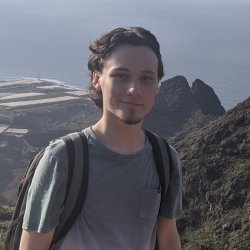
Ollie Camilleri
About
My research project
Neural rendering of object-based audio-visual scenesMy work is part of the AI4ME project and involves researching the relationship between audio and visual signals within a neural rendering framework. Neural rendering is a rapidly developing class of image and video generation that combines physical knowledge from classical computer graphics with deep learning to synthesize controllable scenes. More specifically, my project will attempt to render complex and natural audio-visual scenes under novel conditions such as lighting, viewing angle, or object placement.
Supervisors
My work is part of the AI4ME project and involves researching the relationship between audio and visual signals within a neural rendering framework. Neural rendering is a rapidly developing class of image and video generation that combines physical knowledge from classical computer graphics with deep learning to synthesize controllable scenes. More specifically, my project will attempt to render complex and natural audio-visual scenes under novel conditions such as lighting, viewing angle, or object placement.
Publications
Leveraging machine learning techniques, in the context of object-based media production, could enable provision of personalized media experiences to diverse audiences. To fine-tune and evaluate techniques for personalization applications, as well as more broadly, datasets which bridge the gap between research and production are needed. We introduce and publicly release such a dataset, themed around a UK weather forecast and shot against a blue-screen background, of three professional actors/presenters – one male and one female (English) and one female (British Sign Language). Scenes include both production and research-oriented examples, with a range of dialogue, motions, and actions. Capture techniques consisted of a synchronized 4K resolution 16-camera array, production-typical microphones plus professional audio mix, a 16-channel microphone array with collocated Grasshopper3 camera, and a photogrammetry array. We demonstrate applications relevant to virtual production and creation of personalized media including neural radiance fields, shadow casting, action/event detection, speaker source tracking and video captioning.
Leveraging machine learning techniques, in the context of object-based media production, could enable provision of personalized media experiences to diverse audiences. To fine-tune and evaluate techniques for personalization applications, as well as more broadly, datasets which bridge the gap between research and production are needed. We introduce and publicly release such a dataset, themed around a UK weather forecast and shot against a blue-screen background, of three professional actors/presenters – one male and one female (English) and one female (British Sign Language). Scenes include both production and research-oriented examples, with a range of dialogue, motions, and actions. Capture techniques consisted of a synchronized 4K resolution 16-camera array, production-typical microphones plus professional audio mix, a 16-channel microphone array with collocated Grasshopper3 camera, and a photogrammetry array. We demonstrate applications relevant to virtual production and creation of personalized media including neural radiance fields, shadow casting, action/event detection, speaker source tracking and video captioning.