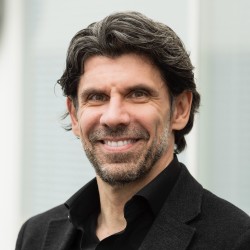
Dr Konstantinos Nikitopoulos
Academic and research departments
Institute for Communication Systems, School of Computer Science and Electronic Engineering.About
Biography
I am currently an Associate Professor (Reader), with the Institute for Communication Systems, University of Surrey, Guildford, UK, and the Director of its newly established “Wireless Systems Lab”. I am an active academic member of the 5G/6G Innovation Centre (5G/6GIC) where I lead the “Theory and Practice of Advanced Concepts in Wireless Communications” Work Area.I also lead the Physical Layer Open RAN development at the University of Surrey, and I am the Consortium and Project Lead of the “HiPer-RAN: Highly Intelligent, Highly Performing RAN” project, supported by the UK’s Department for Science, Innovation and Technology (DSIT) as part of the Open Networks Ecosystem Competition. I am also the Project Lead for the “NL-COMM: Practical, Non-Linear Processing for High Performing Communications” project, supported by Innovate UK and DSIT as part the "Small Business Research Initiative (SBRI): Future Telecommunications challenge", and aiming to bring MIMO non-linear physical layer processing into actual products.
As an academic, I have attracted as a Principal Investigator (PI) research grants of more than £11 million, with a big part of my research being market-driven and industry supported. In terms of teaching, I have been a recipient of the “Tony Jeans Inspirational Teaching Prize” of the University of Surrey, as well as a recipient of the "Teacher of the Year Award" for the School of Computer Science and Electronic Engineering. I am also an IEEE Senior Member and a recipient of the prestigious First Grant of the UK's Engineering and Physical Sciences Research Council.
I received my PhD from the National and Kapodistrian University of Athens where I was a member of the Wireless Systems Group. Since then, I have held research positions at the Institute for Communication Technologies and Embedded Systems at RWTH Aachen University, at the California Institute for Telecommunications and Information Technology at University of California at Irvine and at the Computer Science Department at University College London (UCL). In October 2013 I joined the University of Surrey as a Lecturer.
I have also been a consultant for the Hellenic General Secretariat for Research and Technology, where I also served as a National Delegate of Greece to the Joint Board on Communication Satellite Programmes of European Space Agency.
Areas of specialism
Affiliations and memberships
News
ResearchResearch interests
My research focuses on the intersection of three pivotal areas: advanced signal processing design, innovative computing architectures for wireless communication systems, and system-level design and demonstration. In the realm of signal processing, I have contributed towards highly efficient non-linear processing algorithms and methodologies that can optimize the performance of wireless communication systems while minimizing energy consumption and processing latency (please refer to my "Massively Parallel Non-Linear Processing" work).
In parallel, my research extends to system-level design, demonstration, and evolution, encompassing software-defined approaches and Open-RAN designs. In this context, I endeavour to create flexible, adaptive and intelligent communication systems capable of seamlessly integrating with emerging technologies and evolving user requirements.
In addition, my recent research endeavours include the exploration of unconventional computing architectures, based on the Ising mathematical framework for solving computational intensive and NP mathematical problems that apply to approaches like quantum annealing and neuromorphic computing, as well as in the exploration of analogue processing approaches for performing digital communications (please refer to my published ‘DigiLogue’ processing concept). By harnessing the transformative potential of these emerging technologies, I seek to unlock new avenues for meeting the real-time constraints and energy efficiency requirements of current and future wireless communication systems.
Research projects
NL-COMM: Practical, Non-Linear Processing for High Performing CommunicationsOngoing, Innovate UK, UK's Department for Science Innovation and Technology (DSIT), Small Business Research Initiative, Future Telecommunications Challenge (£904,133, Principal Investigator)
HiPer-RAN (Highly Intelligent, Highly Performing RAN)Ongoing, UK's Department for Science Innovation and Technology (DSIT), Open Networks Ecosystem competition (£7,895,362, Principal Investigator, Consortium Lead)
Ongoing, UK Department for Digital, Culture, Media and Sport (DCMS), Future RAN Competition (£1481k, Co-Investigator and Technical Lead for the University of Surrey)
Tbps Communication SystemOngoing, Industry Supported (£520k, Principal Investigator)
Advanced Detection/Decoding for Multi-stream CommunicationsCompleted, Industry Supported (£140k, Principal Investigator)
Non-linear precoding for 5G Massive MIMOCompleted, Industry Supported (£210k, Principal Investigator)
Programmable Software Defined Radio Access Network for 5GCompleted, Industry Supported (£1590k, Co-Investigator)
AutoAir IICompleted, UK Department for Digital, Culture, Media and Sport (DCMS) (£550k, Principal Investigator)
Completed, UK Department for Digital, Culture, Media and Sport (DCMS) (£1400k, Principal Investigator)
Joint 5GIC/ National Physics Laboratory (NPL) on mm-Wave CommunicationsCompleted, National Physics Laboratory (NPL), (£160k, Principal Investigator)
Completed, EPSRC First Grant, (£100k, Principal Investigator)
Research interests
My research focuses on the intersection of three pivotal areas: advanced signal processing design, innovative computing architectures for wireless communication systems, and system-level design and demonstration. In the realm of signal processing, I have contributed towards highly efficient non-linear processing algorithms and methodologies that can optimize the performance of wireless communication systems while minimizing energy consumption and processing latency (please refer to my "Massively Parallel Non-Linear Processing" work).
In parallel, my research extends to system-level design, demonstration, and evolution, encompassing software-defined approaches and Open-RAN designs. In this context, I endeavour to create flexible, adaptive and intelligent communication systems capable of seamlessly integrating with emerging technologies and evolving user requirements.
In addition, my recent research endeavours include the exploration of unconventional computing architectures, based on the Ising mathematical framework for solving computational intensive and NP mathematical problems that apply to approaches like quantum annealing and neuromorphic computing, as well as in the exploration of analogue processing approaches for performing digital communications (please refer to my published ‘DigiLogue’ processing concept). By harnessing the transformative potential of these emerging technologies, I seek to unlock new avenues for meeting the real-time constraints and energy efficiency requirements of current and future wireless communication systems.
