3pm - 4pm
Wednesday 4 December 2024
Cross-Domain Computer Vision and Machine Learning for Autonomous Vehicle Navigation
PhD Viva Open Presentation - James Ross
Hybrid event - All Welcome!
Free
University of Surrey
Guildford
Surrey
GU2 7XH
This event has passed
Cross-Domain Computer Vision and Machine Learning for Autonomous Vehicle Navigation
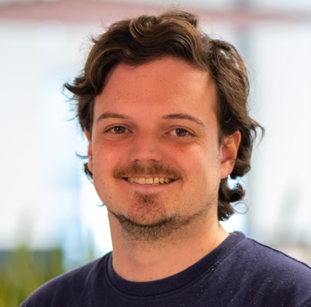
Abstract:
Autonomous vehicles are sophisticated robotic systems that independently navigate unknown or uncertain environments. The real world is complex, and effective navigation requires high-level scene understanding. Breakthroughs in computer vision and the availability of new sensor modalities, such as LiDAR, have enabled the creation of novel spatial representations that preserve layout and semantic information, both essential for robust perceptual awareness.
However, the bulk of existing research focuses on the automotive world; there is little work that brings the technology to other domains or addresses challenges that occur outside automotive. This thesis aims to bridge that gap. Specifically, it aims to address data challenges when shifting to other domains by leveraging simulation and domain transfer techniques, develop mapping systems using state-of-the-art computer vision, and build intuitive, user-friendly representations of unseen environments for use as visual aides as we progress towards full autonomy.
Top-down Bird’s Eye View (BEV) maps are useful representations that naturally lend themselves to navigation and deep learning. They are metric, enabling sensor fusion; orthographic, allowing straightforward alignment and manipulation; and semantic, making them intuitive and easy to parse. This thesis begins by extending BEV prediction to the maritime domain, using a purpose-built simulator, BirdEyeSim, to address the lack of data and perform experiments that are challenging in the real world. A domain transfer technique facilitates BEV network training for real-world prediction.
Then, a new large-scale automotive dataset, Campus Map, is introduced, containing driving and parking data with LiDAR, cameras, GPS and BEV maps. Campus Map builds upon the simulation techniques from Chapter 3 to produce accurate BEV ground truth, addressing a data issue in BEV research. It also enables the quantitative evaluation of BEV mapping systems in the real world, and is made public to encourage research into alternative scene representations.
In the third contribution chapter, BEV is used as a sensor in a novel Simultaneous Localisation and Mapping (SLAM) system, with modifications to improve occlusion reasoning ability. BEV-SLAM produces complete, globally-consistent world maps using only monocular cameras. The semantic, orthographic output is ideal for downstream tasks such as path planning. An initial evaluation is performed in the maritime domain using BirdEyeSim, and a simulated maritime dataset is released publicly.
The final contribution chapter evaluates cutting-edge techniques to produce realistic top-down views from monocular images that complement semantic Bird’s Eye View (BEV) maps. Using the Campus Map dataset, BEV prediction is extended to produce realistic renders, and a study is conducted to gauge the usefulness of the current state-of-the-art and propose a direction for future research.
Combined, this body of work advances the state-of-the-art in autonomous vehicle research and map generation, leveraging simulation to address challenges across domains to provide rich semantic perceptual awareness in unseen environments and support the next generation of autonomous vehicles.