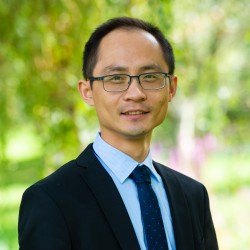
Dr Zhenhua Feng SM-IEEE, FHEA
Academic and research departments
Nature Inspired Computing and Engineering Research Group, Centre for Vision, Speech and Signal Processing (CVSSP), Surrey Institute for People-Centred Artificial Intelligence (PAI), Computer Science Research Centre, School of Computer Science and Electronic Engineering.About
Biography
I am a Senior Lecturer in Computer Vision and Machine Learning at the School of Computer Science and Electronic Engineering, the University of Surrey. I received my PhD in computer vision and pattern recognition from the Centre for Vision, Speech and Signal Processing (CVSSP) at the University of Surrey in 2016. Then I worked as a Research Fellow and Senior Research Fellow at CVSSP, from 2016-2020. In June 2020, I joined the Department of Computer Science at the University of Surrey as a Lecturer and was promoted to Senior Lecturer in August 2023.
I currently serve as a Senior Member of IEEE, Associate Editor for IEEE Transactions on Neural Networks and Learning Systems (IEEE T-NNLS) and the Springer Journal Complex & Intelligent Systems. I also served as the Guest Editor for the International Journal of Computer Vision (IJCV), Programme Chair for the British Machine Vision Conference (BMVC) 2022, Area Chair for BMVC 2021/22/23, Area Chair for ACM SIGGRAPH European Conference on Visual Media Production (CVMP) 2022/23, Senior Programme Committee Member for the International Joint Conference on Artificial Intelligence (IJCAI) 2021, etc.
My research interests include computer vision, machine learning, pattern recognition, image processing, biometrics, bioinformatics, and other AI topics.
Areas of specialism
University roles and responsibilities
- Programme Lead of Computer Science BSc
- Surrey AI Fellow of the People-Centred AI Institute
- UG Personal Tutor
Affiliations and memberships
Supervision
Postgraduate research supervision
I am looking for talented PhD students in machine learning, computer vision and pattern recognition.
Current PhD students
- Fatemeh Nazarieh (October 2022 - ) Cross-modality content generation
- Maxim Tyshkovsky (May 2022 -) Uncertainty estimation for autonomous driving
- Vinal Asodia (October 2021 - ) Perception-based control systems for autonomous driving
- Jinghao Zhang (April 2021 - ) Deep adversarial learning in computer vision
- Lei Ju (January 2020 - ) Attribute-aware full-stack facial analysis
Visitors
- Yixia Zhao (February 2023 - December 2023) AI in E-Learning
- Yue Peng (May 2022 - April 2023) Dynamic train rescheduling of high-speed railway based on deep reinforcement learning
PhD examination
- Guoyang Xie, University of Surrey, October 2023 Thesis title: Few-Shot Image Anomaly Detection in Manufacturing and Medical Imaging
- Peixia Li, University of Sydney, October 2023 Thesis title: Deep Neural Networks for Visual Object Tracking: An Investigation of Performance Optimization
- Ruoqing Yin, University of Surrey, June 2023 (MPhil) Thesis title: Dental Disease Detection with Crowdsourced Radiograph Annotations
- Farshid Rayhan, University of Manchester, November 2022, Thesis title: Estimating driver state from facial behaviour
- Fivos Ntelemis, University of Surrey, August 2022 Thesis title: Deep clustering analysis and representation learning for high-dimensional data
- Soroush Fatemifar, University of Surrey, June 2022 Thesis title: The challenges of anomaly detection
- Monika Jain, IIIT-Delhi, June 2022 Thesis title: Regularized ensemble correlation filter tracking
- Bo Gao, King's College London, March 2022 Thesis title: Novel approaches to suppress tracking drift caused by similar-looking distractors
- Boyan Xu, University of Manchester, November 2021 (MPhil) Thesis Title: Deep learning for single image deblurring
- Ali Shahin Shamsabadi, Queen Mary University of London, March 2021 Thesis title: Designing content-based adversarial perturbations and distributed one-class learning for images
- Matthew Shere, University of Surrey, June 2021 Thesis title: Spherical based human tracking and 3D pose estimation for immersive entertainment production
Teaching
Offic hour: TBD for 2022/23 academic year
2022-2023
2021-2022
- COM2028 Artificial Intelligence
- COM2027 Software Engineering Project
2020-2021
- COM1027 Programming Fundamentals
- COM2027 Software Engineering Project
Publications
—With the rapid development in face recognition, most of the existing systems can perform very well in uncon-strained scenarios. However, it is still a very challenging task to detect face spoofing attacks, thus face anti-spoofing has become one of the most important research topics in the community. Though various anti-spoofing models have been proposed, the generalisation capability of these models usually degrades for unseen attacks in the presence of challenging appearance variations , e.g., background, illumination, diverse spoofing materials and low image quality. To address this issue, we propose to use a Generative Adversarial Network (GAN) that transfers an input face image from the RGB domain to the depth domain. The generated depth clue enables biometric preservation against challenging appearance variations and diverse image qualities. To be more specific, the proposed method has two main stages. The first one is a GAN-based domain transfer module that converts an input image to its corresponding depth map. By design, a live face image should be transferred to a depth map whereas a spoofing face image should be transferred to a plain (black) image. The aim is to improve the discriminative capability of the proposed system. The second stage is a classification model that determines whether an input face image is live or spoofing. Benefit from the use of the GAN-based domain transfer module, the latent variables can effectively represent the depth information, complementarily enhancing the discrimination of the original RGB features. The experimental results obtained on several benchmarking datasets demonstrate the effectiveness of the proposed method, with superior performance over the state-of-the-art methods. The source code of the proposed method is publicly available at https://github.com/coderwangson/DFA. Index Terms—Face anti-spoofing, generative adversarial network , domain transfer.
In recent years, deep-learning-based face detectors have achieved promising results and been successfully used in a wide range of practical applications. However, extreme appearance variations are still the major obstacles for robust and accurate face detection in the wild. To address this issue, we propose an Improved Training Sample Selection (ITSS) strategy for mining effective positive and negative samples during network training. The proposed ITSS procedure collaborates with face sampling during data augmentation and selects suitable positive sample centres and IoU overlap for face detection. Moreover, we propose a Residual Feature Pyramid Fusion (RFPF) module that collects semantically robust features to improve the scale-invariance of deep features and better represent faces at different feature pyramid levels. The experimental results obtained on the FDDB and WiderFace datasets demonstrate the superiority of the proposed method over the state-of-the-art approaches. Specially, the proposed method achieves 96.9% and 96.2% in terms of AP on the easy and medium test sets of WiderFace.
Advanced Siamese visual object tracking architectures are jointly trained using pair-wise input images to perform target classification and bounding box regression. They have achieved promising results in recent benchmarks and competitions. However, the existing methods suffer from two limitations: First, though the Siamese structure can estimate the target state in an instance frame, provided the target appearance does not deviate too much from the template, the detection of the target in an image cannot be guaranteed in the presence of severe appearance variations. Second, despite the classification and regression tasks sharing the same output from the backbone network, their specific modules and loss functions are invariably designed independently, without promoting any interaction. Yet, in a general tracking task, the centre classification and bounding box regression tasks are collaboratively working to estimate the final target location. To address the above issues, it is essential to perform target-agnostic detection so as to promote cross-task interactions in a Siamese-based tracking framework. In this work, we endow a novel network with a target-agnostic object detection module to complement the direct target inference, and to avoid or minimise the misalignment of the key cues of potential template-instance matches. To unify the multi-task learning formulation, we develop a cross-task interaction module to ensure consistent supervision of the classification and regression branches, improving the synergy of different branches. To eliminate potential inconsistencies that may arise within a multi-task architecture, we assign adaptive labels, rather than fixed hard labels, to supervise the network training more effectively. The experimental results obtained on several benchmarks, i.e ., OTB100, UAV123, VOT2018, VOT2019, and LaSOT, demonstrate the effectiveness of the advanced target detection module, as well as the cross-task interaction, exhibiting superior tracking performance as compared with the state-of-the-art tracking methods.
The research in pedestrian detection has made remarkable progress in recent years. However, robust pedestrian detection in crowded scenes remains a considerable challenge. Many methods resort to additional annotations (visible body or head) of a dataset or develop attention mechanisms to alleviate the difficulties posed by occlusions. However, these methods rarely use contextual information to strengthen the features extracted by a backbone network. The main aim of this paper is to extract more effective and discriminative features of pedestrians for robust pedestrian detection with heavy occlusions. To this end, we propose a Global Context-Aware module to exploit contextual information for pedestrian detection. Fusing global context with the information derived from the visible part of occluded pedestrians enhances feature representations. The experimental results obtained on two challenging benchmarks, CrowdHuman and CityPersons, demonstrate the effectiveness and merits of the proposed method. Code and models are available at: https://github.com/FlyingZstar/crowded pedestrian detection.
