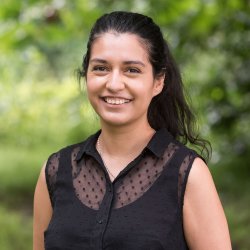
Dr Thalia Rodriguez Garcia
Academic and research departments
Mathematics at the Interface Group, Surrey Sleep Research Centre, School of Biosciences.Publications
Background More than 70% of people living with dementia (PLWD) experience sleep disturbances (e.g., early bedtime, long time in bed) even in the early stages of cognitive decline. Light interventions have been proposed as a promising non‐pharmacological approach to improve sleep timing, but current implementations are burdensome and not personalised. Quantitative tools for designing pragmatic light interventions for the home‐setting could benefit PLWD and their carers. Here, we report on a quantitative computational tool which combines readily collectable data with a new mathematical model to provide personalised advice on light interventions. Our focus is on designing low‐burden interventions requiring minimal changes to lifestyle or sleep‐wake routines. Method Eighteen older adults (65‐80 y) monitored their light exposure and sleep for a period of 7‐10 days at home using a wristworn monitor (Actiwatch Spectrum) and a sleep diary from which we calculated daily sleep timing and duration. We constructed a new mechanistic mathematical model for sleep timing that incorporates sleep homeostasis, circadian rhythmicity and light data (HCL). For each participant, we fitted the model to their sleep timing and duration data. We then used simulations to predict the effect of different light interventions on sleep timing and used optimisation to determine light exposure patterns that promote a target sleep onset time. Result Individuals varied in their sleep duration (07:18 ± 0:56 hh:mm; mean ± SD) and mid‐sleep timing (03:22 ± 01:00 hh:mm). We successfully retrieved ‘personal’ model parameters that accurately captured sleep duration and timing for 17 of the 18 participants. Our simulations indicated that the effect of a given light exposure pattern on sleep timing depends on the personalised model parameters, suggesting that an intervention that works for one person may not work for someone else. We were able to propose light exposure patterns requiring minimal behavioural change, facilitating adherence. Conclusion It is possible to extract individual physiological parameters for sleep‐wake regulation from data collected in the field. These individual parameters can be used to design personalised light interventions. Our fully‐documented code could be combined with passive sleep monitors and environmental light sensors to unobtrusively recommend personalised light exposure patterns in close‐to real time.
Sleep timing varies between individuals and can be altered in mental and physical health conditions. Sleep and circadian sleep phenotypes, including circadian rhythm sleep-wake disorders, may be driven by endogenous physiological processes, exogeneous environmental light exposure along with social constraints and behavioural factors. Identifying the relative contributions of these driving factors to different phenotypes is essential for the design of personalised interventions. The timing of the human sleep-wake cycle has been modelled as an interaction of a relaxation oscillator (the sleep homeostat), a stable limit cycle oscillator with a near 24-hour period (the circadian process), man-made light exposure and the natural light-dark cycle generated by the Earth's rotation. However, these models have rarely been used to quantitatively describe sleep at the individual level. Here, we present a new Homeostatic-Circadian-Light model (HCL) which is simpler, more transparent and more computationally efficient than other available models and is designed to run using longitudinal sleep and light exposure data from wearable sensors. We carry out a systematic sensitivity analysis for all model parameters and discuss parameter identifiability. We demonstrate that individual sleep phenotypes in each of 34 older participants (65-83y) can be described by feeding individual participant light exposure patterns into the model and fitting two parameters that capture individual average sleep duration and timing. The fitted parameters describe endogenous drivers of sleep phenotypes. We then quantify exogenous drivers using a novel metric which encodes the circadian phase dependence of the response to light. Combining endogenous and exogeneous drivers better explains individual mean mid-sleep (adjusted R-squared 0.64) than either driver on its own (adjusted R-squared 0.08 and 0.17 respectively). Critically, our model and analysis highlights that different people exhibiting the same sleep phenotype may have different driving factors and opens the door to personalised interventions to regularize sleep-wake timing that are readily implementable with current digital health technology.