11am - 12 noon
Friday 31 January 2025
Audio Source Separation and Creation Empowered by Natural Language Intelligence
PhD Viva Open Presentation - Xubo Liu
Hybrid event - All Welcome!
Free
University of Surrey
Guildford
Surrey
GU2 7XH
Audio Source Separation and Creation Empowered by Natural Language Intelligence
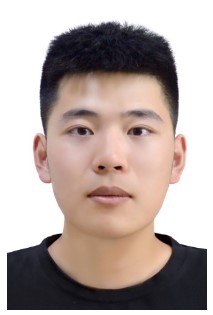
Abstract:
Audio content creation and manipulation are essential aspects of modern digital media, influencing sectors such as entertainment, education, and virtual reality. The growing demand for efficient and flexible audio production tools highlights the need for methods that utilize intuitive interfaces like natural language. This thesis explores new approaches to advance audio source separation and audio content creation by leveraging natural language intelligence.
First, we introduce the task of Language-Queried Audio Source Separation (LASS), which aims to separate target audio sources from mixtures based on natural language descriptions (e.g.,``a man tells a joke followed by people laughing"). We propose LASS-Net, an end-to-end neural network that jointly processes acoustic and linguistic information to perform source separation guided by textual queries. Evaluation on a dataset derived from AudioCaps shows that LASS-Net outperforms baseline methods and effectively handles diverse textual queries.
Second, we present AudioSep, a foundation model for open-domain audio source separation using natural language queries. Trained on large-scale multimodal datasets, AudioSep is evaluated on tasks including audio event separation, musical instrument separation, and speech enhancement. We construct a comprehensive evaluation benchmark for LASS research. AudioSep demonstrates strong separation performance and zero-shot generalization using audio captions or text labels as queries, substantially outperforming previous audio-queried and language-queried separation models. Ablation studies examine the impact of scaling up AudioSep with large-scale multimodal data, providing insights for future research.
Third, we propose WavJourney, a framework that leverages large language models (LLMs) to integrate various audio models for content creation. WavJourney enables users to generate storytelling audio content with diverse elements based on textual descriptions. Given a text instruction, WavJourney prompts an LLM to produce an audio script serving as a structured semantic representation. This script is converted into a computer program, where each line calls a task-specific audio generation model or computational function. Executing the program yields a compositional and interpretable solution for audio creation. Experimental results indicate that WavJourney synthesizes realistic audio aligned with textually described semantic, spatial, and temporal conditions, achieving state-of-the-art results on text-to-audio generation benchmarks. We introduce a new multi-genre story benchmark and demonstrate WavJourney's potential in crafting engaging storytelling audio from text.