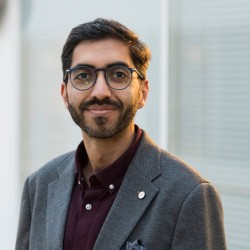
Dr Tanmoy Chatterjee
About
Biography
Dr Tanmoy Chatterjee is a Lecturer in Resilient Design in the School of Mechanical Engineering Sciences (Fall 2022 onwards) and a Fellow of the Surrey Institute for People-Centred AI and the Institute for Sustainability.
Previous to joining the University of Surrey, he was a postdoctoral researcher in the Aerospace Structures Research Group at Swansea University (2018-22) and was funded by the £5M EPSRC programme grant 'DigiTwin: Digital Twins for Improved Dynamic Design.'
Although, his primary research at Swansea involved model reduction, dynamic sub-structuring, uncertainty quantification and data-driven physics discovery of built-up/jointed structures, he also actively collaborated towards investigating the effect of manufacturing variability on the dynamic behaviour and wave propagation of 2D lattices/metamaterials.
He completed his PhD on developing computational meta-models for risk/reliability analysis and robust design optimization of structural systems from the Department of Civil Engineering at the Indian Institute of Technology Roorkee.
He has received awards/scholarships from the Council of Scientific and Industrial Research (CSIR) and Ministry of Education, Government of India for pursuing his master's (2011-13) and PhD (2014-18). Tanmoy has two years of industrial experience and has worked in the structural design (2013-14) and construction sectors (2010-11) before choosing an academic career.
News
In the media
ResearchResearch interests
Algorithmic development
- Stochastic modelling
- Risk/reliability analysis
- Robust and reliability based optimal design
- Machine learning based meta-modelling
- Model-order reduction and domain decomposition
- Data-driven adaptive sparse Bayesian digital twins.
Engineering applications
- Structural mechanics (civil, mechanical, aerospace)
- Linear and non-linear dynamics of built-up/jointed structures (civil, mechanical, aerospace and electro-mechanical)
- Wave propagation of 2D lattices/metamaterials
- Extreme metamaterial microstructural topological design
- Quasi zero stiffness vibration absorber and nonlinear energy sink
- Vibration based piezoelectric energy harvesting
- Offshore jacket platforms (considering pile-soil interaction).
Research projects
Connected Everything II Future Prize Fund 2023 (Co-I)
Research interests
Algorithmic development
- Stochastic modelling
- Risk/reliability analysis
- Robust and reliability based optimal design
- Machine learning based meta-modelling
- Model-order reduction and domain decomposition
- Data-driven adaptive sparse Bayesian digital twins.
Engineering applications
- Structural mechanics (civil, mechanical, aerospace)
- Linear and non-linear dynamics of built-up/jointed structures (civil, mechanical, aerospace and electro-mechanical)
- Wave propagation of 2D lattices/metamaterials
- Extreme metamaterial microstructural topological design
- Quasi zero stiffness vibration absorber and nonlinear energy sink
- Vibration based piezoelectric energy harvesting
- Offshore jacket platforms (considering pile-soil interaction).
Research projects
Connected Everything II Future Prize Fund 2023 (Co-I)
Supervision
Postgraduate research supervision
Wonderful opportunity to join my group and collaborate with world-leading academics and industrial partners to become a part of exciting multidisciplinary research with a focus to solving real-life challenging engineering problems!
Do check out the following links of current PhD openings (application closing date: 03 April 2024, PhD start date: October 2024):
- Design of multifunctional devices for simultaneous sensing, monitoring and energy harvesting: Towards the implementation of artificial intelligence of things (AIoT) (Engineering Materials PhD)
- Developing a sentient digital twin for safe and resilient robotic systems (Automotive Engineering PhD)
- Resilient biomimetic flight in cluttered environments (Aerodynamic and Environmental Flow PhD).
Some more good news: Open to any UK or international candidates. UKRI standard stipend (currently £18,622 p.a.) with an additional bursary of £1,700 p.a. (for the full 3.5 years) for exceptional candidates. Full home or O/S fees (as applicable) covered. A research, training and support grant of £3,000 over the project is offered. Up to 30% of our UKRI funded studentships can be awarded to candidates paying international rate fees.
The links include all the other necessary information of the programme, such as entry requirements, project details. Feel free to contact in case you need additional clarifications before or after applying. All the best!
Postgraduate research supervision
Co-supervisor of PhD scholar James Smith (2022-25) at the School of Mechanical Engineering Sciences, University of Surrey in collaboration with Autodesk (ongoing).
Co-supervisor of EPSRC-DTP ADDED PhD scholar Pushpa Pandey (2026408) of Swansea University (2020-23) in collaboration with UK Atomic Energy Authority (completed).
Teaching
Solid Mechanics 1 (ENG1066): 1st year, Mechanical, Aerospace, Automotive and Biomedical Engineering, University of Surrey (2023-24).
Vehicle Structures and Analysis (ENGM267): Master's level, Mechanical Engineering, University of Surrey (2023-24, 2022-23).
Dynamics 2 (EG-360): 3rd year Mechanical and Aerospace Engineering, Swansea University (2021-22).
