
Stochastic modelling and uncertainty quantification
We are developing prediction systems for weather and climate that consider unknown processes and errors in the mathematical models and measurements, to estimate uncertainties in forecasts.
Research lead
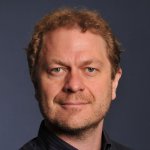
Dr Werner Bauer
Lecturer
Using stochastic models for predictions
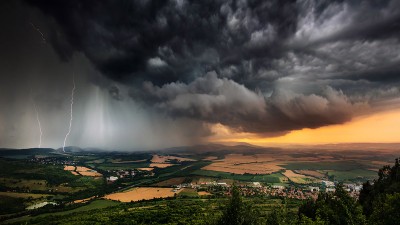
When using numerical models for predictions of weather and climate we face various problems such as errors in the mathematical formulations of the phenomena of interest, unresolved scales, and limited computational resources. This might lead to significant errors in the prediction because, for example, unresolved physical processes might have a significant influence on the large scale atmospheric flow. Traditionally, parameterisations have been used in deterministic weather and climate models to represent subgrid processes to increase the accuracy of simulations.
To capture these different sources of errors, we use stochastic models for predictions because they provide promising alternatives to classical, deterministic prediction systems. These models describe the uncertainty in processes and their effects on the large scale flow by random variables.
We apply this approach to predict, rather than one deterministic solution (which might differ significantly from the “truth”), an ensemble of solutions. The forecasting error is then expressed through the spread of this ensemble. This allows us to provide weather and climate forecasts and an estimation of their uncertainty.