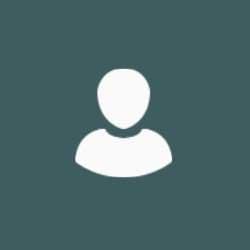
Professor Philip Aston
Academic and research departments
Mathematics at the Interface Group, Centre for Mathematical and Computational Biology, School of Mathematics and Physics.About
Biography
- BSc Mathematics and Computer Science (First class honours), Brunel University, 1979-1983
- PhD, supervisor Prof John Whiteman, Brunel University, 1983-1986
- SERC-funded postdoc working with Prof John Toland and Prof Alastair Spence, University of Bath, 1986-1989
- Department of Mathematics, University of Surrey, 1989-
- Joint appointment with the Data Science group at NPL, Teddington, 2018-
ResearchResearch interests
My research interests include bifurcation theory, symmetry, computation of Lyapunov exponents using spatial integration, the dynamics of bouncing balls, pharmacokinetics/pharmacodynamics (PKPD), non-exponential radioactive decay, mathematical models of hepatitis C infection and attractor reconstruction methods for extracting information from physiological time series, machine learning.
Further details can be found on my personal web page.
Research interests
My research interests include bifurcation theory, symmetry, computation of Lyapunov exponents using spatial integration, the dynamics of bouncing balls, pharmacokinetics/pharmacodynamics (PKPD), non-exponential radioactive decay, mathematical models of hepatitis C infection and attractor reconstruction methods for extracting information from physiological time series, machine learning.
Further details can be found on my personal web page.
Publications
Feature importance methods promise to provide a ranking of features according to importance for a given classification task. A wide range of methods exist but their rankings often disagree and they are inherently difficult to evaluate due to a lack of ground truth beyond synthetic datasets. In this work, we put feature importance methods to the test on real-world data in the domain of cardiology, where we try to distinguish three specific pathologies from healthy subjects based on ECG features comparing to features used in cardiologists' decision rules as ground truth. We found that the SHAP and LIME methods and Chi-squared test all worked well together with the native Random forest and Logistic regression feature rankings. Some methods gave inconsistent results, which included the Maximum Relevance Minimum Redundancy and Neighbourhood Component Analysis methods. The permutation-based methods generally performed quite poorly. A surprising result was found in the case of left bundle branch block, where T-wave morphology features were consistently identified as being important for diagnosis, but are not used by clinicians.
A recent UK report highlighted the fact that measurements of blood oxygenation using pulse oximeters may be inaccurate for patients with darker skin tones. The photo-plethysmography signals collected by pulse oximeters and a multitude of wearable devices are often combined with machine learning to derive physiological parameters that may be useful for health monitoring. So it is natural to question whether machine learning accuracy when using photoplethysmography signals as input can also lead to inaccurate results for darker skin tones, particularly when a model may be trained on data from subjects with predominantly lighter skin tones. To test this, we chose a binary classification problem of classifying systolic blood pressure as high (≥ 140 mm Hg) or not high (< 140 mm Hg). We used the Aurora BP dataset which includes photoplethysmography signals, blood pressure readings and Fitzpatrick skin tone classification. We trained a model using only skin tone class 1 data (lightest skin tone) and then tested all the other skin tone data using this model. We found that the accuracy dropped for all the other skin tone classes. We then trained a model using data from all skin tones and the accuracy for the darker skin tones improved except class 5.
Many methods have been proposed to detect beats in photoplethysmogram (PPG) signals. We present a novel method which uses the Symmetric Projection Attractor Reconstruction (SPAR) method to generate an attractor in a two dimensional phase space from the PPG signal. We can then define a line through the origin of this phase space to be a Poincaré section, as is commonly used in dynamical systems. Beats are detected when the attractor trajectory crosses the Poincaré section. By considering baseline drift, we define an optimal Poincaré section to use. The performance of this method was assessed using the WESAD dataset, achieving median F1 scores of 74.3% in the Baseline phase, 63.0% during Stress, 93.6% during Amusement, and 97.7% during Meditation. Performance was better than an earlier version of the method, and comparable to one of the best algorithms identified in a recent benchmarking study of 15 beat detection algorithms. In addition, our method performed better than two others in the accuracy of the inter-beat intervals for two resting subjects.
AbstractMachine learning (ML) methods for the analysis of electrocardiography (ECG) data are gaining importance, substantially supported by the release of large public datasets. However, these current datasets miss important derived descriptors such as ECG features that have been devised in the past hundred years and still form the basis of most automatic ECG analysis algorithms and are critical for cardiologists’ decision processes. ECG features are available from sophisticated commercial software but are not accessible to the general public. To alleviate this issue, we add ECG features from two leading commercial algorithms and an open-source implementation supplemented by a set of automatic diagnostic statements from a commercial ECG analysis software in preprocessed format. This allows the comparison of ML models trained on clinically versus automatically generated label sets. We provide an extensive technical validation of features and diagnostic statements for ML applications. We believe this release crucially enhances the usability of the PTB-XL dataset as a reference dataset for ML methods in the context of ECG data.
BackgroundObstructive Sleep Apnoea (OSA) is conventionally quantified by the Apnoea-Hypopnea Index (AHI), used to classify disease severity. Automation of AHI detection relies on identification of singular data points in long, multi-channel polysomnography (PSG) recordings. This can be easily compromised by signal noise. We present a novel mathematical method, the Symmetric Projection Attractor Reconstruction (SPAR), that may overcome this problem by transforming whole cyclic physiological recordings into corresponding ‘at-a-glance’ images (‘attractors’) which capture all available waveform morphology information, without relying on single point detection. Attractor quantification may provide a more rapid and robust mean of quantifying the number and duration of overnight apnoeic and hypopneic events.AimTo test whether SPAR can categorize overnight obstructive sleep apnoea recordings according to severity classifications informed by expert-annotated AHI.Methods74 PSG recordings were analysed (52 non-OSA subjects/22 severe-OSA patients, 43.0/27.3% female, 37.4/48.9 average y.o.). Abdominal-band motion data was chosen for pilot analysis due to its relatively low complexity of waveform morphology. Data were processed through bespoke SPAR software to create SPAR attractors. Quantification of high central attractor density corresponded to low/no-amplitude waveform regions, serving as a surrogate for the number and length of apnoeic and hypopneic events. This metric was used to classify between non-OSA subjects (AHI 30/hour). Receiver Operator Characteristics Area Under the Curve (ROC AUC) was used to measure classification accuracy.ResultsSPAR attractors created from 3 continuous hours of overnight abdominal-band data visually differentiated non-OSA and severe OSA patients, showing a high-density central area in the severe-OSA group (figure 1) which was absent in the non-OSA group. Quantification of attractor central density classified these two groups with high accuracy (ROC AUC = 0.99).Abstract P102 Figure 1Overnight 5-minute abdominal movement data and corresponding 3-hour SPAR attractor from a representative non-OSA subject (top, low central attractor density) and severe-OSA patient (bottom, high central attractor density). Y axes on traces shown as ±105 arbitary units[Figure omitted. See PDF]ConclusionSPAR analysis of abdominal-band motion recordings accurately classified non-OSA vs. severe OSA patients. Further analysis will apply SPAR to categorise all types of OSA severities, and test SPAR’s ability to serve as a novel, visual, OSA triage tool. Future studies will compare SPAR to automated AHI scoring in various PSG signals to ascertain if the method could benefit the efficiency of current diagnostic processes.