Sustainable development goals
My research interests are related to the following:
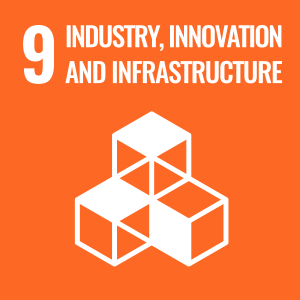
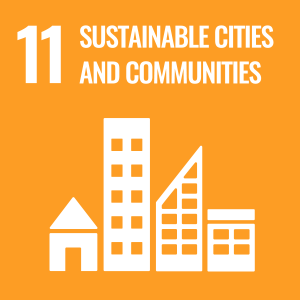
Publications
This study focuses on stochastic modelling of 2D aeroelastic model to quantify the uncertainties in the parameters of aeroelastic models, specifically the plunging and torsional stiffness. To measure the frequency response, a simulation of an aeroelastic model in a wind tunnel is considered. The excitation is provided by Gust. The frequency-velocity curve is generated from the simulated dynamic aeroelastic response. This curve is then used to establish the likelihood function for Bayesian identification of the aeroelastic model. The Metropolis–Hastings algorithm in Markov-Chain Monte Carlo (MCMC) sampling is used to analyze the measurement data obtained from the frequency-velocity curves. Using Bayesian inference, the uncertainties in the aeroelastic model are modelled. Overall, this study provides insights into the identification of uncertainties in aeroelastic models using advanced statistical techniques.
Parametric excitation is introduced to amplify the external harmonic excitation and extend the capabilities of a nonlinear piezoelectric energy harvester device. To investigate the efficiency of the parametrically amplified energy harvester, the time responses of the voltage and power are computed using the state space formulation. It is assumed that the proposed nonlinear model consists of cubic and quadratic nonlinearities, where parametric amplification appears in the form of a trigonometric function. The frequency is tuned as the one-to-one ratio between the external and parametric excitations. An optimal configuration of the nonlinear system is illustrated to achieve the maximum possible harvested energy. Next, the effect of parametric amplification on the harvested power is investigated considering system uncertainties. The complex nonlinear relationship is mapped with the help of a machine learning technique, namely a Gaussian process. Once these techniques are trained using a small number of samples, they emulate the stochastic nonlinear dynamical system without having to solve the actual governing equations. The results obtained by the proposed methodology are verified with results from direct numerical integration combined with Monte Carlo simulation.
Stochastic model updating of joints is a common solution for realistic modelling of built-up structures. However, detailed large-scale models render the simulations to be computationally expensive. This issue is addressed in the following two ways. The first approach involves stochastic decomposition of the functional space by machine learning techniques such as Gaussian processes, artificial neural networks, generalized radial basis neural networks and support vector machines. The second approach comprises a more physics-based strategy which involves reducing the internal degrees of freedom of the sub-components using the fixed-interface component mode synthesis method. The assembly problem is performed on two beam structures connected by a bolted lap joint. Modal testing is performed on nominally identical beam structures to account for manufacturing variability. The results obtained have been validated and the good performance achieved by the proposed reduced models demonstrates their robustness.
Robust design optimization (RDO) has been eminent, ascertaining optimal configuration of engineering systems in presence of uncertainties. However, computational aspect of conventional RDO can often get computationally intensive as neighborhood assessments of every solution are required to compute the performance variance and ensure feasibility. Surrogate assisted optimization is one of the efficient approaches in order to mitigate this issue of computational expense. However, the performance of a surrogate model plays a key factor in determining the optima in multi-modal and highly non-linear landscapes, in presence of uncertainties. In other words, the approximation accuracy of the model is principal in yielding the actual optima and thus, avoiding any misguide to the decision maker on the basis of false or, local optimum points. Therefore, an extensive survey has been carried out by employing most of the well-known surrogate models in the framework of RDO. It is worth mentioning that the numerical study has revealed consistent performance of a model out of all the surrogates utilized. Finally, the best performing model has been utilized in solving a large-scale practical RDO problem. All the results have been compared with that of Monte Carlo simulation results.
The increasing complexity of real-time problems has posed a perpetual challenge to existing simulation models. In particular, such models governing any physical system usually entail long hours of simulation, making them computationally intensive for large-scale problems. In order to mitigate this issue, a novel computational tool has been developed for efficient stochastic computations. The proposed model has been developed by two-tier improvement of the existing approximation techniques. As the first improvement, Kriging (Dubourg et al., in Probab Eng Mech 33: 47–57, 2013, [1]) has been incorporated within high-dimensional model representation (HDMR) (Li et al., in J Phys Chem A 110:2474–2485, 2006, [2]) model so as to enhance approximation capabilities in terms of accuracy. Second, least absolute shrinkage and selection operator (LASSO) (Tibshirani, in 1996, J. R. Stat. Soc. B 58:267–288, [3]) has been utilized and integrated with the refined model so as to construct a sparse HDMR approximation, eventually leading to reduced computational complexity. Implementation of the proposed approach has been demonstrated with two analytical benchmark examples and a 25-element space truss problem.