The Visual Object Tracking challenge VOT2022 is the tenth annual tracker benchmarking activity organized by the VOT initiative. Results of 93 entries are presented; many are state-of-the-art trackers published at major computer vision conferences or in journals in recent years. The VOT2022 challenge was composed of seven sub-challenges focusing on different tracking domains: (i) VOT-STs2022 challenge focused on short-term tracking in RGB by segmentation, (ii) VOT-STb2022 challenge focused on short-term tracking in RGB by bounding boxes, (iii) VOT-RTs2022 challenge focused on “real-time” short-term tracking in RGB by segmentation, (iv) VOT-RTb2022 challenge focused on “real-time” short-term tracking in RGB by bounding boxes, (v) VOT-LT2022 focused on long-term tracking, namely coping with target disappearance and reappearance, (vi) VOT-RGBD2022 challenge focused on short-term tracking in RGB and depth imagery, and (vii) VOT-D2022 challenge focused on short-term tracking in depth-only imagery. New datasets were introduced in VOT-LT2022 and VOT-RGBD2022, VOT-ST2022 dataset was refreshed, and a training dataset was introduced for VOT-LT2022. The source code for most of the trackers, the datasets, the evaluation kit and the results are publicly available at the challenge website (http://votchallenge.net).
A big, diverse and balanced training data is the key to the success of deep neural network training. However, existing publicly available datasets used in facial landmark localization are usually much smaller than those for other computer vision tasks. To mitigate this issue, this paper presents a novel Separable Batch Normalization (SepBN) method. Different from the classical BN layer, the proposed SepBN module learns multiple sets of mapping parameters to adaptively scale and shift the normalized feature maps via a feed-forward attention mechanism. The channels of an input tensor are divided into several groups and the different mapping parameter combinations are calculated for each group according to the attention weights to improve the parameter utilization. The experimental results obtained on several well-known benchmarking datasets demonstrate the effectiveness and merits of the proposed method.
In recent years, attention mechanisms have been widely studied in Discriminative Correlation Filter (DCF) based visual object tracking. To realise spatial attention and discriminative feature mining, existing approaches usually apply regularisation terms to the spatial dimension of multi-channel features. However, these spatial regularisation approaches construct a shared spatial attention pattern for all multi-channel features, without considering the diversity across channels. As each feature map (channel) focuses on a specific visual attribute, a shared spatial attention pattern limits the capability for mining important information from different channels. To address this issue, we advocate channel-specific spatial attention for DCF-based trackers. The key ingredient of the proposed method is an Adaptive Attribute-Aware spatial attention mechanism for constructing a novel DCF-based tracker (A^3 DCF). To highlight the discriminative elements in each feature map, spatial sparsity is imposed in the filter learning stage, moderated by the prior knowledge regarding the expected concentration of signal energy. In addition, we perform a post processing of the identified spatial patterns to alleviate the impact of less significant channels. The net effect is that the irrelevant and inconsistent channels are removed by the proposed method. The results obtained on a number of well-known benchmarking datasets, including OTB2015, DTB70, UAV123, VOT2018, LaSOT, GOT-10 K and TrackingNet, demonstrate the merits of the proposed A^3 DCF tracker, with improved performance compared to the state-of-the-art methods.
Face anti-spoofing (FAS) is crucial for safe and reliable biometric systems. In recent years, deep neural networks have been proven to be very effective for FAS as compared with classical approaches. However, deep learning-based FAS methods are data-driven and use learning-based features only. It is a legitimate question to ask whether hand-crafted features can provide any complementary information to a deep learning-based FAS method. To answer this question, we propose a two-stream network that consists of a convolutional network and a local difference network. To be specific, we first build a texture extraction convolutional block to calculate the gradient magnitude at each pixel of an input image. Our experiments demonstrate that additional liveness cues can be captured by the proposed method. Second, we design an attention fusion module to combine the features obtained from the RGB domain and gradient magnitude domain, aiming for discriminative information mining and information redundancy elimination. Finally, we advocate a simple binary facial mask supervision strategy for further performance boost. The proposed network has only 2.79M parameters and the inference speed is up to 118 frames per second, which makes it very convenient for real-time FAS systems. The experimental results obtained on several well-known benchmarking datasets demonstrate the merits and superiority of the proposed method over the state-of-the-art approaches.
Cross-modal content generation has become very popular in recent years. To generate high-quality and realistic content, a variety of methods have been proposed. Among these approaches, visual content generation has attracted significant attention from academia and industry due to its vast potential in various applications. This survey provides an overview of recent advances in visual content generation conditioned on other modalities, such as text, audio, speech, and music, with a focus on their key contributions to the community. In addition, we summarize the existing publicly available datasets that can be used for training and benchmarking cross-modal visual content generation models. We provide an in-depth exploration of the datasets used for audio-to-visual content generation, filling a gap in the existing literature. Various evaluation metrics are also introduced along with the datasets. Furthermore, we discuss the challenges and limitations encountered in the area, such as modality alignment and semantic coherence. Last, we outline possible future directions for synthesizing visual content from other modalities including the exploration of new modalities, and the development of multi-task multi-modal networks. This survey serves as a resource for researchers interested in quickly gaining insights into this burgeoning field.
In recent years, discriminative correlation filter (DCF) based algorithms have significantly advanced the state of the art in visual object tracking. The key to the success of DCF is an efficient discriminative regression model trained with powerful multi-cue features, including both hand-crafted and deep neural network features. However, the tracking performance is hindered by their inability to respond adequately to abrupt target appearance variations. This issue is posed by the limited representation capability of fixed image features. In this work, we set out to rectify this shortcoming by proposing a complementary representation of a visual content. Specifically, we propose the use of a collaborative representation between successive frames to extract the dynamic appearance information from a target with rapid appearance changes, which results in suppressing the undesirable impact of the background. The resulting collaborative representation coefficients are combined with the original feature maps using a spatially regularised DCF framework for performance boosting. The experimental results on several benchmarking datasets demonstrate the effectiveness and robustness of the proposed method, as compared with a number of state-of-the-art tracking algorithms.
Appearance variations result in many difficulties in face image analysis. To deal with this challenge, we present a Unified Tensor-based Active Appearance Model (UT-AAM) for jointly modelling the geometry and texture information of 2D faces. For each type of face information, namely shape and texture, we construct a unified tensor model capturing all relevant appearance variations. This contrasts with the variation-specific models of the classical tensor AAM. To achieve the unification across pose variations, a strategy for dealing with self-occluded faces is proposed to obtain consistent shape and texture representations of pose-varied faces. In addition, our UT-AAM is capable of constructing the model from an incomplete training dataset, using tensor completion methods. Last, we use an effective cascaded-regression-based method for UT-AAM fitting. With these advancements, the utility of UT-AAM in practice is considerably enhanced. As an example, we demonstrate the improvements in training facial landmark detectors through the use of UT-AAM to synthesise a large number of virtual samples. Experimental results obtained on a number of well-known face datasets demonstrate the merits of the proposed approach.
Recently, the security of multimodal verification has become a grow-ing concern since many fusion systems have been known to be easily deceived by partial spoof attacks, i.e. only a subset of modalities is spoofed. In this paper, we verify such a vulnerability and propose to use two representation-based met-rics to close this gap. Firstly, we use the collaborative representation fidelity with non-target subjects to measure the affinity of a query sample to the claimed client. We further consider sparse coding as a competing comparison among the client and the non-target subjects, and hence explore two sparsity-based measures for recognition. Last, we select the representation-based measure, and assemble its score and the affinity score of each modality to train a support vector machine classifier. Our experimental results on a chimeric multimodal database with face and ear traits demonstrate that in both regular verification and partial spoof at-tacks, the proposed method significant
We propose a new Group Feature Selection method for Discriminative Correlation Filters (GFS-DCF) based visual object tracking. The key innovation of the proposed method is to perform group feature selection across both channel and spatial dimensions, thus to pinpoint the structural relevance of multi-channel features to the filtering system. In contrast to the widely used spatial regularisation or feature selection methods, to the best of our knowledge, this is the first time that channel selection has been advocated for DCF-based tracking. We demonstrate that our GFS-DCF method is able to significantly improve the performance of a DCF tracker equipped with deep neural network features. In addition, our GFS-DCF enables joint feature selection and filter learning, achieving enhanced discrimination and interpretability of the learned filters. To further improve the performance, we adaptively integrate historical information by constraining filters to be smooth across temporal frames, using an efficient low-rank approximation. By design, specific temporal-spatial-channel configurations are dynamically learned in the tracking process, highlighting the relevant features, and alleviating the performance degrading impact of less discriminative representations and reducing information redundancy. The experimental results obtained on OTB2013, OTB2015, VOT2017, VOT2018 and TrackingNet demonstrate the merits of our GFS-DCF and its superiority over the state-of-the-art trackers. The code is publicly available at https://github.com/XU-TIANYANG/GFS-DCF.
