1pm - 2pm
Tuesday 10 September 2024
Distributed Audio-Visual Multi-Target Tracking
PhD Viva Open Presentation for Peipei Wu
Hybrid event - All Welcome!
Free
Arthur C Clarke Building (BA)
University of Surrey
Guildford
Surrey
GU2 7XH
This event has passed
Meeting ID: 373 028 626 322
Passcode: QEY7wa
Distributed Audio-Visual Multi-Target Tracking
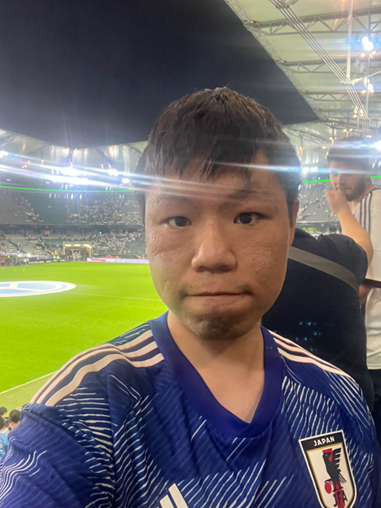
Abstract:
Using audio-visual (AV) sensory data captured by distributed sensors for multi-target tracking has garnered research interest, which has potential applications in speech recognition, human-machine interaction, and surveillance. A recent audio-visual tracking method, i.e. the Audio-Visual Intensity Particle Flow Sequential Monte Carlo Probability Hypothesis Density (AV-IPF-SMC-PHD) filter, demonstrates promising performance with high tracking accuracy in dealing with clutters within measurements. However, this method may be degraded due to miss detections resulting from occlusions.
To address the problem of occlusions, we propose the Occlusion-Aware Audio-Visual Tracker (OAAVT), which introduces an occlusion state to the label space for distinguishing different targets. Label consistency is maintained by updating the associations between measurements and targets based on a likelihood function, ensuring tracking continuity. The occlusion state is determined by an occlusion-aware mechanism using the likelihood function. This method recognizes occlusions per frame and keeps the weights of the particles associated with occluded targets significant, thereby facilitating continuous tracking. Utilizing labelled particles improves tracking accuracy through more accurate clustering of the particles.
However, tracking targets from a single viewpoint may still be limited due to its limited field of view. To address this, we introduce the Distributed IPF-SMC-PHD (D-IFP-SMC-PHD) method for tracking via distributed sensor networks. We propose a novel fusion approach, Confidence-informed Distributed Set-Theoretic Information Flooding Arithmetic Average (C-DSIF-AA), based on arithmetic average fusion to obtain global consensus from local sensor estimates. This method facilitates partial consensus among individual filter outputs and mitigates the impact of unreliable estimates through confidence measures. The confidence scheme assesses sensor node reliability, and filter out unreliable sensor data from both the fusion process and network communications. This approach enhances the precision of distributed fusion, particularly when part of the network sensors are unreliable. However, its effectiveness decreases substantially when the majority of sensors are unreliable.
To overcome this challenge, we introduce a novel approach for distributed fusion based on deep learning, called Distributed Crossmodal Conformer (D-CMCM). First, we implement Optimal Distributed Set-Theoretic Information Flooding (ODSIF) to share local information and balance the weights corresponding to the local and received data. Next, each local node runs the encoding system to convert known information into a Gaussian heat map. Finally, these heat maps are processed by the Crossmodal Conformer (CMCM) to produce distributed fusion results independently at each node. This method eliminates the need for consensus or data association, enhancing the quality of local information in each sensor independently