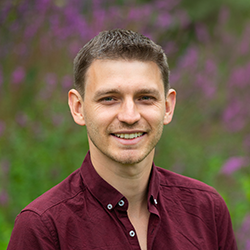
Dr Marco Volino
Academic and research departments
Centre for Vision, Speech and Signal Processing (CVSSP), School of Computer Science and Electronic Engineering.About
Biography
Please see my personal website for an up to date bio and CV
https://marcovolino.github.io/.
Areas of specialism
University roles and responsibilities
- Undergraduate Personal Tutor
- Professional Training Year Tutor
- CVSSP/PAI Internal Seminar Organiser
- Early Career Researcher (ECR) Representative
- Area 5 Health and Safety Representative
ResearchResearch interests
My research interests are at the intersection of computer vision, computer graphics and machine learning with a focus on applications in the creative industry. I am particular interested in media production technology, digital humans and immersive technology.
Research interests
My research interests are at the intersection of computer vision, computer graphics and machine learning with a focus on applications in the creative industry. I am particular interested in media production technology, digital humans and immersive technology.
Supervision
Postgraduate research supervision
Current:
Umar Farooq (principal supervisor)- Controllable neural rendering
Ollie Camilleri (co-supervisor) - Object-based neural rendering
Completed:
Marco Pesavento (co-supervisor) - Shape and Appearance Super-resolution of 3D Humans
Joao Regaterio (co-supervisor) - Learning to animate volumetric video
Teaching
I currently lead or contribute to teaching on the following modules:
- EEE2041 Computer Vision and Graphics
- EEEM067 AR/VR and the Metaverse
- EEE3017 UG Projects
- EEEM004 MSc Projects
I have previously taught on:
- EEE3032 Computer Vision and Pattern Recognition
Publications
We present a novel framework to reconstruct complete 3D human shapes from a given target image by leveraging monocular unconstrained images. The objective of this work is to reproduce high-quality details in regions of the reconstructed human body that are not visible in the input target. The proposed methodology addresses the limitations of existing approaches for reconstructing 3D human shapes from a single image, which cannot reproduce shape details in occluded body regions. The missing information of the monocular input can be recovered by using multiple views captured from multiple cameras. However, multi-view reconstruction methods necessitate accurately calibrated and registered images, which can be challenging to obtain in real-world scenarios. Given a target RGB image and a collection of multiple uncalibrated and unregistered images of the same individual, acquired using a single camera, we propose a novel framework to generate complete 3D human shapes. We introduce a novel module to generate 2D multi-view normal maps of the person registered with the target input image. The module consists of body part-based reference selection and body part-based registration. The generated 2D normal maps are then processed by a multi-view attention-based neural implicit model that estimates an implicit representation of the 3D shape, ensuring the reproduction of details in both observed and occluded regions. Extensive experiments demonstrate that the proposed approach estimates higher quality details in the non-visible regions of the 3D clothed human shapes compared to related methods, without using parametric models.
3D audio-visual production aims to deliver immersive and interactive experiences to the consumer. Yet, faithfully reproducing real-world 3D scenes remains a challenging task. This is partly due to the lack of available datasets enabling audio-visual research in this direction. In most of the existing multi-view datasets, the accompanying audio is neglected. Similarly, datasets for spatial audio research primarily offer unimodal content, and when visual data is included, the quality is far from meeting the standard production needs. We present "Tragic Talkers", an audio-visual dataset consisting of excerpts from the "Romeo and Juliet" drama captured with microphone arrays and multiple co-located cameras for light-field video. Tragic Talkers provides ideal content for object-based media (OBM) production. It is designed to cover various conventional talking scenarios, such as monologues, two-people conversations, and interactions with considerable movement and occlusion, yielding 30 sequences captured from a total of 22 different points of view and two 16-element microphone arrays. Additionally, we provide voice activity labels, 2D face bounding boxes for each camera view, 2D pose detection keypoints, 3D tracking data of the mouth of the actors, and dialogue transcriptions. We believe the community will benefit from this dataset as it can assist multidisciplinary research. Possible uses of the dataset are discussed.
