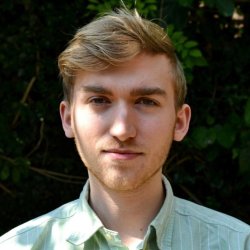
Tavis Shore
Academic and research departments
Centre for Vision, Speech and Signal Processing (CVSSP), Faculty of Engineering and Physical Sciences.About
My research project
AI Navigation in GNSS-denied EnvironmentsDeveloping novel AI localisation techniques for EW-immune navigation in GNSS-denied regions.
Supervisors
Developing novel AI localisation techniques for EW-immune navigation in GNSS-denied regions.
My qualifications
Affiliations and memberships
Business, industry and community links
Publications
Cross-view image matching for geo-localisation is a challenging problem due to the significant visual difference between aerial and ground-level viewpoints. The method provides localisation capabilities from geo-referenced images, eliminating the need for external devices or costly equipment. This enhances the capacity of agents to autonomously determine their position, navigate, and operate effectively in GNSS-denied environments. Current research employs a variety of techniques to reduce the domain gap such as applying polar transforms to aerial images or synthesising between perspectives. However, these approaches generally rely on having a 360 degree field of view, limiting real-world feasibility. We propose BEV-CV, an approach introducing two key novelties with a focus on improving the real-world viability of cross-view geo-localisation. Firstly bringing ground-level images into a semantic Birds-Eye-View before matching embeddings, allowing for direct comparison with aerial image representations. Secondly, we adapt datasets into application realistic format-limited-FOV images aligned to vehicle direction. BEV-CV achieves state-of-the-art recall accuracies, improving Top-1 rates of 70 degree crops of CVUSA and CVACT by 23% and 24% respectively. Also decreasing computational requirements by reducing floating point operations to below previous works, and decreasing embedding dimensionality by 33%-together allowing for faster localisation capabilities.
Cross-view Geo-localisation is typically performed at a coarse granularity, because densely sampled satellite image patches overlap heavily. This heavy overlap would make dis-ambiguating patches very challenging. However, by opting for sparsely sampled patches, prior work has placed an artificial upper bound on the localisation accuracy that is possible. Even a perfect oracle system cannot achieve accuracy greater than the average separation of the tiles. To solve this limitation, we propose combining cross-view geo-localisation and relative pose estimation to increase precision to a level practical for real-world application. We develop PEnG, a 2-stage system which first predicts the most likely edges from a city-scale graph representation upon which a query image lies. It then performs relative pose estimation within these edges to determine a precise position. PEnG presents the first technique to utilise both viewpoints available within cross-view geo-localisation datasets, referring to this as Multi-View Geo-Localisation (MVGL). This enhances accuracy to a sub-metre level, with some examples achieving centimetre level precision. Our proposed ensemble achieves state-of-the-art accuracy-with relative Top-5m retrieval improvements on previous works of 213%. Decreasing the median Euclidean distance error by 96.90% from the previous best of 734m down to 22.77m, when evaluating with 90° horizontal FOV images. Code is available here: github.com/tavisshore/peng.
Cross-View Geo-Localisation within urban regions is challenging in part due to the lack of geo-spatial struc-turing within current datasets and techniques. We propose utilising graph representations to model sequences of local observations and the connectivity of the target location. Modelling as a graph enables generating previously unseen sequences by sampling with new parameter configurations. To leverage this newly available information , we propose a GNN-based architecture, producing spatially strong embeddings and improving discriminabil-ity over isolated image embeddings. We outline SpaG-BOL, introducing three novel contributions. 1) The first graph-structured dataset for Cross-View Geo-Localisation, containing multiple streetview images per node to improve generalisation. 2) Introducing GNNs to the problem, we develop the first system that exploits the correlation between node proximity and feature similarity. 3) Lever-aging the unique properties of the graph representation-we demonstrate a novel retrieval filtering approach based on neighbourhood bearings. SpaGBOL achieves state-of-the-art accuracies on the unseen test graph-with relative Top-1 retrieval improvements on previous techniques of 11%, and 50% when filtering with Bearing Vector Matching on the SpaGBOL dataset. Code and dataset available: github.com/tavisshore/SpaGBOL.
Low Power Wide Area Networks (LPWANs) are a subset of IoT transmission technologies that have gained traction in recent years with the number of such devices exceeding 200 million. This paper considers the scalability of one such LPWAN, LoRaWAN, as the number of devices in a network increases. Various existing optimisation techniques target LoRa characteristics such as collision rate, fairness, and power consumption. This paper proposes a machine learning ensemble to reduce the total distance between devices and improve the average received signal strength, resulting in improved network throughput, the scalability of LoRaWAN, and the cost of networks. The ensemble consists of a constrained K-Means clustering algorithm, a regression model to validate new gateway locations and a Neural network to estimate signal strength based on the location of the devices. Results show a mean distance reduction of 51% with an RSSI improvement of 3% when maintaining the number of gateways, also achieving a distance reduction of 27% and predicting an RSSI increase of 1% after clustering with 50% of the number of gateways.