The electrocardiogram (ECG) appears highly individual in nature. By applying the Symmetric Projection Attractor Reconstruction (SPAR) method, we obtain a unique visualisation of an individual's ECG and show how the subtle inter- and intra-individual differences observed may be quantified. This preliminary study supports further development of the novel SPAR approach for patient stratification and monitoring.
We consider period-doubling cascades in two-dimensional iterated maps. We define forward and backward period-doubling bifurcations, and use these concepts to describe an alternating period-doubling cascade in which forward and backward period-doubling bifurcations alternate. By tracking the eigenvalues of a typical map throughout such cascades we show that two-dimensional maps may give rise to two qualitatively different alternating period-doubling cascades. We apply renormalisation theory to one class of alternating period-doubling cascades, and derive universal spatial scalings for such cascades from fixed points of the appropriate renormalisation operator. We also derive universal parameter scalings for these cascades from the eigenvalues of the linearisation of the renormalisation operator, and provide the corresponding eigenfunctions. The theory is illustrated by an example.
Targeting methods appropriate for systems with discontinuities are considered. The multivalued inverse function is used to generate multiple preimages of the target region which quickly cover the attractor. This method is applied to the current-controlled boost converter in order to jump between two controlled states. A significant reduction in the length of the target orbit is observed when compared with targeting methods for invertible maps.
We consider the possibility of free receptor (antigen/cytokine) levels rebounding to higher than the baseline level after one or more applications of an antibody drug using a target-mediated drug disposition model. Using geometry and dynamical systems analysis, we show that rebound will occur if and only if the elimination rate of the drug-receptor product is slower than the elimination rates of the drug and of the receptor. We also analyse the magnitude of rebound through approximations and simulations and demonstrate that it increases if the drug dose increases or if the difference between the elimination rate of the drug-receptor product and the minimum of the elimination rates of the drug and of the receptor increases.
Transfer learning offers a potentially valuable tool for detecting a pathological electrocardiogram (ECG) trace. Here, four separate methods of generating an image from a single ECG trace are presented. Transfer learning is used to train three deep neural networks for classification of these images into healthy or pathological categories. The performance of the three pretrained networks is compared.
Cardiologists have been using electrocardiogram features to diagnose a wide variety of heart conditions for many decades. There are also numerous algorithms that rank feature importance for a particular classification task. However, different algorithms often give quite different feature rankings. Therefore, we compared the feature importance rankings obtained by various algorithms with the features that cardiologists use for diagnosis.
Ankle brachial pressure index (ABPI) is the first-line test to diagnose peripheral artery disease (PAD). Its adoption in clinical practice is poor and its validity, particularly in diabetes, is limited. We hypothesised that ABPI can be accurately and precisely estimated based on cuffless Doppler waveforms. Retrospective analysis of standard ABPI and handheld Doppler waveform characteristics (n = 200). Prospective analysis of angle-corrected Doppler acceleration index (AccI, n = 148) and standard ABPI with testing of performance to diagnose PAD as assessed with imaging reference standards in consecutive patients. The highest AccI from handheld Doppler at ankle arteries was significantly logarithmically associated with the highest standard ABPI (E[y] = 0.32 ln [1.71 ∗ x + 1], p < 0.001, R2 = 0.68, n = 100 limbs). Estimated ABPI (eABPI) based on AccI closely resembled ABPI (r = 0.81, p < 0.001, average deviation −0.01 ± 0.13 [SD], n = 100 limbs). AccI from angle-corrected Doppler in patients without overt media sclerosis (ABPI ≤ 1.1) improved ABPI prediction (E[y] = 0.297 ∗ ln[0.039 ∗ x + 1], R2 = 0.92, p = 0.006, average deviation 0.00 ± 0.08, n = 100). In a population (n = 148 limbs) including diabetes (56%), chronic limb-threatening ischaemia (51%) and media sclerosis (32%), receiver operating characteristics analysis of (angle-corrected) eABPI performed significantly better than standard ABPI to diagnose PAD defined by ultrasound (ROC AUC = 0.99 ± 0.01, p < 0.001; sensitivity: 97%, specificity: 96%) at the ≤0.9 cut-off. This was confirmed with CT angiography (ROC AUC = 0.98, p < 0.001, sensitivity: 97%, specificity: 100%) and was independent of the presence of diabetes (p = 0.608). ABPI can be estimated based on ankle Doppler AccI without compression, and eABPI performs better than standard ABPI to diagnose PAD independent of diabetes. eABPI has the potential to be included as a standard component of lower extremity ultrasound.
