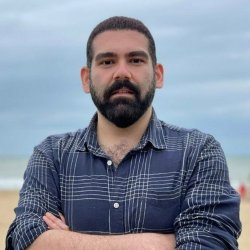
Dr Mahdi Boloursaz Mashhadi
Academic and research departments
Institute for Communication Systems, School of Computer Science and Electronic Engineering.About
Biography
Dr Mahdi Boloursaz Mashhadi (Senior Member, IEEE) is a Lecturer at the 5G/6G Innovation Centre (5G/6GIC) at the Institute for Communication Systems (ICS), School of Computer Science and Electronic Engineering (CSEE), University of Surrey, UK. Prior to joining ICS, he was a postdoctoral research associate at the Intelligent Systems and Networks (ISN) Research Group, Imperial College London, 2019-2021. He received B.S., M.S., and Ph.D. degrees in mobile telecommunications from the Sharif University of Technology (SUT), Tehran, Iran, in 2011, 2013, and 2018, respectively. He was a visiting research associate with the University of Central Florida, Orlando, USA, in 2018, and Queen’s University, Ontario, Canada, in 2017. He has more than 40 peer reviewed publications and patents in the areas of wireless communications, machine learning, and signal processing. He received the Best Paper Award from the IEEE EWDTS 2012 conference, and the Exemplary Reviewer Award from the IEEE ComSoc in 2021 and 2022. He has served as a panel judge for the International Telecommunication Union (ITU) to evaluate innovative submissions on applications of AI/ML in 5G and beyond wireless networks since 2021. He is an associate editor for the Springer Nature Wireless Personal Communications Journal.
Affiliations and memberships
Fellow of the Higher Education Academy (FHEA)
Editor, Springer Nature Wireless Personal Communications Journal
News
ResearchResearch interests
My current research is focused at the intersection of AI and machine learning and wireless communications. I'm interested in the specific role of AI and machine learning in future generation wireless networks. I am working on generative AI for telecommunications, AIoT systems, and the joint design of smart machine learning agents and the underlying wireless network to achieve goal oriented and semantic communications. I am looking at the interactions between AI and wireless communications which can be either AI for wireless communications or wireless communications for collaborative/distributed/federated machine learning.
Research projects
TOWARDS UBIQUITOUS 3D OPEN RESILIENT NETWORK (TUDOR) (Co-PI)
The TUDOR Project is a £12M UK flagship research project funded by the Department of Science, Innovation and Technology (DSIT). It targets low technology readiness level (TRL) research, aiming to tackle strategic technical challenges oriented to the design of future 6G paradigm.
Start date: February 2023 - End date: January 2025.
Research interests
My current research is focused at the intersection of AI and machine learning and wireless communications. I'm interested in the specific role of AI and machine learning in future generation wireless networks. I am working on generative AI for telecommunications, AIoT systems, and the joint design of smart machine learning agents and the underlying wireless network to achieve goal oriented and semantic communications. I am looking at the interactions between AI and wireless communications which can be either AI for wireless communications or wireless communications for collaborative/distributed/federated machine learning.
Research projects
TOWARDS UBIQUITOUS 3D OPEN RESILIENT NETWORK (TUDOR) (Co-PI)
The TUDOR Project is a £12M UK flagship research project funded by the Department of Science, Innovation and Technology (DSIT). It targets low technology readiness level (TRL) research, aiming to tackle strategic technical challenges oriented to the design of future 6G paradigm.
Start date: February 2023 - End date: January 2025.
Supervision
Postgraduate research supervision
Post Doctoral Researchers:
-Dr. Daesung Yu, Researcher in AI for Communications
PhD Students:
-Xinkai Liu (PG/R - Comp Sci & Elec Eng, ICS)
-Sotiris Chatzimiltis (PG/R - Comp Sci & Elec Eng, ICS)
Alumni:
-Li Qiao (PG/R - Comp Sci & Elec Eng, ICS)
-Dr. Chunmei Xu, Researcher in Semantic Communications
-Tatsuya Kikuzuki, Visiting Researcher from Fujitsu Japan
-Mahnoosh Mahdavimoghadam (PG/R - Comp Sci & Elec Eng, ICS)
I am recruiting PhD students in Advanced Wireless and Distributed Data Processing to work on cutting-edge distributed learning technologies over 6G. Interested applicants send CV's to: m.boloursazmashhadi@surrey.ac.uk
Publications
Reconfigurable Intelligent Surfaces (RISs) are envisioned to be employed in next generation wireless networks to enhance the communication and radio localization services. In this paper, we propose novel localization and tracking algorithms exploiting reflections through RISs at multiple receivers. We utilize a single antenna transmitter (Tx) and multiple single antenna receivers (Rxs) to estimate the position and the velocity of users (e.g. vehicles) equipped with RISs. Then, we design the RIS phase shifts to separate the signals from different users. The proposed algorithms exploit the geometry information of the signal at the RISs to localize and track the users. We also conduct a comprehensive analysis of the Cramer-Rao lower bound (CRLB) of the localization system. Compared to the time of arrival (ToA)-based localization approach, the proposed method reduces the localization error by a factor up to three. Also, the simulation results show the accuracy of the proposed tracking approach.
