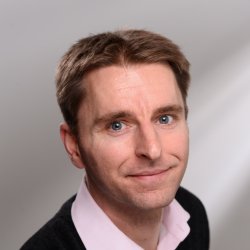
Dr Stefan Klus (habil.)
ResearchResearch interests
- Data-driven model reduction and transfer operator approximation
- Reproducing kernel Hilbert spaces and statistical learning theory
- Molecular dynamics, fluid dynamics, quantum mechanics
- Low-rank tensor decompositions
- Multiscale systems
- Real-time simulation and high-performance computing
- Numerical solution of differential-algebraic equations
- Graph theory
- Combinatorial optimization
Research interests
- Data-driven model reduction and transfer operator approximation
- Reproducing kernel Hilbert spaces and statistical learning theory
- Molecular dynamics, fluid dynamics, quantum mechanics
- Low-rank tensor decompositions
- Multiscale systems
- Real-time simulation and high-performance computing
- Numerical solution of differential-algebraic equations
- Graph theory
- Combinatorial optimization
Publications
Generation and analysis of time-series data is relevant to many quantitative fields ranging from economics to fluid mechanics. In the physical sciences, structures such as metastable and coherent sets, slow relaxation processes, collective variables, dominant transition pathways or manifolds and channels of probability flow can be of great importance for understanding and characterizing the kinetic, thermodynamic and mechanistic properties of the system. Deeptime is a general purpose Python library offering various tools to estimate dynamical models based on time-series data including conventional linear learning methods, such as Markov state models (MSMs), Hidden Markov Models and Koopman models, as well as kernel and deep learning approaches such as VAMPnets and deep MSMs. The library is largely compatible with scikit-learn, having a range of Estimator classes for these different models, but in contrast to scikit-learn also provides deep Model classes, e.g. in the case of an MSM, which provide a multitude of analysis methods to compute interesting thermodynamic, kinetic and dynamical quantities, such as free energies, relaxation times and transition paths. The library is designed for ease of use but also easily maintainable and extensible code. In this paper we introduce the main features and structure of the deeptime software. Deeptime can be found under https://deeptime-ml.github.io/.
More and more diseases have been found to be strongly correlated with disturbances in the microbiome constitution, e.g., obesity, diabetes, or some cancer types. Thanks to modern high-throughput omics technologies, it becomes possible to directly analyze human microbiome and its influence on the health status. Microbial communities are monitored over long periods of time and the associations between their members are explored. These relationships can be described by a time-evolving graph. In order to understand responses of the microbial community members to a distinct range of perturbations such as antibiotics exposure or diseases and general dynamical properties, the time-evolving graph of the human microbial communities has to be analyzed. This becomes especially challenging due to dozens of complex interactions among microbes and metastable dynamics. The key to solving this problem is the representation of the time-evolving graphs as fixed-length feature vectors preserving the original dynamics. We propose a method for learning the embedding of the time-evolving graph that is based on the spectral analysis of transfer operators and graph kernels. We demonstrate that our method can capture temporary changes in the time-evolving graph on both synthetic data and real-world data. Our experiments demonstrate the efficacy of the method. Furthermore, we show that our method can be applied to human microbiome data to study dynamic processes.
Recent years have seen rapid advances in the data-driven analysis of dynamical systems based on Koopman operator theory and related approaches. On the other hand, low-rank tensor product approximations – in particular the tensor train (TT) format – have become a valuable tool for the solution of large-scale problems in a number of fields. In this work, we combine Koopman-based models and the TT format, enabling their application to high-dimensional problems in conjunction with a rich set of basis functions or features. We derive efficient algorithms to obtain a reduced matrix representation of the system’s evolution operator starting from an appropriate low-rank representation of the data. These algorithms can be applied to both stationary and non-stationary systems. We establish the infinite-data limit of these matrix representations, and demonstrate our methods’ capabilities using several benchmark data sets.
