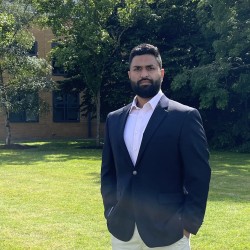
Taimoor Rizwan
Academic and research departments
Centre for Vision, Speech and Signal Processing (CVSSP), Faculty of Engineering and Physical Sciences.About
My research project
Morphology and Identity in Generative Models: High-Resolution Facial Image ManipulationThis research advances the field of generative models by exploring the interplay between morphology and identity in high-resolution facial image manipulation. At its core is DIFF-ID, a novel framework we developed for identity-preserving attribute manipulation using diffusion models. DIFF-ID enables precise control over facial attributes—such as age, expression, and style—while maintaining high-quality, realistic outputs and preserving natural morphological features. Building on this foundation, the research is structured into three interconnected phases. First, we refine and extend DIFF-ID to achieve even greater precision in identity-driven attribute manipulation for high-resolution facial generation. Second, we apply these advancements to create morphology-based deepfakes, leveraging DIFF-ID’s capabilities to generate highly realistic synthetic faces that challenge the limits of existing detection systems. Finally, we develop a deepfake detection system specifically designed to counter morphology-based attacks, using insights from DIFF-ID to improve detection accuracy and robustness. By integrating advancements in generative models, morphological analysis, and deepfake detection, this research not only pushes the boundaries of facial image manipulation but also addresses critical ethical and security concerns in the era of synthetic media.
Supervisors
This research advances the field of generative models by exploring the interplay between morphology and identity in high-resolution facial image manipulation. At its core is DIFF-ID, a novel framework we developed for identity-preserving attribute manipulation using diffusion models. DIFF-ID enables precise control over facial attributes—such as age, expression, and style—while maintaining high-quality, realistic outputs and preserving natural morphological features. Building on this foundation, the research is structured into three interconnected phases. First, we refine and extend DIFF-ID to achieve even greater precision in identity-driven attribute manipulation for high-resolution facial generation. Second, we apply these advancements to create morphology-based deepfakes, leveraging DIFF-ID’s capabilities to generate highly realistic synthetic faces that challenge the limits of existing detection systems. Finally, we develop a deepfake detection system specifically designed to counter morphology-based attacks, using insights from DIFF-ID to improve detection accuracy and robustness. By integrating advancements in generative models, morphological analysis, and deepfake detection, this research not only pushes the boundaries of facial image manipulation but also addresses critical ethical and security concerns in the era of synthetic media.
ResearchResearch interests
I am passionate about exploring the transformative potential of generative AI and its applications in facial image manipulation and synthetic media. My research interests lie at the intersection of computer vision, deep learning, and digital ethics, with a focus on creating and controlling high-resolution synthetic faces while preserving identity and natural features. I am particularly intrigued by the challenges of balancing realism and control in generative models, enabling applications in areas like digital art, entertainment, and personalized media.
Beyond the creative possibilities, I am deeply invested in addressing the ethical and security implications of these technologies. This includes investigating advanced methods for deepfake detection and developing frameworks to mitigate the risks posed by synthetic media. I am also interested in the broader societal impact of generative AI, such as its influence on identity, privacy, and trust in digital content. By combining technical innovation with ethical considerations, my work aims to advance the field of generative AI while ensuring its responsible and beneficial use in shaping the future of digital media.
Research interests
I am passionate about exploring the transformative potential of generative AI and its applications in facial image manipulation and synthetic media. My research interests lie at the intersection of computer vision, deep learning, and digital ethics, with a focus on creating and controlling high-resolution synthetic faces while preserving identity and natural features. I am particularly intrigued by the challenges of balancing realism and control in generative models, enabling applications in areas like digital art, entertainment, and personalized media.
Beyond the creative possibilities, I am deeply invested in addressing the ethical and security implications of these technologies. This includes investigating advanced methods for deepfake detection and developing frameworks to mitigate the risks posed by synthetic media. I am also interested in the broader societal impact of generative AI, such as its influence on identity, privacy, and trust in digital content. By combining technical innovation with ethical considerations, my work aims to advance the field of generative AI while ensuring its responsible and beneficial use in shaping the future of digital media.
Teaching
Lab Demonstrator & PALS Leader
University of Surrey · Part-time
Supported students in COM2039 Parallel Computing and COM2028 Artificial Intelligence by bridging theory and practice.
- Parallel Computing: Guided students in CUDA C/C++ programming, covering GPU architecture, parallel algorithms, and performance optimization. Assisted with debugging in Eclipse IDE and explained concepts like decomposition and load balancing.
- Artificial Intelligence: Taught machine learning fundamentals, including classification (e.g., decision trees, SVMs) and regression (e.g., linear regression). Introduced tools like Google Colab, scikit-learn, and TensorFlow for hands-on model building and evaluation.
Focused on creating an engaging learning environment, helping students develop technical and problem-solving skills through theoretical insights and practical exercises.
Sustainable development goals
My research interests are related to the following:
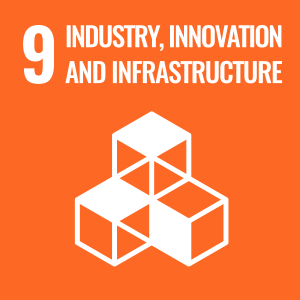
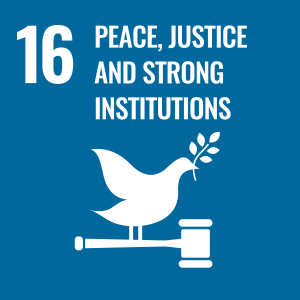
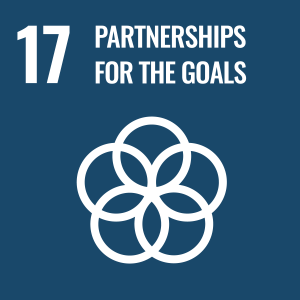