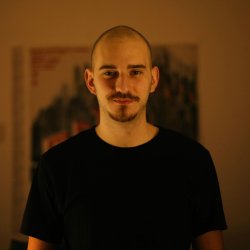
Dr Davide Berghi
Publications
3D audio-visual production aims to deliver immersive and interactive experiences to the consumer. Yet, faithfully reproducing real-world 3D scenes remains a challenging task. This is partly due to the lack of available datasets enabling audio-visual research in this direction. In most of the existing multi-view datasets, the accompanying audio is neglected. Similarly, datasets for spatial audio research primarily offer unimodal content, and when visual data is included, the quality is far from meeting the standard production needs. We present "Tragic Talkers", an audio-visual dataset consisting of excerpts from the "Romeo and Juliet" drama captured with microphone arrays and multiple co-located cameras for light-field video. Tragic Talkers provides ideal content for object-based media (OBM) production. It is designed to cover various conventional talking scenarios, such as monologues, two-people conversations, and interactions with considerable movement and occlusion, yielding 30 sequences captured from a total of 22 different points of view and two 16-element microphone arrays. Additionally, we provide voice activity labels, 2D face bounding boxes for each camera view, 2D pose detection keypoints, 3D tracking data of the mouth of the actors, and dialogue transcriptions. We believe the community will benefit from this dataset as it can assist multidisciplinary research. Possible uses of the dataset are discussed.
As audio-visual systems increasingly bring immersive and interactive capabilities into our work and leisure activities, so the need for naturalistic test material grows. New volumetric datasets have captured high-quality 3D video, but accompanying audio is often neglected, making it hard to test an integrated bimodal experience. Designed to cover diverse sound types and features, the presented volumetric dataset was constructed from audio and video studio recordings of scenes to yield forty short action sequences. Potential uses in technical and scientific tests are discussed.
3D audio-visual production aims to deliver immersive and interactive experiences to the consumer. Yet, faithfully reproducing real-world 3D scenes remains a challenging task. This is partly due to the lack of available datasets enabling audio-visual research in this direction. In most of the existing multi-view datasets, the accompanying audio is neglected. Similarly, datasets for spatial audio research primarily offer unimodal content, and when visual data is included, the quality is far from meeting the standard production needs. We present “Tragic Talkers”, an audio-visual dataset consisting of excerpts from the “Romeo and Juliet” drama captured with microphone arrays and multiple co-located cameras for light-field video. Tragic Talkers provides ideal content for object-based media (OBM) production. It is designed to cover various conventional talking scenarios, such as monologues, two-people conversations, and interactions with considerable movement and occlusion, yielding 30 sequences captured from a total of 22 different points of view and two 16-element microphone arrays. Additionally, we provide voice activity labels, 2D face bounding boxes for each camera view, 2D pose detection keypoints, 3D tracking data of the mouth of the actors, and dialogue transcriptions. We believe the community will benefit from this dataset as it can assist multidisciplinary research. Possible uses of the dataset are discussed. The scenes were captured at the Centre for Vision, Speech & Signal Processing (CVSSP) of the University of Surrey (UK) with the aid of two twin Audio-Visual Array (AVA) Rigs. Each AVA Rig is a custom device consisting of a 16-element microphone array and 11 cameras fixed on a flat perspex sheet. For more information, please refer to the paper (see below) or contact the authors.
Immersive audio-visual perception relies on the spatial integration of both auditory and visual information which are heterogeneous sensing modalities with different fields of reception and spatial resolution. This study investigates the perceived coherence of audiovisual object events presented either centrally or peripherally with horizontally aligned/misaligned sound. Various object events were selected to represent three acoustic feature classes. Subjective test results in a simulated virtual environment from 18 participants indicate a wider capture region in the periphery, with an outward bias favoring more lateral sounds. Centered stimulus results support previous findings for simpler scenes.
This study considers the problem of detecting and locating an active talker's horizontal position from multichannel audio captured by a microphone array. We refer to this as active speaker detection and localization (ASDL). Our goal was to investigate the performance of spatial acoustic features extracted from the multichannel audio as the input of a convolutional recurrent neural network (CRNN), in relation to the number of channels employed and additive noise. To this end, experiments were conducted to compare the generalized cross-correlation with phase transform (GCC-PHAT), the spatial cue-augmented log-spectrogram (SALSA) features, and a recently-proposed beamforming method, evaluating their robustness to various noise intensities. The array aperture and sampling density were tested by taking subsets from the 16-microphone array. Results and tests of statistical significance demonstrate the microphones' contribution to performance on the TragicTalkers dataset, which offers opportunities to investigate audio-visual approaches in the future.
