of complex dynamic scenes from multiple static or moving cameras. Semantic co-segmentation exploits the coherence in semantic class labels both spatially, between views at a single time instant, and temporally, between widely spaced time instants of dynamic objects with similar shape and appearance. We demonstrate that semantic coherence results in improved segmentation and reconstruction for complex scenes. A joint formulation is proposed for semantically coherent object-based co-segmentation and reconstruction of scenes by enforcing consistent semantic labelling between views and over time. Semantic tracklets are introduced to enforce temporal coherence in semantic labelling and reconstruction between widely spaced instances of dynamic objects. Tracklets of dynamic objects enable unsupervised learning of appearance and shape priors that are exploited in joint segmentation and reconstruction. Evaluation on challenging indoor and outdoor sequences with hand-held moving cameras shows improved accuracy in segmentation, temporally coherent semantic labelling and 3D reconstruction of dynamic scenes.
Text-to-image models (T2I) such as StableDiffusion have been used to generate high quality images of people. However , due to the random nature of the generation process, the person has a different appearance e.g. pose, face, and clothing, despite using the same text prompt. The appearance inconsistency makes T2I unsuitable for pose transfer. We address this by proposing a multimodal diffusion model that accepts text, pose, and visual prompting. Our model is the first unified method to perform all person image tasks-generation, pose transfer, and mask-less edit. We also pioneer using small dimensional 3D body model parameters directly to demonstrate new capability-simultaneous pose and camera view interpolation while maintaining the per-son's appearance.
Generating grammatically and semantically correct captions in video captioning is a challenging task.. The captions generated from the existing methods are either word-by-word that do not align with grammatical structure or miss key information from the input videos. To address these issues, we introduce a novel global-local fusion network, with a Global-Local Fusion Block (GLFB) that encodes and fuses features from different parts of speech (POS) components with visual-spatial features. We use novel combinations of different POS components - 'determinant + subject', 'auxiliary verb', 'verb', and 'determinant + object' for supervision of the POS blocks - Det + Subject, Aux Verb, Verb, and Det + Object respectively. The novel global-local fusion network together with POS blocks helps align the visual features with language description to generate grammatically and semantically correct captions. Extensive qualitative and quantitative experiments on benchmark MSVD and MSRVTT datasets demonstrate that the proposed approach generates more grammatically and semantically correct captions compared to the existing methods, achieving the new state-of-the-art. Ablations on the POS blocks and the GLFB demonstrate the impact of the contributions on the proposed method.
This paper presents an approach for reconstruction of 4D temporally coherent models of complex dynamic scenes. No prior knowledge is required of scene structure or camera calibration allowing reconstruction from multiple moving cameras. Sparse-to-dense temporal correspondence is integrated with joint multi-view segmentation and reconstruction to obtain a complete 4D representation of static and dynamic objects. Temporal coherence is exploited to overcome visual ambiguities resulting in improved reconstruction of complex scenes. Robust joint segmentation and reconstruction of dynamic objects is achieved by introducing a geodesic star convexity constraint. Comparative evaluation is performed on a variety of unstructured indoor and outdoor dynamic scenes with hand-held cameras and multiple people. This demonstrates reconstruction of complete temporally coherent 4D scene models with improved nonrigid object segmentation and shape reconstruction.
Transformers have recently been shown to generate high quality images from text input. However, the existing method of pose conditioning using skeleton image tokens is computationally inefficient and generate low quality images. Therefore we propose a new method; Keypoint Pose Encoding (KPE); KPE is 10× more memory efficient and over 73% faster at generating high quality images from text input conditioned on the pose. The pose constraint improves the image quality and reduces errors on body extremities such as arms and legs. The additional benefits include invariance to changes in the target image domain and image resolution, making it easily scalable to higher resolution images. We demonstrate the versatility of KPE by generating photorealistic multiperson images derived from the DeepFashion dataset [1].We also introduce a evaluation method People Count Error (PCE) that is effective in detecting error in generated human images. (a) (b) Figure 1: (a) Our pose constrained text-to-image model supports partial and full pose view, multiple people, different genders, at different scales. (b) The Architectural diagram of our pose-guided text-to-image generation model. The text, pose keypoints and image are encoded into tokens and go into an transformer. *The target image encoding section is required only for training and is not needed in inference.