Split Federated Learning (SFL) improves scalability of Split Learning (SL) by enabling parallel computing of the learning tasks on multiple clients. However, state-of-the-art SFL schemes neglect the effects of heterogeneity in the clients’ computation and communication performance as well as the computation time for the tasks offloaded to the cloud server. In this paper, we propose a fine-grained parallelization framework, called PipeSFL, to accelerate SFL on heterogeneous clients. PipeSFL is based on two key novel ideas. First, we design a server-side priority scheduling mechanism to minimize per-iteration time. Second, we propose a hybrid training mode to reduce per-round time, which employs asynchronous training within rounds and synchronous training between rounds. We theoretically prove the optimality of the proposed priority scheduling mechanism within one round and analyze the total time per round for PipeSFL, SFL and SL. We implement PipeSFL on PyTorch. Extensive experiments on seven 64-client clusters with different heterogeneity demonstrate that at training speed, PipeSFL achieves up to 1.65x and 1.93x speedup compared to EPSL and SFL, respectively. At energy consumption, PipeSFL saves up to 30.8% and 43.4% of the energy consumed within each training round compared to EPSL and SFL, respectively. PipeSFL’s code is available at https://github.com/ZJU-CNLAB/PipeSFL.
—Recent advancements in diffusion models have led to a significant breakthrough in generative modeling. The combination of the generative model and semantic communication (SemCom) enables high-fidelity semantic information exchange at ultra-low rates. In this paper, a novel generative SemCom framework for image tasks is proposed, utilizing pre-trained foundation models as semantic encoders and decoders for semantic feature extraction and image regeneration, respectively. The mathematical relationship between transmission reliability and the perceptual quality of regenerated images is modeled and the semantic values of extracted features are defined accordingly. This relationship is derived through numerical simulations on the Kodak dataset. Furthermore, we investigate the semantic-aware power allocation problem, aiming to minimize total power consumption while guaranteeing semantic performance. To solve this problem, two semantic-aware power allocation methods are proposed by constraint decoupling and bisection search, respectively. Numerical results demonstrate that the proposed semantic-aware methods outperform conventional approach in terms of total power consumption.
The ubiquitous availability of wireless networks and devices provides a unique opportunity to leverage the corresponding communication signals to enable wireless sensing applications. In this article, we develop a new framework for environment sensing by opportunistic use of the mmWave communication signals. The proposed framework is based on a mixture of the conventional and Neural Network (NN) signal processing techniques for simultaneous counting and localization of multiple targets in the environment in a bi-static setting. In this framework, multi-modal delay, Doppler, angular features are first derived from the Channel State Information (CSI) estimated at the receiver, and then a transformer-based NN architecture exploiting attention mechanisms, called CSIformer, is designed to extract the most effective features for sensing. We also develop a novel post-processing technique based on Kullback-Leibler (KL) minimization to transfer knowledge between the counting and localization tasks, thereby simplifying the NN architecture. Our numerical results show accurate counting and localization capabilities that significantly outperform the existing works based on pure conventional signal processing techniques, as well as NN-based approaches. The simulation codes are available at: https://github.com/University-of-Surrey-Mahdi/Attention-on-the-Preambles-Sensing-with-mmWave-CSI.
Massive multiple-input multiple-output (MIMO) systems require downlink channel state information (CSI) at the base station (BS) to better utilize the available spatial diversity and multiplexing gains. However, in a frequency division duplex (FDD) massive MIMO system, the huge CSI feedback overhead becomes restrictive and degrades the overall spectral efficiency. In this paper, we propose a deep learning based channel state matrix compression scheme, called DeepCMC, composed of convolutional layers followed by quantization and entropy coding blocks. Simulation results demonstrate that DeepCMC significantly outperforms the state of the art compression schemes in terms of the reconstruction quality of the channel state matrix for the same compression rate, measured in bits per channel dimension.
This paper devises a novel adaptive framework for the energy-aware acquisition of spectrally sparse signals. The adaptive quantized compressive sensing (CS) techniques, beyond-complementary metal-oxide-semiconductor (CMOS) hardware architecture, and corresponding algorithms which utilize them have been designed concomitantly to minimize the overall of signal acquisition. First, a spin-based adaptive intermittent quantizer (AIQ) is developed to facilitate the realization of the adaptive sampling technique. Next, a framework for smart and adaptive determination of the sampling rate and quantization resolution based on the instantaneous signal and hardware constraints is introduced. Finally, signal reconstruction algorithms which process the quantized CS samples are investigated. Simulation results indicate that an AIQ architecture using a spin-based quantizer incurs only 20.98-mu W power dissipation on average using 22-nm technology for 1-8 bits uniform output. Furthermore, in order to provide 8-bit quantization resolution, 85.302-mu W maximum power dissipation is attained. Our results indicate that the proposed AIQ design provides up to 6.18-mW power savings on average compared to other adaptive rate and resolution CMOS-based CS analog-to-digital converter designs. In addition, the mean square error values achieved by the simulation results confirm efficient reconstruction of the signal based on the proposed approach.
Cellular networks provide widespread and reliable voice communications among subscribers through mobile voice channels. These channels benefit from superior priority and higher availability compared with conventional cellular data communication services, such as General Packet Radio Service, Enhanced Data Rates for GSM Evolution, and High-Speed Downlink Packet Access. These properties are of major interest to applications that require transmitting small volumes of data urgently and reliably, such as an emergency call in vehicular applications. This encourages excessive research to make digital communication through voice channels feasible, leading to the emergence of Data over Voice (DoV) technology. In this research, we investigate the challenges of transmitting data through mobile voice channels. We introduce a simplified information-theoretic model of the vocoder channel and derive bounds on its capacity. By invoking detection theory concepts and conjecturing Weibull and chi-square distributions for approximately modeling the probability distribution of channel output, we propose improved detection schemes based on the mentioned distributions and compare the achieved performances with the calculated bounds and other state-of-the-art DoV structures. Moreover, in common mobile networks, the vocoder compression rate is adopted in accordance with the network traffic adaptively. Although this phenomenon affects the overall capacity significantly, it has been overlooked by previous research studies. In this research, we apply the Gilbert-Elliott (GE) model to the voice channel, extract the required model parameters from the Markov model, and bound the overall voice channel capacity by considering the adaptive rate adjustment phenomenon.