Background Life-threatening arrhythmias resulting from genetic mutations are often missed in current electrocardiogram (ECG) analysis. We combined a new method for ECG analysis that uses all the waveform data with machine learning to improve detection of such mutations from short ECG signals in a mouse model. Objective We sought to detect consequences of Na+ channel deficiencies known to compromise action potential conduction in comparisons of Scn5a+/- mutant and wild-type mice using short ECG signals, examining novel and standard features derived from lead I and II ECG recordings by machine learning algorithms. Methods Lead I and II ECG signals from anesthetized wild-type and Scn5a+/- mutant mice of length 130 seconds were analyzed by extracting various groups of features, which were used by machine learning to classify the mice as wild-type or mutant. The features used were standard ECG intervals and amplitudes, as well as features derived from attractors generated using the novel Symmetric Projection Attractor Reconstruction method, which reformulates the whole signal as a bounded, symmetric 2-dimensional attractor. All the features were also combined as a single feature group. Results Classification of genotype using the attractor features gave higher accuracy than using either the ECG intervals or the intervals and amplitudes. However, the highest accuracy (96%) was obtained using all the features. Accuracies for different subgroups of the data were obtained and compared. Conclusion Detection of the Scn5a+/- mutation from short mouse ECG signals with high accuracy is possible using our Symmetric Projection Attractor Reconstruction method.
The aim of this work is to distinguish between wild-type mice and Scn5a +/- mutant mice using short ECG signals. This mutation results in impaired cardiac sodium channel function and is associated with increased ventricular arrhythmogenic risk which can result in sudden cardiac death. Lead I and Lead II ECG signals from wild-type and Scn5a +/- mice are used and the mice are also grouped as female/male and young/old.We use our novel Symmetric Projection Attractor Reconstruction (SPAR) method to generate an attractor from the ECG signal using all of the available waveform data. We have previously manually extracted a variety of quantitative measures from the attractor and used machine learning to classify each animal as either wild-type or mutant. In this work, we take the attractor images and use these as input to a deep learning algorithm in order to perform the same classification. As there is only data available from 42 mice, we use a transfer learning approach in which a network that has been pretrained on millions of images is used as a starting point and the last few layers are changed in order to fine tune the network for the attractor images.The results for the transfer learning approach are not as good as for the manual features, which is not too surprising as the networks have not been trained on attractor images. However, this approach shows the potential for using deep learning for classification of attractor images.
Current arterial pulse monitoring systems capture data at high frequencies (100{1000Hz). However, they typically report averaged or low frequency summary data such as heart rate and systolic, mean and diastolic blood pressure. In doing so, a potential wealth of information contained in the high fidelity waveform data is discarded, data which has long been known to contain useful information on cardiovascular performance. Here we summarise a new mathematical method, attractor reconstruction, which enables the quantification of arterial waveform shape and variability in real-time. The method can handle long streams of non-stationary data and does not require preprocessing of the raw physiological data by the end user. Whilst the detailed mathematical proofs have been described elsewhere (Aston et al., 2018), the authors were motivated to write a summary of the method and its potential utility for biomedical researchers, physiologists and clinician readers. Here we illustrate how this new method may supplement and potentially enhance the sensitivity of detecting cardiovascular disturbances, to aid with biomedical research and clinical decision making.
Machine learning (ML) methods for the analysis of electrocardiography (ECG) data are gaining importance, substantially supported by the release of large public datasets. However, these current datasets miss important derived descriptors such as ECG features that have been devised in the past hundred years and still form the basis of most automatic ECG analysis algorithms and are critical for cardiologists' decision processes. ECG features are available from sophisticated commercial software but are not accessible to the general public. To alleviate this issue, we add ECG features from two leading commercial algorithms and an open-source implementation supplemented by a set of automatic diagnostic statements from a commercial ECG analysis software in preprocessed format. This allows the comparison of ML models trained on clinically versus automatically generated label sets. We provide an extensive technical validation of features and diagnostic statements for ML applications. We believe this release crucially enhances the usability of the PTB-XL dataset as a reference dataset for ML methods in the context of ECG data.
Background Postoperative delirium (POD) is associated with increased short- and long-term mortality and several risk factors have been described. Decreased regional cerebral oxygen saturations (rScO2) may be a modifiable risk factor for POD yet its measurement is not used in routine clinical practice. Aims The primary aim of this study was to assess the feasibility of measuring rScO2 and screening for POD in patients over 60 years of age having non-cardiac surgery. Our secondary aim was to perform exploratory analyses of the relationship between boluses of intra-operative vasopressor and rScO2 values. Methods rScO2 were recorded in 60 patients over the age of 60 years having non-cardiac surgery in a single centre. Patients were screened daily for delirium for up to 7 days of the inpatient stay or until discharge, which ever occurred sooner. Results Of the 60 patients recruited, 58 underwent complete daily assessment for POD. Of those, 2 developed POD (3.4%). Patients that developed POD tended to be older, but no other statistically significant differences were observed. Analysis of the effect of phenylephrine boluses on rScO2 observed a ‘rise, drop, return’ pattern in an 8-minute window post-bolus administration. Conclusion The study protocol was found to be feasible to deliver. The study was unable to find any associations between rScO2 and POD, however, the study identified a relationship between intraoperative vasopressor boluses and subsequent rScO2.
Groundbreaking new drugs called direct acting antivirals have been introduced recently for the treatment of chronic Hepatitis C virus infection.We introduce a mathematical model for Hepatitis C dynamics treated with the direct acting antiviral drug, telaprevir, alongside traditional interferon and ribavirin treatments to understand how this combination therapy affects the viral load of patients exhibiting different types of response.We use sensitivity and identifiability techniques to determine which model parameters can be best estimated from viral load data. Parameter estimationwith these best estimable parameters is then performed to give patient-specific fits of the model to partial virologic response, sustained virologic response and breakthrough patients.
At the University of Surrey in 1995 an EPSRC Spring School was held in Applied Nonlinear Mathematics for postgraduate students in mathematics, engineering, physics or biology; this volume contains the bulk of the lectures given there by a team of internationally distinguished scientists. The aim of the school was to introduce students to current topics of research interest at an appropriate level. The majority of the courses are in the area of nonlinear dynamics with application to fluid dynamics, boundary layer transition, driven oscillators and waves. However, there are also lectures considering problems in nonlinear elasticity and mathematical biology. The articles have all been edited so that the book forms a coherent and accessible account of recent advances in nonlinear mathematics.
