9:30am - 10:30am
Tuesday 12 March 2024
Audio-Visual Detection and Localisation of Speech and Sound Events
PhD Viva Open Presentation by Davide Berghi.
All Welcome!
Free
University of Surrey
Guildford
Surrey
GU2 7XH
This event has passed
You can join us either in person or online.
Audio-Visual Detection and Localisation of Speech and Sound Events
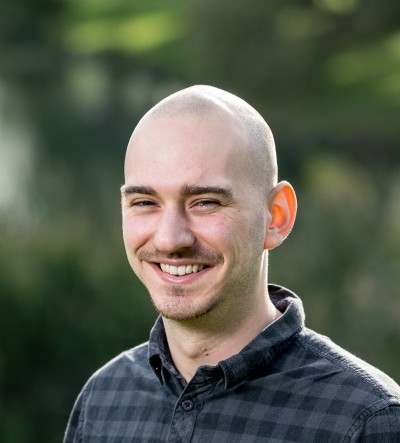
Abstract:
The task of detecting and positioning objects or sound events in space is mainly achieved through audio and visual cues as they embed most spatial awareness and scene understanding. Although vision guarantees high spatial accuracy in localising objects, it is susceptible to visual occlusions and poor lighting conditions. In contrast, audio is omni-directional and can detect sounds even when they are occluded. A system that properly leverages both modalities can capitalise on the strengths of each, or depend on a single modality when the other fails or is not available.
This thesis focuses on two related tasks: video-based Active Speaker Detection and Localisation (ASDL) and Sound Event Localisation and Detection (SELD). Conventional methods to approach these tasks do not take full advantage of the spatial awareness and information that can be derived from the audio and visual modalities. For instance, detecting the active speaker in a video usually involves visual face detection. Therefore, if the face of the active speaker is occluded the overall system fails. Regarding SELD, for years, it has been defined exclusively as an audio task addressed with multichannel audio data. However, vision guarantees more robust localisation accuracy and provides additional cues to help in distinguishing the active sounds. This thesis revisits the ASDL and SELD tasks, aiming to redefine them in a manner that optimally exploits both audio and visual modalities.
A method for ASDL that leverages only the audio modality is proposed to mitigate the limitations derived from visual failures. The goal is to investigate whether these systems can rely solely on audio data in the absence or failure of visual input. This involves extending the audio input front-end from single to multichannel. This extension is underpinned by three key contributions.
The first contribution introduces a novel dataset to support the comparison of traditional audio-visual ASDL and multichannel audio ASDL. This audio-visual dataset includes speech content with audio captured with a microphone array. The second contribution unveils the multichannel audio system for video-based horizontal ASDL. The system not only accomplishes the task but also outperforms in terms of detection and recall rate audio-visual methods that rely on face detection. The third contribution includes experiments and ablations studies aimed at learning how to best leverage multichannel audio and build a deep understanding of its capabilities.
Generating the labels required to train the multichannel audio system is both expensive and time-consuming. Another aspect of this thesis delves into the viability of self-supervised learning solutions to train the multichannel audio system. Specifically, it explores how to leverage the visual data available with the dataset to automatically provide supervision for the audio network. The fourth contribution of this thesis presents a novel “student-teacher” learning method where a pre-trained audio-visual active speaker detector serves as a “teacher” network to produce pseudo-labels automatically. The “student” network is a model trained to produce the same results leveraging multichannel audio input.
The fifth and final contribution of this thesis is the integration of audio and visual feature embeddings for SELD. Leveraging the recently released STARSS23 dataset, this contribution explores methodologies to integrate the visual modality into a problem traditionally addressed with multichannel audio. This integration includes experiments with two different visual feature encoders and two attention-based fusion mechanisms. The proposed methods significantly outperform the baseline systems of the DCASE 2023 Task 3 Challenge by a wide margin. The audio-visual integration tested for SELD is extended to the ASDL task, exhibiting superior performance over the multichannel audio system presented in the previous contributions.
Featured Academics
Visitor information
Find out how to get to the University, make your way around campus and see what you can do when you get here.