This paper studies the problem of Simultaneous Sparse Approximation (SSA). This problem arises in many applications which work with multiple signals maintaining some degree of dependency such as radar and sensor networks. In this paper, we introduce a new method towards joint recovery of several independent sparse signals with the same support. We provide an analytical discussion on the convergence of our method called Simultaneous Iterative Method with Adaptive Thresholding (SIMAT). Additionally, we compare our method with other group-sparse reconstruction techniques, i.e., Simultaneous Orthogonal Matching Pursuit (SOMP), and Block Iterative Method with Adaptive Thresholding (BIMAT) through numerical experiments. The simulation results demonstrate that SIMAT outperforms these algorithms in terms of the metrics Signal to Noise Ratio (SNR) and Success Rate (SR). Moreover, SIMAT is considerably less complicated than BIMAT, which makes it feasible for practical applications such as implementation in MIMO radar systems.
The increased penetration of cellular networks has made voice channels widely available ubiquitously. On the other hand, mobile voice channels possess properties that make them an ideal choice for high priority, low-rate real-time communications. Mobile voice channel with the mentioned properties, could be utilised in emergency applications in vehicular communications area such as the standardised emergency call system planned to be launched in 2015. This study aims to investigate the challenges of data transmission through these channels and proposes an efficient data transfer structure. To this end, a proper statistical model for the channel distortion is proposed and an optimum detector is derived considering the proposed channel model. Optimum symbols are also designed according to the derived rule and analytical bounds on error probability are obtained for the orthogonal signaling and sphere packing techniques. Moreover, analytical evaluation is performed and appropriate simulation results are presented. Finally, it is observed that the proposed structure based on the sphere packing technique achieves superior performance compared with prior works in this field. Although the ideas offered in this study are utilised to cope with voice channel non-idealities, the steps taken in this study could also be applied to channels with similar conditions.
This paper considers the problem of sparse signal reconstruction from the timing of its Level Crossings (LC)s. We formulate the sparse Zero Crossing (ZC) reconstruction problem in terms of a single 1-bit Compressive Sensing (CS) model. We also extend the Smoothed L0 (SL0) sparse reconstruction algorithm to the 1-bit CS framework and propose the Binary SL0 (BSL0) algorithm for iterative reconstruction of the sparse signal from ZCs in cases where the number of sparse coefficients is not known to the reconstruction algorithm a priori. Similar to the ZC case, we propose a system of simultaneously constrained signed-CS problems to reconstruct a sparse signal from its Level Crossings (LC) s and modify both the Binary Iterative Hard Thresholding (BIHT) and BSL0 algorithms to solve this problem. Simulation results demonstrate superior performance of the proposed LC reconstruction techniques in comparison with the literature.
This paper considers the problem of digital data transmission through the Global System for Mobile communications (GSM) for security applications. A data modem is presented that utilizes codebooks of Speech-Like (SL) symbols to transmit data through the GSM Adaptive Multi Rate (AMR) voice codec. Using this codebook of finite alphabet, the continuous vocoder channel is modeled by a Discrete Memory less Channel (DMC). A heuristic optimization algorithm is proposed to select codebook symbols from a database of observed human speech such that the capacity of DMC is maximized. Using the DMC capacity, a lower bound on the capacity of the considered voice channel can be achieved. Simulation results show that the proposed data modem achieves higher data rates and lower symbol error rates compared to previously reported results while requiring lower computational complexity for codebook optimization.
Causal Heart Rate (HR) monitoring using photoplethysmographic (PPG) signals recorded from wrist during physical exercise is a challenging task because the PPG signals in this scenario are highly contaminated by artifacts caused by hand movements of the subject. This paper proposes a novel algorithm for this problem, which consists of two main blocks of Noise Suppression and Peak Selection. The Noise Suppression block removes Motion Artifacts (MAs) from the PPG signals utilizing simultaneously recorded 3D acceleration data. The Peak Selection block applies some decision mechanisms to correctly select the spectral peak corresponding to HR in PPG spectra. Experimental results on benchmark dataset recorded from 12 subjects during fast running at the peak speed of 15 km/hour showed that the proposed algorithm achieves an average absolute error of 1.50 beats per minute (BPM), which outperforms state of the art.
This paper presents a low complexity while accurate Heart Rate (HR) estimation technique from signals captured by Photoplethysmographic (PPG) sensors worn on the wrist during intensive physical exercise. Wrist-type PPG signals experience severe Motion Artifacts (MA) that hinder efficient HR estimation especially during intensive physical exercises. To suppress the motion artifacts efficiently, simultaneous 3 dimensional acceleration signals are used as reference MAs. The proposed method achieves an Average Absolute Error (AAE) of 1.19 Beats Per Minute (BPM) on the 12 benchmark PPG recordings in which subjects run at speeds of up to 15 km/h. This method also achieves an AAE of 2.17 BPM on the whole benchmark database of 23 recordings that include both running and arm movement activities. This performance is comparable with state-of-the-art algorithms while at a significantly reduced computational cost which makes its standalone implementation on wearable devices feasible. The proposed algorithm achieves an average processing time of 32 milliseconds per input frames of length 8 seconds (2 channel PPG and 3D ACC signals) on a 3.2 GHz processor.