—Active speaker detection (ASD) is a multi-modal task that aims to identify who, if anyone, is speaking from a set of candidates. Current audiovisual approaches for ASD typically rely on visually pre-extracted face tracks (sequences of consecutive face crops) and the respective monaural audio. However, their recall rate is often low as only the visible faces are included in the set of candidates. Monaural audio may successfully detect the presence of speech activity but fails in localizing the speaker due to the lack of spatial cues. Our solution extends the audio front-end using a microphone array. We train an audio convolutional neural network (CNN) in combination with beamforming techniques to regress the speaker's horizontal position directly in the video frames. We propose to generate weak labels using a pre-trained active speaker detector on pre-extracted face tracks. Our pipeline embraces the " student-teacher " paradigm, where a trained " teacher " network is used to produce pseudo-labels visually. The " student " network is an audio network trained to generate the same results. At inference, the student network can independently localize the speaker in the visual frames directly from the audio input. Experimental results on newly collected data prove that our approach significantly outperforms a variety of other baselines as well as the teacher network itself. It results in an excellent speech activity detector too.
Audio-visual speaker tracking has drawn increasing attention over the past few years due to its academic values and wide application. Audio and visual modalities can provide complementary information for localization and tracking. With audio and visual information, the Bayesian-based filter can solve the problem of data association, audio-visual fusion and track management. In this paper, we conduct a comprehensive overview of audio-visual speaker tracking. To our knowledge, this is the first extensive survey over the past five years. We introduce the family of Bayesian filters and summarize the methods for obtaining audio-visual measurements. In addition, the existing trackers and their performance on AV16.3 dataset are summarized. In the past few years, deep learning techniques have thrived, which also boosts the development of audio visual speaker tracking. The influence of deep learning techniques in terms of measurement extraction and state estimation is also discussed. At last, we discuss the connections between audio-visual speaker tracking and other areas such as speech separation and distributed speaker tracking.
Sound event localization and detection (SELD) combines two subtasks: sound event detection (SED) and direction of arrival (DOA) estimation. SELD is usually tackled as an audio-only problem, but visual information has been recently included. Few audio-visual (AV)-SELD works have been published and most employ vision via face/object bounding boxes, or human pose keypoints. In contrast, we explore the integration of audio and visual feature embeddings extracted with pre-trained deep networks. For the visual modality, we tested ResNet50 and Inflated 3D ConvNet (I3D). Our comparison of AV fusion methods includes the AV-Conformer and Cross-Modal Attentive Fusion (CMAF) model. Our best models outperform the DCASE 2023 Task3 audio-only and AV baselines by a wide margin on the development set of the STARSS23 dataset, making them competitive amongst state-of-the-art results of the AV challenge, without model ensembling, heavy data augmentation, or prediction post-processing. Such techniques and further pre-training could be applied as next steps to improve performance.
Conventional audio-visual approaches for active speaker detection (ASD) typically rely on visually pre-extracted face tracks and the corresponding single-channel audio to find the speaker in a video. Therefore, they tend to fail every time the face of the speaker is not visible. We demonstrate that a simple audio convolutional recurrent neural network (CRNN) trained with spatial input features extracted from multichannel audio can perform simultaneous horizontal active speaker detection and localization (ASDL), independently of the visual modality. To address the time and cost of generating ground truth labels to train such a system, we propose a new self-supervised training pipeline that embraces a "student-teacher" learning approach. A conventional pre-trained active speaker detector is adopted as a "teacher" network to provide the position of the speakers as pseudo-labels. The multichannel audio "student" network is trained to generate the same results. At inference, the student network can generalize and locate also the occluded speakers that the teacher network is not able to detect visually, yielding considerable improvements in recall rate. Experiments on the TragicTalkers dataset show that an audio network trained with the proposed self-supervised learning approach can exceed the performance of the typical audio-visual methods and produce results competitive with the costly conventional supervised training. We demonstrate that improvements can be achieved when minimal manual supervision is introduced in the learning pipeline. Further gains may be sought with larger training sets and integrating vision with the multichannel audio system.
