11am - 12 noon
Tuesday 26 September 2023
Self-supervised 3D reconstruction in general complex dynamic scenes
PhD Viva Programme for Mertalp Öcal.
Supervisors: Prof Adrian Hilton and Dr Armin Mustafa.
Free
University of Surrey
Guildford
Surrey
GU2 7XH
This event has passed
Abstract
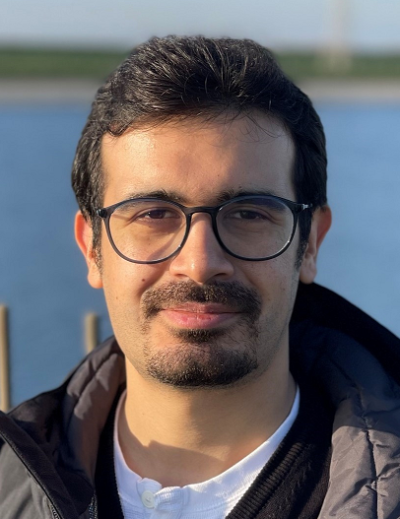
Similar to human visual perception, robust and smart computer vision systems require the ability to perceive the environment in 3D. Existing dynamic 3D reconstruction methods require either/both:
- Expensive depth sensor hardware such as LiDAR for measurement of sparse 3D points or RGB-D camera which is limited to indoor operation
- Template or a prior scene model which limits their application to a narrow range of moving objects or scenes. We circumvent these limitations with an emphasis on unsupervised/self-supervised learning.
The first contribution presents a generalised self-supervised learning approach for monocular estimation of the real depth across scenes with diverse depth ranges from 1-100s of meters. Existing supervised methods for monocular depth estimation require accurate depth measurements for training. This limitation has led to the introduction of self-supervised methods that are trained on stereo image pairs with a fixed camera baseline to estimate disparity which is transformed to depth given known calibration.
Self-supervised approaches have demonstrated impressive results but do not generalise to scenes with different depth ranges or camera baselines. In this chapter, we introduce RealMonoDepth a self-supervised monocular depth estimation approach which learns to estimate the real scene depth for a diverse range of indoor and outdoor scenes. A novel loss function with respect to the true scene depth based on relative depth scaling and warping is proposed.
This allows self-supervised training of a single network with multiple data sets for scenes with diverse depth ranges from both stereo pair and in the wild moving camera data sets. A comprehensive performance evaluation across five benchmark data sets demonstrates that RealMonoDepth provides a single trained network which generalises depth estimation across indoor and outdoor scenes, consistently outperforming previous self-supervised approaches.
Another key drawback of existing self-supervised methods is that they cannot handle moving objects due to limitations of epipolar geometry to static scenes. To address this issue, some approaches rely on pretrained networks to predict optical flow and/or semantic segmentation to impose constraints on moving objects. Success of these methods heavily depends on generalisation ability of pretrained networks which restricts their application to constrained environments.
To overcome this limitation, the second chapter presents a unified framework for simultaneous self-supervised learning of monocular depth and 3D motion from calibrated videos without relying on any auxilary supervision such as optical flow and segmentation.
Our model does not require any pseudo labels and is based on a weak assumption of physics such that dynamic objects undergo constant velocity between time-equidistant frames in a short temporal window. This constraint allows unambiguous reconstruction of depth and 3D motion using at least three frames.
We propose estimation of 3D motion in normalised device coordinates (NDC) which is invariant to both camera matrix and scale of the scene. This allows generalised training on combination of different videos. We introduce a novel multi-frame training pipeline to combine photometric loss with velocity constancy and depth consistency.
Featured Academics
Visitor information
Find out how to get to the University, make your way around campus and see what you can do when you get here.