This paper investigates the evaluation of dense 3D face reconstruction from a single 2D image in the wild. To this end, we organise a competition that provides a new benchmark dataset that contains 2000 2D facial images of 135 subjects as well as their 3D ground truth face scans. In contrast to previous competitions or challenges, the aim of this new benchmark dataset is to evaluate the accuracy of a 3D dense face reconstruction algorithm using real, accurate and high-resolution 3D ground truth face scans. In addition to the dataset, we provide a standard protocol as well as a Python script for the evaluation. Last, we report the results obtained by three state-of-the-art 3D face reconstruction systems on the new benchmark dataset. The competition is organised along with the 2018 13th IEEE Conference on Automatic Face & Gesture Recognition.
Discriminative Correlation Filters (DCF) have been shown to achieve impressive performance in visual object tracking. However, existing DCF-based trackers rely heavily on learning regularised appearance models from invariant image feature representations. To further improve the performance of DCF in accuracy and provide a parsimonious model from the attribute perspective, we propose to gauge the relevance of multi-channel features for the purpose of channel selection. This is achieved by assessing the information conveyed by the features of each channel as a group, using an adaptive group elastic net inducing independent sparsity and temporal smoothness on the DCF solution. The robustness and stability of the learned appearance model are significantly enhanced by the proposed method as the process of channel selection performs implicit spatial regularisation. We use the augmented Lagrangian method to optimise the discriminative filters efficiently. The experimental results obtained on a number of well-known benchmarking datasets demonstrate the effectiveness and stability of the proposed method. A superior performance over the state-of-the-art trackers is achieved using less than $$10\%$$ 10 % deep feature channels.
—Existing studies in facial age estimation have mostly focused on intra-dataset protocols that assume training and test images captured under similar conditions. However, this is rarely valid in practical applications, where training and test sets usually have different characteristics. In this paper, we advocate a cross-dataset protocol for age estimation benchmarking. In order to improve the cross-dataset age estimation performance, we mitigate the inherent bias caused by the learning algorithm itself. To this end, we propose a novel loss function that is more effective for neural network training. The relative smoothness of the proposed loss function is its advantage with regards to the optimisation process performed by stochastic gradient descent. Its lower gradient, compared with existing loss functions, facilitates the discovery of and convergence to a better optimum, and consequently a better generalisation. The crossdataset experimental results demonstrate the superiority of the proposed method over the state-of-the-art algorithms in terms of accuracy and generalisation capability.
We present a new loss function, namely Wing loss, for robust facial landmark localisation with Convolutional Neural Networks (CNNs). We first compare and analyse different loss functions including L2, L1 and smooth L1. The analysis of these loss functions suggests that, for the training of a CNN-based localisation model, more attention should be paid to small and medium range errors. To this end, we design a piece-wise loss function. The new loss amplifies the impact of errors from the interval (-w, w) by switching from L1 loss to a modified logarithm function. To address the problem of under-representation of samples with large out-of-plane head rotations in the training set, we propose a simple but effective boosting strategy, referred to as pose-based data balancing. In particular, we deal with the data imbalance problem by duplicating the minority training samples and perturbing them by injecting random image rotation, bounding box translation and other data augmentation approaches. Last, the proposed approach is extended to create a two-stage framework for robust facial landmark localisation. The experimental results obtained on AFLW and 300W demonstrate the merits of the Wing loss function, and prove the superiority of the proposed method over the state-of-the-art approaches.
Contrastive learning has achieved great success in skeleton-based action recognition. However, most existing approaches encode the skeleton sequences as entangled spatiotemporal representations and confine the contrasts to the same level of representation. Instead, this paper introduces a novel contrastive learning framework, namely Spatiotemporal Clues Disentanglement Network (SCD-Net). Specifically, we integrate the decoupling module with a feature extractor to derive explicit clues from spatial and temporal domains respectively. As for the training of SCD-Net, with a constructed global anchor, we encourage the interaction between the anchor and extracted clues. Further, we propose a new masking strategy with structural constraints to strengthen the contextual associations, leveraging the latest development from masked image modelling into the proposed SCD-Net. We conduct extensive evaluations on the NTU-RGB+D (60&120) and PKU-MMD (I&II) datasets, covering various downstream tasks such as action recognition, action retrieval, transfer learning, and semi-supervised learning. The experimental results demonstrate the effectiveness of our method, which outperforms the existing state-of-the-art (SOTA) approaches significantly.
Modern face recognition systems extract face representations using deep neural networks (DNNs) and give excellent identification and verification results, when tested on high resolution (HR) images. However, the performance of such an algorithm degrades significantly for low resolution (LR) images. A straight forward solution could be to train a DNN, using simultaneously, high and low resolution face images. This approach yields a definite improvement at lower resolutions but suffers a performance degradation for high resolution images. To overcome this shortcoming, we propose to train a network using both HR and LR images under the guidance of a fixed network, pretrained on HR face images. The guidance is provided by minimising the KL-divergence between the output Softmax probabilities of the pretrained (i.e., Teacher) and trainable (i.e., Student) network as well as by sharing the Softmax weights between the two networks. The resulting solution is tested on down-sampled images from FaceScrub and MegaFace datasets and shows a consistent performance improvement across various resolutions. We also tested our proposed solution on standard LR benchmarks such as TinyFace and SCFace. Our algorithm consistently outperforms the state-of-the-art methods on these datasets, confirming the effectiveness and merits of the proposed method.
We present a framework for robust face detection and landmark localisation of faces in the wild, which has been evaluated as part of 'the 2nd Facial Landmark Localisation Competition'. The framework has four stages: face detection, bounding box aggregation, pose estimation and landmark localisation. To achieve a high detection rate, we use two publicly available CNN-based face detectors and two proprietary detectors. We aggregate the detected face bounding boxes of each input image to reduce false positives and improve face detection accuracy. A cascaded shape regressor, trained using faces with a variety of pose variations, is then employed for pose estimation and image pre-processing. Last, we train the final cascaded shape regressor for fine-grained landmark localisation, using a large number of training samples with limited pose variations. The experimental results obtained on the 300W and Menpo benchmarks demonstrate the superiority of our framework over state-of-the-art methods.
Efficient and robust facial landmark localisation is crucial for the deployment of real-time face analysis systems. This paper presents a new loss function, namely Rectified Wing (RWing) loss, for regression-based facial landmark localisation with Convolutional Neural Networks (CNNs). We first systemically analyse different loss functions, including L2, L1 and smooth L1. The analysis suggests that the training of a network should pay more attention to small-medium errors. Motivated by this finding, we design a piece-wise loss that amplifies the impact of the samples with small-medium errors. Besides, we rectify the loss function for very small errors to mitigate the impact of inaccuracy of manual annotation. The use of our RWing loss boosts the performance significantly for regression-based CNNs in facial landmarking, especially for lightweight network architectures. To address the problem of under-representation of samples with large pose variations, we propose a simple but effective boosting strategy, referred to as pose-based data balancing. In particular, we deal with the data imbalance problem by duplicating the minority training samples and perturbing them by injecting random image rotation, bounding box translation and other data augmentation strategies. Last, the proposed approach is extended to create a coarse-to-fine framework for robust and efficient landmark localisation. Moreover, the proposed coarse-to-fine framework is able to deal with the small sample size problem effectively. The experimental results obtained on several well-known benchmarking datasets demonstrate the merits of our RWing loss and prove the superiority of the proposed method over the state-of-the-art approaches.
We present a new Cascaded Shape Regression (CSR) architecture, namely Dynamic Attention-Controlled CSR (DAC-CSR), for robust facial landmark detection on unconstrained faces. Our DAC-CSR divides facial landmark detection into three cascaded sub-tasks: face bounding box refinement, general CSR and attention-controlled CSR. The first two stages refine initial face bounding boxes and output intermediate facial landmarks. Then, an online dynamic model selection method is used to choose appropriate domain-specific CSRs for further landmark refinement. The key innovation of our DAC-CSR is the fault-tolerant mechanism, using fuzzy set sample weighting, for attentioncontrolled domain-specific model training. Moreover, we advocate data augmentation with a simple but effective 2D profile face generator, and context-aware feature extraction for better facial feature representation. Experimental results obtained on challenging datasets demonstrate the merits of our DAC-CSR over the state-of-the-art methods.
Recently, Adversarial Propagation (AdvProp) improves the standard accuracy of a trained model on clean samples. However, the training speed of AdvProp is much slower than vanilla training. Also, we argue that the use of adversarial samples in AdvProp is too drastic for robust feature learning of clean samples. This paper presents Mixup Propagation (MixProp) to further increase the standard accuracy on clean samples and reduce the training cost of AdvProp. The key idea of MixProp is to use mixup to generate samples for the auxiliary batch normalisation layer. This approach provides a moderate dataset as compared with adversarial samples and saves the time used for adversarial sample generation. The experimental results obtained on several datasets demonstrate the merits and superiority of the proposed method.