As audio-visual systems increasingly bring immersive and interactive capabilities into our work and leisure activities, so the need for naturalistic test material grows. New volumetric datasets have captured high-quality 3D video, but accompanying audio is often neglected, making it hard to test an integrated bimodal experience. Designed to cover diverse sound types and features, the presented volumetric dataset was constructed from audio and video studio recordings of scenes to yield forty short action sequences. Potential uses in technical and scientific tests are discussed.
3D audio-visual production aims to deliver immersive and interactive experiences to the consumer. Yet, faithfully reproducing real-world 3D scenes remains a challenging task. This is partly due to the lack of available datasets enabling audio-visual research in this direction. In most of the existing multi-view datasets, the accompanying audio is neglected. Similarly, datasets for spatial audio research primarily offer unimodal content, and when visual data is included, the quality is far from meeting the standard production needs. We present “Tragic Talkers”, an audio-visual dataset consisting of excerpts from the “Romeo and Juliet” drama captured with microphone arrays and multiple co-located cameras for light-field video. Tragic Talkers provides ideal content for object-based media (OBM) production. It is designed to cover various conventional talking scenarios, such as monologues, two-people conversations, and interactions with considerable movement and occlusion, yielding 30 sequences captured from a total of 22 different points of view and two 16-element microphone arrays. Additionally, we provide voice activity labels, 2D face bounding boxes for each camera view, 2D pose detection keypoints, 3D tracking data of the mouth of the actors, and dialogue transcriptions. We believe the community will benefit from this dataset as it can assist multidisciplinary research. Possible uses of the dataset are discussed. The scenes were captured at the Centre for Vision, Speech & Signal Processing (CVSSP) of the University of Surrey (UK) with the aid of two twin Audio-Visual Array (AVA) Rigs. Each AVA Rig is a custom device consisting of a 16-element microphone array and 11 cameras fixed on a flat perspex sheet. For more information, please refer to the paper (see below) or contact the authors.
Room acoustics, and perception thereof, is an important consideration in research, engineering, architecture, creative expression, and many other areas of human activity, particularly indoors. Typical room datasets either contain disparate measurements of diverse spaces, e.g., the OpenAir dataset (Murphy and Shelley, 2010), or rich sets of measurements within a few rooms, e.g., CD4M (Stewart and Sandler, 2010). Development of techniques, such as the RSAO, with 6 degrees of freedom (6DOF) movement in media applications require testing distance-related effects. Hence there is a need for consistency across room measurements in terms of source-room-receiver configuration, such as in (Lokki et al., 2011) but particularly for typical rooms. Those available containing ARIRs, BRIRs and with a consistent measurement procedure tend to be limited in range of rooms measured (Bacila and Lee, 2019). We designed an RIR dataset covering a range of rooms, with typical reverberation times, 1O-ARIRs and BRIRs, and regular measurement procedure including source-receiver distances from 1 m to 3 m in 0.5 m intervals. We measured seven rooms including one room with variable acoustics in two configurations – eight total sets. The rooms within this dataset have mid-range RT60s from 0.24 s to 1.00 s and volumes from 50 m3 to 1600 m3. The accompanying paper includes capture methods, various descriptive metrics and a description of applications across diverse domains. The dataset consists of .WAV files for IRs, .PLY files for LiDAR scans and image files for accompanying 3D pictures. IRs are also available in the SOFA format. The dataset is licensed under CC BY-NC 4.0 and can be accessed from https://cvssp.org/data/SurrRoom1_0/.
Leveraging machine learning techniques, in the context of object-based media production, could enable provision of personalized media experiences to diverse audiences. To fine-tune and evaluate techniques for personalization applications, as well as more broadly, datasets which bridge the gap between research and production are needed. We introduce and publicly release such a dataset, themed around a UK weather forecast and shot against a blue-screen background, of three professional actors/presenters – one male and one female (English) and one female (British Sign Language). Scenes include both production and research-oriented examples, with a range of dialogue, motions, and actions. Capture techniques consisted of a synchronized 4K resolution 16-camera array, production-typical microphones plus professional audio mix, a 16-channel microphone array with collocated Grasshopper3 camera, and a photogrammetry array. We demonstrate applications relevant to virtual production and creation of personalized media including neural radiance fields, shadow casting, action/event detection, speaker source tracking and video captioning.