Moment free sensitivity analysis computes importance of input parameters by taking into account the entire probability distribution of the output response. Due to improvement in the framework of moment free sensitivity analysis, it is widely preferred over other approaches. However, the framework often becomes computationally intensive especially in large-scale systems involving finite-element simulation. In order to minimize the computational effort, two h-p adaptive meta-models have been proposed for replacing the expensive actual response evaluations. The proposed meta-models incorporate a three-layered advantage over the conventional ones, which are, global refinement of the basis functions, enabled with compressive sampling based methods based on l(0)-and l(1)-norms (p-adaptivity) and integrated with an optimal sequential experimental design scheme (h-adaptivity). Both of the proposed models have been merged efficiently in the framework of moment free sensitivity analysis. Five numerical examples have been carried out for accessing the performance of the proposed models. Finally, a real-time engineering structure has been modelled and sensitivity analysis is performed. Significant reduction in computational effort has been achieved along with good level of accuracy by utilizing the proposed meta-model assisted sensitivity tools. The results have been validated with that of Monte Carlo simulation. (C) 2018 Elsevier B.V. All rights reserved.
The computational intensiveness inherently associated with uncertainty quantification of engineering systems has been one of the prime concerns over the years. In order to mitigate this issue, two novel approaches have been developed for efficient stochastic computations. Both the approaches have been developed by amalgamating the advantages of two available techniques namely, high dimensional model representation (HDMR) and Kriging. These two methods are coupled in such a way that HDMR addresses the global variation in the functional space using a set of component functions and the fine aberrations are interpolated by utilizing Kriging, performing as a two level approximation. A Bayesian learning framework has been integrated with the locally refined model so as to construct a sparse configuration. In order to earn additional computational efficiency, an accelerated training algorithm for the sparse Bayesian learning framework has been fused within the refined model. Implementation of the proposed approaches have been demonstrated with two analytical problems and two large scale practical offshore structural problems. The efficiency and accuracy of the proposed approaches in stochastic response analysis have been assessed by comparison with Monte Carlo simulation. Excellent results in terms of accuracy and computational effort obtained makes the proposed methodologies potential for further complex applications.
The computational intensiveness inherently associated with uncertainty quantification of engineering systems has been one of the prime concerns over the years. In order to mitigate this issue, a novel approach has been developed for efficient stochastic computations. The proposed approach has been developed by amalgamating the advantages of two available techniques namely, high dimensional model representation (HDMR) and Kriging. These two methods are coupled in such a way that HDMR addresses the global variation in the functional space using a set of component functions and the fine aberrations are interpolated by utilizing Kriging, performing as a two level approximation. A Bayesian learning framework has been integrated with the locally refined model so as to construct a sparse configuration. Implementation of the proposed approach has been demonstrated with five benchmark problems and a practical offshore structural problem. The efficiency and accuracy of the proposed approach in stochastic response analysis have been assessed by comparison with Monte Carlo simulation. Excellent results in terms of accuracy and computational effort obtained makes the proposed methodology potential for further complex applications. (C) 2017 Elsevier Ltd. All rights reserved.
Optimization for crashworthiness is of vast importance in automobile industry. Recent advancement in computational prowess has enabled researchers and design engineers to address vehicle crashworthiness resulting in reduction of cost and time for new product development. However a deterministic optimum design often resides at the boundary of failure domain leaving little or no room for modeling imperfections parameter uncertainties and/or human error. In this study an operational model-based robust design optimization (RDO) scheme has been developed for designing crashworthiness of vehicle against side impact. Within this framework differential evolution algorithm (DEA) has been coupled with polynomial correlated function expansion (PCFE). An adaptive framework for determining the optimum basis order in PCFE has also been presented. It is argued that the coupled DEA-PCFE is more efficient and accurate as compared to conventional techniques. For RDO of vehicle against side impact minimization of the weight and lower rib deflection of the vehicle are considered to be the primary design objectives. Case studies by providing various emphases on the two objectives have also been performed. For all the cases DEA-PCFE is found to yield highly accurate results.
Robust design optimization (RDO) is a field of optimization in which certain measure of robustness is sought against uncertainty. Unlike conventional optimization, the number of function evaluations in RDO is significantly more which often renders it time consuming and computationally cumbersome. This paper presents two new methods for solving the RDO problems. The proposed methods couple differential evolution algorithm (DEA) with polynomial correlated function expansion (PCFE). While DEA is utilized for solving the optimization problem, PCFE is utilized for calculating the statistical moments. Three examples have been presented to illustrate the performance of the proposed approaches. Results obtained indicate that the proposed approaches provide accurate and computationally efficient estimates of the RDO problems. Moreover, the proposed approaches outperforms popular RDO techniques such as tensor product quadrature, Taylor's series and Kriging. Finally, the proposed approaches have been utilized for robust hydroelectric flow optimization, demonstrating its capability in solving large scale problems. (C) 2017 Elsevier Inc. All rights reserved.