The paper presents a dictionary integration algorithm using 3D morphable face models (3DMM) for poseinvariant collaborative-representation-based face classification. To this end, we first fit a 3DMM to the 2D face images of a dictionary to reconstruct the 3D shape and texture of each image. The 3D faces are used to render a number of virtual 2D face images with arbitrary pose variations to augment the training data, by merging the original and rendered virtual samples to create an extended dictionary. Second, to reduce the information redundancy of the extended dictionary and improve the sparsity of reconstruction coefficient vectors using collaborative-representation-based classification (CRC), we exploit an on-line class elimination scheme to optimise the extended dictionary by identifying the training samples of the most representative classes for a given query. The final goal is to perform pose-invariant face classification using the proposed dictionary integration method and the on-line pruning strategy under the CRC framework. Experimental results obtained for a set of well-known face datasets demonstrate the merits of the proposed method, especially its robustness to pose variations.
—This letter presents a feature alignment method for domain adaptive Acoustic Scene Classification (ASC) across recording devices. First, we design a two-stream network, in which each stream processes two features, i.e., Log-Mel spec-trogram and delta-deltas, using two sub-networks. Second, we investigate different loss functions for feature alignment between the feature maps obtained by the source and target domains. Last, we present an alternate training strategy to deal with the data imbalance problem between paired and unpaired samples. The experimental results obtained on the DCASE benchmarks demonstrate the effectiveness and superiority of the proposed method. The source code of the proposed method is available at https://github.com/Jingqiao-Zhao/FAASC.
Self-supervised pretraining (SSP) has emerged as a popular technique in machine learning, enabling the extraction of meaningful feature representations without labelled data. In the realm of computer vision, pretrained vision transformers (ViTs) have played a pivotal role in advancing transfer learning. Nonetheless, the escalating cost of finetuning these large models has posed a challenge due to the explosion of model size. This study endeavours to evaluate the effectiveness of pure self-supervised learning (SSL) techniques in computer vision tasks, obviating the need for finetuning, with the intention of emulating human-like capabilities in generalisation and recognition of unseen objects. To this end, we propose an evaluation protocol for zero-shot segmentation based on a prompting patch. Given a point on the target object as a prompt, the algorithm calculates the similarity map between the selected patch and other patches, upon that, a simple thresholding is applied to segment the target. Another evaluation is intra-object and inter-object similarity to gauge discriminatory ability of SSP ViTs. Insights from zero-shot segmentation from prompting and discriminatory abilities of SSP led to the design of a simple SSP approach, termed MMC. This approaches combines Masked image modelling for encouraging similarity of local features, Momentum based self-distillation for transferring semantics from global to local features, and global Contrast for promoting semantics of global features, to enhance discriminative representations of SSP ViTs. Consequently, our proposed method significantly reduces the overlap of intra-object and inter-object similarities, thereby facilitating effective object segmentation within an image. Our experiments reveal that MMC delivers top-tier results in zero-shot semantic segmentation across various datasets.
Effective data augmentation is crucial for facial landmark localisation with Convolutional Neural Networks (CNNs). In this letter, we investigate different data augmentation techniques that can be used to generate sufficient data for training CNN-based facial landmark localisation systems. To the best of our knowledge, this is the first study that provides a systematic analysis of different data augmentation techniques in the area. In addition, an online Hard Augmented Example Mining (HAEM) strategy is advocated for further performance boosting. We examine the effectiveness of those techniques using a regression-based CNN architecture. The experimental results obtained on the AFLW and COFW datasets demonstrate the importance of data augmentation and the effectiveness of HAEM. The performance achieved using these techniques is superior to the state-of-the-art algorithms.
Existing facial age estimation studies have mostly focused on intra-database protocols that assume training and test images are captured under similar conditions. This is rarely valid in practical applications, where we typically encounter training and test sets with different characteristics. In this article, we deal with such situations, namely subjective-exclusive cross-database age estimation. We formulate the age estimation problem as the distribution learning framework, where the age labels are encoded as a probability distribution. To improve the cross-database age estimation performance, we propose a new loss function which provides a more robust measure of the difference between ground-truth and predicted distributions. The desirable properties of the proposed loss function are theoretically analysed and compared with the state-of-the-art approaches. In addition, we compile a new balanced large-scale age estimation database. Last, we introduce a novel evaluation protocol, called subject-exclusive cross-database age estimation protocol, which provides meaningful information of a method in terms of the generalisation capability. The experimental results demonstrate that the proposed approach outperforms the state-of-the-art age estimation methods under both intra-database and subject-exclusive cross-database evaluation protocols. In addition, in this article, we provide a comparative sensitivity analysis of various algorithms to identify trends and issues inherent to their performance. This analysis introduces some open problems to the community which might be considered when designing a robust age estimation system.
3D assisted 2D face recognition involves the process of reconstructing 3D faces from 2D images and solving the problem of face recognition in 3D. To facilitate the use of deep neural networks, a 3D face, normally represented as a 3D mesh of vertices and its corresponding surface texture, is remapped to image-like square isomaps by a conformal mapping. Based on previous work, we assume that face recognition benefits more from texture. In this work, we focus on the surface texture and its discriminatory information content for recognition purposes. Our approach is to prepare a 3D mesh, the corresponding surface texture and the original 2D image as triple input for the recognition network, to show that 3D data is useful for face recognition. Texture enhancement methods to control the texture fusion process are introduced and we adapt data augmentation methods. Our results show that texture-map-based face recognition can not only compete with state-of-the-art systems under the same preconditions but also outperforms standard 2D methods from recent years.
3D Morphable Face Models (3DMM) have been used in pattern recognition for some time now. They have been applied as a basis for 3D face recognition, as well as in an assistive role for 2D face recognition to perform geometric and photometric normalisation of the input image, or in 2D face recognition system training. The statistical distribution underlying 3DMM is Gaussian. However, the single-Gaussian model seems at odds with reality when we consider different cohorts of data, e.g. Black and Chinese faces. Their means are clearly different. This paper introduces the Gaussian Mixture 3DMM (GM-3DMM) which models the global population as a mixture of Gaussian subpopulations, each with its own mean. The proposed GM-3DMM extends the traditional 3DMM naturally, by adopting a shared covariance structure to mitigate small sample estimation problems associated with data in high dimensional spaces. We construct a GM-3DMM, the training of which involves a multiple cohort dataset, SURREY-JNU, comprising 942 3D face scans of people with mixed backgrounds. Experiments in fitting the GM-3DMM to 2D face images to facilitate their geometric and photometric normalisation for pose and illumination invariant face recognition demonstrate the merits of the proposed mixture of Gaussians 3D face model.
Discriminative correlation filter (DCF) has achieved advanced performance in visual object tracking with remarkable efficiency guaranteed by its implementation in the frequency domain. However, the effect of the structural relationship of DCF and object features has not been adequately explored in the context of the filter design. To remedy this deficiency, this paper proposes a Low-rank and Sparse DCF (LSDCF) that improves the relevance of features used by discriminative filters. To be more specific, we extend the classical DCF paradigm from ridge regression to lasso regression, and constrain the estimate to be of low-rank across frames, thus identifying and retaining the informative filters distributed on a low-dimensional manifold. To this end, specific temporal-spatial-channel configurations are adaptively learned to achieve enhanced discrimination and interpretability. In addition, we analyse the complementary characteristics between hand-crafted features and deep features, and propose a coarse-to-fine heuristic tracking strategy to further improve the performance of our LSDCF. Last, the augmented Lagrange multiplier optimisation method is used to achieve efficient optimisation. The experimental results obtained on a number of well-known benchmarking datasets, including OTB2013, OTB50, OTB100, TC128, UAV123, VOT2016 and VOT2018, demonstrate the effectiveness and robustness of the proposed method, delivering outstanding performance compared to the state-of-the-art trackers.
In recent years, Discriminative Correlation Filters (DCFs) have gained popularity due to their superior performance in visual object tracking. However, existing DCF trackers usually learn filters using fixed attention mechanisms that focus on the centre of an image and suppresses filter amplitudes in surroundings. In this paper, we propose an Adaptive Context-Aware Discriminative Correlation Filter (ACA-DCF) that is able to improve the existing DCF formulation with complementary attention mechanisms. Our ACA-DCF integrates foreground attention and background attention for complementary context-aware filter learning. More importantly, we ameliorate the design using an adaptive weighting strategy that takes complex appearance variations into account. The experimental results obtained on several well-known benchmarks demonstrate the effectiveness and superiority of the proposed method over the state-of-the-art approaches.
