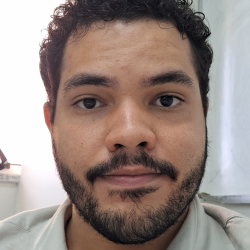
Rafael Bessa Loureiro
Academic and research departments
Surrey Institute for People-Centred Artificial Intelligence (PAI), Centre for Vision, Speech and Signal Processing (CVSSP), Institute for Sustainability.About
My research project
Sustainable Foundation Models for Time Series Processing Applied to SustainabilityThis research focuses on developing sustainable AI foundation models for time series processing to address key sustainability challenges, including weather prediction, clean air monitoring, renewable energy forecasting, and climate change analysis. While foundation models have revolutionised natural language processing and computer vision, their potential in sustainability remains underexplored.
By leveraging their ability to learn deep patterns from multimodal datasets, this project aims to create AI-driven solutions that support the UN’s Sustainable Development Goals (SDGs). The research benefits from an interdisciplinary environment that foster trustworthy, responsible, and inclusive AI, collaborating with academia, industry, and policymakers to develop innovative solutions for global challenges.
Supervisors
This research focuses on developing sustainable AI foundation models for time series processing to address key sustainability challenges, including weather prediction, clean air monitoring, renewable energy forecasting, and climate change analysis. While foundation models have revolutionised natural language processing and computer vision, their potential in sustainability remains underexplored.
By leveraging their ability to learn deep patterns from multimodal datasets, this project aims to create AI-driven solutions that support the UN’s Sustainable Development Goals (SDGs). The research benefits from an interdisciplinary environment that foster trustworthy, responsible, and inclusive AI, collaborating with academia, industry, and policymakers to develop innovative solutions for global challenges.
My qualifications
Master’s Dissertation: Computational method for grouping and reducing representative metrics for identification and mitigation of bias and unfairness in machine learning models.
Publications
One of the difficulties of artificial intelligence is to ensure that model decisions are fair and free of bias. In research, datasets, metrics, techniques, and tools are applied to detect and mitigate algorithmic unfairness and bias. This study examines the current knowledge on bias and unfairness in machine learning models. The systematic review followed the PRISMA guidelines and is registered on OSF plataform. The search was carried out between 2021 and early 2022 in the Scopus, IEEE Xplore, Web of Science, and Google Scholar knowledge bases and found 128 articles published between 2017 and 2022, of which 45 were chosen based on search string optimization and inclusion and exclusion criteria. We discovered that the majority of retrieved works focus on bias and unfairness identification and mitigation techniques, offering tools, statistical approaches, important metrics, and datasets typically used for bias experiments. In terms of the primary forms of bias, data, algorithm, and user interaction were addressed in connection to the preprocessing, in-processing, and postprocessing mitigation methods. The use of Equalized Odds, Opportunity Equality, and Demographic Parity as primary fairness metrics emphasizes the crucial role of sensitive attributes in mitigating bias. The 25 datasets chosen span a wide range of areas, including criminal justice image enhancement, finance, education, product pricing, and health, with the majority including sensitive attributes. In terms of tools, Aequitas is the most often referenced, yet many of the tools were not employed in empirical experiments. A limitation of current research is the lack of multiclass and multimetric studies, which are found in just a few works and constrain the investigation to binary-focused method. Furthermore, the results indicate that different fairness metrics do not present uniform results for a given use case, and that more research with varied model architectures is necessary to standardize which ones are more appropriate for a given context. We also observed that all research addressed the transparency of the algorithm, or its capacity to explain how decisions are taken.
The majority of current approaches for bias and fairness identification or mitigation in machine learning models are applications for a particular issue that fails to account for the connection between the application context and its associated sensitive attributes, which contributes to the recognition of consistent patterns in the application of bias and fairness metrics. This can be used to drive the development of future models, with the sensitive attribute acting as a connecting element to these metrics. Hence, this study aims to analyze patterns in several metrics for identifying bias and fairness, applying the gender-sensitive attribute as a case study, for three different areas of applications in machine learning models: computer vision, natural language processing, and recommendation systems. The gender attribute case study has been used in computer vision, natural language processing, and recommendation systems. The method entailed creating use cases for facial recognition in the FairFace dataset, message toxicity in the Jigsaw dataset, and movie recommendations in the MovieLens100K dataset, then developing models based on the VGG19, BERT, and Wide Deep architectures and evaluating them using the accuracy, precision, recall, and F1-score classification metrics, as well as assessing their outcomes using fourteen fairness metrics. Certain metrics disclosed bias and fairness, while others did not, revealing a consistent pattern for the same sensitive attribute across different application domains, and similarities for the statistical parity, PPR disparity, and error disparity metrics across domains, indicating fairness related to the studied sensitive attribute. Some attributes, on the other hand, did not follow this pattern. As a result, we conclude that the sensitive attribute may play a crucial role in defining the fairness metrics for a specific context.
Addressing bias and unfairness in machine learning models in different application domains is a multifaceted challenge. Despite the variety of fairness metrics available, identifying an optimal set for evaluating a model’s fairness is still an open question due to the diverse nature of these metrics and the lack of a comprehensive approach for ensuring fairness across different applications. This study aims to propose a method that allows the selection of the most representative metrics for bias and fairness assessment, in post-processing for machine learning models in different contexts. We delve into the use of a correlation-based strategy as a heuristic for fairness metric selection, applying bootstrap sampling using the Markov chain Monte Carlo technique, with our proposed improvements, including stratified sampling, stopping criterion, and Kendall correlation, to address the data bias representation, the computational cost, and the robustness, respectively. An average decrease of 64.37% in the number of models and of 20.00% in processing time was achieved. Moreover, the proposed method effectively paired metrics with similar behaviour, highlighting the presence of a similar term as a strong indicator of a direct relationship. While no standout metric emerges across all contexts, within specific models or datasets, certain metrics consistently stand out. In a complex scenario using a large language model for sexism detection, the proposed method achieved a 71.93% reduction in execution time while forming more comprehensive metric groups. Overall, the proposed method successfully selects the representative metric with a considerable gain in computational costs, demonstrating its practicality for real-world applications.