PPG based heart rate (HR) monitoring has recently attracted much attention with the advent of wearable devices such as smart watches and smart bands. However, due to severe motion artifacts (MA) caused by wristband stumbles, PPG based HR monitoring is a challenging problem in scenarios where the subject performs intensive physical exercises. This work proposes a novel approach to the problem based on supervised learning by Neural Network (NN). By simulations on the benchmark datasets [1], we achieve acceptable estimation accuracy and improved run time in comparison with the literature. A major contribution of this work is that it alleviates the need to use simultaneous acceleration signals. The simulation results show that although the proposed method does not process the simultaneous acceleration signals, it still achieves the acceptable Mean Absolute Error (MAE) of 1.39 Beats Per Minute (BPM) on the benchmark data set.
This paper studies the problem of Simultaneous Sparse Approximation (SSA). This problem arises in many applications that work with multiple signals maintaining some degree of dependency, e.g., radar and sensor networks. We introduce a new method towards joint recovery of several independent sparse signals with the same support. We provide an analytical discussion of the convergence of our method, called Simultaneous Iterative Method (SIM). In this study, we compared our method with other group-sparse reconstruction techniques, namely Simultaneous Orthogonal Matching Pursuit (SOMP) and Block Iterative Method with Adaptive Thresholding (BIMAT), through numerical experiments. The simulation results demonstrated that SIM outperformed these algorithms in terms of the metrics Signal to Noise Ratio (SNR) and Success Rate (SR). Moreover, SIM is considerably less complicated than BIMAT, which makes it feasible for practical applications such as implementation in MIMO radar systems.
The common voice channels existing in cellular communication networks provide reliable, ubiquitously available and top priority communication mediums. These properties make voice dedicated channels an ideal choice for high priority, real time communication. However, such channels include voice codecs that hamper the data flow by compressing the waveforms prior to transmission. This study designs codebooks of speech-like symbols for reliable data transfer through the voice channel of cellular networks. An efficient algorithm is proposed to select proper codebook symbols from a database of natural speech to optimise a desired objective. Two variants of this codebook optimisation algorithm are presented: One variant minimises the symbol error rate and the other maximises the capacity achievable by the codebook. It is shown both analytically and by the simulation results that under certain circumstances, these two objective functions reach the same performance. Simulation results also show that the proposed codebook optimisation algorithm achieves higher data rates and lower symbol error rates compared with previously reported results while requiring lower computational complexity for codebook optimisation. The Gilbert–Elliot channel model is utilised to study the effects of adaptive compression rate adjustment of the vocoder on overall voice channel capacity. Finally, practical implementation issues are addressed.
The authors propose asynchronous level crossing (LC) A/D converters for low redundancy voice sampling. They propose to utilise the family of iterative methods with adaptive thresholding (IMAT) for reconstructing voice from non-uniform LC and adaptive LC (ALC) samples thereby promoting sparsity. The authors modify the basic IMAT algorithm and propose the iterative method with adaptive thresholding for level crossing (IMATLC) algorithm for improved reconstruction performance. To this end, the authors analytically derive the basic IMAT algorithm by applying the gradient descent and gradient projection optimisation techniques to the problem of square error minimisation subjected to sparsity. The simulation results indicate that the proposed IMATLC reconstruction method outperforms the conventional reconstruction method based on low-pass signal assumption by 6.56dBs in terms of reconstruction signal-to-noise ratio (SNR) for LC sampling. In this scenario, IMATLC outperforms orthogonal matching pursuit, least absolute shrinkage and selection operator and smoothed L0 sparsity promoting algorithms by average amounts of 12.13, 10.31, and 10.28dBs, respectively. Finally, the authors compare the performance of the proposed LC/ALC-based A/Ds with the conventional uniform sampling-based A/Ds and their random sampling-based counterparts both in terms of perceptual evaluation of speech quality and reconstruction SNR.
This paper considers the problem of interpolating signals defined on graphs. A major presumption considered by many previous approaches to this problem has been low-pass/band-limitedness of the underlying graph signal. However, inspired by the findings on sparse signal reconstruction, we consider the graph signal to be rather sparse/compressible in the Graph Fourier Transform (GFT) domain and propose the Iterative Method with Adaptive Thresholding for Graph Interpolation (IMATGI) algorithm for sparsity promoting interpolation of the underlying graph signal. We analytically prove convergence of the proposed algorithm. We also demonstrate efficient performance of the proposed IMATGI algorithm in reconstructing randomly generated sparse graph signals. Finally, we consider the widely desirable application of recommendation systems and show by simulations that IMATGI outperforms state-of-the-art algorithms on the benchmark datasets in this application.
Global System for Mobile communications (GSM) is a widely spread, reliable and P2P channel through all over the world. These characteristics make GSM a channel suitable for a variety of applications in different domains especially security applications such as secure voice communication. Performance and usage of GSM applications extremely depends on the transmission data rate. Hence, transmitting data over GSM is still an attractive topic for research. This paper considers the problem of digital data transmission through the GSM voice channel. A lower capacity bound for data transmission through the GSM Adaptive Multi Rate (AMR) voice codec is presented. The GSM channel is modeled in a simple manner to overcome it's memory and non-linearity effects. A new statistic based on received samples is extracted and a novel method to transmit data over that channel which asymptotically tends to the achieved lower bound is offered.