We propose supervised spatial attention that employs a heatmap generator for instructive feature learning.•We formulate a rectified Gaussian scoring function to generate informative heatmaps.•We present scale-aware layer attention that eliminates redundant information from pyramid features.•A voting strategy is designed to produce more reliable classification results.•Our face detector achieves encouraging performance in accuracy and speed on several benchmarks. Modern anchor-based face detectors learn discriminative features using large-capacity networks and extensive anchor settings. In spite of their promising results, they are not without problems. First, most anchors extract redundant features from the background. As a consequence, the performance improvements are achieved at the expense of a disproportionate computational complexity. Second, the predicted face boxes are only distinguished by a classifier supervised by pre-defined positive, negative and ignored anchors. This strategy may ignore potential contributions from cohorts of anchors labelled negative/ignored during inference simply because of their inferior initialisation, although they can regress well to a target. In other words, true positives and representative features may get filtered out by unreliable confidence scores. To deal with the first concern and achieve more efficient face detection, we propose a Heatmap-assisted Spatial Attention (HSA) module and a Scale-aware Layer Attention (SLA) module to extract informative features using lower computational costs. To be specific, SLA incorporates the information from all the feature pyramid layers, weighted adaptively to remove redundant layers. HSA predicts a reshaped Gaussian heatmap and employs it to facilitate a spatial feature selection by better highlighting facial areas. For more reliable decision-making, we merge the predicted heatmap scores and classification results by voting. Since our heatmap scores are based on the distance to the face centres, they are able to retain all the well-regressed anchors. The experiments obtained on several well-known benchmarks demonstrate the merits of the proposed method.
•A formulation of the DCF design problem which focuses on informative feature channels and spatial structures by means of novel regularisation.•A proposed relaxed optimisation algorithm referred to as R_A-ADMM for optimising the regularised DCF. In contrast with the standard ADMM, the algorithm achieves a better convergence rate.•A temporal smoothness constraint, implemented by an adaptive initialisation mechanism, to achieve further speed up via transfer learning among video frames.•The proposed adoption of AlexNet to construct a light-weight deep representation with a tracking accuracy comparable to more complicated deep networks, such as VGG and ResNet.•An extensive evaluation of the proposed methodology on several well-known visual object tracking datasets, with the results confirming the acceleration gains for the regularised DCF paradigm.
We propose a new Group Feature Selection method for Discriminative Correlation Filters (GFS-DCF) based visual object tracking. The key innovation of the proposed method is to perform group feature selection across both channel and spatial dimensions, thus to pinpoint the structural relevance of multi-channel features to the filtering system. In contrast to the widely used spatial regularisation or feature selection methods, to the best of our knowledge, this is the first time that channel selection has been advocated for DCF-based tracking. We demonstrate that our GFS-DCF method is able to significantly improve the performance of a DCF tracker equipped with deep neural network features. In addition, our GFS-DCF enables joint feature selection and filter learning, achieving enhanced discrimination and interpretability of the learned filters. To further improve the performance, we adaptively integrate historical information by constraining filters to be smooth across temporal frames, using an efficient low-rank approximation. By design, specific temporal-spatial-channel configurations are dynamically learned in the tracking process, highlighting the relevant features, and alleviating the performance degrading impact of less discriminative representations and reducing information redundancy. The experimental results obtained on OTB2013, OTB2015, VOT2017, VOT2018 and TrackingNet demonstrate the merits of our GFS-DCF and its superiority over the state-of-the-art trackers. The code is publicly available at \url{https://github.com/XU-TIANYANG/GFS-DCF}.
In recent years, facial landmark detection – also known as face alignment or facial landmark localisation – has become a very active area, due to its importance to a variety of image and video-based face analysis systems, such as face recognition, emotion analysis, human-computer interaction and 3D face reconstruction. This article looks at the challenges and latest technology advances in facial landmarks.
Any high-dimensional data arising from practical applications usually contains irrelevant features that may impact on the performance of existing subspace clustering methods. This paper proposes a novel subspace clustering method which reconstructs the feature matrix by the means of unsupervised feature selection (UFS) to achieve a better dictionary for subspace clustering (SC). Different from most existing clustering methods, the proposed approach uses the reconstructed feature matrix as the dictionary rather than the original data matrix. As the feature matrix reconstructed by representative features is more discriminative and closer to the ground-truth, it results in improved performance. The corresponding non-convex optimization problem is effectively solved using the half-quadratic and augmented Lagrange multiplier methods. Extensive experiments on four real datasets demonstrate the effectiveness of the proposed method.
3D assisted 2D face recognition involves the process of reconstructing 3D faces from 2D images and solving the problem of face recognition in 3D. To facilitate the use of deep neural networks, a 3D face, normally represented as a 3D mesh of vertices and its corresponding surface texture, is remapped to image-like square isomaps by a conformal mapping. Based on previous work, we assume that face recognition benefits more from texture. In this work, we focus on the surface texture and its discriminatory information content for recognition purposes. Our approach is to prepare a 3D mesh, the corresponding surface texture and the original 2D image as triple input for the recognition network, to show that 3D data is useful for face recognition. Texture enhancement methods to control the texture fusion process are introduced and we adapt data augmentation methods. Our results show that texture-map-based face recognition can not only compete with state-of-the-art systems under the same preconditions but also outperforms standard 2D methods from recent years.
Train Timetable Rescheduling (TTR) is a crucial task in the daily operation of high-speed railways to maintain punctuality and efficiency in the presence of unexpected disturbances. However, it is challenging to promptly create a rescheduled timetable in real time. In this study, we propose a reinforcement-learning-based method for real-time rescheduling of high-speed trains. The key innovation of the proposed method is to learn a well-generalized dispatching policy from a large amount of samples, which can be applied to the TTR task directly. At first, the problem is transformed into a multi-stage decision process, and the decision agent is designed to predict dispatching rules. To enhance the training efficiency, we generate a small yet good-quality action set to reduce invalid explorations. Besides, we propose an action sampling strategy for action selection, which implements forward planning with consideration of evaluation uncertainty, thus improving search efficiency. Extensive experimental results demonstrate the effectiveness and competitiveness of the proposed method. It has been proven that the local policies trained by the proposed method can be applied to numerous problem instances directly, rendering it unnecessary to use human-designed rules.
Recently, deep learning has become the mainstream methodology for Compound-Protein Interaction (CPI) prediction. However, the existing compound-protein feature extraction methods have some issues that limit their performance. First, graph networks are widely used for structural compound feature extraction, but the chemical properties of a compound depend on functional groups rather than graphic structure. Besides, the existing methods lack capabilities in extracting rich and discriminative protein features. Last, the compound-protein features are usually simply combined for CPI prediction, without considering information redundancy and effective feature mining. To address the above issues, we propose a novel CPInformer method. Specifically, we extract heterogeneous compound features, including structural graph features and functional class fingerprints, to reduce prediction errors caused by similar structural compounds. Then, we combine local and global features using dense connections to obtain multi-scale protein features. Last, we apply ProbSparse self-attention to protein features, under the guidance of compound features, to eliminate information redundancy, and to improve the accuracy of CPInformer. More importantly, the proposed method identifies the activated local regions that link a CPI, providing a good visualisation for the CPI state. The results obtained on five benchmarks demonstrate the merits and superiority of CPInformer over the state-of-the-art approaches.
This report presents results from the Video Person Recognition Evaluation held in conjunction with the 11th IEEE International Conference on Automatic Face and Gesture Recognition. Two experiments required algorithms to recognize people in videos from the Point-and-Shoot Face Recognition Challenge Problem (PaSC). The first consisted of videos from a tripod mounted high quality video camera. The second contained videos acquired from 5 different handheld video cameras. There were 1401 videos in each experiment of 265 subjects. The subjects, the scenes, and the actions carried out by the people are the same in both experiments. Five groups from around the world participated in the evaluation. The video handheld experiment was included in the International Joint Conference on Biometrics (IJCB) 2014 Handheld Video Face and Person Recognition Competition. The top verification rate from this evaluation is double that of the top performer in the IJCB competition. Analysis shows that the factor most effecting algorithm performance is the combination of location and action: where the video was acquired and what the person was doing.
To learn disentangled representations of facial images, we present a Dual Encoder-Decoder based Generative Adversarial Network (DED-GAN). In the proposed method, both the generator and discriminator are designed with deep encoder-decoder architectures as their backbones. To be more specific, the encoder-decoder structured generator is used to learn a pose disentangled face representation, and the encoder-decoder structured discriminator is tasked to perform real/fake classification, face reconstruction, determining identity and estimating face pose. We further improve the proposed network architecture by minimizing the additional pixel-wise loss defined by the Wasserstein distance at the output of the discriminator so that the adversarial framework can be better trained. Additionally, we consider face pose variation to be continuous, rather than discrete in existing literature, to inject richer pose information into our model. The pose estimation task is formulated as a regression problem, which helps to disentangle identity information from pose variations. The proposed network is evaluated on the tasks of pose-invariant face recognition (PIFR) and face synthesis across poses. An extensive quantitative and qualitative evaluation carried out on several controlled and in-the-wild benchmarking datasets demonstrates the superiority of the proposed DED-GAN method over the state-of-the-art approaches.