In the last years, ensemble methods have been widely popular in atmospheric, climate, and ocean dynamics investigations and forecasts as convenient methods to obtain statistical information on these systems. In many cases, ensembles have been used as an approximation to the probability distribution that has acquired more and more a central role, as the importance of a single trajectory, or member, was recognized as less informative. This paper shows that using results from the dynamical systems and more recent results from the machine learning and AI communities, we can arrive at a direct estimation of the probability distribution evolution and also at the formulation of predictor systems based on a nonlinear formulation. The paper introduces the theory and demonstrates its application to two examples. The first is a one-dimensional system based on the Nino-3 index; the second is a multidimensional case based on time series of monthly mean SST in the Pacific. We show that we can construct the probability distribution and set up a system to forecast its evolution and derive various quantities from it. The objective of the paper is not strict realism, but the introduction of these methods and the demonstration that they can be used also in the complex, multidimensional environment typical of atmosphere and ocean applications.
We derive symmetric and antisymmetric kernels by symmetrizing and antisymmetrizing conventional kernels and analyze their properties. In particular, we compute the feature space dimensions of the resulting polynomial kernels, prove that the reproducing kernel Hilbert spaces induced by symmetric and antisymmetric Gaussian kernels are dense in the space of symmetric and antisymmetric functions, and propose a Slater determinant representation of the antisymmetric Gaussian kernel, which allows for an efficient evaluation even if the state space is high-dimensional. Furthermore, we show that by exploiting symmetries or antisymmetries the size of the training data set can be significantly reduced. The results are illustrated with guiding examples and simple quantum physics and chemistry applications.
We propose a method for the approximation of high- or even infinite-dimensional feature vectors, which play an important role in supervised learning. The goal is to reduce the size of the training data, resulting in lower storage consumption and computational complexity. Furthermore, the method can be regarded as a regularization technique, which improves the generalizability of learned target functions. We demonstrate significant improvements in comparison to the computation of data-driven predictions involving the full training data set. The method is applied to classification and regression problems from different application areas such as image recognition, system identification, and oceanographic time series analysis.
The dynamical behavior of social systems can be described by agent-based models. Although single agents follow easily explainable rules, complex time-evolving patterns emerge due to their interaction. The simulation and analysis of such agent-based models, however, is often prohibitively time-consuming if the number of agents is large. In this paper, we show how Koopman operator theory can be used to derive reduced models of agent-based systems using only simulation data. Our goal is to learn coarse-grained models and to represent the reduced dynamics by ordinary or stochastic differential equations. The new variables are, for instance, aggregated state variables of the agent-based model, modeling the collective behavior of larger groups or the entire population. Using benchmark problems with known coarse-grained models, we demonstrate that the obtained reduced systems are in good agreement with the analytical results, provided that the numbers of agents is sufficiently large.
Additional publications
- P. Gelß, SK, Z. Shakibaei, and S. Pokutta. Low-rank tensor decompositions of quantum circuits. Preprint, 2022. (arXiv)
- SK and N. Djurdjevac Conrad. Koopman-based spectral clustering of directed and time-evolving graphs. Preprint, 2022. (arXiv)
- H. Zhu, SK, and T. Sahai. A Dynamic Mode Decomposition Approach for Decentralized Spectral Clustering of Graphs. Proceedings of the IEEE CCTA 2022. (arXiv)
- SK, F. Nüske and S. Peitz. Koopman analysis of quantum systems. Journal of Physics A, 55(31): 314002, 2022. (arxiv) (JPhysA)