With the increasing global popularity of self-driving cars, there is an immediate need for challenging real-world datasets for benchmarking and training various computer vision tasks such as 3D object detection. Existing datasets either represent simple scenarios or provide only day-time data. In this paper, we introduce a new challenging A*3D dataset which consists of RGB images and LiDAR data with a significant diversity of scene, time, and weather. The dataset consists of high-density images (≈ 10 times more than the pioneering KITTI dataset), heavy occlusions, a large number of nighttime frames (≈ 3 times the nuScenes dataset), addressing the gaps in the existing datasets to push the boundaries of tasks in autonomous driving research to more challenging highly diverse environments. The dataset contains 39K frames, 7 classes, and 230K 3D object annotations. An extensive 3D object detection benchmark evaluation on the A*3D dataset for various attributes such as high density, day-time/night-time, gives interesting insights into the advantages and limitations of training and testing 3D object detection in real-world setting.
We introduce the first approach to solve the challenging problem of unsupervised 4D visual scene understanding for complex dynamic scenes with multiple interacting people from multi-view video. Our approach simultaneously estimates a detailed model that includes a per-pixel semantically and temporally coherent reconstruction, together with instance-level segmentation exploiting photo-consistency, semantic and motion information. We further leverage recent advances in 3D pose estimation to constrain the joint semantic instance segmentation and 4D temporally coherent reconstruction. This enables per person semantic instance segmentation of multiple interacting people in complex dynamic scenes. Extensive evaluation of the joint visual scene understanding framework against state-of-the-art methods on challenging indoor and outdoor sequences demonstrates a significant (≈ 40%) improvement in semantic segmentation, reconstruction and scene flow accuracy.
A common problem in wide-baseline matching is the sparse and non-uniform distribution of correspondences when using conventional detectors such as SIFT, SURF, FAST, A-KAZE and MSER. In this paper we introduce a novel segmentation based feature detector (SFD) that produces an increased number of accurate features for wide-baseline matching. A multi-scale SFD is proposed using bilateral image decomposition to produce a large number of scale-invariant features for wide-baseline reconstruction. All input images are over-segmented into regions using any existing segmentation technique like Watershed, Mean-shift, and SLIC. Feature points are then detected at the intersection of the boundaries of three or more regions. The detected feature points are local maxima of the image function. The key advantage of feature detection based on segmentation is that it does not require global threshold setting and can therefore detect features throughout the image. A comprehensive evaluation demonstrates that SFD gives an increased number of features which are accurately localised and matched between wide-baseline camera views; the number of features for a given matching error increases by a factor of 3-5 compared to SIFT; feature detection and matching performance is maintained with increasing baseline between views; multi-scale SFD improves matching performance at varying scales. Application of SFD to sparse multi-view wide-baseline reconstruction demonstrates a factor of ten increase in the number of reconstructed points with improved scene coverage compared to SIFT/MSER/A-KAZE. Evaluation against ground-truth shows that SFD produces an increased number of wide-baseline matches with reduced error.
In this paper we present S3R-Net, the Self-Supervised Shadow Removal Network. The two-branch WGAN model achieves self-supervision relying on the unify-and-adaptphenomenon - it unifies the style of the output data and infers its characteristics from a database of unaligned shadow-free reference images. This approach stands in contrast to the large body of supervised frameworks. S3R-Net also differentiates itself from the few existing self-supervised models operating in a cycle-consistent manner, as it is a non-cyclic, unidirectional solution. The proposed framework achieves comparable numerical scores to recent selfsupervised shadow removal models while exhibiting superior qualitative performance and keeping the computational cost low. Code & pretrained models are available at https://github.com/n-kubiak/S3R-Net
We present SILT, a Self-supervised Implicit Lighting Transfer method. Unlike previous research on scene relighting, we do not seek to apply arbitrary new lighting configurations to a given scene. Instead, we wish to transfer the lighting style from a database of other scenes, to provide a uniform lighting style regardless of the input. The solution operates as a two-branch network that first aims to map input images of any arbitrary lighting style to a unified domain, with extra guidance achieved through implicit image decomposition. We then remap this unified input domain using a discriminator that is presented with the generated outputs and the style reference, i.e. images of the desired illumination conditions. Our method is shown to outperform supervised relighting solutions across two different datasets without requiring lighting supervision.