With efficient appearance learning models, Discriminative Correlation Filter (DCF) has been proven to be very successful in recent video object tracking benchmarks and competitions. However, the existing DCF paradigm suffers from two major issues, i.e., spatial boundary effect and temporal filter degradation. To mitigate these challenges, we propose a new DCF-based tracking method. The key innovations of the proposed method include adaptive spatial feature selection and temporal consistent constraints, with which the new tracker enables joint spatial-temporal filter learning in a lower dimensional discriminative manifold. More specifically, we apply structured spatial sparsity constraints to multi-channel filers. Consequently, the process of learning spatial filters can be approximated by the lasso regularisation. To encourage temporal consistency, the filter model is restricted to lie around its historical value and updated locally to preserve the global structure in the manifold. Last, a unified optimisation framework is proposed to jointly select temporal consistency preserving spatial features and learn discriminative filters with the augmented Lagrangian method. Qualitative and quantitative evaluations have been conducted on a number of well-known benchmarking datasets such as OTB2013, OTB50, OTB100, Temple-Colour, UAV123 and VOT2018. The experimental results demonstrate the superiority of the proposed method over the state-of-the-art approaches.
We present a new loss function, namely Wing loss, for robust facial landmark localisation with Convolutional Neural Networks (CNNs). We first compare and analyse different loss functions including L2, L1 and smooth L1. The analysis of these loss functions suggests that, for the training of a CNN-based localisation model, more attention should be paid to small and medium range errors. To this end, we design a piece-wise loss function. The new loss amplifies the impact of errors from the interval (-w, w) by switching from L1 loss to a modified logarithm function. To address the problem of under-representation of samples with large out-of-plane head rotations in the training set, we propose a simple but effective boosting strategy, referred to as pose-based data balancing. In particular, we deal with the data imbalance problem by duplicating the minority training samples and perturbing them by injecting random image rotation, bounding box translation and other data augmentation approaches. Last, the proposed approach is extended to create a two-stage framework for robust facial landmark localisation. The experimental results obtained on AFLW and 300W demonstrate the merits of the Wing loss function, and prove the superiority of the proposed method over the state-of-the-art approaches.
Siamese trackers have become the mainstream framework for visual object tracking in recent years. However, the extraction of the template and search space features is disjoint for a Siamese tracker, resulting in a limited interaction between its classification and regression branches. This degrades the model capacity accurately to estimate the target, especially when it exhibits severe appearance variations. To address this problem, this paper presents a target-cognisant Siamese network for robust visual tracking. First, we introduce a new target-cognisant attention block that computes spatial cross-attention between the template and search branches to convey the relevant appearance information before correlation. Second, we advocate two mechanisms to promote the precision of obtained bounding boxes under complex tracking scenarios. Last, we propose a max filtering module to utilise the guidance of the regression branch to filter out potential interfering predictions in the classification map. The experimental results obtained on challenging benchmarks demonstrate the competitive performance of the proposed method.
In this paper, we propose a novel fitting method that uses local image features to fit a 3D Morphable Face Model to 2D images. To overcome the obstacle of optimising a cost function that contains a non-differentiable feature extraction operator, we use a learning-based cascaded regression method that learns the gradient direction from data. The method allows to simultaneously solve for shape and pose parameters. Our method is thoroughly evaluated on Morphable Model generated data and first results on real data are presented. Compared to traditional fitting methods, which use simple raw features like pixel colour or edge maps, local features have been shown to be much more robust against variations in imaging conditions. Our approach is unique in that we are the first to use local features to fit a 3D Morphable Model. Because of the speed of our method, it is applicable for real-time applications. Our cascaded regression framework is available as an open source library at github.com/patrikhuber/superviseddescent.
3D face reconstruction of shape and skin texture from a single 2D image can be performed using a 3D Morphable Model (3DMM) in an analysis-by-synthesis approach. However, performing this reconstruction (fitting) efficiently and accurately in a general imaging scenario is a challenge. Such a scenario would involve a perspective camera to describe the geometric projection from 3D to 2D, and the Phong model to characterise illumination. Under these imaging assumptions the reconstruction problem is nonlinear and, consequently, computationally very demanding. In this work, we present an efficient stepwise 3DMM-to-2D image-fitting procedure, which sequentially optimises the pose, shape, light direction, light strength and skin texture parameters in separate steps. By linearising each step of the fitting process we derive closed-form solutions for the recovery of the respective parameters, leading to efficient fitting. The proposed optimisation process involves all the pixels of the input image, rather than randomly selected subsets, which enhances the accuracy of the fitting. It is referred to as Efficient Stepwise Optimisation (ESO). The proposed fitting strategy is evaluated using reconstruction error as a performance measure. In addition, we demonstrate its merits in the context of a 3D-assisted 2D face recognition system which detects landmarks automatically and extracts both holistic and local features using a 3DMM. This contrasts with most other methods which only report results that use manual face landmarking to initialise the fitting. Our method is tested on the public CMU-PIE and Multi-PIE face databases, as well as one internal database. The experimental results show that the face reconstruction using ESO is significantly faster, and its accuracy is at least as good as that achieved by the existing 3DMM fitting algorithms. A face recognition system integrating ESO to provide a pose and illumination invariant solution compares favourably with other state-of-the-art methods. In particular, it outperforms deep learning methods when tested on the Multi-PIE database.
Face recognition (FR) using deep convolutional neural networks (DCNNs) has seen remarkable success in recent years. One key ingredient of DCNN-based FR is the design of a loss function that ensures discrimination between various identities. The state-of-the-art (SOTA) solutions utilise normalised Softmax loss with additive and/or multiplicative margins. Despite being popular and effective, these losses are justified only intuitively with little theoretical explanations. In this work, we show that under the LogSumExp (LSE) approximation, the SOTA Softmax losses become equivalent to a proxy-triplet loss that focuses on nearest-neighbour negative proxies only. This motivates us to propose a variant of the proxy-triplet loss, entitled Nearest Proxies Triplet (NPT) loss, which unlike SOTA solutions, converges for a wider range of hyper-parameters and offers flexibility in proxy selection and thus outperforms SOTA techniques. We generalise many SOTA losses into a single framework and give theoretical justifications for the assertion that minimising the proposed loss ensures a minimum separability between all identities. We also show that the proposed loss has an implicit mechanism of hard-sample mining. We conduct extensive experiments using various DCNN architectures on a number of FR benchmarks to demonstrate the efficacy of the proposed scheme over SOTA methods.
The problem of re-identification of people in a crowd com- monly arises in real application scenarios, yet it has received less atten- tion than it deserves. To facilitate research focusing on this problem, we have embarked on constructing a new person re-identification dataset with many instances of crowded indoor and outdoor scenes. This paper proposes a two-stage robust method for pedestrian detection in a complex crowded background to provide bounding box annotations. The first stage is to generate pedestrian proposals using Faster R-CNN and locate each pedestrian using Non-maximum Suppression (NMS). Candidates in dense proposal regions are merged to identify crowd patches. We then apply a bottom-up human pose estimation method to detect individual pedestrians in the crowd patches. The locations of all subjects are achieved based on the bounding boxes from the two stages. The identity of the detected subjects throughout each video is then automatically annotated using multiple features and spatial-temporal clues. The experimental results on a crowded pedestrians dataset demonstrate the effectiveness and efficiency of the proposed method.
This paper proposes a progressive sparse representation-based classification algorithm using local discrete cosine transform (DCT) evaluation to perform face recognition. Specifically, the sum of the contributions of all training samples of each subject is first taken as the contribution of this subject, then the redundant subject with the smallest contribution to the test sample is iteratively eliminated. Second, the progressive method aims at representing the test sample as a linear combination of all the remaining training samples, by which the representation capability of each training sample is exploited to determine the optimal “nearest neighbors” for the test sample. Third, the transformed DCT evaluation is constructed to measure the similarity between the test sample and each local training sample using cosine distance metrics in the DCT domain. The final goal of the proposed method is to determine an optimal weighted sum of nearest neighbors that are obtained under the local correlative degree evaluation, which is approximately equal to the test sample, and we can use this weighted linear combination to perform robust classification. Experimental results conducted on the ORL database of faces (created by the Olivetti Research Laboratory in Cambridge), the FERET face database (managed by the Defense Advanced Research Projects Agency and the National Institute of Standards and Technology), AR face database (created by Aleix Martinez and Robert Benavente in the Computer Vision Center at U.A.B), and USPS handwritten digit database (gathered at the Center of Excellence in Document Analysis and Recognition at SUNY Buffalo) demonstrate the effectiveness of the proposed method.
3D Morphable Face Models (3DMM) have been used in face recognition for some time now. They can be applied in their own right as a basis for 3D face recognition and analysis involving 3D face data. However their prevalent use over the last decade has been as a versatile tool in 2D face recognition to normalise pose, illumination and expression of 2D face images. A 3DMM has the generative capacity to augment the training and test databases for various 2D face processing related tasks. It can be used to expand the gallery set for pose-invariant face matching. For any 2D face image it can furnish complementary information, in terms of its 3D face shape and texture. It can also aid multiple frame fusion by providing the means of registering a set of 2D images. A key enabling technology for this versatility is 3D face model to 2D face image fitting. In this paper recent developments in 3D face modelling and model fitting will be overviewed, and their merits in the context of diverse applications illustrated on several examples, including pose and illumination invariant face recognition, and 3D face reconstruction from video.