Immersive audio-visual perception relies on the spatial integration of both auditory and visual information which are heterogeneous sensing modalities with different fields of reception and spatial resolution. This study investigates the perceived coherence of audiovisual object events presented either centrally or peripherally with horizontally aligned/misaligned sound. Various object events were selected to represent three acoustic feature classes. Subjective test results in a simulated virtual environment from 18 participants indicate a wider capture region in the periphery, with an outward bias favoring more lateral sounds. Centered stimulus results support previous findings for simpler scenes.
Leveraging machine learning techniques, in the context of object-based media production, could enable provision of personalized media experiences to diverse audiences. To fine-tune and evaluate techniques for personalization applications, as well as more broadly, datasets which bridge the gap between research and production are needed. We introduce and publicly release such a dataset, themed around a UK weather forecast and shot against a blue-screen background, of three professional actors/presenters – one male and one female (English) and one female (British Sign Language). Scenes include both production and research-oriented examples, with a range of dialogue, motions, and actions. Capture techniques consisted of a synchronized 4K resolution 16-camera array, production-typical microphones plus professional audio mix, a 16-channel microphone array with collocated Grasshopper3 camera, and a photogrammetry array. We demonstrate applications relevant to virtual production and creation of personalized media including neural radiance fields, shadow casting, action/event detection, speaker source tracking and video captioning.
IEEE VR 2020 Immersive audio-visual perception relies on the spatial integration of both auditory and visual information which are heterogeneous sensing modalities with different fields of reception and spatial resolution. This study investigates the perceived coherence of audiovisual object events presented either centrally or peripherally with horizontally aligned/misaligned sound. Various object events were selected to represent three acoustic feature classes. Subjective test results in a simulated virtual environment from 18 participants indicate a wider capture region in the periphery, with an outward bias favoring more lateral sounds. Centered stimulus results support previous findings for simpler scenes.
3D audiovisual production aims to deliver immersive and interactive experiences to the consumer. Yet, faithfully reproducing real-world 3D scenes remains a challenging task. This is partly due to the lack of available datasets enabling audiovisual research in this direction. In most of the existing multi-view datasets, the accompanying audio is neglected. Similarly, datasets for spatial audio research primarily offer unimodal content, and when visual data is included, the quality is far from meeting the standard production needs. We present " Tragic Talkers " , an audiovisual dataset consisting of excerpts from the " Romeo and Juliet " drama captured with microphone arrays and multiple co-located cameras for light-field video. Tragic Talkers provides ideal content for object-based media (OBM) production. It is designed to cover various conventional talking scenarios, such as monologues, two-people conversations, and interactions with considerable movement and occlusion, yielding 30 sequences captured from a total of 22 different points of view and two 16-element microphone arrays. Additionally, we provide voice activity labels, 2D face bounding boxes for each camera view, 2D pose detection keypoints, 3D tracking data of the mouth of the actors, and dialogue transcriptions. We believe the community will benefit from this dataset as it can assist multidisciplinary research. Possible uses of the dataset are discussed. * This is the author's version of the work. It is posted here for your personal use. This paper is published under a Creative Commons Attribution (CC-BY) license. The definitive version was published in CVMP '22, https://doi.org/10.1145/3565516.3565522.
Immersive audio-visual perception relies on the spatial integration of both auditory and visual information which are heterogeneous sensing modalities with different fields of reception and spatial resolution. This study investigates the perceived coherence of audio-visual object events presented either centrally or peripherally with horizontally aligned/misaligned sound. Various object events were selected to represent three acoustic feature classes. Subjective test results in a simulated virtual environment from 18 participants indicate a wider capture region in the periphery, with an outward bias favoring more lateral sounds. Centered stimulus results support previous findings for simpler scenes.