Leveraging machine learning techniques, in the context of object-based media production, could enable provision of personalized media experiences to diverse audiences. To fine-tune and evaluate techniques for personalization applications, as well as more broadly, datasets which bridge the gap between research and production are needed. We introduce and publicly release such a dataset, themed around a UK weather forecast and shot against a blue-screen background, of three professional actors/presenters – one male and one female (English) and one female (British Sign Language). Scenes include both production and research-oriented examples, with a range of dialogue, motions, and actions. Capture techniques consisted of a synchronized 4K resolution 16-camera array, production-typical microphones plus professional audio mix, a 16-channel microphone array with collocated Grasshopper3 camera, and a photogrammetry array. We demonstrate applications relevant to virtual production and creation of personalized media including neural radiance fields, shadow casting, action/event detection, speaker source tracking and video captioning.
We present PAT, a transformer-based network that learns complex temporal co-occurrence action dependencies in a video by exploiting multi-scale temporal features. In existing methods, the self-attention mechanism in transformers loses the temporal positional information, which is essential for robust action detection. To address this issue, we (i) embed relative positional encoding in the self-attention mechanism and (ii) exploit multi-scale temporal relationships by designing a novel non-hierarchical network, in contrast to the recent transformer-based approaches that use a hierarchical structure. We argue that joining the self-attention mechanism with multiple sub-sampling processes in the hierarchical approaches results in increased loss of positional information. We evaluate the performance of our proposed approach on two challenging dense multi-label benchmark datasets, and show that PAT improves the current state-of-the-art result by 1.1% and 0.6% mAP on the Charades and MultiTHUMOS datasets, respectively, thereby achieving the new state-of-the-art mAP at 26.5% and 44.6%, respectively. We also perform extensive ablation studies to examine the impact of the different components of our proposed network.
We present a novel method to learn temporally consistent 3D reconstruction of clothed people from a monocular video. Recent methods for 3D human reconstruction from monocular video using volumetric, implicit or parametric human shape models, produce per frame reconstructions giving temporally inconsistent output and limited performance when applied to video. In this paper, we introduce an approach to learn temporally consistent features for textured reconstruction of clothed 3D human sequences from monocular video by proposing two advances: a novel temporal consistency loss function; and hybrid representation learning for implicit 3D reconstruction from 2D images and coarse 3D geometry. The proposed advances improve the temporal consistency and accuracy of both the 3D reconstruction and texture prediction from a monocular video. Comprehensive comparative performance evaluation on images of people demonstrates that the proposed method significantly outperforms the state-of-the-art learning-based single image 3D human shape estimation approaches achieving significant improvement of reconstruction accuracy, completeness, quality and temporal consistency.
Existing shadow detection models struggle to differentiate dark image areas from shadows. In this paper, we tackle this issue by verifying that all detected shadows are real, i.e. they have paired shadow casters. We perform this step in a physically-accurate manner by dif-ferentiably re-rendering the scene and observing the changes stemming from carving out estimated shadow casters. Thanks to this approach, the RenDetNet proposed in this paper is the first learning-based shadow detection model whose supervisory signals can be computed in a self-supervised manner. The developed system compares favourably against recent models trained on our data. As part of this publication, we release our code on github.