Background Respiratory pattern analysis is conventionally reduced to simplified metrics, such as respiratory rate (RR), tidal volume (Vt) or respiratory rate variability (RRV). Respiratory waveforms are nonetheless complex and generally sampled with high fidelity. A novel mathematical method, Symmetric Projection Attractor Reconstruction (SPAR), analyses waveforms without discarding any of this high-fidelity data, focusing on subtle changes in morphology and variability. SPAR creates a simpler visual representation of waveforms (attractor) which can be qualitatively and quantitatively analysed. Aim To investigate whether SPAR attractors can provide additional physiological biomarkers of pathophysiological respiratory changes in COPD patients. Methods Pneumotachograph airflow waveform data, recorded during resting tidal breathing and maximum voluntary ventilation (MVV), were analysed conventionally and using SPAR. Data from 56 healthy subjects (43.5 years median age, 7% female) and 10 COPD patients (68.5 years median age, 10% female, 39% median%predicted FEV1) were compared. Receiver operating characteristics area under the curve (ROC AUC) was used to ascertain sensitivity and specificity of classifying changes between healthy and COPD subjects (arbitrary threshold > 0.7). Results During resting tidal breathing, RR, Vt and attractor morphology metrics were not notably different between the two groups (ROC AUC = 0.70, 0.58, 0.50). RRV was higher in COPD patients (ROC AUC = 0.74) but more sensitively measured using attractor variability metrics which map wave-to-wave variability (ROC AUC = 0.86). During MVV, RR did not change (ROC AUC = 0.58). Vt was lower in COPD patients (ROC AUC = 0.95). Clear morphological differences were seen between the groups where COPD attractors were ‘pinched’ corresponding to a flattening of the expiratory trace (ROC AUC = 0.95). RRV was not different (ROC AUC = 0.54), but attractor variability was decreased in the COPD group (ROC AUC = 0.72) (figure 1). Conclusions SPAR provides a new ‘at-a glance’ representation of waveform morphology and variability. SPAR differentiated COPD patients from healthy subjects by highlighting changes in the non-spirometric, resting and MVV airflow waveforms. The morphological change of MVV attractors most likely reflects expiratory airflow limitation. Further work is needed to explore the use of SPAR to facilitate respiratory diagnostics and disease monitoring.
When a superball is thrown forwards but with backspin, it is observed to reverse both direction and spin for a few bounces before settling to bouncing motion in one direction. The bouncing ball is modelled by a two-dimensional iterated map in terms of the horizontal velocity and spin immediately after each bounce. The asymptotic motion of this system is easily determined. However, of more interest is the transient behaviour. The two-dimensional linear map is reduced to a one-dimensional non-linear map and this map is used to determine the number of direction and spin reversals that can occur for given initial conditions. The model is then generalised to describe a ball bouncing up and down a single step or successively down a staircase.
To study the dynamic changes in cognition across the human menstrual cycle, twenty, healthy, naturally-cycling women undertook a lateralized spatial figural comparison task on twelve occasions at approximately 3-4 day intervals. Each session was conducted in laboratory conditions with response times, accuracy rates, eye movements, salivary estrogen and progesterone concentrations and Profile of Mood states questionnaire data collected on each occasion. The first two sessions of twelve for the response variables were discarded to avoid early effects of learning thereby providing 10 sessions spread across each participant's complete menstrual cycle. Salivary progesterone data for each participant was utilized to normalize each participant's data to a standard 28 day cycle. Data was analysed categorically by comparing peak progesterone (luteal phase) to low progesterone (follicular phase) to emulate two-session repeated measures typical studies. Neither a significant difference in reaction times or accuracy rates was found. Moreover no significant effect of lateral presentation was observed upon reaction times or accuracy rates although inter and intra individual variance was sizeable. Using a 'phase plane' plot, we demonstrated that hormone concentrations alone cannot be used to predict the response times or accuracy rates. In contrast, we constructed a standard linear model using salivary estrogen, salivary progesterone and their respective derivative values and found these inputs to be very accurate for predicting variance observed in the reaction times for all stimuli and accuracy rates for right visual field stimuli but not left visual field stimuli. The identification of sex-hormone derivatives as predictors of cognitive behaviours is of importance. The finding suggests that there is a fundamental difference between the up-surge and decline of hormonal concentrations where previous studies typically assume all points near the peak of a hormonal surge are the same. How contradictory findings in sex-hormone research may have come about are discussed.
We consider the possibility of free receptor (antigen/cytokine) levels rebounding to higher than the baseline level after the application of an antibody drug using a target-mediated drug disposition model. It is assumed that the receptor synthesis rate experiences homeostatic feedback from the receptor levels. It is shown for a very fast feedback response, that the occurrence of rebound is determined by the ratio of the elimination rates, in a very similar way as for no feedback. However, for a slow feedback response, there will always be rebound. This result is illustrated with an example involving the drug efalizumab for patients with psoriasis. It is shown that slow feedback can be a plausible explanation for the observed rebound in this example.
When a superball is thrown forwards but with backspin, it is observed to reverse both direction and spin for a few bounces before settling to bouncing motion in one direction. The bouncing ball is modelled by a two-dimensional iterated map in terms of the horizontal velocity and spin immediately after each bounce. The asymptotic motion of this system is easily determined. However, of more interest is the transient behaviour. The two-dimensional linear map is reduced to a one-dimensional nonlinear map and this map is used to determine the number of direction and spin reversals that can occur for given initial conditions. The model is then generalised to describe a ball bouncing up and down a single step or successively down a staircase.
Changes in our climate and environment make it ever more important to understand the processes involved in Earth systems, such as the carbon cycle. There are many models that attempt to describe and predict the behaviour of carbon stocks and stores but, despite their complexity, significant uncertainties remain. We consider the qualitative behaviour of one of the simplest carbon cycle models, the Data Assimilation Linked Ecosystem Carbon (DALEC) model, which is a simple vegetation model of processes involved in the carbon cycle of forests, and consider in detail the dynamical structure of the model. Our analysis shows that the dynamics of both evergreen and deciduous forests in DALEC are dependent on a few key parameters and it is possible to find a limit point where there is stable sustainable behaviour on one side but unsustainable conditions on the other side. The fact that typical parameter values reside close to this limit point highlights the difficulty of predicting even the correct trend without sufficient data and has implications for the use of data assimilation methods.