Research projects
Ongoing, Innovate UK, UK's Department for Science Innovation and Technology (DSIT), Small Business Research Initiative, Future Telecommunications Challenge (£904,133, Principal Investigator)
Ongoing, UK's Department for Science Innovation and Technology (DSIT), Open Networks Ecosystem competition (£7,895,362, Principal Investigator, Consortium Lead)
Ongoing, UK Department for Digital, Culture, Media and Sport (DCMS), Future RAN Competition (£1481k, Co-Investigator and Technical Lead for the University of Surrey)
Ongoing, Industry Supported (£520k, Principal Investigator)
Completed, Industry Supported (£140k, Principal Investigator)
Completed, Industry Supported (£210k, Principal Investigator)
Completed, Industry Supported (£1590k, Co-Investigator)
Completed, UK Department for Digital, Culture, Media and Sport (DCMS) (£550k, Principal Investigator)
Completed, UK Department for Digital, Culture, Media and Sport (DCMS) (£1400k, Principal Investigator)
Completed, National Physics Laboratory (NPL), (£160k, Principal Investigator)
Completed, EPSRC First Grant, (£100k, Principal Investigator)
Supervision
Completed postgraduate research projects I have supervised
As Principal Supervisor:
- C. Jayawardena, “Generalized, Massively Parallel Receiver Processing for Non-Orthogonal Signal Transmissions”
- C. Husmann, “Advanced Transceiver Processing for Large MIMO Systems and its Application to the 5th Generation of Mobile Communications”
- F. Mehran, “Physical Layer Design for Grant Free Multiple Access”
Teaching
Postgraduate
- Advanced 5G Wireless Technologies (Module Leader)
- Applied Mathematics for Communication Systems
Undergraduate
- Digital Signal Processing B
Publications
Multi-user (MU)-multiple-input, multiple-output (MIMO) technology has been central to the evolution of wireless networks, since it can provide substantial network gains by enabling the concurrent transmission of a large number of information streams, over the same frequency. However, reliably detecting these mutually interfering streams comes at a very high computational cost that increases exponentially with the number of concurrently transmitted streams. This makes the corresponding MU-MIMO systems highly inefficient in terms of power consumption and processing latency. In this context, and in order to unlock the full MU-MIMO potential, alternative computing architectures are required, able to efficiently detect a large number of information streams, in a power-efficient manner. In this context, NeuroMIMO, is the first attempt to apply the principles of neuromorphic computing to achieve highly efficient MIMO detection. NeuroMIMO suggests and evaluates two different ways to translate the MIMO detection problem into a neuromorphic one. The first (i.e., Massive-NeuroMIMO) is appropriate for massive MIMO systems, where the number of receive, base-station/access-point antennas is much higher than the number of information streams. The second (i.e., Highly-Efficient-NeuroMIMO) is appropriate for the case where the number of transmitted streams approaches the number of base station antennas, and can reach the performance of the optimal Maximum-Likelihood detector. We discuss the trade-offs between the two NeuroMIMO approaches, and we show that both can provide substantial power gains compared to their traditional counterparts, while accounting for the preprocessing overhead required to translate the MIMO detection problem into a neuromorphic one. In addition, despite the current limitations in the "speed" of existing neuromorphic chips, we discuss that real-time processing detection can be achieved, even for a 5G NR system with 100 MHz operating bandwidth.
The increasing demand for massive connectivity with low latency requirements has triggered a paradigm shift towards Non-Orthogonal transmissions. Still, to translate the theoretical gains of Non-Orthogonal transmissions into practical, efficient “soft” detection schemes are required. The detection latency and/or complexity of state-of-the-art detection methods becomes impractical for large Non-Orthogonal systems, both due to the large number of interfering streams and due to the rank-deficient or ill-determined nature of the corresponding interference matrix. Extending the recently proposed MultiSphere framework, this work introduces NorthCore; a massively parallel sphere-decoding-based scheme for the detection of large and illdetermined Non-Orthogonal systems. Similarly to MultiSphere, NorthCore reduces the corresponding search space by focusing the available processing power to the most promising vector solutions that are processed in parallel. As a result, the proposed detection scheme can attain a detection processing latency similar to that of highly-suboptimal linear detectors and even outperform state-of-the-art sophisticated detection approaches with up to an order of magnitude reduced complexity. To identify the most promising vector solutions, NorthCore introduces a sortfree candidate selection technique that reduces the necessary preprocessing complexity by up to an order of magnitude, making the proposed approach practical.
The increasing demand for connectivity and throughput, despite the spectrum limitations, has triggered a paradigm shift towards non-orthogonal signal transmissions. However, the complexity requirements of near-optimal detection methods for such systems becomes impractical, due to the large number of mutually interfering streams and to the rank-deficient or ill-determined nature of the corresponding interference matrix. This work introduces g-MultiSphere; a generic massively parallel and near-optimal sphere-decoding-based approach that, in contrast to prior work, applies to both well- and ill-determined non-orthogonal systems. We show that g-MultiSphere is the first approach that can support large uplink multi-user MIMO systems with numbers of concurrently transmitting users that exceed the number of receive antennas by a factor of two or more, while attaining throughput gains of up to 60% and with reduced complexity requirements in comparison to known approaches. By eliminating the need for sparse signal transmissions for nonorthogonal multiple access (NOMA) schemes, g-MultiSphere can support more users than existing systems with better detection performance and practical complexity requirements. In comparison to state- of-the-art detectors for NOMA schemes and nonorthogonal signal waveforms (e.g., SEFDM) g-MultiSphere can be up to an order of magnitude less complex, and can provide throughput gains of up to 60%.
The recent paradigm shift towards the transmission of large numbers of mutually interfering information streams, as in the case of aggressive spatial multiplexing, combined with requirements towards very low processing latency despite the frequency plateauing of traditional processors, initiates a need to revisit the fundamental maximum-likelihood (ML) and, consequently, the sphere-decoding (SD) detection problem. This work presents the design and VLSI architecture of MultiSphere; the first method to massively parallelize the tree search of large sphere decoders in a nearly-concurrent manner, without compromising their maximum-likelihood performance, and by keeping the overall processing complexity comparable to that of highly-optimized sequential sphere decoders. For a 10 ⇥ 10 MIMO spatially multiplexed system with 16-QAM modulation and 32 processing elements, our MultiSphere architecture can reduce latency by 29⇥ against well-known sequential SDs, approaching the processing latency of linear detection methods, without compromising ML optimality. In MIMO multicarrier systems targeting exact ML decoding, MultiSphere achieves processing latency and hardware efficiency that are orders of magnitude improved compared to approaches employing one SD per subcarrier. In addition, for 16⇥16 both “hard”- and “soft”-output MIMO systems, approximate MultiSphere versions are shown to achieve similar error rate performance with state-of-the art approximate SDs having akin parallelization properties, by using only one tenth of the processing elements, and to achieve up to approximately 9⇥ increased energy efficiency.