In this paper we present a method for single-view illumination estimation of indoor scenes, using image-based lighting, that incorporates state-of-the-art outpainting methods. Recent advancements in illumination estimation have focused on improving the detail of the generated environment map so it can realistically light mirror reflective surfaces. These generated maps often include artefacts at the borders of the image where the panorama wraps around. In this work we make the key observation that inferring the panoramic HDR illumination of a scene from a limited field of view LDR input can be framed as an outpainting problem (whereby the original image must be expanded beyond its original borders). We incorporate two key techniques used in outpainting tasks: i) separating the generation into multiple networks (a diffuse lighting network and a high-frequency detail network) to reduce the amount to be learnt by a single network, ii) utilising an inside-out method of processing the input image to reduce the border artefacts. Further to incorporating these outpainting methods we also introduce circular padding before the network to help remove the border artefacts. Results show the proposed approach is able to relight diffuse, specular and mirror surfaces more accurately than existing methods in terms of the position of the light sources and pixelwise accuracy, whilst also reducing the artefacts produced at the borders of the panorama.
This paper introduces Deep4D a compact generative representation of shape and appearance from captured 4D volumetric video sequences of people. 4D volumetric video achieves highly realistic reproduction, replay and free-viewpoint rendering of actor performance from multiple view video acquisition systems. A deep generative network is trained on 4D video sequences of an actor performing multiple motions to learn a generative model of the dynamic shape and appearance. We demonstrate the proposed generative model can provide a compact encoded representation capable of high-quality synthesis of 4D volumetric video with two orders of magnitude compression. A variational encoder-decoder network is employed to learn an encoded latent space that maps from 3D skeletal pose to 4D shape and appearance. This enables high-quality 4D volumetric video synthesis to be driven by skeletal motion, including skeletal motion capture data. This encoded latent space supports the representation of multiple sequences with dynamic interpolation to transition between motions. Therefore we introduce Deep4D motion graphs, a direct application of the proposed generative representation. Deep4D motion graphs allow real-tiome interactive character animation whilst preserving the plausible realism of movement and appearance from the captured volumetric video. Deep4D motion graphs implicitly combine multiple captured motions from a unified representation for character animation from volumetric video, allowing novel character movements to be generated with dynamic shape and appearance detail.
Light fields are becoming an increasingly popular method of digital content production for visual effects and virtual/augmented reality as they capture a view dependent representation enabling photo realistic rendering over a range of viewpoints. Light field video is generally captured using arrays of cameras resulting in tens to hundreds of images of a scene at each time instance. An open problem is how to efficiently represent the data preserving the view-dependent detail of the surface in such a way that is compact to store and efficient to render. In this paper we show that constructing an Eigen texture basis representation from the light field using an approximate 3D surface reconstruction as a geometric proxy provides a compact representation that maintains view-dependent realism. We demonstrate that the proposed method is able to reduce storage requirements by > 95% while maintaining the visual quality of the captured data. An efficient view-dependent rendering technique is also proposed which is performed in eigen space allowing smooth continuous viewpoint interpolation through the light field.
This paper proposes a novel Attention-based Multi-Reference Super-resolution network (AMRSR) that, given a low-resolution image, learns to adaptively transfer the most similar texture from multiple reference images to the super-resolution output whilst maintaining spatial coherence. The use of multiple reference images together with attention-based sampling is demonstrated to achieve significantly improved performance over state-of-the-art reference super-resolution approaches on multiple benchmark datasets. Reference super-resolution approaches have recently been proposed to overcome the ill-posed problem of image super-resolution by providing additional information from a high-resolution reference image. Multi-reference super-resolution extends this approach by providing a more diverse pool of image features to overcome the inherent information deficit whilst maintaining memory efficiency. A novel hierarchical attention-based sampling approach is introduced to learn the similarity between low-resolution image features and multiple reference images based on a perceptual loss. Ablation demonstrates the contribution of both multi-reference and hierarchical attention-based sampling to overall performance. Perceptual and quantitative ground-truth evaluation demonstrates significant improvement in performance even when the reference images deviate significantly from the target image.