The computational intensiveness of evolutionary algorithms (EAs) in dealing with large-scale problems has been of great concern over the years. In order to reduce the computational burden associated with EAs initially, an anchored ANOVA decomposition model was integrated with the elitist nondominated sorting genetic algorithm (NSGA-II). Later, an improved version of the former framework, a novel approximation technique was developed and specialized to solve typical case scenarios often encountered, such as problems with nonconvex and disconnected Pareto optimal fronts. This proposed tool has been referred to as adaptive bilevel error-sensitivity based anchored ANOVA decomposition (ABE-ANOVA). ABE-ANOVA has been developed by the amalgamation of anchored ANOVA decomposition and Gaussian process (GP) model. They are coupled in such a way that anchored ANOVA handles the global behavior of the model using a set of component functions and GP interpolates local variations as a function of sample points, resulting in a two-level approximation. The study proves that ABE-ANOVA assisted NSGA-II is much more robust as compared to ANOVA assisted NSGA-II, especially in handling complex scenarios. Implementation of the proposed approaches have been demonstrated with the help of several multiobjective analytical examples and a few finite element problems. Performance has been assessed by comparing simulation of the actual model with NSGA-II and a few other algorithms. Excellent results in terms of accuracy and computational effort makes the proposed method potential for real-time applications. (C) 2016 American Society of Civil Engineers.
Frequency response functions (FRFs) are considered to be a significant aspect in the evaluation of structural response subjected to dynamic loading. A new approach, referred to as the hybrid polynomial correlated function expansion (H-PCFE) has been developed for predicting the natural frequencies and the FRF of stochastic dynamical systems. H-PCFE has been developed by incorporating the advantages of two available techniques namely, PCFE and Gaussian process (GP) modeling. These two methods are coupled in such a way that PCFE handles the global behavior of the model using a set of component functions and GP interpolates local variations as a function of the sample points, performing as a two level approximation. Implementation of the proposed approach for stochastic dynamic problems has been demonstrated with four problems. The main focus of this study lies in the prediction of FRFs. The efficiency and accuracy of H-PCFE to compute FRFs of stochastic dynamic systems is assessed by a comparison with direct Monte Carlo simulation (MCS). Excellent results in terms of accuracy and computational effort obtained makes the proposed methodology potential for application in large scale structural applications. (C) 2015 Elsevier Ltd. All rights reserved.
The effective performance of two passive vibration mitigating devices namely tuned liquid column damper (TLCD) and tuned liquid column ball damper (TLCBD) are studied for control of wave induced vibration. The stochastic dynamic analysis of structure with and without damper is first presented in frequency domain for parametric study on the performance of both the dampers to control wave induced vibration response. The optimum performance of TLCD and TLCBD systems is further investigated to study the effectiveness of a particular damper system over the other. In order to obtain optimal damper parameters to yield the best possible TLCD and TLCBD performance under a given condition, optimization is performed by minimizing the root mean square displacement of the structure. Numerical study is taken up to explore the effectiveness of both the TLCD and TLCBD. Though there is a wide gap between structural frequency and frequency of wave load, the performances of both the dampers are found to be potential for wave vibration mitigation. The optimum performance study in general shows that TLCBD perform better than TLCD. The performances of both the damper system are further verified in time domain under random wave load. (C) 2014 Elsevier Ltd. All rights reserved.
The study presents a novel approach for stochastic nonlinear model updating in structural dynamics, employing a Bayesian framework integrated with Markov Chain Monte Carlo (MCMC) sampling for parameter estimation by using an approximated likelihood function. The proposed methodology is applied to both numerical and experimental cases. The paper commences by introducing Bayesian inference and its constituents: the likelihood function, prior distribution, and posterior distribution. The resonant decay method is employed to extract backbone curves, which capture the non-linear behaviour of the system. A mathematical model based on a single degree of freedom (SDOF) system is formulated, and backbone curves are obtained from time response data. Subsequently, MCMC sampling is employed to estimate the parameters using both numerical and experimental data. The obtained results demonstrate the convergence of the Markov chain, present parameter trace plots, and provide estimates of posterior distributions of updated parameters along with their uncertainties. Experimental validation is performed on a cantilever beam system equipped with permanent magnets and electromagnets. The proposed methodology demonstrates promising results in estimating parameters of stochastic non-linear dynamical systems. Through the use of the proposed likelihood functions using backbone curves, the probability distributions of both linear and non-linear parameters are simultaneously identified. Based on this view, the necessity to segregate stochastic linear and non-linear model updating is eliminated. •A novel likelihood function for stochastic nonlinear model updating is proposed.•The method incorporates the backbone curve in likelihood function.•Bayesian-MCMC for parameter estimation in nonlinear dynamic systems is used.•The application of the method is shown numerically and experimentally.•Enhanced MCMC sampling demonstrated robust parameter distribution.
We demonstrate that the consideration of material uncertainty can dramatically impact the optimal topological micro-structural configuration of mechanical metamaterials. The robust optimization problem is formulated in such a way that it facilitates the emergence of extreme mechanical properties of metamaterials. The algorithm is based on the bi-directional evolutionary topology optimization and energy-based homogenization approach. To simulate additive manufacturing uncertainty, combinations of spatial variation of the elastic modulus and/or, parametric variation of the Poisson’s ratio at the unit cell level are considered. Computationally parallel Monte Carlo simulations are performed to quantify the effect of input material uncertainty to the mechanical properties of interest. Results are shown for four configurations of extreme mechanical properties: (1) maximum bulk modulus (2) maximum shear modulus (3) minimum negative Poisson’s ratio (auxetic metamaterial) and (4) maximum equivalent elastic modulus. The study illustrates the importance of considering uncertainty for topology optimization of metamaterials with extreme mechanical performance. The results reveal that robust design leads to improvement in terms of (1) optimal mean performance (2) least sensitive design, and (3) elastic properties of the metamaterials compared to the corresponding deterministic design. Many interesting topological patterns have been obtained for guiding the extreme material robust design.