We present PAT, a transformer-based network that learns complex temporal co-occurrence action dependencies in a video by exploiting multi-scale temporal features. In existing methods, the self-attention mechanism in transformers loses the temporal positional information, which is essential for robust action detection. To address this issue, we (i) embed relative positional encoding in the self-attention mechanism and (ii) exploit multi-scale temporal relationships by designing a novel non hierarchical network, in contrast to the recent transformer-based approaches that use a hierarchical structure. We argue that joining the self-attention mechanism with multiple sub-sampling processes in the hierarchical approaches results in increased loss of positional information. We evaluate the performance of our proposed approach on two challenging dense multi-label benchmark datasets, and show that PAT improves the current state-of-the-art result by 1.1% and 0.6% mAP on the Charades and MultiTHUMOS datasets, respectively, thereby achieving the new state-of-the-art mAP at 26.5% and 44.6%, respectively. We also perform extensive ablation studies to examine the impact of the different components of our proposed network.
A common problem in wide-baseline stereo is the sparse and non-uniform distribution of correspondences when using conventional detectors such as SIFT, SURF, FAST and MSER. In this paper we introduce a novel segmentation based feature detector SFD that produces an increased number of ‘good’ features for accurate wide-baseline reconstruction. Each image is segmented into regions by over-segmentation and feature points are detected at the intersection of the boundaries for three or more regions. Segmentation-based feature detection locates features at local maxima giving a relatively large number of feature points which are consistently detected across wide-baseline views and accurately localised. A comprehensive comparative performance evaluation with previous feature detection approaches demonstrates that: SFD produces a large number of features with increased scene coverage; detected features are consistent across wide-baseline views for images of a variety of indoor and outdoor scenes; and the number of wide-baseline matches is increased by an order of magnitude compared to alternative detector-descriptor combinations. Sparse scene reconstruction from multiple wide-baseline stereo views using the SFD feature detector demonstrates at least a factor six increase in the number of reconstructed points with reduced error distribution compared to SIFT when evaluated against ground-truth and similar computational cost to SURF/FAST.
We introduce the first method to automatically generate 3D mesh sequences from text, inspired by the challenging problem of Sign Language Production (SLP). The approach only requires simple 2D annotations for training, which can be automatically extracted from video. Rather than incorporating high-definition or motion capture data, we propose back-translation as a powerful paradigm for supervision: By first addressing the arguably simpler problem of translating 2D pose sequences to text, we can leverage this to drive a transformer-based architecture to translate text to 2D poses. These are then used to drive a 3D mesh generator. Our mesh generator Pose2Mesh uses temporal information, to enforce temporal coherence and significantly reduce processing time. The approach is evaluated by generating 2D pose, and 3D mesh sequences in DGS (German Sign Language) from German language sentences. An extensive analysis of the approach and its sub-networks is conducted, reporting BLEU and ROUGE scores, as well as Mean 2D Joint Distance. Our proposed Text2Pose model outperforms the current state-of-the-art in SLP, and we establish the first benchmark for the complex task of text-to-3D-mesh-sequence generation with our Text2Mesh model.
Light field video for content production is gaining both research and commercial interest as it has the potential to push the level of immersion for augmented and virtual reality to a close-to-reality experience. Light fields densely sample the viewing space of an object or scene using hundreds or even thousands of images with small displacements in between. However, a lack of standardised formats for compression, storage and transmission, along with the lack of tools to enable editing of light field data currently make it impractical for use in real-world content production. In this chapter we address two fundamental problems with light field data, namely representation and compression. Firstly we propose a method to obtain a 4D temporally coherent representation from the input light field video. This is an essential problem to solve that will enable efficient compression editing. Secondly, we present a method for compression of light field data based on the eigen texture method that provides a compact representation and enables efficient view-dependent rendering at interactive frame rates. These approaches achieve an order of magnitude compression and temporally consistent representation that are important steps towards practical toolsets for light field video content production.
Existing techniques for dynamic scene re- construction from multiple wide-baseline cameras pri- marily focus on reconstruction in controlled environ- ments, with fixed calibrated cameras and strong prior constraints. This paper introduces a general approach to obtain a 4D representation of complex dynamic scenes from multi-view wide-baseline static or moving cam- eras without prior knowledge of the scene structure, ap- pearance, or illumination. Contributions of the work are: An automatic method for initial coarse reconstruc- tion to initialize joint estimation; Sparse-to-dense tem- poral correspondence integrated with joint multi-view segmentation and reconstruction to introduce tempo- ral coherence; and a general robust approach for joint segmentation refinement and dense reconstruction of dynamic scenes by introducing shape constraint. Com- parison with state-of-the-art approaches on a variety of complex indoor and outdoor scenes, demonstrates im- proved accuracy in both multi-view segmentation and dense reconstruction. This paper demonstrates unsuper- vised reconstruction of complete temporally coherent 4D scene models with improved non-rigid object seg- mentation and shape reconstruction and its application to various applications such as free-view rendering and virtual reality.
