11am - 12 noon
Thursday 14 December 2023
Generating virtual camera views using generative networks
PhD Viva Open Presentation by Violeta Menendez Gonzalez.
All welcome!
Free
2ND FLOOR OF THE ARTHUR C. CLARKE BUILDING
University of Surrey
Guildford
Surrey
GU2 7XH
This event has passed
Speakers
Abstract
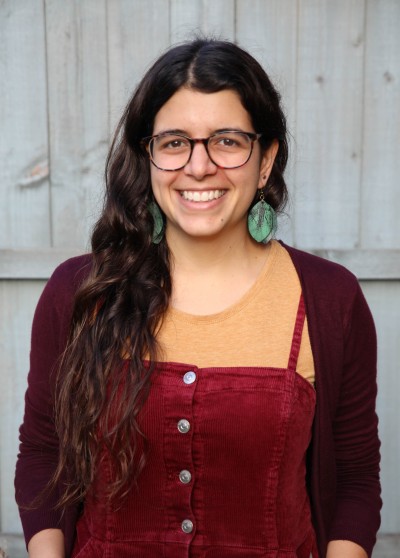
The challenge of novel view synthesis involves generating new synthetic images from different points of view based on existing views. This task is essential for various creative endeavours, like computer graphics, free viewpoint video, and media production. The primary motivation driving our research lies within television production and event coverage of resource-constrained events, where ideal camera positions are often limited.
Our goal is to overcome physical limitations by computationally generating optimal camera views. However, this task comes with its own set of challenges, including constraints on the number of available cameras and specialised equipment, feasible camera positions, and computational resources.
The task of novel view synthesis is intrinsically ill-posed, as there exist many equally valid solutions to a single problem, and the presence of these physical constraints further amplifies the uncertainty and ambiguity of the problem. Traditional computer vision methods struggle to capture scene semantics and reconstruct high ambiguity areas given a sparse set of inputs. Instead, we harness the power of generative deep learning algorithms to recreate realistic and consistent novel content.
We first delve into inpainting techniques designed to fill the information gaps resulting from disocclusions caused by the camera change of perspective. Our research begins with a stereo camera scenario, wherein the inpainting model learns to see behind objects and reconstruct the disocclusion holes while ensuring consistency across cameras.
We introduce a novel deep learning framework that transforms the inpainting problem into a self-supervised task. This involves the use of a bank of geometrically-meaningful object masks, comprising context and synthesis areas used to create virtual objects on our datasets. The network then recovers image content by propagating textures and structures in these synthesis areas, conditioned on the information available in the context regions. Multi-camera consistency is achieved by leveraging the stereo-camera object context and the introduction of a disparity-based training loss.
Subsequently, our research explores more complex and realistic event capture scenarios and camera arrangements, including larger baseline changes and different camera planes. This new setting results in more significant disocclusions in areas unseen by any camera input. To address this, we study sparse scene-agnostic representation methods based on Neural Radiance Fields, which facilitate generalisation from a limited number of input views, suitable for both static and dynamic scenes.
We employ convolutional encoding volumes to aid the networks in learning general geometry and motion priors. To enhance our system's creative capabilities, we also leverage adversarial training to hallucinate novel and diverse content in missing areas. This allows us to represent and render new points of view without the need for scene-specific optimisation.
Featured Academics
Visitor information
Find out how to get to the University, make your way around campus and see what you can do when you get here.