Data-driven discovery of governing laws for complex nonlinear structural dynamic systems remains a challenging issue of paramount importance. This work addresses the above issue by leveraging the available noisy data and integrating sparse Bayesian machine learning (ML) techniques to discover the governing equations. The problem of discovery is re-cast as the automatic relevance determination of models (model selection) from the library of potential candidate basis terms and their coefficients are determined (parameter identification) using sparse Bayesian linear regression. Two sparsity promoting ML algorithms based on relevance vector machines have been employed. Both these approaches use Bayesian statistics and quantify the uncertainty associated with the model predictions. Results from four representative numerical examples of nonlinear structural dynamics illustrate excellent performance of both proposed approaches. The results have been validated with the true governing equations and time response data. Comparison has also been made with a recent and popular sparse discovery approach. Finally, the proposed framework is applied to real datasets that were generated from an in-house designed experimental setup of a quasi zero stiffness device and good performance has been observed.
Robust design optimization (RDO) has been eminent in determining the optimal design of real-time complex systems under stochastic environment. Unlike conventional optimization, RDO involves uncertainty quantification which may render the procedure to be computationally intensive, if not prohibitive. In order to deal with such issues, an efficient approximation-based generalized RDO framework has been proposed. Since RDO formulation comprises of statistical terms of the performance functions, the proposed framework deals with approximation of those statistical quantities, rather than the performance functions. Consequently, the proposed framework allows transformation of the RDO problem to an equivalent deterministic one. As a result, unlike traditional surrogate-assisted RDO, the proposed framework yields desirable results in significantly less number of functional evaluations. For performing such response statistical approximation, two adaptive sparse refined Kriging-based computational models have been proposed. However, the generality of the proposed methodology allows any surrogate models to be employed within this framework, provided it is capable of capturing the functional non-linearity. Implementation of the proposed framework in three test examples and two finite element-based practical problems clearly illustrates its potential for further complicated applications.
Inerter-based periodic structures have attracted significant interest among the research community due to their wide range of applications. However, existing studies on quasiperiodic structures, especially with inerters, are limited. Therefore, this timely work investigates the dynamics of novel one-dimensional inerter-based quasiperiodic lattices with and without local resonators. The quasiperiodicity is introduced through the modulation of both spring and inerter properties resulting in the well-known Hofstadter-like butterfly spectrum having a fractal structure. Moreover, by considering the appropriate boundary conditions and many mass- spring-inerter subsystems, the bulk spectrum of the system is investigated demonstrating the existence of multiple edge states in lower and higher frequency ranges. The deterministic study showed that the effect of inertia amplification is to reduce the frequencies of the Hofstadter-like butterfly and to widen the low frequency band gaps. The second contribution of this work involves investigating the effect of parametric uncertainty for the acoustic-metamaterial-like chain based on Monte Carlo simulations. Moreover, to address the computational aspect of the problem, grey-box modeling using machine learning was performed. A Gaussian process model was trained on a limited dataset and was found to capture the stochastic responses of the lattices adequately. The statistical variation of parameters with different levels of uncertainty demonstrated significant effects on the Hofstadter-like butterfly, band gaps, corresponding edge states and frequency responses. The sensitivity of the dynamic behavior in quasiperiodic lattices to variabilities reveal the need to account for system uncertainties for their targeted performance as future vibration absorbers and energy harvesters. The study also paves the way to utilize the results as useful prior information for robust design optimization of real inerter-based quasiperiodic lattice devices.
This paper characterizes the stochastic dynamic response of periodic structures by accounting for manufacturing variabilities. Manufacturing variabilities are simulated through a probabilistic description of the structural material and geometric properties. The underlying uncertainty propagation problem has been efficiently carried out by func-tional decomposition in the stochastic space with the help of Gaussian Process (GP) meta modelling. The decomposition is performed by projected the response onto the eigenspace and involves a nominal number of actual physics-based function evaluations (the eigenvalue analysis). This allows the stochastic dynamic response evaluation to be solved with low computational cost. Two numerical examples, namely an analytical model of a damped mechanical chain and a finite-element model of multiple beam-mass systems, are undertaken. Two key findings from the results are that the proposed GP based approximation scheme is capable of (i) capturing the stochastic dynamic response in systems with well separated modes in the presence of high levels of uncertainties (up to 20%), and (ii) adequately capturing the stochastic dynamic response in systems with multiple sets of identical modes in the presence of 5-10% uncertainty. The results are validated by Monte Carlo simulations. (c) 2020 Elsevier Ltd. All rights reserved.