This paper presents a method for dense 4D temporal alignment of partial reconstructions of non-rigid surfaces observed from single or multiple moving cameras of complex scenes. 4D Match Trees are introduced for robust global alignment of non-rigid shape based on the similarity between images across sequences and views. Wide-timeframe sparse correspondence between arbitrary pairs of images is established using a segmentation-based feature detector (SFD) which is demonstrated to give improved matching of non-rigid shape. Sparse SFD correspondence allows the similarity between any pair of image frames to be estimated for moving cameras and multiple views. This enables the 4D Match Tree to be constructed which minimises the observed change in non-rigid shape for global alignment across all images. Dense 4D temporal correspondence across all frames is then estimated by traversing the 4D Match tree using optical flow initialised from the sparse feature matches. The approach is evaluated on single and multiple view images sequences for alignment of partial surface reconstructions of dynamic objects in complex indoor and outdoor scenes to obtain a temporally consistent 4D representation. Comparison to previous 2D and 3D scene flow demonstrates that 4D Match Trees achieve reduced errors due to drift and improved robustness to large non-rigid deformations.
In the context of Audio Visual Question Answering (AVQA) tasks, the audio visual modalities could be learnt on three levels: 1) Spatial, 2) Temporal, and 3) Semantic. Existing AVQA methods suffer from two major shortcomings; the audio-visual (AV) information passing through the network isn't aligned on Spatial and Temporal levels; and, inter-modal (audio and visual) Semantic information is often not balanced within a context; this results in poor performance. In this paper, we propose a novel end-to-end Contextual Multi-modal Alignment (CAD) network that addresses the challenges in AVQA methods by i) introducing a parameter-free stochastic Contextual block that ensures robust audio and visual alignment on the Spatial level; ii) proposing a pre-training technique for dynamic audio and visual alignment on Temporal level in a self-supervised setting, and iii) introducing a cross-attention mechanism to balance audio and visual information on Semantic level. The proposed novel CAD network improves the overall performance over the state-of-the-art methods on average by 9.4% on the MUSIC-AVQA dataset. We also demonstrate that our proposed contributions to AVQA can be added to the existing methods to improve their performance without additional complexity requirements.
We present a generalised self-supervised learning approach for monocular estimation of the real depth across scenes with diverse depth ranges from 1--100s of meters. Existing supervised methods for monocular depth estimation require accurate depth measurements for training. This limitation has led to the introduction of self-supervised methods that are trained on stereo image pairs with a fixed camera baseline to estimate disparity which is transformed to depth given known calibration. Self-supervised approaches have demonstrated impressive results but do not generalise to scenes with different depth ranges or camera baselines. In this paper, we introduce RealMonoDepth a self-supervised monocular depth estimation approach which learns to estimate the real scene depth for a diverse range of indoor and outdoor scenes. A novel loss function with respect to the true scene depth based on relative depth scaling and warping is proposed. This allows self-supervised training of a single network with multiple data sets for scenes with diverse depth ranges from both stereo pair and in the wild moving camera data sets. A comprehensive performance evaluation across five benchmark data sets demonstrates that RealMonoDepth provides a single trained network which generalises depth estimation across indoor and outdoor scenes, consistently outperforming previous self-supervised approaches.
