11am - 12 noon
Friday 17 November 2023
Towards efficient temporal activity detection from videos
PhD Viva Open Presentation by Sauradip Nag.
All welcome!
Free
University of Surrey
Guildford
Surrey
GU2 7XH
This event has passed
Abstract
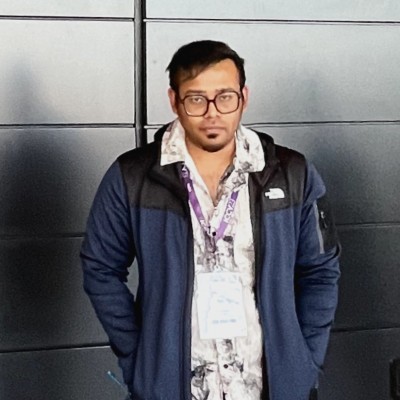
With the number of videos grows tremendously, video understanding becomes a hot question and a challenging direction in computer vision. Temporal action detection (TAD) is thus one of the most crucial and challenging problems for video understanding in computer vision. It has wide use-cases in Social-media, Healthcare and Film Industry.
For a long untrimmed video, temporal action localisation mainly solves two tasks which are recognition and localisation. Specifically,
- When does the action occur, that is the start time and the end time of the action
- What category does each proposal belong to (such as waving, climbing, or basketball-dunk).
Although both action recognition and action detection are important tasks of video understanding, temporal action detection is more challenging than action recognition. The difficulties mostly lies in:
- Temporal complexity
- Costly annotation
- Class imbalance
- Real-world generalisability.
This thesis is aimed in solving these aforementioned issues in a holistic fashion. To solve temporal complexity, we reformulate the inefficient proposal-based start/end regression problem into a more efficient proposal-free binary action mask prediction problem. This gives our model faster training and inference speed than the competitors.
In the second part of our thesis, we discover that existing TAD models suffers from localisation-error propagation issue due to the inherent two-stage (proposal prediction then classification) design. When trained with partial labels (e.g. semi-supervised setting) it worsens further thus leading to inferior performance. We solved this by proposing a novel single-stage design and a novel self-supervised pre-text task to utilize unlabeled videos.
The third chapter focuses on TAD problem in even fewer data (e.g. few-shot setting). In such a scenario, it suffers from intra-class variance in the support set thus our solution lies in designs that mitigate this variance. We further showcase that incorporating multimodal data (e.g video and text) in the support set can solve this intra-class variance further.
Finally, in the last chapter, we focus on solving TAD when there is no annotation for the novel open-world videos (i.e zero-shot setting). We utilized the large-scale pretraining of vision-language models to compensate for the lack of training annotations. We also demonstrate that large-scale models are not directly applicable to dense-detection tasks like TAD.
Given the contributions above, this thesis proposes to solve the problem of TAD towards making it more practical and generalizable to real world. As we have shown a potential designs and methods to solve TAD problems in various data scarce and efficiency scenario, we suggest future work focuses on more general direction of generative modeling and also extending the algorithms to egocentric videos.
Visitor information
Find out how to get to the University, make your way around campus and see what you can do when you get here.