Next-generation 6G networks are expected to feature an extremely high density of network and user devices. MU-MIMO non-linear processing can provide substantially improved performance over linear processing in dense conditions, but suffers from a high complexity and processing latency. The use of the massively parallel non-linear (MPNL) processing framework can overcome such limitations. This work discusses three potential 6G transmission scenarios and evaluates their detection and precoding performance using link-level simulations and a system-level, over-the-air, 3GPP standards-based testbed. The results validate that MPNL processing has the potential to transform the way 6G MU-MIMO systems are designed.
It is well documented that the achievable throughput of MIMO systems that employ linear beamforming can significantly degrade when the number of concurrently transmitted information streams approaches the number of base-station antennas. To increase the number of the supported streams, and therefore, to increase the achievable net throughput, non-linear beamforming techniques have been proposed. These beamforming approaches are typically evaluated via simulations or via simplified over-the-air experiments that are sufficient for validating their basic principles, but they neither provide insights about potential practical challenges when trying to adopt such approaches in a standards-compliant framework, nor they provide any indication about the achievable performance when they are part of a standards-compliant protocol stack. In this work, for first time, we evaluate non-linear beamforming in a 3GPP standards- compliant framework, using our recently-proposed SWORD research platform. SWORD is a flexible, open for research, software-driven platform that enables the rapid evaluation of advanced algorithms without extensive hardware optimizations that can prevent promising algorithms from being evaluated in a standards-compliant stack. We show that in an indoor environment, vector perturbation-based non-linear beamforming can provide up to 46% throughput gains compared to linear approaches for 4×4 MIMO systems, while it can still provide gains of nearly 10% even if the number of base-station antennas is doubled.
Multiple-user, multiple-input, multiple-output (MU-MIMO) systems supporting a large number of concurrent streams have the potential to substantially improve the connectivity and throughput of future wireless communication systems. Towards this goal, deep learning (DL)-based techniques have recently been proposed for MIMO signal detection. Good performance results have been reported when compared to conventional detection methods, but it is unclear how they measure against state-of-the-art detection techniques. In this work, for the first time, we perform a critical evaluation of DetNet, MMNet, GEPNet, and RE-MIMO, four prominent model-based DL techniques based on different working principles, and assess their reliability, complexity, and robustness against the practical Massively Parallel Non-Linear processing (MPNL) detection approach. The results show that the model-based DL approaches offer promising results but have difficulty adapting to channel models that differ from those on which they were trained. They also exhibit lower reliability and higher complexity than MPNL, even without considering the training stage. We find that, at present, the human-designed MPNL outperforms the DL-based detection methods in virtually all the metrics. Nevertheless, DL-based solutions are rapidly advancing, and further research intended to address their current shortcomings may one day offer advantages over human-designed detection methods.
Non-orthogonal multiple access schemes (NOMA), such as sparse code multiple access (SCMA), are among the most promising technologies to support massive numbers of connected devices. Still, to minimize the transmission delay and to maximize the utilization of the transmission channel, "grant-free" NOMA techniques are required that eliminate any prior information exchange between the users and the base-stations. However, if a large number of users transmit simultaneously in an "unsupervised" manner, (i.e., without any prior signaling for controlling the number of users and the corresponding transmission patterns), it is likely that a large number of users may share the same frequency-resource element, rendering the corresponding user detection impractical. In this context, we present a new multi-user detection approach, which aims to maximize the detection performance, with respect to given processing and latency limitations. We show that our approach enables practical detection for grant-free SCMA schemes that support hundreds of interfering users, with a complexity that is up to two orders of magnitude less than that of conventional detection approaches.
Open Radio Access Networks (Open-RAN) require cost- and energy-efficient solutions to facilitate their deployment at scale. A significant concern in multiple-input multiple-output (MIMO) systems employing traditional linear processing is the substantial number of radio frequency (RF) chains at the base station (BS), which is required to ensure the accurate decoding of spatially multiplexed streams. Recently, however, practical non-linear approaches, which facilitate near-optimal parallelizable tree searches, have been successfully implemented on actual systems and demonstrated the capability to considerably reduce the required RF chains without affecting user performance. Like QR decomposition (QRD) being used to perform channel inversion in linear systems, these non-linear approaches employ a sorted QRD (SQRD) to curtail the search complexity. However, this can be a significant bottleneck for general software-based non-linear solutions, preventing them from fully exploiting the gains. To address the latency limitations with SQRD, this work presents a high throughput hardware accelerator based on reformulating the underlying Modified Gram Schmidt process (MGS) to extract further parallelism than previous designs. Implementations of the proposed architecture demonstrate at least 2-fold improvements in the achievable throughput and processing latency over existing 4×4 and 8×8 field programmable gate array (FPGA) implementations and can be scaled up to 16×16 MIMO systems. Further, the proposed accelerator is integrated with the software framework that can considerably offload the processing burden for higher number of streams under strict latency conditions.
Open Radio Access Networks (Open RANs), realized fully in software, require excessive computing resources to support time-sensitive signal-processing algorithms in the physical layer. Among them, multiple-input-multiple-output (MIMO) processing is a key functionality used to drive higher connectivity in the uplink, but it is computationally intensive, triggering the need for hardware acceleration to overcome the processing inefficiency of software-based solutions. Additionally, energy efficiency is becoming a key focus in Open RAN to enable sustainable deployments that utilize available resources efficiently. Because channel-inversion complexity increases polynomially with the number of users in linear detectors, such as zero-forcing (ZF) and minimum-mean-square-error (MMSE), acceleration based on channel-inverse approximations has gained significant attention. However, they unnecessarily multiply the number of base station (BS) antennas to ensure accurate detection, leading to a drastic increase in power consumption owing to the additional radio frequency (RF) chains employed. In contrast, linear detectors achieve a sufficiently good performance with only twice the number of BS antennas as users. This work introduces an exact-MMSE and soft-output hardware accelerator that includes an inversion-free, highly-parallel QR decomposition (QRD) architecture and a low-complexity detector stage with per-cycle soft-output generation, significantly improving the processing latency and throughput. The proposed architecture is fully scalable to support diverse MIMO configurations. Implementation evaluations on a Xilinx Virtex Ultrascale+ field-programmable gate array (FPGA) demonstrate that the proposed exact solution can achieve more than 2x improvement in hardware throughput over existing approximate designs. Moreover, the peak throughput can be increased around 10-fold in slowly fading channels.