Light-field video has recently been used in virtual and augmented reality applications to increase realism and immersion. However, existing light-field methods are generally limited to static scenes due to the requirement to acquire a dense scene representation. The large amount of data and the absence of methods to infer temporal coherence pose major challenges in storage, compression and editing compared to conventional video. In this paper, we propose the first method to extract a spatio-temporally coherent light-field video representation. A novel method to obtain Epipolar Plane Images (EPIs) from a spare lightfield camera array is proposed. EPIs are used to constrain scene flow estimation to obtain 4D temporally coherent representations of dynamic light-fields. Temporal coherence is achieved on a variety of light-field datasets. Evaluation of the proposed light-field scene flow against existing multiview dense correspondence approaches demonstrates a significant improvement in accuracy of temporal coherence.
Existing person image generative models can do either image generation or pose transfer but not both. We propose a unified diffusion model, UPGPT to provide a universal solution to perform all the person image tasks - generative, pose transfer, and editing. With fine-grained multimodality and disentanglement capabilities, our approach offers fine-grained control over the generation and the editing process of images using a combination of pose, text, and image, all without needing a semantic segmentation mask which can be challenging to obtain or edit. We also pioneer the parameterized body SMPL model in pose-guided person image generation to demonstrate new capability - simultaneous pose and camera view interpolation while maintaining a person's appearance. Results on the benchmark DeepFashion dataset show that UPGPT is the new state-of-the-art while simultaneously pioneering new capabilities of edit and pose transfer in human image generation.
Generating grammatically and semantically correct captions in video captioning is a challenging task. The captions generated from the existing methods are either word-by-word that do not align with grammatical structure or miss key information from the input videos. To address these issues, we introduce a novel global-local fusion network, with a Global-Local Fusion Block (GLFB) that encodes and fuses features from different parts of speech (POS) components with visual-spatial features. We use novel combinations of different POS components - 'determinant + subject', 'auxiliary verb', 'verb', and 'determinant + object' for supervision of the POS blocks - Det + Subject, Aux Verb, Verb, and Det + Object respectively. The novel global-local fusion network together with POS blocks helps align the visual features with language description to generate grammatically and semantically correct captions. Extensive qualitative and quantitative experiments on benchmark MSVD and MSRVTT datasets demonstrate that the proposed approach generates more grammatically and semantically correct captions compared to the existing methods, achieving the new state-of-the-art. Ablations on the POS blocks and the GLFB demonstrate the impact of the contributions on the proposed method.
We present a new end-to-end learning framework to obtain detailed and spatially coherent reconstructions of multiple people from a single image. Existing multi-person methods suffer from two main drawbacks: they are often model-based and therefore cannot capture accurate 3D models of people with loose clothing and hair; or they require manual intervention to resolve occlusions or interactions. Our method addresses both limitations by introducing the first end-to-end learning approach to perform model-free implicit reconstruction for realistic 3D capture of multiple clothed people in arbitrary poses (with occlusions) from a single image. Our network simultaneously estimates the 3D geometry of each person and their 6DOF spatial locations, to obtain a coherent multi-human reconstruction. In addition, we introduce a new synthetic dataset that depicts images with a varying number of inter-occluded humans and a variety of clothing and hair styles. We demonstrate robust, high-resolution reconstructions on images of multiple humans with complex occlusions, loose clothing and a large variety of poses and scenes. Our quantitative evaluation on both synthetic and real world datasets demonstrates state-of-the-art performance with significant improvements in the accuracy and completeness of the reconstructions over competing approaches.
This paper introduces a general approach to dynamic scene reconstruction from multiple moving cameras without prior knowledge or limiting constraints on the scene structure, appearance, or illumination. Existing techniques for dynamic scene reconstruction from multiple wide-baseline camera views primarily focus on accurate reconstruction in controlled environments, where the cameras are fixed and calibrated and background is known. These approaches are not robust for general dynamic scenes captured with sparse moving cameras. Previous approaches for outdoor dynamic scene reconstruction assume prior knowledge of the static background appearance and structure. The primary contributions of this paper are twofold: an automatic method for initial coarse dynamic scene segmentation and reconstruction without prior knowledge of background appearance or structure; and a general robust approach for joint segmentation refinement and dense reconstruction of dynamic scenes from multiple wide-baseline static or moving cameras. Evaluation is performed on a variety of indoor and outdoor scenes with cluttered backgrounds and multiple dynamic non-rigid objects such as people. Comparison with state-of-the-art approaches demonstrates improved accuracy in both multiple view segmentation and dense reconstruction. The proposed approach also eliminates the requirement for prior knowledge of scene structure and appearance.