Reliability analysis of highly sensitive structures is crucial to prevent catastrophic failures and ensure safety. Therefore, these safety-critical systems are to be designed for extremely rare failure events. Accurate statistical quantification of these events associated with a very low probability of occurrence requires millions of evaluations of the limit state function (LSF) involving computationally expensive numerical simulations. Variance reduction techniques like importance sampling (IS) reduce such repetitions to a few thousand. The use of a data-centric metamodel can further cut it down to a few hundred. In data-centric metamodeling approaches, the actual complex numerical analysis is performed at a few points to train the metamodel for approximating the structural response. On the other hand, a physics-informed neural network (PINN) can predict the structural response based on the governing differential equation describing the physics of the problem, without a single evaluation of the complex numerical solver, i.e., data-free. However, the existing PINN models for reliability analysis have been effective only in estimating a large range of failure probabilities (10−1∼10−3). To address this issue, the present study develops a PINN-based data-free reliability analysis for low failure probabilities (
This paper addresses computational aspects in dynamic sub-structuring of built-up structures with uncertainty. Component mode synthesis (CMS), which is a model reduction technique, has been integrated within the framework of domain decomposition (DD), so that reduced models of individual sub-systems can be solved with smaller computational cost compared to solving the full (unreduced) system by DD. This is particularly relevant for structural dynamics applications where the overall system physics can be captured by a relatively low number of modes. The theoretical framework of the proposed methodology has been extended for application in stochastic dynamic systems. To limit the number of eigen-value analyses to be performed corresponding to the random realizations of input parameters, a locally refined high dimensional model representation model with stepwise least squares regression is presented. Effectively, a bi-level decomposition is proposed, one in the physical space and the other in the stochastic space. The geometric decomposition in the physical space by the proposed model reduction-based DD reduces the computational cost of a single analysis of the system and the functional decomposition in the stochastic space by the proposed meta-model lowers the number of simulations to be performed on the actual system. The results achieved by solving a finite-element model of an assembled beam structure and a 3D space frame illustrate good performance of the proposed methodology, highlighting its potential for complex problems. (C) 2020 Elsevier B.V. All rights reserved.
This paper addresses the influence of manufacturing variability of a helicopter rotor blade on its aeroelastic responses. An aeroelastic analysis using finite elements in spatial and temporal domains is used to compute the helicopter rotor frequencies, vibratory hub loads, power required and stability in forward flight. The novelty of the work lies in the application of advanced data-driven machine learning (ML) techniques, such as convolution neural networks (CNN), multi-layer perceptron (MLP), random forests, support vector machines and adaptive Gaussian process (GP) for capturing the nonlinear responses of these complex spatio-temporal models to develop an efficient physics-informed ML framework for stochastic rotor analysis. Thus, the work is of practical significance as (i) it accounts for manufacturing uncertainties, (ii) accurately quantifies their effects on nonlinear response of rotor blade and (iii) makes the computationally expensive simulations viable by the use of ML. A rigorous performance assessment of the aforementioned approaches is presented by demonstrating validation on the training dataset and prediction on the test dataset. The contribution of the study lies in the following findings: (i) The uncertainty in composite material and geometric properties can lead to significant variations in the rotor aeroelastic responses and thereby highlighting that the consideration of manufacturing variability in analyzing helicopter rotors is crucial for assessing their behaviour in real-life scenarios. (ii) Precisely, the substantial effect of uncertainty has been observed on the six vibratory hub loads and the damping with the highest impact on the yawing hub moment. Therefore, sufficient factor of safety should be considered in the design to alleviate the effects of perturbation in the simulation results. (iii) Although advanced ML techniques are harder to train, the optimal model configuration is capable of approximating the nonlinear response trends accurately. GP and CNN followed by MLP achieved satisfactory performance. Excellent accuracy achieved by the above ML techniques demonstrates their potential for application in the optimization of rotors under uncertainty.
Periodic structures attenuate wave propagation in a specified frequency range, such that a desired bandgap behaviour can be obtained. Most periodic structures are produced by additive manufacturing. However, it is recently found that the variability in the manufacturing processes can lead to a significant deviation from the desired behaviour. This paper investigates the elastic wave propagation of stochastic hexagonal periodic lattice structures considering micro-structural variability. Thus, the effect of uncertainties in the material and geometrical parameters of the unit cell is quantified on the wave propagation in hexagonal lattices. Based on Bloch’s theorem and the finite element method, the band structures are determined as a function of the frequency and wave vector at the unit cell level and later scaled-up via full-scale simulations of finite metamaterials with a prescribed number of cells. State of the practice machine learning techniques, namely the Gaussian process, multi-layer perceptron, radial basis neural network and support vector machine, are employed as grey-box meta-models to capture the stochastic wave propagation response. The results demonstrate good accuracy by validation with Monte Carlo simulations. The study illustrates that considering the effect of uncertainties on the wave propagation behaviour of hexagonal periodic lattices is critical for their practical applicability and desirable performance. Based on the results, the manufacturing tolerances of the hexagonal lattices can be obtained to attain a bandgap within a certain frequency band.