In conventional hybrid beamforming approaches, the number of radio-frequency (RF) chains is the bottleneck on the achievable spatial multiplexing gain. Recent studies have overcome this limitation by increasing the update-rate of the RF beamformer. This paper presents a framework to design and evaluate such approaches, which we refer to as agile RF beamforming, from theoretical and practical points of view. In this context, we consider the impact of the number of RF-chains, phase shifters speed, and resolution to design agile RF beamformers. Our analysis and simulations indicate that even an RF-chain-free transmitter, which its beamformer has no RF-chains, can provide a promising performance compared with fully-digital systems and significantly outperform the conventional hybrid beamformers. Then, we show that the phase shifter's limited switching speed can result in signal aliasing, in-band distortion, and out-of-band emissions. We introduce performance metrics and approaches to measure such effects and compare the performance of the proposed agile beamformers using the Gram-Schmidt orthogonalization process. Although this paper aims to present a generic framework for deploying agile RF beamformers, it also presents extensive performance evaluations in communication systems in terms of adjacent channel leakage ratio, sum-rate, power efficiency, error vector magnitude, and bit-error rates.
In this paper two complexity efficient soft sphere-decoder modifications are proposed for computing the max-log LLR values in iterative MIMO systems, which avoid the costly, typically needed, full enumeration and sorting (FES) procedure during the tree traversal without compromising the max-log performance. It is shown that despite the resulting increase in the number of expanded nodes, they can be more computationally efficient than the typical soft sphere decoders by avoiding the unnecessary complexity of FES.
While the deployment of 5G mobile networks is still in its early stage, we are currently experiencing a paradigm shift towards Open Radio Access Network (RAN) architectures. In this context, RAN solutions that are heavily designed on software can reduce the implementation cost and time-to-market, as well as increase flexibility by making such solutions 'future-ready' through enabling new features, such as new advanced signal processing algorithms, to be included even via a simple software update. Realization of software-based RANs enabled by this paradigm shift, although attractive is however non-trivial, especially the realization of physical layer which mandates fast and efficient processing to meet strict real-time requirements. In this direction, we propose in this work a specialized acceleration software solution (SACCESS) to accelerate computationally expensive physical layer processing operations. We show that SACCESS can provide a slot processing speed-up of over 2.2, compared to OpenAirInterface (OAI) which emerged as one of the most advanced software-based 5G-NR RAN solutions available to a wider community. Our results demonstrate, for first time, that a peak throughput for a 40MHz TDD and FDD single antenna 5G-NR system based on OAI's development can be achieved without any hardware acceleration.
Multi-user multiple-input, multiple-output (MU-MIMO) designs can substantially increase the achievable throughput and connectivity capabilities of wireless systems. However, existing MU-MIMO deployments typically employ linear processing that, despite its practical benefits, can leave capacity and connectivity gains unexploited. On the other hand, traditional non-linear processing solutions (e.g., sphere decoders) promise improved throughput and connectivity capabilities, but can be impractical in terms of processing complexity and latency, and with questionable practical benefits that have not been validated in actual system realizations. At the same time, emerging new Open Radio Access Network (Open-RAN) designs call for physical layer (PHY) processing solutions that are also practical in terms of realization, even when implemented purely on software. This work demonstrates the gains that our highly efficient, massively parallelizable, non-linear processing (MPNL) framework can provide, both in the uplink and downlink, when running in real-time and over-the-air, using our new 5G-New Radio (5G-NR) and Open-RAN compliant, software-based PHY. We showcase that our MPNL framework can provide substantial throughput and connectivity gains, compared to traditional, linear approaches, including increased throughput, the ability to halve the number of base-station antennas without any performance loss compared to linear approaches, as well as the ability to support a much larger number of users than base-station antennas, without the need for any traditional Non-Orthogonal Multiple Access (NOMA) techniques, and with overloading factors that can be up to 300%.
Conference Title: 2022 IEEE Globecom Workshops (GC Wkshps) Conference Start Date: 2022, Dec. 4 Conference End Date: 2022, Dec. 8 Conference Location: Rio de Janeiro, BrazilThe general tendency to deliver Open Radio Access Network (Open-RAN) solutions by means of software-based, or even cloud-native, realizations drives the development community to fully capitalize on software architectures, even for the computationally demanding 5G physical layer (PHY) processing. However, software solutions are typically orders of magnitude less efficient than dedicated hardware in terms of power consumption and processing speed. Consequently, realizing highly-efficient, massive multiple-input multiple-output (mMIMO) solutions in software, while exploiting the wide 5G transmission bandwidths, becomes extremely challenging and requires the massive parallelization of the PHY processing tasks. In this work, for the first time, we show that massively parallel software solutions are capable of meeting the processing requirements of 5G New Radio (NR), still, with a significant increase in the corresponding power consumption. In this context, we quantify this power consumption overhead, both in terms of Watts and carbon emissions, as a function of the concurrently transmitted information streams, of the base-station antennas, and of the utilized bandwidth. We show that the computational power consumption of such PHY processing is no longer negligible and that, for mMIMO solutions supporting a large number of information streams, it can become comparable to the power consumption of the Radio Frequency (RF) chains. Finally, we discuss how a shift towards non-linear PHY processing can significantly boost energy efficiency, and we further highlight the importance of energy-aware digital signal processing design in future PHY processing architectures.
—In this work, Generalized Space-Time Super-Modulation (GSTSM) is introduced which enables the transmission of an additional flexible-rate and highly-reliable information stream concurrently with the conventionally transmitted symbols , without the need for increasing the corresponding packet length. This is attained by jointly exploiting the spatial and temporal dimensions of multiple-antenna systems, which enables efficient detection for conventional and additional information subchannels even in highly correlated channel conditions or AWGN channels. In the context of machine-type communications, GSTSM enables grant-free medium access without transmitting additional headers to convey each machine's signature information. Hence, it is shown that even at an extreme case where the data packets of two users are always colliding, GSTSM offers throughput gains of up to 33% compared to the best examined header-based scheme. For the same scenario, it is shown that GSTSM based on joint multiuser detection provides throughput gains of up to 2.5× compared with the case where users' signals are detected independently. In addition, it yields over 90% improvement in achievable rates compared with the schemes that require centralized medium-access coordination. For both joint and independent signal detection schemes, it is also shown that adopting an iterative detection/decoding approach allows to further improve the throughput gains.
—This work introduces Gyre Precoding (GP), a novel linear multiuser multiple-input multiple-output (MU-MIMO) precoding approach. GP performs rotations of the symbols of each spatial layer to optimize the precoding performance. To find the rotation angles, we propose a near-optimal, gradient descent–based low-complexity algorithm. GP is constellation-agnostic and does not require significant changes to conventional receiver procedures or wireless standards. Computer evaluation results show that GP can achieve 8 dB SNR gains over linear precoding techniques and 2 dB over suboptimal symbol-level precoding (SLP) methods for a 16 × 16 MU-MIMO system. Furthermore, in a 64×12 massive-MIMO scenario in a 5G New Radio (5GNR) setup, GP achieves a 13% higher throughput gain over zero-forcing precoding. Index Terms—Multi-user multiple-input multiple-output (MU-MIMO), precoding.