This paper considers the problem of secure data communication through the Global System for Mobile communications (GSM). The algebraic codebook method for data transmission through the Adaptive Multi Rate 12.2Kbps voice channel is investigated and its maximum achievable data rate is calculated. Based on the vocoder channel properties, the method's Bit Error Rate (BER) performance is improved by repetition coding and classification methods. Simulation results show that by simultaneous application of repetition coding and clustering methods, the decoder's performance improves about 6.5% compared to the case of no clustering for 1Kbps data communication in AMR 4.75 voice codec.
The voice channels present in cellular communication networks provide reliable, widespread and high priority communication mediums. Using these voice channels as a bearer for data transmission allows to deliver high Quality of Service data. But voice channels include vocoders that hinder the data flow by compressing the waveforms prior to transmission. Calculating vocoder channel capacity remains a challenging problem since no analytical model has been proposed for the vocoder channel so far. In this research, simplified models for the vocoder channel are proposed and bounds on vocoder channel capacity are derived based on them. In common cellular networks, the vocoder compression rate is adjusted adaptively according to the network's traffic conditions which further complicates calculating an overall capacity for the voice channel. In this research, the Gilbert-Elliot channel model is applied to the cellular voice channel to enable the study of the effect of adaptive vocoder rate adjustment on overall voice channel capacity. Modeling the voice channel and calculating its capacity provides reference bounds for comparison with any newly proposed communication scheme over this channel.
In this letter, we propose a sparsity promoting feedback acquisition and reconstruction scheme for sensing, encoding and subsequent reconstruction of spectrally sparse signals. In the proposed scheme, the spectral components are estimated utilizing a sparsity-promoting, sliding-window algorithm in a feedback loop. Utilizing the estimated spectral components, a level signal is predicted and sign measurements of the prediction error are acquired. The sparsity promoting algorithm can then estimate the spectral components iteratively from the sign measurements. Unlike many batch-based compressive sensing algorithms, our proposed algorithm gradually estimates and follows slow changes in the sparse components utilizing a sliding-window technique. We also consider the scenario in which possible flipping errors in the sign bits propagate along iterations (due to the feedback loop) during reconstruction. We propose an iterative error correction algorithm to cope with this error propagation phenomenon considering a binary-sparse occurrence model on the error sequence. Simulation results show effective performance of the proposed scheme in comparison with the literature.
This paper considers the problem of digital data transmission through the Global System for Mobile communications (GSM). A data modem is presented that utilizes codebooks of Speech-Like (SL) symbols to transmit data through the GSM Adaptive Multi Rate (AMR) voice codec. Using this codebook of finite alphabet, the continuous vocoder channel is modeled by a Discrete Memory less Channel (DMC). A heuristic optimization algorithm is proposed to select codebook symbols from a database of observed human speech such that the capacity of DMC is maximized. Simulation results show that the proposed data modem achieves higher data rates and lower symbol error rates compared to previously reported results while requiring lower computational complexity for codebook optimization.
Massive multiple-input multiple-output (MIMO) systems require downlink channel state information (CSI) at the base station (BS) to better utilize the available spatial diversity and multiplexing gains. However, in a frequency division duplex (FDD) massive MIMO system, CSI feedback overhead degrades the overall spectral efficiency. Deep Learning (DL)based CSI feedback compression schemes have received a lot of attention recently as they provide significant improvements in compression efficiency; however, they still require reliable feedback links to convey the compressed CSI information to the BS. Instead, we propose here a Convolutional neural network (CNN)-based analog feedback scheme, called AnalogDeepCMC, which directly maps the downlink CSI to uplink channel input. Corresponding noisy channel outputs are used by another CNN to reconstruct the downlink channel estimate. The proposed analog scheme not only outperforms existing digital CSI feedback schemes in terms of the achievable downlink rate, but also simplifies the feedback transmission as it does not require explicit quantization, coding, and modulation, and provides a low-latency alternative particularly in rapidly changing MIMO channels, where the CSI needs to be estimated and fed back periodically.
—With the huge number of broadband users, automated network management becomes of huge interest to service providers. A major challenge is automated monitoring of user Quality of Experience (QoE), where Artificial Intelligence (AI) and Machine Learning (ML) models provide powerful tools to predict user QoE from basic protocol indicators such as Round Trip Time (RTT), retransmission rate, etc. In this paper, we introduce an effective feature selection method along with the corresponding classification algorithms to address this challenge. The simulation results show a prediction accuracy of 78% on the benchmark ITU ML5G-PS-012 dataset, improving 11% over the state-of-the-art result whilst reducing the model complexity at the same time. Moreover, we show that the local area network round trip time (LAN RTT) value during daytime and midweek plays the most prominent factor affecting the user QoE.
In this paper, we consider an uplink transmission of a multiuser single-input multiple-output (SIMO) system assisted with multiple reconfigurable intelligent surfaces (RISs). We investigate the energy efficiency (EE) maximization problem with an electromagnetic field (EMF) exposure constraint. In order to solve the problem, we present a lower bound for the EE and adopt an alternate optimization problem. Then, we propose the Energy Efficient Multi-RIS (EEMR) algorithm to obtain the optimal transmit power of the users and phase shifts of the RISs. Moreover, we study this problem for a system with a central RIS and compare the results. The simulation results show that for a sufficient total number of RIS elements, the system with distributed RISs is more energy efficient compared to the system with a central RIS. In addition, for both the systems the EMF exposure constraints enforce a trade-off between the EE and EMF-awareness of the system.