Suppose a chaotic attractor A in an invariant subspace loses stability on varying a parameter. At the point of loss of stability, the most positive Lyapunov exponent of the natural measure on A crosses zero at what has been called a ‘blowout’ bifurcation. We introduce the notion of an essential basin of an attractor A. This is the set of points x such that accumulation points of the sequence of measures are supported on A. We characterise supercritical and subcritical scenarios according to whether the Lebesgue measure of the essential basin of A is positive or zero. We study a drift-diffusion model and a model class of piecewise linear mappings of the plane. In the supercritical case, we find examples where a Lyapunov exponent of the branch of attractors may be positive (‘hyperchaos’) or negative, depending purely on the dynamics far from the invariant subspace. For the mappings we find asymptotically linear scaling of Lyapunov exponents, average distance from the subspace and basin size on varying a parameter. We conjecture that these are general characteristics of blowout bifurcations.
Periodic physiological waveform data, such as blood pressure, pulse oximetry and ECG, are routinely sampled between 100 and 1000 Hz in preclinical research and in the clinical setting from a wide variety of implantable, bedside and wearable monitoring devices. Despite the under-lying numerical waveform data being captured at such high fidelity, conventional analysis tends to reside in reporting only averages of minimum, maximum, amplitude and rate, as single point averages. Although these averages are undoubtedly of value, simplification of the data in this way means that most of the available numerical data are discarded. In turn, this may lead to subtle physiological changes being missed when investigating the cardiovascular system over time. We have developed a mathematical method (symmetric projection attractor reconstruction) that uses all the numerical data, replotting and revisualizing them in a manner that allows unique quantification of multiple changes in waveform morphology and variability. We propose that the additional quantification of these features will allow the complex behaviour of the cardiovascular system to be mapped more sensitively in different physiological and pathophysiological settings.
DNA repair capacity varies greatly between individuals, and evidence has begun to link this variation to cancer risk, obesity and related chronic diseases. There is also emerging evidence that dietary components can affect DNA repair, but research to date has been restricted by methods for measuring DNA repair. This study made use of newly developed microplate-based assays for the direct determination of DNA repair enzyme activities. Lipid loading of the HepG2 human hepatocellular carcinoma cell line was employed as a model to test the hypothesis that hepatic steatosis affects DNA repair activity via induction of oxidative stress.
We consider a generalisation of Ulam's method for approximating invariant densities of one-dimensional maps. Rather than use piecewise constant polynomials to approximate the density, we use polynomials of degree $ n$ which are defined by the requirement that they preserve the measure on $ n+1$ neighbouring subintervals. Over the whole interval, this results in a discontinuous piecewise polynomial approximation to the density. We prove error results where this approach is used to approximate smooth densities. We also consider the computation of the Lyapunov exponent using the polynomial density and show that the order of convergence is one order better than for the density itself. Together with using cubic polynomials in the density approximation, this yields a very efficient method for computing highly accurate estimates of the Lyapunov exponent. We illustrate the theoretical findings with some examples.
We consider the relationship between the target affinity of a monoclonal antibody and its in vivo potency. The dynamics of the system is described mathematically by a target-mediated drug disposition model. As a measure of potency, we consider the minimum level of the free receptor following a single bolus injection of the ligand into the plasma compartment. From the differential equations, we derive two expressions for this minimum level in terms of the parameters of the problem, one of which is valid over the full range of values of the equilibrium dissociation constant K(D) and the other which is valid only for a large drug dose or for a small value of K(D). Both of these formulae show that the potency achieved by increasing the association constant k(on) can be very different from the potency achieved by decreasing the dissociation constant k(off). In particular, there is a saturation effect when decreasing k(off) where the increase in potency that can be achieved is limited, whereas there is no such effect when increasing k(on). Thus, for certain monoclonal antibodies, an increase in potency may be better achieved by increasing k(on) than by decreasing k(off).
Background: The electrocardiogram (ECG) is a key tool in patient management. Automated ECG analysis supports clinical decision-making, but traditional fiducial point identification discards much of the time-series data that captures the morphology of the whole waveform. Our Symmetric Projection Attractor Reconstruction (SPAR) method uses all the available data to provide a new visualization and quantification of the morphology and variability of any approximately periodic signal. We therefore applied SPAR to ECG signals to ascertain whether this more detailed investigation of ECG morphology adds clinical value. Methods: Our aim was to demonstrate the accuracy of the SPAR method in discriminating between two biologically distinct groups. As sex has been shown to influence the waveform appearance, we investigated sex differences in normal sinus rhythm ECGs. We applied the SPAR method to 9,007 10 second 12-lead ECG recordings from Physionet, which comprised; Dataset 1: 104 subjects (40% female), Dataset 2: 8,903 subjects (54% female). Results: SPAR showed clear visual differences between female and male ECGs (Dataset 1). A stacked machine learning model achieved a cross-validation sex classification accuracy of 86.3% (Dataset 2) and an unseen test accuracy of 91.3% (Dataset 1). The mid-precordial leads performed best in classification individually, but the highest overall accuracy was achieved with all 12 leads. Classification accuracy was highest for young adults and declined with older age. Conclusions: SPAR allows quantification of the morphology of the ECG without the need to identify conventional fiducial points, whilst utilizing of all the data reduces inadvertent bias. By intuitively re-visualizing signal morphology as two-dimensional images, SPAR accurately discriminated ECG sex differences in a small dataset. We extended the approach to a machine learning classification of sex for a larger dataset, and showed that the SPAR method provided a means of visualizing the similarities of subjects given the same classification. This proof-of-concept study therefore provided an implementation of SPAR using existing data and showed that subtle differences in the ECG can be amplified by the attractor. SPAR's supplementary analysis of ECG morphology may enhance conventional automated analysis in clinically important datasets, and improve patient stratification and risk management.
Motivated by a problem from pharmacology, we consider a general two parameter slow-fast system in which the critical set consists of a one dimensional manifold and a two dimensional manifold, intersecting transversally at the origin. Using geometric desingularisation, we show that for a subset of the parameter set there is an exchange of stabilities between the attracting components of the critical set and the direction of the continuation can be expressed in terms of the parameters.
Deep learning models for electrocardiogram (ECG) classification can be affected by the presence of physiological noise on the ECG, as shown in previous work. In this study, we explore the impact of different physiological noise types, and differing signal-to-noise ratios (SNRs) of noise on classification performance. We find that classification performance is impacted differently by different noise types. In addition, the best classification performance comes from using a network trained on clean ECGs to classify clean ECGs. In conclusion, this study has revealed several questions regarding inclusion or exclusion of noise on the ECG for training and classification by deep learning models.