- M. Hoffmann, M. Scherer, T. Hempel, A. Mardt, B. de Silva, B. E. Husic, SK, H. Wu, N. Kutz, S. L. Brunton, and F. Noé. Deeptime: a Python library for machine learning dynamical models from time series data. Machine Learning: Science and Technology, 2021. (arXiv) (MLST)
- F. Nüske, P. Gelß, SK, and C. Clementi. Tensor-based computation of metastable and coherent sets. Physica D, 2021. (arXiv) (Physica D)
- SK, P. Gelß, F. Nüske, and F. Noé. Symmetric and antisymmetric kernels for machine learning problems in quantum physics and chemistry. Machine Learning: Science and Technology, 2021. (arXiv) (MLST)
- J.-H. Niemann, SK, and C. Schütte. Data-driven model reduction of agent-based systems using the Koopman generator. PLOS ONE, 2020. (arXiv) (PLOS ONE)
- A. Navarra, J. Tribbia, and SK. Estimation of Koopman Transfer Operators for the Equatorial Pacific SST. Journal of the Atmospheric Sciences, 2020. (JAS)
- P. Gelß, SK, I. Schuster, and C. Schütte. Feature space approximation for kernel-based supervised learning. Knowledge-Based Systems, 2020. (arXiv) (JKS)
- A. Bittracher, SK, B. Hamzi, P. Koltai, and C. Schütte. Dimensionality Reduction of Complex Metastable Systems via Kernel Embeddings of Transition Manifolds. Journal of Nonlinear Science, 2020. (arXiv) (JNLS)
- K. Melnyk, SK, G. Montavon, and T. Conrad. GraphKKE: Graph Kernel Koopman Embedding for Human Microbiome Analysis. Applied Network Science, 96:5, 2020. (arXiv) (ANS)
- I. Schuster, M. Mollenhauer, SK, and K. Muandet. Kernel Conditional Density Operators. Proceedings of the Twenty Third International Conference on Artificial Intelligence and Statistics, 108:993-1004, 2020. (arXiv) (PMLR)
- M. Mollenhauer, I. Schuster, SK, and C. Schütte. Singular Value Decomposition of Operators on Reproducing Kernel Hilbert Spaces. In O. Junge et al. Advances in Dynamics, Optimization and Computation. Springer, 2020. (arXiv) (Springer)
- M. Dellnitz, B. Gebken, R. Gerlach, and SK. On the Equivariance Properties of Self-adjoint Matrices. Dynamical Systems, 35(2):197-215, 2020. (arxiv) (DS)
- SK. Data-driven analysis of complex dynamical systems. Habilitation thesis, Freie Universität Berlin, 2020.
- SK, F. Nüske, and B. Hamzi. Kernel-based approximation of the Koopman generator and Schrödinger operator. Entropy, 22(7):722, 2020. (arXiv) (entropy)
- M. Mollenhauer, SK, C. Schütte, and P. Koltai. Kernel autocovariance operators of stationary processes: Estimation and convergence. Preprint, 2020. (arXiv)
- SK, F. Nüske, S. Peitz, J.-H. Niemann, C. Clementi, and C. Schütte. Data-driven approximation of the Koopman generator: Model reduction, system identification, and control. Physica D: Nonlinear Phenomena, 406:132416, 2020. (arXiv) (Physica D)
- S. Peitz and SK. Feedback control of nonlinear PDEs using data-efficient reduced order models based on the Koopman operator. In A. Mauroy, I. Mezic, and Y. Susuki. The Koopman Operator in Systems and Control: Concepts, Methodologies and Applications. Springer, 2020. (arXiv) (Springer)
- SK, I. Schuster, and K. Muandet. Eigendecompositions of Transfer Operators in Reproducing Kernel Hilbert Spaces. Journal of Nonlinear Science, 30(1):283-315, 2020. (arXiv) (JNLS)
- SK, B. E. Husic, M. Mollenhauer, and F. Noé. Kernel methods for detecting coherent structures in dynamical data. Chaos, 2019. (arXiv) (Chaos)
- W. Zhang, SK, T. Conrad, and C. Schütte. Learning Chemical Reaction Networks from Trajectory Data. SIAM Journal on Applied Dynamical Systems , 18(4):2000–2046, 2019. (arXiv) (SIADS)
- SK and P. Gelß. Tensor-based algorithms for image classification. Algorithms, 12(11):240, 2019. (arXiv) (Algorithms)
- T. Sahai, A. Ziessler, SK, and M. Dellnitz. Continuous Relaxations for the Traveling Salesman Problem. Nonlinear Dynamics, 97(4): 2003-2022, 2019. (arXiv) (Nonlinear Dynamics)
- S. Peitz and SK. Koopman operator-based model reduction for switched-system control of PDEs. Automatica, 106: 184-191, 2019. (arXiv) (automatica)
- P. Gelß, SK, J. Eisert, and C. Schütte. Multidimensional approximation of nonlinear dynamical systems. Journal of Computational and Nonlinear Dynamics, 14(6):061006, 2019. (arXiv) (CND)
- SK, A. Bittracher, I. Schuster, and C. Schütte. A kernel-based approach to molecular conformation analysis. The Journal of Chemical Physics, 149(24):244109, 2018. (arXiv) (J. Chem. Phys.)