Millimeter wave (mmWave) systems with effective beamforming capability play a key role in fulfilling the high data-rate demands of current and future wireless technologies. Hybrid analog-todigital beamformers have been identified as a cost-effective and energy-efficient solution towards deploying such systems. Most of the existing hybrid beamforming architectures rely on a subconnected phase shifter network with a large number of antennas. Such approaches, however, cannot fully exploit the advantages of large arrays. On the other hand, the current fully-connected beamformers accommodate only a small number of antennas, which substantially limits their beamforming capabilities. In this paper, we present a mmWave hybrid beamformer testbed with a fully-connected network of phase shifters and adjustable attenuators and a large number of antenna elements. To our knowledge, this is the first platform that connects two RF inputs from the baseband to a 16 8 antenna array, and it operates at 26 GHz with a 2 GHz bandwidth. It provides a wide scanning range of 60, and the flexibility to control both the phase and the amplitude of the signals between each of the RF chains and antennas. This beamforming platform can be used in both short and long-range communications with linear equivalent isotropically radiated power (EIRP) variation between 10 dBm and 60 dBm. In this paper, we present the design, calibration procedures and evaluations of such a complex system as well as discussions on the critical factors to consider for their practical implementation.
Multi-user (MU) MIMO-OFDM systems with aggressive spatial multiplexing are promising to enhance through-put and enable massive connectivity. In such systems, residual carrier frequency offsets (CFOs), due to the instability of oscilla-tors and doppler shifts, can substantially degrade the achievable uplink throughput, especially when the number of connected devices becomes large. Existing approaches to mitigate CFOs in MU scenarios, typically involve closed-loop feedback that can result in high signaling overhead and/or significant residual CFO. Being able to compensate for the CFO of the multiple users at the receiver side, can enable the joint transmission of frequency asynchronous users, can obviate the need for high overhead synchronization procedures, can enable the use of cheaper oscillators, and can potentially unlock new user access schemes. However, as we discuss here in detail, compensating for the multiple user CFOs at the receiver is currently impractical due to the corresponding exponential complexity requirements. At the same time, methods that are typically used in single-user MIMO-OFDM systems are inappropriate for MU-MIMO scenarios and, as we show, can result in substantial (e.g., 80%) throughput degradation. To fill this gap, for the first time, we propose a joint CFO compensation and MU detection scheme that can support a large number of spatially transmitted information streams with practical processing complexity and latency requirements. We show that the proposed scheme enables frequency asynchronous user transmission and approaches the performance of perfectly synchronized systems with complexity requirements that are comparable to current MU-MIMO detection schemes that assume perfect synchronization.
A-posteriori probability (APP) receivers operating over multiple-input, multiple-output channels provide enhanced bit error rate (BER) performance at the cost of increased complexity. However, employing full APP processing over favorable transmission environments, where less efficient approaches may already provide the required performance at a reduced complexity, results in unnecessary processing. For slowly varying channel statistics substantial complexity savings can be achieved by simple adaptive schemes. Such schemes track the BER performance and adjust the complexity of the soft output sphere decoder by adaptively setting the related log-likelihood ratio (LLR) clipping value.
Unlocking new wireless applications such as mobile extended reality and holographic telepresence necessitates ultra-power efficient systems that are able to support data rates of hundreds of gigabits per second. Utilizing the multi-gigahertz bandwidth that is currently available in higher frequencies (e.g., millimeter-wave or terahertz) is a promising pathway in this direction. However, exploiting such ultra-wide bandwidths by using conventional transceiver processing brings us in front of significant challenges in terms of power consumption and signal processing speed. For example, the power consumption of high-precision and ultra-high-speed digital-to-analogue and analogue-to-digital converters (DAC/ADC) for ultra-wide band-widths becomes impractical. At the same time, conventional, state-of-the-art signal processing functionalities, like detection and decoding are becoming not only too power-hungry but also too complex to meet the corresponding latency requirements of ultra-fast systems. In order to overcome these challenges, we herein propose a shift towards "DigiLogue" transceiver processing, according to which, computationally intensive and power-hungry digital signal processing tasks take place directly in the analogue domain, avoiding traditional signal up/down-conversion and ADC/DACs, but still preserving the performance of traditional, near-optimal, digital transceiver algorithms. In this context, we give the first example of a simple to realize joint detection/decoding scheme that outperforms existing analogue-domain approaches and reaches the performance of digitally optimal solutions with power consumption that can be up to two orders of magnitude less.
Next-generation wireless networks are expected to be ultra-dense in terms of users and be able to support delay-sensitive traffic. Multiple-user, multiple-input, multiple-output (MU-MIMO) offers a potential solution by multiplexing a large number of concurrent data streams in the spatial domain. The MU-MIMO user scheduling process involves allocating the users across the space, and time or frequency resources, such that a performance metric is maximized, and subject to specific (e.g., rate) constraints being met. However, user scheduling is a combinato-rial problem, making its optimal solution highly intricate. This paper introduces the orthonormal subspace alignment scheduling (OSAS) approach, designed to be scalable for use in highly-dense networks and optimized for low-latency communications. Its design prioritizes users that align to the standard orthonormal basis and features a novel pruning process that enhances the users' transmission rates. Comparative evaluations reveal that OSAS makes more efficient use of the available resources and offers higher performance than other state-of-the-art techniques, while exhibiting lower complexity.
This chapter has presented the insight methodologies on how to design, implement, and evaluate a width-bandwidth mm-wave fully connected hybrid beamforming metrological testbed with a large antenna array. The focus has been given on discussions include testbed design, calibration procedures, experimental evaluations, as well as the critical factors to consider for their practical implementation. If RF harmonics and spurious signal issues are avoided, one envisages that the testbed could be setup to work between 25 and 30 GHz with 2-GHz instantaneous bandwidth. Each of the phase shifters and attenuators in the mm-wave fully connected hybrid beamformer has six separate DIO control bits. Apart from describing the calibration procedures for the phase and amplitudes of the established fully connected hybrid beamformer system, the linearity, phase, and attenuation performance of the beamformer system between 25.5 and 26.5 GHz have been evaluated as well as the beamforming and link performance of a 128-element planar phased array at 26 GHz where the measured radiation patterns with and without amplitude tapering are compared.