A real-time full-body motion capture system is presented which uses input from a sparse set of inertial measurement units (IMUs) along with images from two or more standard video cameras and requires no optical markers or specialized infra-red cameras. A real-time optimization-based framework is proposed which incorporates constraints from the IMUs, cameras and a prior pose model. The combination of video and IMU data allows the full 6-DOF motion to be recovered including axial rotation of limbs and drift-free global position. The approach was tested using both indoor and outdoor captured data. The results demonstrate the effectiveness of the approach for tracking a wide range of human motion in real time in unconstrained indoor/outdoor scenes.
We present an approach to multi-person 3D pose estimation and tracking from multi-view video. Following independent 2D pose detection in each view, we: (1) correct errors in the output of the pose detector; (2) apply a fast greedy algorithm for associating 2D pose detections between camera views; and (3) use the associated poses to generate and track 3D skeletons. Previous methods for estimating skeletons of multiple people suffer long processing times or rely on appearance cues, reducing their applicability to sports. Our approach to associating poses between views works by seeking the best correspondences first in a greedy fashion, while reasoning about the cyclic nature of correspondences to constrain the search. The associated poses can be used to generate 3D skeletons, which we produce via robust triangulation. Our method can track 3D skeletons in the presence of missing detections, substantial occlusions, and large calibration error. We believe ours is the first method for full-body 3D pose estimation and tracking of multiple players in highly dynamic sports scenes. The proposed method achieves a significant improvement in speed over state-of-the-art methods.
Video-based free-viewpoint rendering from multiple view video capture has achieved video-realistic performance replay. Existing free-viewpoint rendering approaches require storage, streaming and re-sampling of multiple videos, which requires high bandwidth and computational resources limiting applications to local replay on high-performance computers. This paper introduces a layered texture representation for efficient storage and view-dependent rendering from multiple view video capture whilst maintaining the video-realism. Layered textures re-sample the captured video according to the surface visibility. Prioritisation of layers according to surface visibility allows the N-best views for all surface elements to be pre-computed significantly reducing both storage and rendering cost. Typically 3 texture map layers are required for free-viewpoint rendering with an equivalent visual quality to the multiple view video giving a significant reduction in storage cost. Quantitative evaluation demonstrates that the layered representation achieves a 90% reduction in storage cost and 50% reduction in rendering cost without loss of visual quality compared to storing only the foreground of the original multiple view video. This reduces the storage and transmission cost for free-viewpoint video rendering from eight cameras to be similar to the requirements for a single video. Streaming the layered representation enables, for the first time, demonstration of free-viewpoint video rendering on mobile devices and web platforms.
The RE@CT project set out to revolutionise the production of realistic 3D characters for game-like applications and interactive video productions, and significantly reduce costs by developing an automated process to extract and represent animated characters from actor performance captured in a multi-camera studio. The key innovation is the development of methods for analysis and representation of 3D video to allow reuse for real-time interactive animation. This enables efficient authoring of interactive characters with video quality appearance and motion.
A real-time full-body motion capture system is presented which uses input from a sparse set of inertial measurement units (IMUs) along with images from two or more standard video cameras and requires no optical markers or specialized infra-red cameras. A real-time optimization-based framework is proposed which incorporates constraints from the IMUs, cameras and a prior pose model. The combination of video and IMU data allows the full 6-DOF motion to be recovered including axial rotation of limbs and drift-free global position. The approach was tested using both indoor and outdoor captured data. The results demonstrate the effectiveness of the approach for tracking a wide range of human motion in real time in unconstrained indoor/outdoor scenes.
A common problem in the 4D reconstruction of people from multi-view video is the quality of the captured dynamic texture appearance which depends on both the camera resolution and capture volume. Typically the requirement to frame cameras to capture the volume of a dynamic performance (> 50m^3) results in the person occupying only a small proportion < 10% of the field of view. Even with ul-tra high-definition 4k video acquisition this results in sampling the person at less-than standard definition 0.5k video resolution resulting in low-quality rendering. In this paper we propose a solution to this problem through super-resolution appearance transfer from a static high-resolution appearance capture rig using digital stills cameras (> 8k) to capture the person in a small volume (< 8m 3). A pipeline is proposed for super-resolution appearance transfer from high-resolution static capture to dynamic video performance capture to produce super-resolution dynamic textures. This addresses two key problems: colour mapping between different camera systems; and dynamic texture map super-resolution using a learnt model. Comparative evaluation demonstrates a significant qualitative and quantitative improvement in rendering the 4D performance capture with super-resolution dynamic texture appearance. The proposed approach reproduces the high-resolution detail of the static capture whilst maintaining the appearance dynamics of the captured video.