Attractor reconstruction analysis has previously been applied to analyse arterial blood pressure and photoplethysmogram signals. This study extends this novel technique to ECG signals. We show that the method gives high accuracy in identifying gender from ECG signals, performing significantly better than the same classification by interval measures.
We study a codimension two steady-state/steady-state mode interaction with D-4 symmetry, where the center manifold is three-dimensional. Primary branches of equilibria undergo secondary Hopf bifurcations to periodic solutions which undergo further bifurcations leading to chaotic dynamics. This is not an exponentially small effect, and the chaos obtained in simulations using DsTool is large-scale, in contrast to the "weak" chaos associated with Shilnikov theory. Moreover, there is an abundance of symmetric chaotic attractors and symmetry-increasing bifurcations. The local bifurcation studied in this paper is the simplest ( in terms of dimension of the center manifold and codimension of the bifurcation) in which such phenomena have been identified. Numerical investigations demonstrate that the symmetric chaos is part of the local codimension two bifurcation. The two-dimensional parameter space is mapped out in detail for a specific choice of Taylor coefficients for the center manifold vector field. We use AUTO to compute the transitions involving periodic solutions, Lyapunov exponents to determine the chaotic region, and symmetry detectives to determine the symmetries of the various attractors.
Attractor reconstruction (AR) analysis has been used previously to quantify the variability in arterial blood pressure (ABP) signals. Since ABP signals are only available in a minority of clinical scenarios, we sought to determine whether AR could also be performed on more widely available photoplethysmogram (PPG) signals. AR analysis was performed on simultaneous ABP and PPG signals before, during and after a change in cardiovascular state. A novel quality metric was used to eliminate windows of low quality AR. A high level of agreement was found between the detected periodicity of each signal, tau-opt, a measure of the heart rate. The remaining cardiovascular parameters derived using AR analysis exhibited similar trends between the two signals in response to the change in state, although there was poor agreement between their absolute values. This demonstrates the feasibility of applying AR to the PPG signal, providing opportunity to increase the range of patients in whom cardiovascular state can be measured using AR analysis.
The photoplethysmogram (PPG) signal is widely used in pulse oximeters and smartwatches. A fundamental step in analysing the PPG is the detection of heartbeats. Several PPG beat detection algorithms have been proposed, although it is not clear which performs best. Objective: This study aimed to: (i) develop a framework with which to design and test PPG beat detectors; (ii) assess the performance of PPG beat detectors in different use cases; and (iii) investigate how their performance is affected by patient demographics and physiology. Approach: Fifteen beat detectors were assessed against electrocardiogram-derived heartbeats using data from eight datasets. Performance was assessed using the F1 score, which combines sensitivity and positive predictive value. Main results: Eight beat detectors performed well in the absence of movement with F1 scores of ≥90% on hospital data and wearable data collected at rest. Their performance was poorer during exercise with F1 scores of 55%–91%; poorer in neonates than adults with F1 scores of 84%–96% in neonates compared to 98%–99% in adults; and poorer in atrial fibrillation (AF) with F1 scores of 92%–97% in AF compared to 99%–100% in normal sinus rhythm. Significance: Two PPG beat detectors denoted 'MSPTD' and 'qppg' performed best, with complementary performance characteristics. This evidence can be used to inform the choice of PPG beat detector algorithm. The algorithms, datasets, and assessment framework are freely available.
We consider coupled identical chaotic systems. In some circumstances, the coupled systems synchronize. When this does not happen naturally, we derive methods based on small parameter perturbations which result in synchronous behavior. The perturbations are applied in the neighborhood of a fixed or periodic point in the synchronous subspace which is stable in the normal direction. By keeping iterates in the neighborhood of such points using parameter perturbations, they are naturally drawn closer to the subspace by the stable manifold of the fixed or periodic points. Different ways of varying the parameters are also considered. Methods for two-dimensional systems are first explored and then extended to higher-dimensional systems. Examples are presented to illustrate the methods.
In a previous paper (Aston, P. J. & Dellnitz, M. 1999 Comput. Meth. Appl. Mech. Engng 170, 223-237) we introduced a new method for computing the dominant Lyapunov exponent of a chaotic map by using spatial integration involving a matrix norm. We conjectured that this sequence of integrals decayed proportional to 1/n. We now prove this conjecture and derive a bound on the next term in the asymptotic expansion of the terms in the sequence. The Hénon map and a system of coupled Duffing oscillators are explored in detail in the light of these theoretical results.
We study a codimension two steady-state/steady-state mode interaction with D4 symmetry, where the center manifold is three-dimensional. Primary branches of equilibria undergo secondary Hopf bifurcations to periodic solutions which undergo further bifurcations leading to chaotic dynamics. This is not an exponentially small effect, and the chaos obtained in simulations using DsTool is large-scale, in contrast to the "weak" chaos associated with Shilnikov theory. Moreover, there is an abundance of symmetric chaotic attractors and symmetry-increasing bifurcations. The local bifurcation studied in this paper is the simplest (in terms of dimension of the center manifold and codimension of the bifurcation) in which such phenomena have been identified. Numerical investigations demonstrate that the symmetric chaos is part of the local codimension two bifurcation. The two-dimensional parameter space is mapped out in detail for a specific choice of Taylor coefficients for the center manifold vector field. We use AUTO to compute the transitions involving periodic solutions, Lyapunov exponents to determine the chaotic region, and symmetry detectives to determine the symmetries of the various attractors.
In embodiments of the present invention, periodic data is analysed by obtaining (SI) a vector of delay coordinates for each one of a plurality of samples of the periodic data in a time window, and transforming (S2) each of the vectors into a coordinate system comprising a plurality of predefined vectors, to obtain a projection of an attractor of the periodic data along one of the predefined vectors. The periodic data may be physiological data. Information representing one or more characteristics of the obtained attractor, which is of diagnostic value, is then displayed (S3) to enable a diagnosis.