Facial landmark localization aims to detect a sparse set of facial fiducial points on a human face, some of which include “eye corner”, “nose tip”, and “chin center”. In the pipeline of face analysis, landmark detectors take the input of a face image and the bounding box provided by face detection, and output a set of coordinates of the predefined landmarks. It provides a fine-grained description of the face topology, such as facial features locations and face region contours, which is essential for many face analysis tasks, e.g., recognition, animation, attributes classification, and face editing. These applications usually run on lightweight devices in uncontrolled environments, requiring landmark detectors to be accurate, robust, and computationally efficient, all at the same time.
Extended sparse representation-based classifcation (ESRC) has shown interesting results on the problem of undersampled face recognition by generating an auxiliary intraclass variant dictionary for the representation of possible appearance variations. However, the method has high computational complexity due to the l1-minimization problem. To address this issue, this paper proposes two strategies to speed up SRC using quadratic optimisation in downsized coefient solution subspace. The frst one, namely Fast SRC using Quadratic Optimisation (FSRC-QO), applies PCA and LDA hybrid constrained optimisation method to achieve compressed linear representations of test samples. By design, more accurate and discriminative reconstruction of a test sample can be achieved for face classifcation, using the downsized coefficient space. Secondly, to explore the positive impact of our proposed method on deep-learning-based face classifcation, we enhance FSRC-QO using CNN-based features (FSRC-QO-CNN), in which we replace the original input image using robust CNN features in our FSRC-QO framework. Experimental results conducted on a set of well known face datasets, including AR, FERET, LFW and FRGC, demonstrate the merits of the proposed methods, especially in computational efficiency.
Animal pose estimation has received increasing attention in recent years. The main challenge for this task is the diversity of animal species compared to their human counterpart. To address this issue, we design a keypoint-interactive Transformer model for high-resolution animal pose estimation, namely KITPose. Since a high-resolution network maintains local perception and the self-attention module in Transformer is an expert in connecting long-range dependencies, we equip the high-resolution network with a Transformer to enhance the model capacity, achieving keypoints interaction in the decision stage. Besides, to smoothly fit the pose estimation task, we simultaneously train the model parameters and joint weights, which can automatically adjust the loss weight for each specific keypoint. The experimental results obtained on the AP10K and ATRW datasets demonstrate the merits of KITPose, as well as its superior performance over the state-of-the-art approaches.
This paper presents a new Self-growing and Pruning Generative Adversarial Network (SP-GAN) for realistic image generation. In contrast to traditional GAN models, our SPGAN is able to dynamically adjust the size and architecture of a network in the training stage, by using the proposed selfgrowing and pruning mechanisms. To be more specific, we first train two seed networks as the generator and discriminator, each only contains a small number of convolution kernels. Such small-scale networks are much easier and faster to train than large-capacity networks. Second, in the self-growing step,we replicate the convolution kernels of each seed network to augment the scale of the network, followed by fine-tuning the augmented/expanded network. More importantly, to prevent the excessive growth of each seed network in the self-growing stage, we propose a pruning strategy that reduces the redundancy of an augmented network, yielding the optimal scale of the network. Last, we design a new adaptive loss function that is treated as a variable loss computational process for the training of the proposed SP-GAN model. By design, the hyperparameters of the loss function can dynamically adapt to different training stages. Experimental results obtained on a set of datasets demonstrate the merits of the proposed method, especially in terms of the stability and efficiency of network training. The source code of the proposed SP-GAN method is publicly available at https://github.com/Lambert-chen/SPGAN.git.
Recently, word enhancement has become very popular for Chinese Named Entity Recognition (NER), reducing segmentation errors and increasing the semantic and boundary information of Chinese words. However, these methods tend to ignore the information of the Chi-nese character structure after integrating the lexical information. Chinese characters have evolved from pictographs since ancient times, and their structure often reflects more information about the characters. This paper presents a novel Multi-metadata Embedding based Cross-Transformer (MECT) to improve the performance of Chinese NER by fusing the structural information of Chinese characters. Specifically , we use multi-metadata embedding in a two-stream Transformer to integrate Chinese character features with the radical-level embedding. With the structural characteristics of Chinese characters, MECT can better capture the semantic information of Chinese characters for NER. The experimental results obtained on several well-known benchmarking datasets demonstrate the merits and superiority of the proposed MECT method.
Active appearance model is a statistically parametrical model, which is widely used to extract human facial features and recognition. However, intensity values used in original AAM cannot provide enough information for image texture, which will lead to a larger error or a failure fitting of AAM. In order to overcome these defects and improve the fitting performance of AAM model, an improved texture representation is proposed in this paper. Firstly, translation invariant wavelet transform is performed on face images and then image structure is represented using the measure which is obtained by fusing the low-frequency coefficients with edge intensity. Experimental results show that the improved algorithm can increase the accuracy of the AAM fitting and express more information for structures of edge and texture.
•Propose a novel decision-based black-box adversarial attack method.•Employ the attention mechanism to improve evolutionary attack.•Generate more indistinguishable perturbations with limited queries. In recent years, face recognition has achieved promising results along with the development of advanced Deep Neural Networks (DNNs). The existing face recognition systems are vulnerable to adversarial examples, which brings potential security risks. Evolutionary Attack (EA) has been successfully used to fool face recognition by inducing a minimum perturbation to a face image with few queries. However, EA employs the global information of face images but ignores their local characteristics. In addition, restricting the ℓ2-norm of adversarial perturbations hinders the diversity of adversarial perturbations. To solve the above problems, we propose Attention-guided Evolutionary Attack with Elastic-Net Regularization (ERAEA) for attacking face recognition. ERAEA extracts local facial characteristics by attention mechanism, effectively improving the attack effect and image perception quality. In particular, ERAEA adopts an attention mechanism to guide evolutionary direction, which operates on the covariance matrix as it contains crucial information about the evolutionary path. Furthermore, we design an adaptive elastic-net regularization to diversify the adversarial perturbation, accelerating the optimization performance. Extensive experiments obtained on three benchmarks demonstrate that our proposed method achieves better perturbation norm than the state-of-the-art methods with limited queries on face recognition and generates adversarial face images with higher perceptual quality. Besides, ERAEA requires fewer queries to achieve a fixed adversarial perturbation norm.
TP391; 药物一般通过抑制或激活人体中某些蛋白活性反应进而发挥效能,因此预测蛋白和药物的相互作用对新药开发的筛选工作极为关键.然而,基于传统方法在湿实验中进行该类实验需要耗费巨大的人力和物力.为解决这一问题,提出了一种基于自注意力机制和多药物特征融合的蛋白质-药物相互作用预测算法.首先,合理融合基于药物分子结构特征的Morgan指纹、Mol2Vec表示向量以及消息传递网络所提特征;随后,将融合结果对由密集型卷积所提取的蛋白特征做注意力加权;接着综合两者特征,利用 自注意力机制和双向门控循环单元预测蛋白质药物相互作用;最后,根据训练模型设计了可应用的预测系统,并展示了其在筛选治愈阿尔兹海默症药物的具体使用方法和效果.实验结果表明,较现有的预测方法,新方法在BindingDB,Kinase,Human,C.elegans数据集上均达到了更好的预测 效果.最优的AUC分别达到了 0.963,0.937,0.983,0.990,较同类方法具有十分明显的优势.
Video anomaly detection is crucial for behavior analysis, which has witnessed continuous progress in recent years with the auto-encoder based reconstruction framework. However, in some cases, abnormal frames may also be reconstructed well due to the strong representation ability of deep networks, increasing missed detection. To mitigate this issue, the existing methods usually the memory bank method. This method records normal patterns and assigns high errors for the reconstruction of abnormal frames into normal frames. In this paper, to better use the semantic information of normal videos recorded in the memory module, we introduce the Memory-Token Transformer (MTT) to boost the reconstruction performance on normal frames. We assume that the anomalies in a video mainly concentrate on the regions containing people and relevant objects. Therefore, during the decoding stage, we first extract the semantic concepts of a feature map and generate the corresponding semantic tokens. Then the tokens are combined with the proposed memory module. Last, we introduce a transformer to fuse the complex relationship among different tokens, and use 3D convolution with the pooling operator in our encoder to enhance spatio-temporal feature extraction as compared with 2D models. The experimental results obtained on various benchmarks demonstrate the effectiveness of the proposed method.
Recently, word enhancement has become very popular for Chinese Named Entity Recognition (NER), reducing segmentation errors and increasing the semantic and boundary information of Chinese words. However, these methods tend to ignore the information of the Chinese character structure after integrating the lexical information. Chinese characters have evolved from pictographs since ancient times, and their structure often reflects more information about the characters. This paper presents a novel Multi-metadata Embedding based Cross-Transformer (MECT) to improve the performance of Chinese NER by fusing the structural information of Chinese characters. Specifically, we use multi-metadata embedding in a two-stream Transformer to integrate Chinese character features with the radical-level embedding. With the structural characteristics of Chinese characters, MECT can better capture the semantic information of Chinese characters for NER. The experimental results obtained on several well-known benchmarking datasets demonstrate the merits and superiority of the proposed MECT method.\footnote{The source code of the proposed method is publicly available at https://github.com/CoderMusou/MECT4CNER.