Light field video for content production is gaining both research and commercial interest as it has the potential to push the level of immersion for augmented and virtual reality to a close-to-reality experience. Light fields densely sample the viewing space of an object or scene using hundreds or even thousands of images with small displacements in between. However, a lack of standardised formats for compression, storage and transmission, along with the lack of tools to enable editing of light field data currently make it impractical for use in real-world content production. In this chapter we address two fundamental problems with light field data, namely representation and compression. Firstly we propose a method to obtain a 4D temporally coherent representation from the input light field video. This is an essential problem to solve that will enable efficient compression editing. Secondly, we present a method for compression of light field data based on the eigen texture method that provides a compact representation and enables efficient view-dependent rendering at interactive frame rates. These approaches achieve an order of magnitude compression and temporally consistent representation that are important steps towards practical toolsets for light field video content production.
Existing techniques for dynamic scene re- construction from multiple wide-baseline cameras pri- marily focus on reconstruction in controlled environ- ments, with fixed calibrated cameras and strong prior constraints. This paper introduces a general approach to obtain a 4D representation of complex dynamic scenes from multi-view wide-baseline static or moving cam- eras without prior knowledge of the scene structure, ap- pearance, or illumination. Contributions of the work are: An automatic method for initial coarse reconstruc- tion to initialize joint estimation; Sparse-to-dense tem- poral correspondence integrated with joint multi-view segmentation and reconstruction to introduce tempo- ral coherence; and a general robust approach for joint segmentation refinement and dense reconstruction of dynamic scenes by introducing shape constraint. Com- parison with state-of-the-art approaches on a variety of complex indoor and outdoor scenes, demonstrates im- proved accuracy in both multi-view segmentation and dense reconstruction. This paper demonstrates unsuper- vised reconstruction of complete temporally coherent 4D scene models with improved non-rigid object seg- mentation and shape reconstruction and its application to various applications such as free-view rendering and virtual reality.
4D Video Textures (4DVT) introduce a novel representation for rendering video-realistic interactive character animation from a database of 4D actor performance captured in a multiple camera studio. 4D performance capture reconstructs dynamic shape and appearance over time but is limited to free-viewpoint video replay of the same motion. Interactive animation from 4D performance capture has so far been limited to surface shape only. 4DVT is the final piece in the puzzle enabling video-realistic interactive animation through two contributions: a layered view-dependent texture map representation which supports efficient storage, transmission and rendering from multiple view video capture; and a rendering approach that combines multiple 4DVT sequences in a parametric motion space, maintaining video quality rendering of dynamic surface appearance whilst allowing high-level interactive control of character motion and viewpoint. 4DVT is demonstrated for multiple characters and evaluated both quantitatively and through a user-study which confirms that the visual quality of captured video is maintained. The 4DVT representation achieves >90% reduction in size and halves the rendering cost.
This paper presents a framework for creating realistic virtual characters that can be delivered via the Internet and interactively controlled in a WebGL enabled web-browser. Four-dimensional performance capture is used to capture realistic human motion and appearance. The captured data is processed into efficient and compact representations for geometry and texture. Motions are analysed against a high-level, user-defined motion graph and suitable inter- and intra-motion transitions are identified. This processed data is stored on a webserver and downloaded by a client application when required. A Javascript-based character animation engine is used to manage the state of the character which responds to user input and sends required frames to a WebGL-based renderer for display. Through the efficient geometry, texture and motion graph representations, a game character capable of performing a range of motions can be represented in 40-50 MB of data. This highlights the potential use of four-dimensional performance capture for creating web-based content. Datasets are made available for further research and an online demo is provided.
