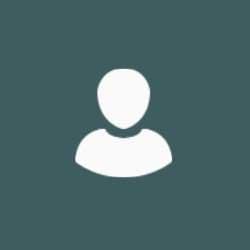
Dr Sauradip Nag
About
My research project
Hierarchical visual activity analysisIn this project, we aim to develop models for recognising complex and hierarchical visual activities. The current state-of-the-art methods such as variants of the two-steam network or 3D CNNs work very well on sports/film type videos, but struggle with complex activities with hierarchical structure such as cooking and assembling flat pack furniture. Analysing these activities is only achievable by delving deep into the interaction between humans and objects and compositionally modelling the hierarchical structure. To this end, we will develop novel compositional learning models and exploit deep graph convolutional networks for structured data analysis.
In this project, we aim to develop models for recognising complex and hierarchical visual activities. The current state-of-the-art methods such as variants of the two-steam network or 3D CNNs work very well on sports/film type videos, but struggle with complex activities with hierarchical structure such as cooking and assembling flat pack furniture. Analysing these activities is only achievable by delving deep into the interaction between humans and objects and compositionally modelling the hierarchical structure. To this end, we will develop novel compositional learning models and exploit deep graph convolutional networks for structured data analysis.
Publications
Sound Event Detection (SED) aims to predict the temporal boundaries of all the events of interest and their class labels, given an unconstrained audio sample. Taking either the splitand-classify (i.e., frame-level) strategy or the more principled event-level modeling approach, all existing methods consider the SED problem from the discriminative learning perspective. In this work, we reformulate the SED problem by taking a generative learning perspective. Specifically, we aim to generate sound temporal boundaries from noisy proposals in a denoising diffusion process, conditioned on a target audio sample. During training, our model learns to reverse the noising process by converting noisy latent queries to the groundtruth versions in the elegant Transformer decoder framework. Doing so enables the model generate accurate event boundaries from even noisy queries during inference. Extensive experiments on the Urban-SED and EPIC-Sounds datasets demonstrate that our model significantly outperforms existing alternatives, with 40+% faster convergence in training.
Sound Event Detection (SED) aims to predict the temporal boundaries of all the events of interest and their class labels, given an unconstrained audio sample. Taking either the split-and-classify (i.e., frame-level) strategy or the more principled event-level modeling approach, all existing methods consider the SED problem from the discriminative learning perspective. In this work, we reformulate the SED problem by taking a generative learning perspective. Specifically, we aim to generate sound temporal boundaries from noisy proposals in a denoising diffusion process, conditioned on a target audio sample. During training, our model learns to reverse the noising process by converting noisy latent queries to the groundtruth versions in the elegant Transformer decoder framework. Doing so enables the model generate accurate event boundaries from even noisy queries during inference. Extensive experiments on the Urban-SED and EPIC-Sounds datasets demonstrate that our model significantly outperforms existing alternatives, with 40+% faster convergence in training. Code: https://github.com/Surrey-UPLab/DiffSED.
Existing temporal action detection (TAD) methods rely on large training data including segment-level annotations, limited to recognizing previously seen classes alone during inference. Collecting and annotating a large training set for each class of interest is costly and hence unscalable. Zero-shot TAD (ZS-TAD) resolves this obstacle by enabling a pre-trained model to recognize any unseen action classes. Meanwhile, ZS-TAD is also much more challenging with significantly less investigation. Inspired by the success of zero-shot image classification aided by vision-language (ViL) models such as CLIP, we aim to tackle the more complex TAD task. An intuitive method is to integrate an off-the-shelf proposal detector with CLIP style classification. However, due to the sequential localization (e.g., proposal generation) and classification design, it is prone to localization error propagation. To overcome this problem, in this paper we propose a novel zero-Shot Temporal Action detection model via Vision-LanguagE prompting (STALE). Such a novel design effectively eliminates the dependence between localization and classification by breaking the route for error propagation in-between. We further introduce an interaction mechanism between classification and localization for improved optimization. Extensive experiments on standard ZS-TAD video benchmarks show that our STALE significantly outperforms state-of-the-art alternatives. Besides, our model also yields superior results on supervised TAD over recent strong competitors. The PyTorch implementation of STALE is available on https://github.com/sauradip/STALE.
Garment pattern design aims to convert a 3D garment to the corresponding 2D panels and their sewing structure. Existing methods rely either on template fitting with heuristics and prior assumptions, or on model learning with complicated shape parameterization. Importantly, both approaches do not allow for personalization of the output garment, which today has increasing demands. To fill this demand, we introduce PersonalTailor: a personalized 2D pattern design method, where the user can input specific constraints or demands (in language or sketch) for personal 2D panel fabrication from 3D point clouds. PersonalTailor first learns a multi-modal panel embeddings based on unsupervised cross-modal association and attentive fusion. It then predicts a binary panel masks individually using a transformer encoder-decoder framework. Extensive experiments show that our PersonalTailor excels on both personalized and standard pattern fabrication tasks.