—This work introduces MultiSphere, a method to massively parallelize the tree search of large sphere decoders in a nearly-independent manner, without compromising their maximum-likelihood performance, and by keeping the overall processing complexity at the levels of highly-optimized sequential sphere decoders. MultiSphere employs a novel sphere decoder tree partitioning which can adjust to the transmission channel with a small latency overhead. It also utilizes a new method to distribute nodes to parallel sphere decoders and a new tree traversal and enumeration strategy which minimize redundant computations despite the nearly-independent parallel processing of the subtrees. For an 8 × 8 MIMO spatially multiplexed system with 16-QAM modulation and 32 processing elements MultiSphere can achieve a latency reduction of more than an order of magnitude, approaching the processing latency of linear detection methods, while its overall complexity can be even smaller than the complexity of well-known sequential sphere decoders. For 8×8 MIMO systems, MultiSphere’s sphere decoder tree partitioning method can achieve the processing latency of other partitioning schemes by using half of the processing elements. In addition, it is shown that for a multi-carrier system with 64 subcarriers, when performing sequential detection across subcarriers and using MultiSphere with 8 processing elements to parallelize detection, a smaller processing latency is achieved than when parallelizing the detection process by using a single processing element per subcarrier (64 in total).
—Multi-user multiple-input, multiple-output (MU-MIMO) designs can substantially increase wireless systems' achievable throughput and connectivity capabilities. However, existing MU-MIMO deployments typically utilize linear processing techniques that, despite their practical benefits, such as low computational complexity and easy integrability, can leave much of the available throughput and connectivity gains unexploited. They typically require many power-intensive antennas and RF chains to support a smaller number of MIMO streams, even when the transmitted information streams are of low rate. Alternatively, non-linear (NL) processing methods can maximize the capabilities of the MIMO channel. Despite their potential, traditional NL methods are challenged by high computational complexity and processing latency, making them impractical for real-time applications, especially in software-based systems envisioned for emerging Open Radio Access Networks (Open-RAN). Additionally, essential functionalities such as rate adaptation (RA) are currently unavailable for NL systems, limiting their practicality in real-world deployments. In this demo, we present the latest capabilities of our advanced NL processing framework (NL-COMM) in real-time and over-the-air, comparing them side-by-side with conventional linear processing. For the first time, NL-COMM not only meets the practical 5G-NR real-time latency requirements in pure software but also does so within a standard-compliant ecosystem. To achieve this, we significantly extended the NL-COMM algorithmic framework to support the first practical RA for NL processing. The demonstrated gains include enhanced connectivity by supporting four MIMO streams with a single base-station antenna, substantially increased throughput, and the ability to halve the number of base-station antennas without any performance loss to linear approaches.
Large MIMO base stations remain among wireless network designers’ best tools for increasing wireless throughput while serving many clients, but current system designs, sacrifice throughput with simple linear MIMO detection algorithms. Higher-performance detection techniques are known, but remain off the table because these systems parallelize their computation at the level of a whole OFDM subcarrier, sufficing only for the lessdemanding linear detection approaches they opt for. This paper presents FlexCore, the first computational architecture capable of parallelizing the detection of large numbers of mutually-interfering information streams at a granularity below individual OFDM subcarriers, in a nearly-embarrassingly parallel manner while utilizing any number of available processing elements. For 12 clients sending 64-QAM symbols to a 12-antenna base station, our WARP testbed evaluation shows similar network throughput to the state-of-the-art while using an order of magnitude fewer processing elements. For the same scenario, our combined WARP-GPU testbed evaluation demonstrates a 19× computational speedup, with 97% increased energy efficiency when compared with the state of the art. Finally, for the same scenario, an FPGAbased comparison between FlexCore and the state of the art shows that FlexCore can achieve up to 96% better energy efficiency, and can offer up to 32× the processing throughput.
Hybrid beamforming for frequency-selective channels is a challenging problem, as the phase shifters provide the same phase shift to all the subcarriers. The existing approaches solely rely on the channel’s frequency response, and the hybrid beamformers maximize the average spectral efficiency over the whole frequency band. Compared to state-of-the-art, we show that substantial sum-rate gains can be achieved, both for rich and sparse scattering channels, by jointly exploiting the frequency- and time-domain characteristics of the massive multiple-input multiple-output (MIMO) channels. In our proposed approach, the radio frequency (RF) beamformer coherently combines the received symbols in the time domain and, thus, it concentrates the signal’s power on a specific time sample. As a result, the RF beamformer flattens the frequency response of the “effective” transmission channel and reduces its root-mean-square delay spread. Then, a baseband combiner mitigates the residual interference in the frequency domain. We present the closed-form expressions of the proposed beamformer and its performance by leveraging the favorable propagation condition of massive MIMO channels, and we prove that our proposed scheme can achieve the performance of fully digital zero-forcing when the number of employed phases shifter networks is twice the resolvable multipath components in the time domain.characteristics of the massive multiple-input multiple-output (MIMO) channels. In our proposed approach, the radio frequency (RF) beamformer coherently combines the received symbols in the time domain and, thus, it concentrates the signal's power on a specific time sample. As a result, the RF beamformer flattens the frequency response of the ``effective'' transmission channel and reduces its root-mean-square delay spread. Then, a baseband combiner mitigates the residual interference in the frequency domain. We present the closed-form expressions of the proposed beamformer and its performance by leveraging the favorable propagation condition of massive MIMO channels, and we prove that our proposed scheme can achieve the performance of fully digital zero-forcing when the number of employed phases shifter networks is twice the resolvable multipath components in the time domain.
The discrete cosine transform (DCT) based multicarrier modulation (MCM) system is regarded as one of the promising transmission techniques for future wireless communications. By employing cosine basis as orthogonal functions for multiplexing each real-valued symbol with symbol period of T , it is able to maintain the subcarrier orthogonality while reducing frequency spacing to 1/(2T ) Hz, which is only half of that compared to discrete Fourier transform (DFT) based multicarrier systems. In this paper, following one of the effective transmission models by which zeros are inserted as guard sequence and the DCT operation at the receiver is replaced by DFT of double length, we reformulate and evaluate three classic detection methods by appropriately processing the post- DFT signals both for single antenna and multiple-input multipleoutput (MIMO) DCT-MCM systems. In all cases, we show that with our reformulated detection approaches, DCT-MCM schemes can outperform, in terms of error-rate, conventional OFDMbased systems.