We present a convolutional autoencoder that enables high fidelity volumetric reconstructions of human performance to be captured from multi-view video comprising only a small set of camera views. Our method yields similar end-to-end reconstruction error to that of a prob- abilistic visual hull computed using significantly more (double or more) viewpoints. We use a deep prior implicitly learned by the autoencoder trained over a dataset of view-ablated multi-view video footage of a wide range of subjects and actions. This opens up the possibility of high-end volumetric performance capture in on-set and prosumer scenarios where time or cost prohibit a high witness camera count.
Light-field video has recently been used in virtual and augmented reality applications to increase realism and immersion. However, existing light-field methods are generally limited to static scenes due to the requirement to acquire a dense scene representation. The large amount of data and the absence of methods to infer temporal coherence pose major challenges in storage, compression and editing compared to conventional video. In this paper, we propose the first method to extract a spatio-temporally coherent light-field video representation. A novel method to obtain Epipolar Plane Images (EPIs) from a spare lightfield camera array is proposed. EPIs are used to constrain scene flow estimation to obtain 4D temporally coherent representations of dynamic light-fields. Temporal coherence is achieved on a variety of light-field datasets. Evaluation of the proposed light-field scene flow against existing multiview dense correspondence approaches demonstrates a significant improvement in accuracy of temporal coherence.
This paper presents techniques to animate realistic human-like motion using a compressed learnt model from 4D volumetric performance capture data. Sequences of 4D dynamic geometry representing a human performing an arbitrary motion are encoded through a generative network into a compact space representation, whilst maintaining the original properties, such as, surface dynamics. An animation framework is proposed which computes an optimal motion graph using the novel capabilities of compression and generative synthesis properties of the network. This approach significantly reduces the memory space requirements, improves quality of animation, and facilitates the interpolation between motions. The framework optimises the number of transitions in the graph with respect to the shape and motion of the dynamic content. This generates a compact graph structure with low edge connectivity, and maintains realism when transitioning between motions. Finally, it demonstrates that generative networks facilitate the computation of novel poses, and provides a compact motion graph representation of captured dynamic shape enabling real-time interactive animation and interpolation of novel poses to smoothly transition between motions.
We propose a novel framework to reconstruct super-resolution human shape from a single low-resolution input image. The approach overcomes limitations of existing approaches that reconstruct 3D human shape from a single image, which require high-resolution images together with auxiliary data such as surface normal or a parametric model to reconstruct high-detail shape. The proposed framework represents the reconstructed shape with a high-detail implicit function. Analogous to the objective of 2D image super-resolution, the approach learns the mapping from a low-resolution shape to its high-resolution counterpart and it is applied to reconstruct 3D shape detail from low-resolution images. The approach is trained end-to-end employing a novel loss function which estimates the information lost between a low and high-resolution representation of the same 3D surface shape. Evaluation for single image reconstruction of clothed people demonstrates that our method achieves high-detail surface reconstruction from low-resolution images without auxiliary data. Extensive experiments show that the proposed approach can estimate super-resolution human geometries with a significantly higher level of detail than that obtained with previous approaches when applied to low-resolution images. https://marcopesavento.github.io/SuRS/.
A common problem in the 4D reconstruction of people from multi-view video is the quality of the captured dynamic texture appearance which depends on both the camera resolution and capture volume. Typically the requirement to frame cameras to capture the volume of a dynamic performance (> 50m 3 ) results in the person occupying only a small proportion < 10% of the field of view. Even with ultra high-definition 4k video acquisition this results in sampling the person at less-than standard definition 0.5k video resolution resulting in low-quality rendering. In this paper we propose a solution to this problem through super-resolution appearance transfer from a static high-resolution appearance capture rig using digital stills cameras (> 8k) to capture the person in a small volume (< 8m 3 ). A pipeline is proposed for super-resolution appearance transfer from high-resolution static capture to dynamic video performance capture to produce super-resolution dynamic textures. This addresses two key problems: colour mapping between different camera systems; and dynamic texture map super-resolution using a learnt model. Comparative evaluation demonstrates a significant qualitative and quantitative improvement in rendering the 4D performance capture with super-resolution dynamic texture appearance. The proposed approach reproduces the high-resolution detail of the static capture whilst maintaining the appearance dynamics of the captured video.