We present a novel method to learn temporally consistent 3D reconstruction of clothed people from a monocular video. Recent methods for 3D human reconstruction from monocular video using volumetric, implicit or parametric human shape models, produce per frame reconstructions giving temporally inconsistent output and limited performance when applied to video. In this paper we introduce an approach to learn temporally consistent features for textured reconstruction of clothed 3D human sequences from monocular video by proposing two advances: a novel temporal consistency loss function; and hybrid representation learning for implicit 3D reconstruction from 2D images and coarse 3D geometry. The proposed advances improve the temporal consistency and accuracy of both the 3D reconstruction and texture prediction from a monocular video. Comprehensive comparative performance evaluation on images of people demonstrates that the proposed method significantly outperforms the state-of-the-art learning-based single image 3D human shape estimation approaches achieving significant improvement of reconstruction accuracy, completeness, quality and temporal consistency.
We present a novel method to improve the accuracy of the 3D reconstruction of clothed human shape from a single image. Recent work has introduced volumetric, implicit and model-based shape learning frameworks for reconstruction of objects and people from one or more images. However, the accuracy and completeness for reconstruction of clothed people is limited due to the large variation in shape resulting from clothing, hair, body size, pose and camera viewpoint. This paper introduces two advances to overcome this limitation: firstly a new synthetic dataset of realistic clothed people, 3DVH; and secondly, a novel multiple-view loss function for training of monocular volumetric shape estimation, which is demonstrated to significantly improve generalisation and reconstruction accuracy. The 3DVH dataset of realistic clothed 3D human models rendered with diverse natural backgrounds is demonstrated to allows transfer to reconstruction from real images of people. Comprehensive comparative performance evaluation on both synthetic and real images of people demonstrates that the proposed method significantly outperforms the previous state-of-the-art learning-based single image 3D human shape estimation approaches achieving significant improvement of reconstruction accuracy, completeness, and quality. An ablation study shows that this is due to both the proposed multiple-view training and the new 3DVH dataset. The code and the dataset can be found at the project website: https://akincaliskan3d.github.io/MV3DH/.
The rise of autonomous machines in our day-to-day lives has led to an increasing demand for machine perception of real-world to be more robust, accurate and human-like. The research in visual scene un- derstanding over the past two decades has focused on machine perception in controlled environments such as indoor, static and rigid objects. There is a gap in literature for machine perception in general complex scenes (outdoor with multiple interacting people). The proposed research ad- dresses the limitations of existing methods by proposing an unsupervised framework to simultaneously model, semantically segment and estimate motion for general dynamic scenes captured from multiple view videos with a network of static or moving cameras. In this talk I will explain the proposed joint framework to understand general dynamic scenes for ma- chine perception; give a comprehensive performance evaluation against state-of-the-art techniques on challenging indoor and outdoor sequences; and demonstrate applications such as virtual, augmented, mixed reality (VR/AR/MR) and broadcast production (Free-view point video - FVV).
We introduce the first approach to solve the challenging problem of automatic 4D visual scene understanding for complex dynamic scenes with multiple interacting people from multi-view video. Our approach simultaneously estimates a detailed model that includes a per-pixel semantically and temporally coherent reconstruction, together with instance-level segmentation exploiting photo-consistency, semantic and motion information. We further leverage recent advances in 3D pose estimation to constrain the joint semantic instance segmentation and 4D temporally coherent reconstruction. This enables per person semantic instance segmentation of multiple interacting people in complex dynamic scenes. Extensive evaluation of the joint visual scene understanding framework against state-of-the-art methods on challenging indoor and outdoor sequences demonstrates a significant (≈40%) improvement in semantic segmentation, reconstruction and scene flow accuracy. In addition to the evaluation on several indoor and outdoor scenes, the proposed joint 4D scene understanding framework is applied to challenging outdoor sports scenes in the wild captured with manually operated wide-baseline broadcast cameras.