This paper investigates the elastic wave propagation, mode veering, and in-plane vibration of pre-stressed hexagonal lattice embedded in an elastic medium and composed of axially loaded Timoshenko beams with attached point masses. The frequency band structure of the lattice system is obtained by solving the corresponding eigenvalue problem based on the Bloch theorem and the finite element method. The parametric study is performed by investigating the effects of the pre-stress magnitude, stiffness of elastic medium, and attached point masses on the band structure of a lattice unit cell. For simulating the free vibration behavior of the proposed lattices with different topologies, the Hurty-Craig-Bampton method is introduced to reduce the number of degrees of freedom. Based on the reduced finite element model, the natural frequencies are determined for various boundary conditions. The additional interface reduction technique, called system-level reduction, has been observed to achieve accurate results compared to that of the full model. Numerical experiments demonstrated a significant influence of the additional masses, pre-stress, and stiffness of elastic medium on Bloch waves and eigenvalues of the proposed lattice systems. The effects of different parameters on the emergence of mode veering phenomenon and band gaps are investigated in detail.
The role of robust design optimization (RDO) has been eminent, ascertaining optimal configuration of engineering systems in the presence of uncertainties. However, computational aspect of RDO can often get tediously intensive in dealing with large scale systems. To address this issue, hybrid polynomial correlated function expansion (H-PCFE) based RDO framework has been developed for solving computationally expensive problems. H-PCFE performs as a bi-level approximation tool, handling the global model behavior and local functional variation. Analytical formula for the mean and standard deviation of the responses have been proposed, which reduces significant level of computations as no further simulations are required for evaluating the statistical moments within the optimization routine. Implementation of the proposed approaches have been demonstrated with two benchmark examples and two practical engineering problems. The performance of H-PCFE and its analytical version have been assessed by comparison with direct Monte Carlo simulation (MCS). Comparison with popular state-of-the-art techniques has also been presented. Excellent results in terms of accuracy and computational effort obtained makes the proposed methodology potential for further large scale industrial applications.
Two novel surrogate-based approaches have been developed for uncertainty quantification of engineering systems. In doing so, two well-known techniques, namely, high dimensional model representation (HDMR) and Kriging, have been integrated. Specifically, the trend portion of Kriging has been replaced by HDMR such that the approximation accuracy may be enhanced. The improvement in accuracy is the result of the fact that the proposed hybrid surrogate model performs a two-tier approximation, first capturing the global variation in the functional space using a set of component functions by HDMR and subsequently interpolating the local fluctuations by Kriging. Additionally, to improve the computational cost of this proposed model, feature selection approaches, namely, least absolute shrinkage and selection operator and least angle regression have been employed. These efficient schemes utilized to determine the relevant unknown coefficients induces adaptive sparsity for the proposed surrogate models. The performance of the proposed approaches has been assessed by solving an analytical and practical engineering problem. The results illustrate excellent performance of the proposed approaches in terms of both approximation accuracy and computational effort.
System uncertainties often lead to deviation from the anticipated behaviour of acoustic metamaterials (AMs) and thus, make their intended design ineffective. Therefore, in this study, the effect of stochastic parameters on a recently developed phenomenon in AMs, known as active metadamping is quantified using machine learning (ML). The enhancement of damping in an active feedback-controlled metamaterial over an equivalent uncontrolled counterpart is defined as active metadamping. Metadamping enables a metamaterial to dissipate energy faster in addition to its inherent bandgap characteristics. The ML technique, Gaussian process is used as the surrogate model to capture the stochastic dynamics of the active AM. A trade-off in the spatial attenuation and temporal energy dissipation characteristics is observed while velocity-feedback control is applied within the resonating units of the metamaterial. The overall dissipation and the decay ratio increase around five times in the controlled case; whereas, the attenuation bandwidth reduces or even vanishes. Most importantly, the results elucidate that metadamping is robust to the uncertainty of the system parameters, unlike the energy and the decay amplitude for the controlled case. Additionally, Gaussian process is able to capture the behaviour of active AMs by using only 1% computational cost as compared to that of the Monte Carlo simulations. •Uncertainty in the metadamping is investigated for the first time.•Gaussian Process can capture the stochastic response by using 1% cost compared to MCS.•Metadamping is relatively robust compared to normalized energy and the decay amplitude.
AbstractTo reduce the computational cost of assembled stochastic linear structural dynamic systems, a three-staged reduced order model-based framework for forward uncertainty propagation was developed. First, the physical domain was decomposed by constructing an equivalent reduced order numerical model that limited the cost of a single deterministic simulation. This was done in two phases: (1) reducing the system matrices of the subcomponents using component mode synthesis and (2) solving the resulting reduced system with the help of domain decomposition in an efficient manner. Second, functional decomposition was carried out in the stochastic space by employing a multioutput machine learning model that reduced the number of eigenvalue analyses to be performed. Thus, a multilevel framework was developed that propagated the dynamic response from the subcomponent level to the assembled global system level efficiently. Subsequently, reliability analysis was performed to assess the safety level and failure probability of linear stochastic dynamic systems. The results achieved by solving a two-dimensional (2D) building frame and a three-dimensional (3D) transmission tower model illustrated good performance of the proposed methodology, highlighting its potential for complex problems.