This paper presents a hybrid skeleton-driven surface registration (HSDSR) approach to generate temporally consistent meshes from multiple view video of human subjects. 2D pose detections from multiple view video are used to estimate 3D skeletal pose on a per-frame basis. The 3D pose is embedded into a 3D surface reconstruction allowing any frame to be reposed into the shape from any other frame in the captured sequence. Skeletal motion transfer is performed by selecting a reference frame from the surface reconstruction data and reposing it to match the pose estimation of other frames in a sequence. This allows an initial coarse alignment to be performed prior to refinement by a patch-based non-rigid mesh deformation. The proposed approach overcomes limitations of previous work by reposing a reference mesh to match the pose of a target mesh reconstruction, providing a closer starting point for further non-rigid mesh deformation. It is shown that the proposed approach is able to achieve comparable results to existing model-based and model-free approaches. Finally, it is demonstrated that this framework provides an intuitive way for artists and animators to edit volumetric video.
In immersive and interactive audio-visual content, there is very significant scope for spatial misalignment between the two main modalities. So, in productions that have both 3D video and spatial audio, the positioning of sound sources relative to the visual display requires careful attention. This may be achieved in the form of object-based audio, moreover allowing the producer to maintain control over individual elements within the mix. Yet each object?s metadata is needed to define its position over time. In the present study, audio-visual studio recordings were made of short scenes representing three genres: drama, sport and music. Foreground video was captured by a light-field camera array, which incorporated a microphone array, alongside more conventional sound recording by spot microphones and a first-order ambisonic room microphone. In the music scenes, a direct feed from the guitar pickup was also recorded. Video data was analysed to form a 3D reconstruction of the scenes, and human figure detection was applied to the 2D frames of the central camera. Visual estimates of the sound source positions were used to provide ground truth. Position metadata were encoded within audio definition model (ADM) format audio files, suitable for standard object-based rendering. The steered response power of the acoustical signals at the microphone array were used, with the phase transform (SRP-PHAT), to determine the dominant source position(s) at any time, and given as input to a Sequential Monte Carlo Probability Hypothesis Density (SMC-PHD) tracker. The output of this was evaluated in relation to the ground truth. Results indicate a hierarchy of accuracy in azimuth, elevation and range, in accordance with human spatial auditory perception. Azimuth errors were within the tolerance bounds reported by studies of the Ventriloquism Effect, giving an initial promising indication that such an approach may open the door to object-based production for live events.
Multi-view video acquisition is widely used for reconstruction and free-viewpoint rendering of dynamic scenes by directly resampling from the captured images. This paper addresses the problem of optimally resampling and representing multi-view video to obtain a compact representation without loss of the view-dependent dynamic surface appearance. Spatio-temporal optimisation of the multi-view resampling is introduced to extract a coherent multi-layer texture map video. This resampling is combined with a surface-based optical flow alignment between views to correct for errors in geometric reconstruction and camera calibration which result in blurring and ghosting artefacts. The multi-view alignment and optimised resampling results in a compact representation with minimal loss of information allowing high-quality free-viewpoint rendering. Evaluation is performed on multi-view datasets for dynamic sequences of cloth, faces and people. The representation achieves >90% compression without significant loss of visual quality.
4D Video Textures (4DVT) introduce a novel representation for rendering video-realistic interactive character animation from a database of 4D actor performance captured in a multiple camera studio. 4D performance capture reconstructs dynamic shape and appearance over time but is limited to free-viewpoint video replay of the same motion. Interactive animation from 4D performance capture has so far been limited to surface shape only. 4DVT is the final piece in the puzzle enabling video-realistic interactive animation through two contributions: a layered view-dependent texture map representation which supports efficient storage, transmission and rendering from multiple view video capture; and a rendering approach that combines multiple 4DVT sequences in a parametric motion space, maintaining video quality rendering of dynamic surface appearance whilst allowing high-level interactive control of character motion and viewpoint. 4DVT is demonstrated for multiple characters and evaluated both quantitatively and through a user-study which confirms that the visual quality of captured video is maintained. The 4DVT representation achieves >90% reduction in size and halves the rendering cost.