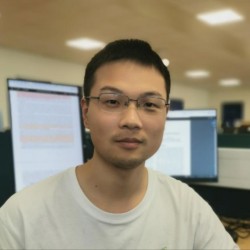
Li Qiao
Academic and research departments
Institute for Communication Systems, Faculty of Engineering and Physical Sciences.About
My research project
On efficient multiple access for AIoT networksThis project aims to investigate and propose efficient multiple access mechanisms to optimize communication efficiency among massive devices in future wireless networks. By conducting a thorough analysis of the technical challenges and requirements associated with multiple access, the project seeks to design a solution that enhances network capacity, reduces conflicts, and improves data transmission efficiency. The ultimate goal is to contribute to the advancement of next-generation wireless networks, making them more streamlined and intelligent. The source code for the project will be accessible to everyone at: gaozhen16.github.io
Supervisors
This project aims to investigate and propose efficient multiple access mechanisms to optimize communication efficiency among massive devices in future wireless networks. By conducting a thorough analysis of the technical challenges and requirements associated with multiple access, the project seeks to design a solution that enhances network capacity, reduces conflicts, and improves data transmission efficiency. The ultimate goal is to contribute to the advancement of next-generation wireless networks, making them more streamlined and intelligent. The source code for the project will be accessible to everyone at: gaozhen16.github.io
ResearchResearch interests
My current research interests include massive IoT connectivity, federated edge intelligence, and massive MIMO systems.
Research interests
My current research interests include massive IoT connectivity, federated edge intelligence, and massive MIMO systems.
Publications
This paper proposes a grant-free massive access scheme based on the millimeter wave (mmWave) extra-large-scale multiple-input multiple-output (XL-MIMO) to support massive Internet-of-Things (IoT) devices with low latency, high data rate, and high localization accuracy in the next-generation networks.
This paper introduces a digital over-the-air computation scheme based on unsourced massive access protocols, thereby enhancing compatibility with both present and future wireless networks.
A non-coherent grant-free random access scheme is proposed in MIMO-OFDM systems, enabling efficient massive access for cost- and energy-limited IoT devices.
As electromagnetic materials continue to advance, media-based modulation (MBM) has emerged as a promising technique to improve the energy and spectrum efficiency of transmitters. This paper suggests an effective grant-free random access scheme tailored for IoT devices employing MBM.
This letter proposes a media modulation based massive machine-type communications solution for increasing the throughput, where a massive multi-input multi-output based base station is used for enhancing the detection performance.
The grant-free non-coherent index-modulation (NC-IM) has been recently emerging as an efficient embedded information transmission scheme for massive machine-type communications without channel state information, especially for cost-efficient Internet-of- Things (IoT) devices with small data packets. In this paper, we combine the grant-free NC-IM with OFDM for overcoming frequency-selective channel fading. Specifically, each device is allocated with a unique signature sequence codebook and active device transmits one of its signature sequences in each subcarrier. Information is embedded in the index of the transmitted signature sequence. By exploiting the structured sparsity of the equivalent channel matrix in the spatial domain and the common device activity in the subcarrier domain, we propose an efficient space-frequency joint activity and blind information detection algorithm with significantly improved device activity and embedded information detection performance. Simulation results verify the superiority of the proposed algorithm over state-of-the-art algorithms.
The Internet of Things promises the massive connectivity of everything ubiquitously, whereas enabling massive machine-type communications (mMTC) is one of the paramount hurdles. To this end, a new paradigm is conceived for mMTC by employing spatial modulation at the devices for enhancing throughput and massive multi-input multi-output at the base station with improved detection performance. However, the associated massive access poses the intractable active device and data detection challenge. In this paper, we formulate the massive access problem as sparse signal recovery problem by exploiting the sporadic traffic of mMTC. To solve this problem, we propose a joint structured approximate message passing (JS-AMP) algorithm for joint active device and data detection. Specifically, we use AMP to decouple the superimposed received signal into uncoupled scalar elements. Furthermore, to achieve enhanced data detection performance, we compute the posterior estimation of each scalar element by using the structured sparsity of spatial modulated symbols. Moreover, we estimate the device activity by exploiting expectation maximization, where the block sparsity of successive time slots is considered for improving performance. Finally, simulation results demonstrate that the proposed solution exhibits a significant performance gain over the state-of- the-art solutions.
Millimeter-wave (mmWave) extra-large scale multiple-input-multiple-output (XL-MIMO) is a promising technique for achieving high data rates in the upcoming sixth-generation communication networks. This paper considers an indoor massive Internet-of-Things (IoT) access scenario served by mmWave XL-MIMO, where the wireless channels exhibit spatial non-stationarity and the coexistence of far-field and near-field communication. By analyzing and exploiting such mmWave XL-MIMO channels, we propose a low-latency grant-free massive IoT access scheme based on joint active user detection (AUD) and channel estimation (CE). Specifically, by exploiting the common user activity in different pilot subcarriers and the block sparsity of the angular-domain XL-MIMO channels, we propose a low-complexity generalized multiple measurement vector-joint AUD and CE algorithm for efficient indoor massive access. Simulation results verify that the proposed solutions outperform the state-of-the-art greedy compressive sensing-based schemes in terms of AUD and CE performance.
This paper proposes a model-driven deep learning based channel feedback and multi-user precoding scheme for cell-free massive MIMO systems, where the downlink pilot signals, CSI compressor (from received pilots to quantized bits) at user equipments (UEs), CSI reconstruction at BSs, and multi-user precoding are designed. Specifically, based on the proposed Transformer-based auto-encoder, the non-orthogonal downlink pilots from different BSs, the CSI compressor at UEs, and the CSI reconstruction (from bits to CSI matrix) at different BSs are end-to-end trained in a distributed manner. Moreover, by utilizing the angular-domain reciprocity of downlink/uplink channels, the CSI reconstruction at the BSs can be further improved with the aid of uplink CSI, which can be easily obtained at the UEs' initial access stage. Additionally, we propose a model-driven deep unfolding based multi-user precoding by unfolding the conventional zero-forcing algorithm and integrating learnable parameters, which substantially reduces the computational complexity and improves the robustness to imperfect CSI.
The advent of the sixth-generation (6G) of wireless communications has given rise to the necessity to connect vast quantities of heterogeneous wireless devices, which requires advanced system capabilities far beyond existing network architectures. In particular, such massive communication has been recognized as a prime driver that can empower the 6G vision of future ubiquitous connectivity, supporting Internet of Human-Machine-Things for which massive access is critical. This paper surveys the most recent advances toward massive access in both academic and industry communities, focusing primarily on the promising compressive sensing-based grant-free massive access paradigm. We first specify the limitations of existing random access schemes and reveal that the practical implementation of massive communication relies on a dramatically different random access paradigm from the current ones mainly designed for human-centric communications. Then, a compressive sensing-based grant-free massive access roadmap is presented, where the evolutions from single-antenna to large-scale antenna array-based base stations, from single-station to cooperative massive multiple-input multiple-output systems, and from unsourced to sourced random access scenarios are detailed. Finally, we discuss the key challenges and open issues to shed light on the potential future research directions of grant-free massive access.