Robust design optimization (RDO) of large-scale engineering systems is computationally intensive and requires significant CPU time. Considerable computational effort is still required within conventional meta-model assisted RDO frameworks. The primary objective of this article is to minimize further the computational requirements of meta-model assisted RDO by developing a global two-layered approximation based RDO technique. The meta-model in the inner layer approximates the response quantity and the meta-model in the outer layer approximates the response statistics computed from the response meta-model. This approach eliminates both model building and Monte Carlo simulation from the optimization cycle, and requires considerably fewer actual response evaluations than a single-layered approximation. To demonstrate the approach, two recently developed compressive sensing enabled globally refined Kriging models have been utilized. The proposed framework is applied to one test example and two real-life applications to illustrate clearly its potential to yield robust optimal solutions with minimal computational cost.
In this work, we illustrate the implementation of physics informed neural networks (PINNs) for solving forward and inverse problems in structural vibration. Physics informed deep learning has lately proven to be a powerful tool for the solution and data-driven discovery of physical systems governed by differential equations. In spite of the popularity of PINNs, their application in structural vibrations is limited. This motivates the extension of the application of PINNs in yet another new domain and leverages from the available knowledge in the form of governing physical laws. On investigating the performance of conventional PINNs in vibrations, it is mostly found that it suffers from a very recently pointed out similar scaling or regularization issue, leading to inaccurate predictions. It is thereby demonstrated that a simple strategy of modifying the loss function helps to combat the situation and enhance the approximation accuracy significantly without adding any extra computational cost. In addition to the above two contributing factors of this work, the implementation of the conventional and modified PINNs is performed in the MATLAB environment owing to its recently developed rich deep learning library. Since all the developments of PINNs till date is Python based, this is expected to diversify the field and reach out to greater scientific audience who are more proficient in MATLAB but are interested to explore the prospect of deep learning in computational science and engineering. As a bonus, complete executable codes of all four representative (both forward and inverse) problems in structural vibrations have been provided along with their line-by-line lucid explanation and well-interpreted results for better understanding.
[Display omitted] •Parametric amplification of nonlinear piezoelectric energy harvester is studied.•Two parametric excitations with quadratic and cubic geometrical nonlinearities.•Frequency response is obtained by path-following and IHB method.•Parametric excitation causes significant amplification of energy harvester voltage. The steady-state response of a nonlinear piezoelectric energy harvester subjected to external and parametric excitation is investigated based on the Mathieu-Duffing nonlinear oscillator model. The parametric excitation is introduced to amplify the external harmonic excitation and extend the capabilities of the nonlinear piezoelectric energy harvester device. To obtain the approximated solution of the nonlinear periodic responses for displacement and electrical voltage of the energy harvester, the incremental harmonic balance method in combination with the path-following technique is adopted. It is assumed that the proposed nonlinear model consists of cubic and quadratic nonlinearity, where parametric amplification appears in the form of a trigonometric function. The frequency is tuned as one-to-one and the one-to-two ratio between external and parametric excitation. The effects of quadratic and cubic nonlinearity as well as parametric amplification are studied in detail, and their incredible properties to extend harvester application performance is illustrated. It is explicitly demonstrated that for some particular combination of the system parameters, vibration amplitudes and harvested power can be amplified up to three or five times in comparison to the classical broadband nonlinear energy harvester based on the forced Duffing oscillator. This extraordinary amplification shown to be a key motivation to realize the proposed concept in practice. The presence of combined quadratic and cubic nonlinearities resulted in both hardening and softening spring behavior and leading to the appearance of coexisting periodic solutions in the amplitude-frequency responses. Periodic orbits obtained by the proposed methodology are verified with the results from direct numerical integration and fine agreement is demonstrated. Moreover, a significant influence of the parametric amplification on the instantaneous power is revealed in time response diagrams, thus showing better performance of the proposed energy harvester system.
Multiscale Topology Optimisation (MTO), by definition, operates over multiple length scales to achieve an optimised structural solution. Consequentially MTO can be computationally very expensive. Machine learning provides the opportunity to accelerate the process utilising surrogate models that approximate the behaviour of the system, allowing for faster evaluation and optimisation. This is particularly advantageous in scenarios where direct simulations at all scales are impractical or prohibitively time-consuming. In this study we demonstrate the effectiveness of a convolutional neural network (CNN) to map topologies of 2D micro-architectures to their corresponding stiffness property matrices. We utilise the topological dataset presented by Jiang et al [1] and generate the stiffness properties using 2D homogenisation [2] for micro-architectures made of a combination of printable polymeric materials (i.e. from a multi-material 3D printer such as an Object 260). We present a successful mapping, achieving results of R 2 greater than 99% and a mean average error of 0.012. Additionally, we examine the transferability of the initial learning of the above trained network. The trained mapping was used on the same topological dataset but with a different material combination. R 2 reduced to 34% and mean average error rose to 0.436. Mappings trained on one material combination will therefore be valid for only a small section of the total design space. To further explore the transferability of CNN, two architectures are proposed and evaluated. One network architecture employs transfer learning and the other incorporates branched connections which account for different printable material combinations. These models are assessed in terms of both the reduction in the computational cost, by leveraging the already trained CNN, and accuracy.