Transitioning to more intelligent, autonomous transportation systems necessitates network infrastructure capable of accommodating both substantial uplink traffic and massive vehicle connectivity. Current approaches addressing these throughput and connectivity requirements rely on the utilization of the multiple input, multiple output (MIMO) technology. However, When traditional linear detection/precoding processing methods are adopted, they require the deployment of an extensive number of co-located, access-point antennas to support a comparatively much smaller number of data streams. Such a setup significantly increases the power consumption on the radio side, raising substantial concerns about the operational costs and sustainability of such deployments, particularly in densely deployed scenarios, across extensive road networks. Addressing these concerns, this work proposes an Open Radio Access Network (Open-RAN) deployment that incorporates a Massively Parallelizable, Nonlinear (MPNL) MIMO processing framework and assesses, for the first time, its impact on the power consumption and vehicular connectivity in various Vehicle-to-Infrastructure (V2I) and Network (V2N) scenarios. We show that flexible, Open-RAN physical layer deployments, incorporating MPNL, emerge as a critical power efficiency enabler, especially when flexibly activating/deactivating employed RF elements. Our field-programmable gate array (FPGA) based evaluation of MPNL, reveals that it can lead to significant power savings on the radio side, by eliminating the need for a " massive " number of base station antennas and radio frequency (RF) chains. Additionally, our findings show substantial connectivity gains, exceeding 400%, in terms of concurrently transmitting vehicles compared to traditional processing approaches, without significantly affecting the access point power consumption budgets, thereby catalyzing the evolution towards more intelligent, fully autonomous, and sustainable transportation systems.
MIMO mobile systems, with a large number of antennas at the base-station side, enable the concurrent transmission of multiple, spatially separated information streams, and therefore, enable improved network throughput and connectivity both in uplink and downlink transmissions. Traditionally, such MIMO transmissions adopt linear base-station processing, that translates the MIMO channel into several single-antenna channels. While such approaches are relatively easy to implement, they can leave on the table a significant amount of unexploited MIMO capacity and connectivity capabilities. Recently-proposed non-linear base-station processing methods claim this unexplored capacity and promise substantially increased network throughput and connectivity capabilities. Still, to the best of the authors' knowledge, non-linear base-station processing methods not only have not yet been adopted by actual systems, but have not even been evaluated in a standard-compliant framework, involving of all the necessary algorithmic modules required by a practical system. In this work, for the first time, we incorporate and evaluate non-linear base-station processing in a 3GPP standard environment. We outline the required research platform modifications and we verify that significant throughput gains can be achieved, both in indoor and outdoor settings, even when the number of base-station antennas is much larger than the number of transmitted information streams. Then, we identify missing algorithmic components that need to be developed to make non-linear base-station practical, and discuss future research directions towards potentially transformative next-generation mobile systems and base-stations (i.e., 6G) that explore currently unexploited non-linear processing gains.
An index modulation (IM) assisted Discrete Cosine Transform based Orthogonal Frequency Division Multiplexing (DCT-OFDM) with Enhanced Transmitter Design (termed as EDCT-OFDM-IM) is proposed. It amalgamates the concept of Discrete Cosine Transform assisted Orthogonal Frequency Division Multiplexing (DCT-OFDM) and Index Modulation (IM) to exploit the design freedom provided by the double number of available subcarrier under the same bandwidth. In the proposed EDCT-OFDM-IM scheme, the maximum likelihood (ML) detector used for symbol bits and index bits recovering is derived and the sophisticated designing guidelines for EDCTOFDM-IM are provided. Based on the derived pairwise error event probability, a theoretical upper bound on the average biterror probability (ABEP) of EDCT-OFDM-IM is provided over multipath fading channels. Furthermore, the maximum peak-toaverage power ratio (PAPR) of our proposed EDCT-OFDM-IM scheme is derived and compared to than the general Discrete Fourier Transform (DFT) based OFDM-IM counterpart.
Discrete cosine transform (DCT) based orthogonal frequency division multiplexing (OFDM), which has double number of subcarrier compared to the classic discrete fourier transform (DFT) based OFDM (DFT-OFDM) at the same bandwidth, is a promising high spectral efficiency multicarrier techniques for future wireless communication. In this paper, an enhanced DCT-OFDM with index modulation (IM) (EDCT-OFDM-IM) is proposed to further exploit the benefits of the DCT-OFDM and IM techniques. To be more specific, a pre-filtering method based DCT-OFDM-IM transmitter is first designed and the non-linear maximum likelihood (ML) is developed for our EDCT-OFDM-IM system. Moreover, the average bit error probability (ABEP) of the proposed EDCT-OFDM-IM system is derived, which is confirmed by our simulation results. Both simulation and theoretical results are shown that the proposed EDCT-OFDM-IM system exhibits better bit error rate (BER) performance over the conventional DFT-OFDM-IM and DCT-OFDM-IM counterparts.
This work introduces Generalized Space-Time Super-Modulation (GSTSM), a generalization of the recently proposed Space-Time Super-Modulation scheme that enables the transmission of additional, highly-reliable information on the top of conventionally transmitted symbols, without increasing the corresponding packet length. GSTSM jointly exploits the spatial and temporal dimensions of multiple-antenna systems but, in contrast to the initially proposed approach, it does not require the use of space-time block codes. Instead, GSTSM jointly elaborates on the concepts of spatial modulation and spatial diversity, while intentionally introducing temporal correlation to the transmitted symbol sequence. In the context of machine-type communications, GSTSM enables one-shot and grant-free medium access without transmitting additional headers to convey each machine’s ID. As a result, we show that GSTSM can provide throughput gains of up to 2.5 X compared to conventional header-based schemes, even in the case of colliding packets.
Current trends in developing cost-effective and energy-efficient wireless systems operating at millimeter-wave (mm-wave) frequencies and with large-scale phased array antennas for fulfilling the high data-rate demands of 5G and beyond has driven the needs to explore the use of hybrid beamforming technologies. This paper presents an experimental study of a wide-bandwidth millimeter-wave fully-connected hybrid beamformer system that operates at 26 GHz with 128 antenna elements arranged in a 16 x 8 planar array, 6-bit phase shifter, 6-bit attenuators and two separate radio frequency (RF) channels each capable of fully independent beamforming. The linearity, phase, and attenuation performance of the beamformer system between 25.5 GHz and 26.5 GHz are evaluated as well as the beamforming performance of a 128-element planar phased array at 26 GHz where the measured radiation patterns with and without amplitude tapering are compared.
Targeting always the best achievable bit error rate (BER) performance in iterative receivers operating over multiple-input multiple-output (MIMO) channels may result in significant waste of resources, especially when the achievable BER is orders of magnitude better than the target performance (e.g., under good channel conditions and at high signal-to-noise ratio (SNR)). In contrast to the typical iterative schemes, a practical iterative decoding framework that approximates the soft-information exchange is proposed which allows reduced complexity sphere and channel decoding, adjustable to the transmission conditions and the required bit error rate. With the proposed approximate soft information exchange the performance of the exact soft information can still be reached with significant complexity gains.