This study considers the problem of detecting and locating an active talker's horizontal position from multichannel audio captured by a microphone array. We refer to this as active speaker detection and localization (ASDL). Our goal was to investigate the performance of spatial acoustic features extracted from the multichan-nel audio as the input of a convolutional recurrent neural network (CRNN), in relation to the number of channels employed and additive noise. To this end, experiments were conducted to compare the generalized cross-correlation with phase transform (GCC-PHAT), the spatial cue-augmented log-spectrogram (SALSA) features, and a recently-proposed beamforming method, evaluating their robust-ness to various noise intensities. The array aperture and sampling density were tested by taking subsets from the 16-microphone array. Results and tests of statistical significance demonstrate the micro-phones' contribution to performance on the TragicTalkers dataset, which offers opportunities to investigate audiovisual approaches in the future.
Leveraging machine learning techniques, in the context of object-based media production, could enable provision of personalized media experiences to diverse audiences. To fine-tune and evaluate techniques for personalization applications, as well as more broadly, datasets which bridge the gap between research and production are needed. We introduce and publicly release such a dataset, themed around a UK weather forecast and shot against a blue-screen background, of three professional actors/presenters – one male and one female (English) and one female (British Sign Language). Scenes include both production and research-oriented examples, with a range of dialogue, motions, and actions. Capture techniques consisted of a synchronized 4K resolution 16-camera array, production-typical microphones plus professional audio mix, a 16-channel microphone array with collocated Grasshopper3 camera, and a photogrammetry array. We demonstrate applications relevant to virtual production and creation of personalized media including neural radiance fields, shadow casting, action/event detection, speaker source tracking and video captioning.
IEEE VR 2020 Immersive audio-visual perception relies on the spatial integration of both auditory and visual information which are heterogeneous sensing modalities with different fields of reception and spatial resolution. This study investigates the perceived coherence of audiovisual object events presented either centrally or peripherally with horizontally aligned/misaligned sound. Various object events were selected to represent three acoustic feature classes. Subjective test results in a simulated virtual environment from 18 participants indicate a wider capture region in the periphery, with an outward bias favoring more lateral sounds. Centered stimulus results support previous findings for simpler scenes.
This study considers the problem of detecting and locating an active talker's horizontal position from multichannel audio captured by a microphone array. We refer to this as active speaker detection and localization (ASDL). Our goal was to investigate the performance of spatial acoustic features extracted from the multichannel audio as the input of a convolutional recurrent neural network (CRNN), in relation to the number of channels employed and additive noise. To this end, experiments were conducted to compare the generalized cross-correlation with phase transform (GCC-PHAT), the spatial cue-augmented log-spectrogram (SALSA) features, and a recently-proposed beamforming method, evaluating their robustness to various noise intensities. The array aperture and sampling density were tested by taking subsets from the 16-microphone array. Results and tests of statistical significance demonstrate the microphones' contribution to performance on the TragicTalkers dataset, which offers opportunities to investigate audio-visual approaches in the future.
3D audiovisual production aims to deliver immersive and interactive experiences to the consumer. Yet, faithfully reproducing real-world 3D scenes remains a challenging task. This is partly due to the lack of available datasets enabling audiovisual research in this direction. In most of the existing multi-view datasets, the accompanying audio is neglected. Similarly, datasets for spatial audio research primarily offer unimodal content, and when visual data is included, the quality is far from meeting the standard production needs. We present " Tragic Talkers " , an audiovisual dataset consisting of excerpts from the " Romeo and Juliet " drama captured with microphone arrays and multiple co-located cameras for light-field video. Tragic Talkers provides ideal content for object-based media (OBM) production. It is designed to cover various conventional talking scenarios, such as monologues, two-people conversations, and interactions with considerable movement and occlusion, yielding 30 sequences captured from a total of 22 different points of view and two 16-element microphone arrays. Additionally, we provide voice activity labels, 2D face bounding boxes for each camera view, 2D pose detection keypoints, 3D tracking data of the mouth of the actors, and dialogue transcriptions. We believe the community will benefit from this dataset as it can assist multidisciplinary research. Possible uses of the dataset are discussed. * This is the author's version of the work. It is posted here for your personal use. This paper is published under a Creative Commons Attribution (CC-BY) license. The definitive version was published in CVMP '22, https://doi.org/10.1145/3565516.3565522.