In this letter, we investigate the signal-to-interference-plus-noise-ratio (SINR) maximization problem in a multi-user massive multiple-input-multiple-output (massive MIMO) system enabled with multiple reconfigurable intelligent surfaces (RISs). We examine two zero-forcing (ZF) beamforming approaches for interference management namely BS-UE-ZF and BS-RIS-ZF that enforce the interference to zero at the users (UEs) and the RISs, respectively. Then, for each case, we resolve the SINR maximization problem to find the optimal phase shifts of the elements of the RISs. Also, we evaluate the asymptotic expressions for the optimal phase shifts and the maximum SINRs when the number of the base station (BS) antennas tends to infinity. We show that if the channels of the RIS elements are independent and the number of the BS antennas tends to infinity, random phase shifts achieve the maximum SINR using the BS-UE-ZF beamforming approach. The simulation results illustrate that by employing the BS-RIS-ZF beamforming approach, the asymptotic expressions of the phase shifts and maximum SINRs achieve the rate obtained by the optimal phase shifts even for a small number of the BS antennas.
—Generative foundation AI models have recently shown great success in synthesizing natural signals with high perceptual quality using only textual prompts and conditioning signals to guide the generation process. This enables semantic communications at extremely low data rates in future wireless networks. In this paper, we develop a latency-aware semantic communications framework with pre-trained generative models. The transmitter performs multi-modal semantic decomposition on the input signal and transmits each semantic stream with the appropriate coding and communication schemes based on the intent. For the prompt, we adopt a re-transmission-based scheme to ensure reliable transmission, and for the other semantic modalities we use an adaptive modulation/coding scheme to achieve robustness to the changing wireless channel. Furthermore , we design a semantic and latency-aware scheme to allocate transmission power to different semantic modalities based on their importance subjected to semantic quality constraints. At the receiver, a pre-trained generative model synthesizes a high fidelity signal using the received multi-stream semantics. Simulation results demonstrate ultra-low-rate, low-latency, and channel-adaptive semantic communications.
Massive multiple-input multiple-output (MIMO) systems require downlink channel state information (CSI) at the base station (BS) to achieve spatial diversity and multiplexing gains. In a frequency division duplex (FDD) multiuser massive MIMO network, each user needs to compress and feedback its downlink CSI to the BS. The CSI overhead scales with the number of antennas, users and subcarriers, and becomes a major bottleneck for the overall spectral efficiency. In this paper, we propose a deep learning (DL)-based CSI compression scheme, called DeepCMC , composed of convolutional layers followed by quantization and entropy coding blocks. In comparison with previous DL-based CSI reduction structures, DeepCMC proposes a novel fully-convolutional neural network (NN) architecture, with residual layers at the decoder, and incorporates quantization and entropy coding blocks into its design. DeepCMC is trained to minimize a weighted rate-distortion cost, which enables a trade-off between the CSI quality and its feedback overhead. Simulation results demonstrate that DeepCMC outperforms the state of the art CSI compression schemes in terms of the reconstruction quality of CSI for the same compression rate. We also propose a distributed version of DeepCMC for a multi-user MIMO scenario to encode and reconstruct the CSI from multiple users in a distributed manner. Distributed DeepCMC not only utilizes the inherent CSI structures of a single MIMO user for compression, but also benefits from the correlations among the channel matrices of nearby users to further improve the performance in comparison with DeepCMC. We also propose a reduced-complexity training method for distributed DeepCMC, allowing to scale it to multiple users, and suggest a cluster-based distributed DeepCMC approach for practical implementation.
This paper studies issues that arise with respect to the joint optimization for convergence time in federated learning over wireless networks (FLOWN). We consider the criterion and protocol for selection of participating devices in FLOWN under the energy constraint and derive its impact on device selection. In order to improve the training efficiency, age-of-information (AoI) enables FLOWN to assess the freshness of gradient updates among participants. Aiming to speed up convergence, we jointly investigate global loss minimization and latency minimization in a Stackelberg game based framework. Specifically, we formulate global loss minimization as a leader-level problem for reducing the number of required rounds, and latency minimization as a follower-level problem to reduce time consumption of each round. By decoupling the follower-level problem into two sub-problems, including resource allocation and sub-channel assignment, we achieve an optimal strategy of the follower through monotonic optimization and matching theory. At the leader-level, we derive an upper bound of convergence rate and subsequently refor-mulate the global loss minimization problem and propose a new age-of-update (AoU) based device selection algorithm. Simulation results indicate the superior performance of the proposed AoU based device selection scheme in terms of the convergence rate, as well as efficient utilization of available sub-channels.
Current technological advancements in Software Defined Networks (SDN) can provide efficient solutions for smart grids (SGs). An SDN-based SG promises to enhance the efficiency, reliability and sustainability of the communication network. However, new security breaches can be introduced with this adaptation. A layer of defence against insider attacks can be established using machine learning based intrusion detection system (IDS) located on the SDN application layer. Conventional centralised practises, violate the user data privacy aspect, thus distributed or collaborative approaches can be adapted so that attacks can be detected and actions can be taken. This paper proposes a new SDN-based SG architecture, highlighting the existence of IDSs in the SDN application layer. We implemented a new smart meter (SM) collaborative intrusion detection system (SM-IDS), by adapting the split learning methodology. Finally, a comparison of a federated learning and split learning neighbourhood area network (NAN) IDS was made. Numerical results showed, a five class classification accuracy of over 80.3% and F1-score 78.9 for a SM-IDS adapting the split learning technique. Also, the split learning NAN-IDS exhibit an accuracy of over 81.1% and F1-score 79.9.