Existing Temporal Action Detection (TAD) methods typ- ically take a pre-processing step in converting an input varying-length video into a fixed-length snippet represen- tation sequence, before temporal boundary estimation and action classification. This pre-processing step would tem- porally downsample the video, reducing the inference res- olution and hampering the detection performance in the original temporal resolution. In essence, this is due to a temporal quantization error introduced during the resolu- tion downsampling and recovery. This could negatively im- pact the TAD performance, but is largely ignored by existing methods. To address this problem, in this work we intro- duce a novel model-agnostic post-processing method with- out model redesign and retraining. Specifically, we model the start and end points of action instances with a Gaussian distribution for enabling temporal boundary inference at a sub-snippet level. We further introduce an efficient Taylor- expansion based approximation, dubbed as Gaussian Ap- proximated Post-processing (GAP). Extensive experiments demonstrate that our GAP can consistently improve a wide variety of pre-trained off-the-shelf TAD models on the chal- lenging ActivityNet (+0.2%∼0.7% in average mAP) and THUMOS (+0.2%∼0.5% in average mAP) benchmarks. Such performance gains are already significant and highly comparable to those achieved by novel model designs. Also, GAP can be integrated with model training for further performance gain. Importantly, GAP enables lower tem- poral resolutions for more efficient inference, facilitating low-resource applications. The code will be available in https://github.com/sauradip/GAP
Existing action recognition methods are typically actor-specific due to the intrinsic topological and apparent differences among the actors. This requires actor-specific pose estimation (e.g., humans vs. animals), leading to cumbersome model design complexity and high maintenance costs. Moreover, they often focus on learning the visual modality alone and single-label classification whilst neglecting other available information sources (e.g., class name text) and the concurrent occurrence of multiple actions. To overcome these limitations, we propose a new approach called 'actor-agnostic multi-modal multi-label action recognition,' which offers a unified solution for various types of actors, including humans and animals. We further formulate a novel Multi-modal Semantic Query Network (MSQNet) model in a transformer-based object detection framework (e.g., DETR), characterized by leveraging visual and textual modalities to represent the action classes better. The elimination of actor-specific model designs is a key advantage, as it removes the need for actor pose estimation altogether. Extensive experiments on five publicly available benchmarks show that our MSQNet consistently outperforms the prior arts of actor-specific alternatives on human and animal single- and multi-label action recognition tasks by up to 50%. Code is made available at https://github.com/mondalanindya/MSQNet.
Existing temporal action detection (TAD) methods rely on a large number of training data with segment-level annotations. Collecting and annotating such a training set is thus highly expensive and unscalable. Semi-supervised TAD (SS-TAD) alleviates this problem by leveraging unlabeled videos freely available at scale. However, SS-TAD is also a much more challenging problem than supervised TAD, and consequently much under-studied. Prior SS-TAD methods directly combine an existing proposal-based TAD method and a SSL method. Due to their sequential localization (e.g., proposal generation) and classification design, they are prone to proposal error propagation. To overcome this limitation, in this work we propose a novel Semi - supervised Temporal action detection model based on PropOsal - free Temporal mask (SPOT) with a parallel localization (mask generation) and classification architecture. Such a novel design effectively eliminates the dependence between localization and classification by cutting off the route for error propagation in-between. We further introduce an interaction mechanism between classification and localization for prediction refinement, and a new pretext task for self-supervised model pre-training. Extensive experiments on two standard benchmarks show that our SPOT outperforms state-of-the-art alternatives, often by a large margin. The PyTorch implementation of SPOT is available at https://github.com/sauradip/SPOT
Existing temporal action detection (TAD) methods rely on generating an overwhelmingly large number of proposals per video. This leads to complex model designs due to proposal generation and/or per-proposal action instance evaluation and the resultant high computational cost. In this work, for the first time, we propose a proposal-free Temporal Action detection model via Global Segmentation mask (TAGS). Our core idea is to learn a global segmentation mask of each action instance jointly at the full video length. The TAGS model differs significantly from the conventional proposal-based methods by focusing on global temporal representation learning to directly detect local start and end points of action instances without proposals. Further, by modeling TAD holistically rather than locally at the individual proposal level, TAGS needs a much simpler model architecture with lower computational cost. Extensive experiments show that despite its simpler design, TAGS outperforms existing TAD methods, achieving new state-of-the-art performance on two benchmarks. Importantly, it is -20x faster to train and -1.6x more efficient for inference. Our PyTorch implementation of TAGS is available at https://github.com/sauradip/TAGS.
Existing action recognition methods are typically actor-specific due to the intrinsic topological and apparent differences among the actors. This requires actor-specific pose estimation (e.g., humans vs. animals), leading to cumbersome model design complexity and high maintenance costs. Moreover, they often focus on learning the visual modality alone and single-label classification whilst neglecting other available information sources (e.g., class name text) and the concurrent occurrence of multiple actions. To overcome these limitations, we propose a new approach called 'actor-agnostic multi-modal multi-label action recognition,' which offers a unified solution for various types of actors, including humans and animals. We further formulate a novel Multi-modal Semantic Query Network (MSQNet) model in a transformer-based object detection framework (e.g., DETR), characterized by leveraging visual and textual modalities to represent the action classes better. The elimination of actor-specific model designs is a key advantage, as it removes the need for actor pose estimation altogether. Extensive experiments on five publicly available benchmarks show that our MSQNet consistently outperforms the prior arts of actor-specific alternatives on human and animal single- and multi-label action recognition tasks by up to 50%. Code will be released at https://github.com/mondalanindya/MSQNet.