This paper proposes a two-step subspace learning framework by combining non-linear kernel PCA (KPCA) and with contextual constraints based linear discriminant analysis (CCLDA) for face recognition. The linear CCLDA approach does not consider the higher order non-linear information in facial images, whereas the wide face variations posed by some factors, such as viewpoint, illumination and expression, existing in nonlinear subspaces may lead to many difficulties in face recognition and classification problems. To counteract the above problem, we incorporate the contextual information into kernel discriminant analysis by using KPCA in a two-step process, which provides more useful information for face recognition and classification. Experimental results on three well-known face databases, ORL, Yale and XM2VTS, validate the effectiveness of the proposed method. (4 pages)
Recently, prompt-based learning has gained popularity across many natural language processing (NLP) tasks by reformulating them into a cloze-style format to better align pre-trained language models (PLMs) with downstream tasks. However, applying this approach to relation classification poses unique challenges. Specifically, associating natural language words that fill the masked token with semantic relation labels (\textit{e.g.} \textit{``org:founded\_by}'') is difficult. To address this challenge, this paper presents a novel prompt-based learning method, namely LabelPrompt, for the relation classification task. Motivated by the intuition to ``GIVE MODEL CHOICES!'', we first define additional tokens to represent relation labels, which regard these tokens as the verbaliser with semantic initialisation and explicitly construct them with a prompt template method. Then, to mitigate inconsistency between predicted relations and given entities, we implement an entity-aware module with contrastive learning. Last, we conduct an attention query strategy within the self-attention layer to differentiates prompt tokens and sequence tokens. Together, these strategies enhance the adaptability of prompt-based learning, especially when only small labelled datasets is available. Comprehensive experiments on benchmark datasets demonstrate the superiority of our method, particularly in the few-shot scenario.
ASM (Active Shape Model) and AAM (Active Appearance Model) model are both parametric model based on statistics. We can locate the key points of a face by AAM model accurately. There is a shape model both in the ASM and AAM. When we build the ASM model and AAM model, we must align the shapes of the training set to a unified framework. In the original alignment process, there are only scale, rotation and translation transformation. In order to improve the accuracy of the alignment, we propose a new algorithm with shear transformation besides the original geometric transformations. At last, the experimental results indicate that the new algorithm is effective for the alignment of the shape model.
Recently, deep learning has become the mainstream methodology for Drug-Target binding Affinity (DTA) prediction. However, two deficiencies of the existing methods restrict their practical applications. On the one hand, most existing methods ignore the individual information of sequence elements, resulting in poor sequence feature representations. On the other hand, without prior biological knowledge, the prediction of drug-target binding regions based on attention weights of a deep neural network could be difficult to verify, which may bring adverse interference to biological researchers. We propose a novel Multi-Functional and Robust Drug-Target binding Affinity prediction (MFR-DTA) method to address the above issues. Specifically, we design a new biological sequence feature extraction block, namely BioMLP, that assists the model in extracting individual features of sequence elements. Then, we propose a new Elem-feature fusion block to refine the extracted features. After that, we construct a Mix-Decoder block that extracts drug-target interaction information and predicts their binding regions simultaneously. Last, we evaluate MFR-DTA on two benchmarks consistently with the existing methods and propose a new dataset, sc-PDB, to better measure the accuracy of binding region prediction. We also visualise some samples to demonstrate the locations of their binding sites and the predicted multi-scale interaction regions. The proposed method achieves excellent performance on these datasets, demonstrating its merits and superiority over the state-of-the-art methods. https://github.com/JU-HuaY/MFR. Supplementary data are available at Bioinformatics online.
Traditional collaborative representation based classification (CRC) method usually faces the challenge of data uncertainty hence results in poor performance, especially in the presence of appearance variations in pose, expression and illumination. To overcome this issue, this paper presents a CRC-based face classification method by jointly using block weighted LBP and analysis dictionary learning. To this end, we first design a block weighted LBP histogram algorithm to form a set of local histogram-based feature vectors instead of using raw images. By this means we are able to effectively decrease data redundancy and uncertainty derived from image noises and appearance variations. Second, we adopt an analysis dictionary learning model as the projection transform to construct an analysis subspace, in which a new sample is characterized with the improved sparsity of its reconstruction coefficient vector. The crucial role of the analysis dictionary learning method in CRC is revealed by its capacity of the collaborative representation in an analytic coefficient space. Extensive experimental results conducted on a set of well-known face databases demonstrate the merits of the proposed method.
Graph convolutional networks (GCN) have attracted increasing interest in action recognition in recent years. GCN models human skeleton sequences as spatio-temporal graphs. Also, attention mechanisms are often jointly used with GCNs to highlight important frames or body joints in a sequence. However, attention modules learn parameters offline and are fixed, so may not adapt well to various action samples. In this paper, we propose a simple but effective motion-driven spatial and temporal adaptation strategy to dynamically strengthen the features of important frames and joints for skeleton-based action recognition. The rationale is that the joints and frames with dramatic motions are generally more informative and discriminative. We decouple and combine the spatial and temporal refinements by using a two-branch structure, in which the joint and frame-wise feature refinements perform in parallel. Such a structure can also lead to learn more complementary feature representations. Moreover, we propose to use the fully connected graph convolution to learn the long-range spatial dependencies. Besides, we investigate two high-resolution skeleton graphs by creating virtual joints, aiming to improve the representation of skeleton features. By combining the above proposals, we develop a novel motion-driven spatial and temporal adaptive high-resolution GCN. Experimental results demonstrate that the proposed model achieves state-of-the-art (SOTA) results on the challenging large-scale Kinetics-Skeleton and UAV-Human datasets, and it is on par with the SOTA methods on the two NTU-RGB+D 60\&120 datasets. Additionally, our motion-driven adaptation method shows encouraging performance when compared with the attention mechanisms.
In this study, we present a new sparse-representation-based face-classification algorithm that exploits dynamic dictionary optimization on an extended dictionary using synthesized faces. More specifically, given a dictionary consisting of face examples, we first augment the dictionary with a set of virtual faces generated by calculating the image difference of a pair of faces. This results in an extended dictionary with hybrid training samples, which enhances the capacity of the dictionary to represent new samples. Second, to reduce the redundancy of the extended dictionary and improve the classification accuracy, we use a dictionary-optimization method. We truncate the extended dictionary with a more compact structure by discarding the original samples with small contributions to represent a test sample. Finally, we perform sparserepresentation- based face classification using the optimized dictionary. Experimental results obtained using the AR and FERRET face datasets demonstrate the superiority of the proposed method in terms of accuracy, especially for small-sample-size problems.
Traditional discriminant analysis (DA) methods are usually not amenable to being studied only with a few or even single facial image per subject. The fundamental reason lies in the fact that the traditional DA approaches cannot fully reflect the variations of a query sample with illumination, occlusion and pose variations, especially in the case of small sample size. In this paper, we develop a multi-scale fuzzy sparse discriminant analysis using a local third-order tensor model to perform robust face classification. More specifically, we firstly introduced a local third-order tensor model of face images to exploit a set of multi-scale characteristics of the Ridgelet transform. Secondly, a set of Ridgelet transformed coefficients with respect to each block from a face image are respectively generated. We then merge all these coefficients to form a new representative vector for the image. Lastly, we evaluate the sparse similarity grade between each training sample and class by constructing a sparse similarity metric, and redesign the traditional discriminant criterion that contains considerable fuzzy sparse similarity grades to perform robust classification. Experimental results conducted on a set of well-known face databases demonstrate the merits of the proposed method, especially in the case of insufficient training samples.
This paper presents a half-face dictionary integration (HFDI) algorithm for representation-based classification. The proposed HFDI algorithm measures residuals between an input signal and the reconstructed one, using both the original and the synthesized dual-column (row) half-face training samples. More specifically, we first generate a set of virtual half-face samples for the purpose of training data augmentation. The aim is to obtain high-fidelity collaborative representation of a test sample. In this half-face integrated dictionary, each original training vector is replaced by an integrated dual-column (row) half-face matrix. Second, to reduce the redundancy between the original dictionary and the extended half-face dictionary, we propose an elimination strategy to gain the most robust training atoms. The last contribution of the proposed HFDI method is the use of a competitive fusion method weighting the reconstruction residuals from different dictionaries for robust face classification. Experimental results obtained from the Facial Recognition Technology, Aleix and Robert, Georgia Tech, ORL, and Carnegie Mellon University-pose, illumination and expression data sets demonstrate the effectiveness of the proposed method, especially in the case of the small sample size problem.