The communication bottleneck severely constrains the scalability of distributed deep learning, and efficient communication scheduling accelerates distributed DNN training by overlapping computation and communication tasks. However, existing approaches based on tensor partitioning are not efficient and suffer from two challenges: (1) the fixed number of tensor blocks transferred in parallel can not necessarily minimize the communication overheads; (2) although the scheduling order that preferentially transmits tensor blocks close to the input layer can start forward propagation in the next iteration earlier, the shortest per-iteration time is not obtained. In this paper, we propose an efficient communication framework called US-Byte. It can schedule unequal-sized tensor blocks in a near-optimal order to minimize the training time. We build the mathematical model of US-Byte by two phases: (1) the overlap of gradient communication and backward propagation, and (2) the overlap of gradient communication and forward propagation. We theoretically derive the optimal solution for the second phase and efficiently solve the first phase with a low-complexity algorithm. We implement the US-Byte architecture on PyTorch framework. Extensive experiments on two different 8-node GPU clusters demonstrate that US-Byte can achieve up to 1.26x and 1.56x speedup compared to ByteScheduler and WFBP, respectively. We further exploit simulations of 128 GPUs to verify the potential scaling performance of US-Byte. Simulation results show that US-Byte can achieve up to 1.69x speedup compared to the state-of-the-art communication framework.
We present a new Deep Neural Network (DNN)-based error correction code for fading channels with output feedback, called the Deep SNR-Robust Feedback (DRF) code. At the encoder, parity symbols are generated by a Long Short Term Memory (LSTM) network based on the message, as well as the past forward channel outputs observed by the transmitter in a noisy fashion. The decoder uses a bidirectional LSTM architecture along with a Signal to Noise Ratio (SNR)-aware attention NN to decode the message. The proposed code overcomes two major shortcomings of DNN-based codes over channels with passive output feedback: (i) the SNR-aware attention mechanism at the decoder enables reliable application of the same trained NN over a wide range of SNR values; (ii) curriculum training with batch size scheduling is used to speed up and stabilize training while improving the SNR-robustness of the resulting code. We show that the DRF codes outperform the existing DNN-based codes in terms of both the SNR-robustness and the error rate in an Additive White Gaussian Noise (AWGN) channel with noisy output feedback. In fading channels with perfect phase compensation at the receiver, DRF codes learn to efficiently exploit knowledge of the instantaneous fading amplitude (which is available to the encoder through feedback) to reduce the overhead and complexity associated with channel estimation at the decoder. Finally, we show the effectiveness of DRF codes in multicast channels with feedback, where linear feedback codes are known to be strictly suboptimal. These results show the feasibility of automatic design of new channel codes using DNN-based language models.
Over-the-air computation (AirComp) is a promising technology converging communication and computation over wireless networks, which can be particularly effective in model training, inference, and more emerging edge intelligence applications. AirComp relies on uncoded transmission of individual signals, which are added naturally over the multiple access channel thanks to the superposition property of the wireless medium. Despite significantly improved communication efficiency, how to accommodate AirComp in the existing and future digital communication networks, that are based on discrete modulation schemes, remains a challenge. This paper proposes a massive digital AirComp (MD-AirComp) scheme, that leverages an unsourced massive access protocol, to enhance compatibility with both current and next-generation wireless networks. MD-AirComp utilizes vector quantization to reduce the uplink communication overhead, and employs shared quantization and modulation codebooks. At the receiver, we propose a near-optimal approximate message passing-based algorithm to compute the model aggregation results from the superposed sequences, which relies on estimating the number of devices transmitting each code sequence, rather than trying to decode the messages of individual transmitters. We apply MD-AirComp to the federated edge learning (FEEL), and show that it significantly accelerates FEEL convergence compared to state-of-the-art while using the same amount of communication resources.
Deep neural networks (DNNs) in the wireless communication domain have been shown to be hardly generalizable to scenarios where the train and test datasets follow a different distribution. This lack of generalization poses a significant hurdle to the practical utilization of DNNs in wireless communication. In this paper, we propose a generalizable deep learning approach for millimeter wave (mmWave) beam selection using sub-6 GHz channel state information (CSI) measurements, referred to as PARAMOUNT. First, we provide a detailed discussion on physical aspects of the electromagnetic wave scattering in the mmWave and sub-6 GHz bands. Based on this discussion, we develop the augmented discrete angle delay profile (ADADP) which is a novel linear transformation for the sub-6 GHz CSI that extracts the angle-delay attributes and provides a semantic visual representation of the multi-path clusters. Next, we introduce a convolutional neural network (CNN) structure that can learn the signatures of the path clusters in the sub-6 GHz ADADP representation and transform it to mmWave band beam indices. We demonstrate by extensive simulations on several different datasets that PARAMOUNT can generalize beyond the training dataset which is mainly due to transfer learning principles that allow transferring information from previously learned tasks to the learning of new unseen tasks.