This volume highlights successful projects in which academic mathematicians in the United Kingdom got involved with solving problems in industry for which mathematics was essential. It contains a wide range of articles in areas ranging from music composition to climate change which describe successful collaborations between mathematicians / statisticians and industry. The impact that the work had on the companies involved and on people’s everyday lives is included in each article. The book conveys to the non-expert some of the many ways that mathematics and statistics have contributed to economic growth and societal well-being.
Different methods for computing transient lengths in chaotic systems can give very different answers. This situation is resolved by the use of a waiting time paradox.
We consider the possibility of free receptor (antigen/cytokine) levels rebounding to higher than the baseline level after one or more applications of an antibody drug using a target-mediated drug disposition model. Using geometry and dynamical systems analysis, we show that rebound will occur if and only if the elimination rate of the drug-receptor product is slower than the elimination rates of the drug and of the receptor. We also analyse the magnitude of rebound through approximations and simulations and demonstrate that it increases if the drug dose increases or if the difference between the elimination rate of the drug-receptor product and the minimum of the elimination rates of the drug and of the receptor increases. © 2013 Springer-Verlag Berlin Heidelberg.
The electrocardiogram (ECG) is one of the most widespread diagnostic tools in healthcare and supports the diagnosis of cardiovascular disorders. Deep learning methods are a successful and popular technique to detect indications of disorders from an ECG signal. However, there are open questions around the robustness of these methods to various factors, including physiological ECG noise. In this study we generate clean and noisy versions of an ECG dataset before applying Symmetric Projection Attractor Reconstruction (SPAR) and scalogram image transformations. A pretrained convolutional neural network is trained using transfer learning to classify these image transforms. For the clean ECG dataset, F1 scores for SPAR attractor and scalogram transforms were 0.70 and 0.79, respectively, and the scores decreased by less than 0.05 for the noisy ECG datasets. Notably, when the network trained on clean data was used to classify the noisy datasets, performance decreases of up to 0.18 in F1 scores were seen. However, when the network trained on the noisy data was used to classify the clean dataset, the performance decrease was less than 0.05. We conclude that physiological ECG noise impacts classification using deep learning methods and careful consideration should be given to the inclusion of noisy ECG signals in the training data when developing supervised networks for ECG classification.
Introduction Photoplethysmogram signals from wearable devices typically measure heart rate and blood oxygen saturation, but contain a wealth of additional information about the cardiovascular system. In this study, we compared two signal-processing techniques: fiducial point analysis and Symmetric Projection Attractor Reconstruction, on their ability to extract new cardiovascular information from a photoplethysmogram signal. The aim was to identify fiducial point analysis and Symmetric Projection Attractor Reconstruction indices that could classify photoplethysmogram signals, according to age, sex and physical activity. Methods Three datasets were used: an in-silico dataset of simulated photoplethysmogram waves for healthy male participants (25–75 years old); an in-vivo dataset containing 10-min photoplethysmogram recordings from 57 healthy subjects at rest (18–39 or > 70 years old; 53% female); and an in-vivo dataset containing photoplethysmogram recordings collected for 4 weeks from a single subject, in daily life. The best-performing indices from the in-silico study (5/48 fiducial point analysis and 6/49 Symmetric Projection Attractor Reconstruction) were applied to the in-vivo datasets. Results Key fiducial point analysis and Symmetric Projection Attractor Reconstruction indices, which showed the greatest differences between groups, were found to be consistent across datasets. These indices were related to systolic augmentation, diastolic peak positioning and prominence, and waveform variability. Both fiducial point analysis and Symmetric Projection Attractor Reconstruction techniques provided indices that supported the classification of age and physical activity, but not sex. Conclusions Both fiducial point analysis and Symmetric Projection Attractor Reconstruction techniques demonstrated utility in identifying cardiovascular differences between individuals and within an individual over time. Future research should investigate the potential utility of these techniques for extracting information on fitness and disease, to support healthcare-decision making.
This work is aimed at a middle ground between these two. Articles contain some mathematical detail, but the emphasis is on telling the story of a successful collaboration between academia and industry and on the results obtained.
Many methods have been proposed for analysing high frequency blood pressure or ECG data. We review a recently proposed new approach for analysing such data based on attractor reconstruction and compare it to heart rate variability that analyses the beat-to-beat intervals. Our new approach uses all the available data and so can detect changes in the shape of the waveform.
Symmetric Projection Attractor Reconstruction (SPAR) provides an intuitive visualization and simple quantification of the morphology and variability of approximately periodic signals. The original method takes a three-dimensional delay coordinate embedding of a signal and subsequently projects this phase space reconstruction to a two-dimensional image with threefold symmetry, providing a bounded visualization of the waveform. We present an extension of the original work to apply delay coordinate embedding in any dimension 𝑁≥3 while still deriving a two-dimensional output with some rotational symmetry property that provides a meaningful visualization of the higher dimensional attractor. A generalized result is developed for taking 𝑁≥3 delay coordinates from a continuous periodic signal, where we determine invariant subspaces of the phase space that provide a two-dimensional projection with the required rotational symmetry. The result in each subspace is shown to be equivalent to following each pair of coefficients of the trigonometric interpolating polynomial of 𝑁 evenly spaced points as the signal is translated horizontally. Bounds on the mean and the frequency response of our new coordinates are derived. We demonstrate how this aids our understanding of the attractor properties and its relationship to the underlying waveform. Our generalized result is then extended to real, approximately periodic signals, where we demonstrate that the higher dimensional SPAR method provides information on subtle changes in different parts of the waveform morphology. Data science is challenged to derive meaningful information from large volumes of data. The Symmetric Projection Attractor Reconstruction (SPAR) method1 provides an innovative approach to the analysis of approximately periodic time-series data by generating a unique, bounded, visualization of the signal that encapsulates waveform shape and variability from a three-dimensional time delay embedding of a signal. This paper extends this work to present a general form giving a similar visualization derived from an embedding of the signal in any dimension 𝑁≥3, and the result is shown to be closely related to trigonometric polynomial interpolation. While the theoretical results are stated in the context of periodic signals, significantly, we show that the properties of this theoretical generalization can be translated to the analysis of real, approximately periodic signals where we demonstrate the potential clinical utility of the SPAR approach in the novel visualization and quantification of physiological signals.