- SK, P. Gelß, S. Peitz, and C. Schütte. Tensor-based dynamic mode decomposition. Nonlinearity, 2018. (arXiv) (nonlinearity)
- R. Banisch, Z. Trstanova, A. Bittracher, SK, and P. Koltai. Diffusion maps tailored to arbitrary non-degenerate Itô processes. Applied and Computational Harmonic Analysis, 2018. (arXiv) (ACHA)
- SK, S. Peitz, and I. Schuster. Analyzing high-dimensional time-series data using kernel transfer operator eigenfunctions. Preprint, 2018. (arXiv)
- SK, F. Nüske, P. Koltai, H. Wu, I. Kevrekidis, C. Schütte, and F. Noé. Data-driven model reduction and transfer operator approximation. Journal of Nonlinear Science, 28(3):985-1010, 2018. (arXiv) (JNLS)
- S. Hanke, S. Peitz, O. Wallscheid, SK, J. Böcker, and M. Dellnitz. Koopman Operator Based Finite-Set Model Predictive Control for Electrical Drives. Preprint, 2018. (arXiv)
- A. Bittracher, P. Koltai, SK, R. Banisch, M. Dellnitz, and C. Schütte. Transition manifolds of complex metastable systems: Theory and data-driven computation of effective dynamics. Journal of Nonlinear Science, 28(2):471-512, 2018. (arXiv) (JNLS)
- SK and T. Sahai. A spectral assignment approach for the graph isomorphism problem. Information and Inference: a Journal of the IMA, 7(4):689-706, 2018. (arXiv) (IMAIAI)
- S. Peleš and SK. Sparse automatic differentiation for large-scale computations using abstract elementary algebra. International Journal of Numerical Analysis and Modeling, 14(6):916-934, 2017. (arXiv) (IJNAM)
- P. Gelß, SK, S. Matera, and C. Schütte. Nearest-Neighbor Interaction Systems in the Tensor Train Format. Journal of Computational Physics, 341:140-162, 2017. (arXiv) (J. Comp. Phys.)
- H. Wu, F. Nüske, F. Paul, SK, P. Koltai, and F. Noé. Variational Koopman models: Slow collective variables and molecular kinetics from short off-equilibrium simulations. The Journal of Chemical Physics, 146(15):154104, 2017. (arXiv) (J. Chem. Phys.)
- M. Dellnitz and SK. Sensing and Control in Symmetric Networks. Dynamical Systems, 32(1):61-79, 2017. (arXiv) (DS)
- M. Dellnitz, SK, and A. Ziessler. A Set-Oriented Numerical Approach for Dynamical Systems with Parameter Uncertainty. SIAM Journal on Applied Dynamical Systems, 16(1):120-138, 2016. (arXiv) (SIADS) (DSWEB)
- SK and C. Schütte. Towards tensor-based methods for the numerical approximation of the Perron-Frobenius and Koopman operator. Journal of Computational Dynamics, 3(2):139-161, 2016. (arXiv) (JCD)
- SK, P. Koltai, and C. Schütte. On the numerical approximation of the Perron-Frobenius and Koopman operator. Journal of Computational Dynamics, 3(1):51-79, 2016. (arXiv) (JCD)
- SK. Signal-flow based Runge-Kutta methods for the simulation of complex networks. ArXiv e-prints, 2015.
- SK. Signal-Flow Based Circuit Simulation. PhD thesis, Institute for Industrial Mathematics, Paderborn University, 2011.
- SK, T. Sahai, C. Liu, and M. Dellnitz. An efficient algorithm for the parallel solution of high-dimensional differential equations. Journal of Computational and Applied Mathematics, 235(9):3053-3062, 2011. (arXiv) (JCAM)
- C. Lakemeyer, SK, K. Anger, H. Hörmann, and V. Schöppner. Temperature modelling of the melt for tempered screws in single screw extrusion by giving constant heat fluxes. In Proceedings of the Polymer Processing Society, Istanbul, Turkey, 2010.
- H. Potente, V. Schöppner, SK, C. Anger, and H. Hörmann. Temperature modelling of the melt for tempered screws in single screw extrusion. In Proceedings of the 25th Annual Meeting of the Polymer Processing Society, Goa, India, 2009.
- H. Potente, M. Kurte-Jardin, K. Timmermann, and SK. Temperature development of wall-slipping melts. In Proceedings of the 22nd Annual Meeting of the Polymer Processing Society, Yamagata, Japan, 2006.
- H. Potente, M. Kurte-Jardin, SK, and K. Timmermann. Two dimensional description of pressure-throughput behaviour of Newtonian materials considering wall slippage effects. International Polymer Processing, 20(3):312-321, 2005.