In this paper, the problem of drone-assisted collaborative learning is considered. In this scenario, swarm of intelligent wireless devices train a shared neural network (NN) model with the help of a drone. Using its sensors, each device records samples from its environment to gather a local dataset for training. The training data is severely heterogeneous as various devices have different amount of data and sensor noise level. The intelligent devices iteratively train the NN on their local datasets and exchange the model parameters with the drone for aggregation. For this system, the convergence rate of collaborative learning is derived while considering data heterogeneity, sensor noise levels, and communication errors, then, the drone trajectory that maximizes the final accuracy of the trained NN is obtained. The proposed trajectory optimization approach is aware of both the devices data characteristics (i.e., local dataset size and noise level) and their wireless channel conditions, and significantly improves the convergence rate and final accuracy in comparison with baselines that only consider data characteristics or channel conditions. Compared to state-of-the-art baselines, the proposed approach achieves an average 3.85 improvement in the final accuracy of the trained NN on benchmark datasets for image recognition and semantic segmentation tasks, respectively. Moreover, the proposed framework achieves a significant speedup in training, leading to an average 24% and 87% saving in the drone's hovering time, communication overhead, and battery usage, respectively for these tasks.
Wireless communications is often subject to channel fading. Various statistical models have been proposed to capture the inherent randomness in fading, and conventional model-based receiver designs rely on accurate knowledge of this underlying distribution, which, in practice, may be complex and intractable. In this work, we propose a neural network-based symbol detection technique for down-link fading channels, which is based on the maximum a-posteriori probability (MAP) detector. To enable training on a diverse ensemble of fading realizations, we propose a federated training scheme, in which multiple users collaborate to jointly learn a universal data-driven detector, hence the name FedRec. The performance of the resulting receiver is shown to approach the MAP performance in diverse channel conditions without requiring knowledge of the fading statistics, while inducing a substantially reduced communication overhead in its training procedure compared to centralized training.
Efficient millimeter wave (mmWave) beam selection in vehicle-to-infrastructure (V2I) communication is a crucial yet challenging task due to the narrow mmWave beamwidth and high user mobility. To reduce the search overhead of iterative beam discovery procedures, contextual information from light detection and ranging (LIDAR) sensors mounted on vehicles has been leveraged by data-driven methods to produce useful side information. In this paper, we propose a lightweight neural network (NN) architecture along with the corresponding LIDAR preprocessing, which significantly outperforms previous works. Our solution comprises multiple novelties that improve both the convergence speed and the final accuracy of the model. In particular, we define a novel loss function inspired by the knowledge distillation idea, introduce a curriculum training approach exploiting line-of-sight (LOS)/non-line-of-sight (NLOS) information, and we propose a non-local attention module to improve the performance for the more challenging NLOS cases. Simulation results on benchmark datasets show that utilizing solely LIDAR data and the receiver position, our NN-based beam selection scheme can achieve 79.9% throughput of an exhaustive beam sweeping approach without any beam search overhead and 95% by searching among as few as 6 beams. In a typical mmWave V2I scenario, our proposed method considerably reduces the beam search time required to achieve a desired throughput, in comparison with the inverse fingerprinting and hierarchical beam selection schemes.
Massive multiple-input multiple-output (MIMO) systems are a main enabler of the excessive throughput requirements in 5G and future generation wireless networks as they can serve many users simultaneously with high spectral and energy efficiency. To achieve this massive MIMO systems require accurate and timely channel state information (CSI), which is acquired by a training process that involves pilot transmission, CSI estimation, and feedback. This training process incurs a training overhead, which scales with the number of antennas, users, and subcarriers. Reducing the training overhead in massive MIMO systems has been a major topic of research since the emergence of the concept. Recently, deep learning (DL)-based approaches have been proposed and shown to provide significant reduction in the CSI acquisition and feedback overhead in massive MIMO systems compared to traditional techniques. In this paper, we present an overview of the state-of-the-art DL architectures and algorithms used for CSI acquisition and feedback, and provide further research directions.
With the large number of antennas and subcarriers the overhead due to pilot transmission for channel estimation can be prohibitive in wideband massive multiple-input multiple-output (MIMO) systems. This can degrade the overall spectral efficiency significantly, and as a result, curtail the potential benefits of massive MIMO. In this paper, we propose a neural network (NN)-based joint pilot design and downlink channel estimation scheme for frequency division duplex (FDD) MIMO orthogonal frequency division multiplex (OFDM) systems. The proposed NN architecture uses fully connected layers for frequency-aware pilot design, and outperforms linear minimum mean square error (LMMSE) estimation by exploiting inherent correlations in MIMO channel matrices utilizing convolutional NN layers. Our proposed NN architecture uses a non-local attention module to learn longer range correlations in the channel matrix to further improve the channel estimation performance.We also propose an effective pilot reduction technique by gradually pruning less significant neurons from the dense NN layers during training. This constitutes a novel application of NN pruning to reduce the pilot transmission overhead. Our pruning-based pilot reduction technique reduces the overhead by allocating pilots across subcarriers non-uniformly and exploiting the inter-frequency and inter-antenna correlations in the channel matrix efficiently through convolutional layers and attention module.
Efficient link configuration in millimeter wave (mmWave) communication systems is a crucial yet challenging task due to the overhead imposed by beam selection. For vehicle-to-infrastructure (V2I) networks, side information from LIDAR sensors mounted on the vehicles has been leveraged to reduce the beam search overhead. In this letter, we propose a federated LIDAR aided beam selection method for V2I mmWave communication systems. In the proposed scheme, connected vehicles collaborate to train a shared neural network (NN) on their locally available LIDAR data during normal operation of the system. We also propose a reduced-complexity convolutional NN (CNN) classifier architecture and LIDAR preprocessing, which significantly outperforms previous works in terms of both the performance and the complexity.
Additional publications
For a comprehensive list of my publications please refer to my Google Scholar.