Radioactive decay of an unstable isotope is widely believed to be exponential. This view is supported by experiments on rapidly decaying isotopes but is more difficult to verify for slowly decaying isotopes. The decay of 14C can be calibrated over a period of 12550 years by comparing radiocarbon dates with dates obtained from dendrochronology. It is well known that this approach shows that radiocarbon dates of over 3000 years are in error, which is generally attributed to past variation in atmospheric levels of 14C. We note that predicted atmospheric variation (assuming exponential decay) does not agree with results from modelling, and that theoretical quantum mechanics does not predict exact exponential decay. We give mathematical arguments that non-exponential decay should be expected for slowly decaying isotopes and explore the consequences of non-exponential decay. We propose an experimental test of this prediction of non-exponential decay for 14C. If confirmed, a foundation stone of current dating methods will have been removed, requiring a radical reappraisal both of radioisotope dating methods and of currently predicted dates obtained using these methods.
Acute exacerbations of COPD (AECOPD) are the second most common cause of emergency hospitalisation worldwide, with one-quarter of patients readmitted within 30 days of discharge [1]. Each exacerbation accelerates lung function decline [2] and is associated with a deterioration in health-related quality of life and an increased risk of mortality in the post-discharge period [3]. Several pathophysiological changes including decreased peak expiratory flow rate and increased dyspnoea often occur in the 3–5 days preceding an exacerbation [4]. Respiratory waveforms can be reduced to simple metrics, such as rate, but this may miss information about waveform shape and whole breathing pattern. A novel analysis method quantifying the whole waveform shape identifies AECOPD earlier. https://bit.ly/3M6uIEB
The aim of this study is a preliminary investigation into the application of our novel Symmetric Projection Attractor Reconstruction (SPAR) method to the electrocardiogram (ECG) signals of individuals treated with the cardioactive drug dofetilide. We show that our SPAR technique correlates with standard assessment, and is also able to discriminate gender from the ECG response to dofetilide more accurately than the standard metrics.
Methodologies are presented for assessing the feasibility of parameter estimation in nonlinear ordinary differential equation (ODE) models. These methods are applied to a recent model for hepatitis C viral dynamics. Subset selection is performed on the model parameters, and maximum likelihood estimation is conducted using available data from the literature.
Applications are beginning to be found for chaotic power converters. Using the peak current controlled boost converter as an example throughout, the paper reviews the theory of chaos, shows how it may be employed to improve the electromagnetic compatibility (EMC) of power supplies, and presents a targeting scheme that can make a chaotic converter jump rapidly between two stabilised modes of operation.
We review various existing models of hepatitis C (HCV) infection and show that there are inconsistencies between the models and known behaviour of the infection. A new model for HCV infection is proposed, based on various dynamical processes that occur during the infection that are described in the literature. This new model is analysed and three steady state branches of solutions are found when there is no stem cell generation of hepatocytes. Unusually, the branch of infected solutions that connects the uninfected branch and the pure infection branch can be found analytically and always includes a limit point. When the action of stem cells is included, the bifurcation between the pure infection and infected branches unfolds, leaving a single branch of infected solutions. It is shown that this model can generate various viral load profiles that have been described in the literature which is confirmed by fitting the model to four viral load datasets. Suggestions for possible changes in treatment are made based on the model.
Advances in monitoring technology allow blood pressure waveforms to be collected at sampling frequencies of 250-1000Hz for long time periods. However, much of the raw data are under analysed. Heart rate variability (HRV) methods, in which beat-to-beat interval lengths are extracted and analysed, have been extensively studied, However, this approach discards the majority of the raw data. Objective: Our aim is to detect changes in the shape of the waveform in long streams of blood pressure data. Approach: Our approach involves extracting key features from large complex datasets by generating a reconstructed attractor in a three-dimensional phase space using delay coordinates from a window of the entire raw waveform data. The naturally occurring baseline variation is removed by projecting the attractor onto a plane from which new quantitative measures are obtained. The time window is moved through the data to give a collection of signals which relate to various aspects of the waveform shape. Main results: This approach enables visualisation and quantification of changes in the waveform shape and has been applied to blood pressure data collected from conscious unrestrained mice and to human blood pressure data. The interpretation of the attractor measures is aided by the analysis of simple artificial waveforms. Significance: We have developed and analysed a new method for analysing blood pressure data that uses all of the waveform data and hence can detect changes in the waveform shape that HRV methods cannot, which is confirmed with an example, and hence our method goes "beyond HRV".
Background: Atrial fibrillation (AF) is a common cardiac arrhythmia in both human and equine populations. It is associated with adverse outcomes in humans, and decreased athletic performance in both populations. Paroxysmal atrial fibrillation (PAF) presents with intermittent, self-terminating AF episodes, and is difficult to diagnose once sinus rhythm resumes.Objective: We aimed to detect PAF subjects from normal sinus rhythm equine ECGs using the Symmetric Projection Attractor Reconstruction (SPAR) method to encapsulate the waveform morphology and variability as the basis of a machine learning classification.Methods: We obtained ECG signals from 139 active equine athletes (120 control, 19 with a PAF diagnosis). The SPAR method was applied to nine short (20-second) ECG strips for each subject. An optimal SPAR feature set was determined by forward feature selection for input to a machine learning model ensemble of three different classifiers (k-NN, linear SVM and RBF kernel SVM). Imbalanced data was handled by upsampling the minority (PAF) class. A final subject classification was made by taking a majority vote over results from the nine ECG strips.Results: Our final cross-validated classification for a subject gave an accuracy of 89.0%, sensitivity of 94.8%, specificity of 87.1% and ROC AUC of 0.98, taking PAF as the positive class.Conclusion: The SPAR method and machine learning generated a final model with high sensitivity, suggesting that PAF can be discriminated from short equine ECG strips. This preliminary study indicated that SPAR analysis of human ECG could support patient monitoring, risk stratification and clinical decision-making.
Deep learning models for electrocardiogram (ECG) classification can be affected by the presence of physiologicalnoise on the ECG, as shown in previous work. In this study, we explore the impact of different physiological noise types, and differing signal-to-noise ratios (SNRs) of noise on classification performance. We find that classification performance is impacted differently by different noise types. In addition, the best classification performance comes from using a network trained on clean ECGs to classify clean ECGs. In conclusion, this study has revealed several questions regarding inclusion or exclusion of noise on the ECG for training and classification by deep learning models.