11am - 12 noon GMT
Friday 17 December 2021
A mathematical investigation of community boundaries on urban crime and policing and data-driven analysis of complex dynamical systems talks
Free
This event has passed
This seminar will take place online via Zoom.
Overview
This seminar comprises of two talks: 'A mathematical investigation of community boundaries on urban crime and policing' by Professor David Lloyd and 'data-driven analysis of complex dynamical systems' by Dr Stefan Klus.
Keynote talks
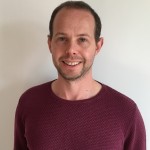
A mathematical investigation of community boundaries on urban crime and policing
Professor David Lloyd
Speaker
About the talk
The effect of communities on crime is a well studied topic in criminology where increased crime is related to intrinsic community properties such as concentrated disadvantage, residential stability and immigrant concentration; see for instance Morenoff, Sampson, Raudenbush (2001). Furthermore, with the emergence of predictive policing (such as PredPol and HunchLab) there is an increased interest in understanding how the effect of different communities impacts urban crime and policing; see for instance the report by Liberty on Policing by Machine (2019).
In this talk, I present a phenomenological model for how urban crime occurs (capturing the criminological theories of repeat victimisation and broken windows) with hotspot policing strategies in an agent-based model. We then show some simulations and demonstrate how one can write down a mean-field approximation of the agent-based model that allows for mathematical analysis in two crime hotspot forming limits across different communities to try and learn some qualitative lessons for policing across different communities.
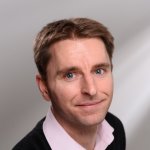
Data-driven analysis of complex dynamical systems
Dr Stefan Klus
Speaker
About the talk
Over the last few years, numerical methods for the analysis of large data sets have gained a lot of attention. Recently, different purely data-driven methods have been proposed which enable the user to extract relevant information about the global behaviour of the underlying dynamical system, to identify low-order dynamics, and to compute finite-dimensional approximations of transfer operators associated with the system.
However, due to the curse of dimensionality, analysing high-dimensional systems is often infeasible using conventional methods since the amount of memory required to compute and store the results grows exponentially with the size of the system.
We extend transfer operator theory to reproducing kernel Hilbert spaces and show that these operators are related to Hilbert space representations of conditional distributions, known as conditional mean embeddings in the machine learning community. One main benefit of the presented kernel-based approaches is that these methods can be applied to any domain where a similarity measure given by a kernel is available. We will illustrate the results with the aid of guiding examples and highlight potential applications in molecular dynamics, fluid dynamics, and quantum mechanics.
Join the seminar
This is an online seminar taking place via Zoom, please join us 11am - 12 noon UK time.
Get in contact
If you have any questions then please contact the organiser Matteo Barberis at m.barberis@surrey.ac.uk.