Sphere decoding (SD) has been proposed as an efficient way to perform maximum-likelihood (ML) decoding of Polar codes. Its latency requirements, however, are determined by its ability to promptly exclude from the ML search (i.e., prune) large parts of the corresponding SD tree, without compromising the ML optimality. Traditional depth-first approaches initially find a “promising" candidate solution and then prune parts of the tree that cannot result to a “better" solution. Still, if this candidate solution is far (in terms of Euclidean distance) from the ML one, pruning becomes inefficient and decoding latency explodes. To reduce this processing latency, an early termination approach is, first, introduced that exploits the binary nature of the transmitted information. Then, a simple but very efficient SD approach is proposed that performs multiple tree searches that perform decreasingly aggressive pruning. These searches are almost independent and can take place sequentially, in parallel, or even in a hybrid (sequential/parallel) manner. For Polar codes of 128 block size, both realizations can provide a latency reduction of up to four orders of magnitude compared to state-of-the-art Polar sphere decoders. Then, a further 50% latency reduction can be achieved by exploiting the parallel nature of the approach.
The future mobile networks will face challenges in support of heterogeneous services over a unified physical layer, calling for a waveform with good frequency localization. Filtered orthogonal frequency division multiplexing (f-OFDM), as a representative subband filtered waveform, can be employed to improve the spectrum localization of orthogonal frequency-division multiplexing (OFDM) signal. However, the applied filtering operations will impact the performance in various aspects, especially for narrow subband cases. Unlike existing studies which mainly focus its benefits, this paper investigates two negative consequences inflicted on single subband f-OFDM systems: in-band interference and filter frequency response (FFR) selectivity. The exact-form expression for the in-band interference is derived, and the effect of FFR selectivity is analyzed for both single antenna and multiple antenna cases. The in-band interference-free and nearly-free conditions for f-OFDM systems are studied. A low-complexity blockwise parallel interference cancellation (BwPIC) algorithm and a pre-equalizer are proposed to tackle the two issues caused by the filtering operations, respectively. Numerical results show that narrower subbands suffer more performance degradation compared to wider bands. In addition, the proposed BwPIC algorithm effectively suppresses interference, and pre-equalized f-OFDM (pf-OFDM) considerably outperforms f- OFDM in both single antenna and multi-antenna systems.
This paper presents a DSP acceleration and assessment framework targeting SDR platforms on x86 64 architectures. Driven by the potential of rapid prototyping and evaluation of breakthrough concepts that these platforms provide, our work builds upon the wellknown OpenAirInterface codebase, extending it for advanced, previously unsupported modes towards large and massive MIMO such as non-codebook-based multi-user transmissions. We then develop an acceleration/profiling framework, through which we present finegrained execution results for DSP operations. Incorporating the latest SIMD instructions, our acceleration framework achieves a unitary speedup of up to 10. Integrated into OpenAirInterface, it accelerates computationally expensive MIMO operations by up to 88% across tested modes. Besides resulting in a useful tool for the community, this work provides insight on runtime DSP complexity and the potential of modern x86 64 systems.
Large multi-user MIMO systems with spatial multiplexing are among the most promising approaches for increasing wireless throughput while serving many clients. Yet, the achievable spectral efficiency of current large MIMO systems is limited by the adoption of simple, but sub-optimal, linear precoding techniques (e.g, minimum-mean-square-error (MMSE)). Nonlinear precoding methods, like Vector Perturbation (VP), claim to be able to provide improved network throughput. However, such methods are still purely theoretical and they do not account for the practical aspects of actual wireless systems, as the corresponding complexity and latency requirements, or the need for practical rate adaptation. This paper presents ViPer, the first practical VP-based MIMO system design. ViPer substantially reduces the latency requirements of VP by employing massively parallel processing and realizes a practical rate adaptation method that efficiently translates VP’s signal-to-noise-ratio (SNR) gains into actual throughput gains. In our first systematic experimental evaluation of VP-based precoders, we show that ViPer can deliver in practice up to 30% higher throughput than MMSE precoding with comparable latency requirements. In addition, ViPer can match the performance of state-of-the-art parallel VP precoding schemes, by utilizing less than one tenth of the processing elements.
This paper proposes a complex-valued discrete multicarrier modulation (MCM) system based on the real-valued discrete Hartley transform (DHT) and its inverse (IDHT). Unlike conventional discrete Fourier transform (DFT), DHT can not diagonalize the multipath fading channel due to its inherent properties, which results in the mutual interference between subcarriers in the same mirror-symmetrical pair.We explore the interference pattern in order to seek an optimal solution to utilize the channel diversity for the purpose of enhancing system bit error performance (BEP). It is shown that the optimal channel diversity gain can be achieved via a pairwise maximum likelihood (ML) detection, taking into account not only the subcarrier’s own channel quality but also the channel state of its mirror-symmetrical peer. Performance analysis indicates that DHT-based MCM mitigates the fast fading effect by averaging the channel power gain on the mirror-symmetrical subcarriers. Simulation results show that the proposed system has a substantial improvement in BEP over conventional DFT-Based MCM.
This paper presents the measurement results and analysis for outdoor wireless propagation channels at 26 GHz over 2 GHz bandwidth for two receiver antenna polarization modes. The angular and wideband properties of directional and virtually omni-directional channels, such as angular spread, root-mean-square delay spread and coherence bandwidth, are analyzed. The results indicate that the reflections can have a significant contribution in some realistic scenarios and increase the angular and delay spreads, and reduce the coherence bandwidth of the channel. The analysis in this paper also show that using a directional transmission can result in an almost frequencyflat fading channel over the measured 2 GHz bandwidth; which consequently has a major impact on the choice of system design choices such as beamforming and transmission numerology.
—Multi-User Multiple-Input, Multiple-Output (MU-MIMO), and massive-MIMO (mMIMO) have been central technologies to the evolution of the latest mobile generations since they promise substantial throughput increase and enhanced connectivity capabilities. Still, the type of signal processing that will unlock the full potential of MU-MIMO developments, has not yet been determined. Existing realizations typically employ linear processing that, despite its practical benefits, can leave capacity and connectivity gains unexploited. On the other hand, traditional non-linear processing solutions (e.g., sphere decoders) promise improved throughput and connectivity capabilities but can be computationally impractical, with exponentially increasing computational complexity, and with their implementability in 5G-NR systems still being unverified. At the same time, emerging new Open Radio Access Network (Open-RAN) designs call for physical layer (PHY) processing solutions that are also practical in terms of realization, even when implemented purely on software. In this work, we present a first, purely software-based, Open-RAN compliant, 5G-NR MIMO PHY that operates in real-time and over-the-air, encompassing both linear and non-linear MIMO processing, and achieving support for 8 concurrently transmitted MIMO streams at a 10MHz bandwidth with just 12 processing cores. Here, we not only demonstrate that implementing non-linear processing is feasible in software within the stringent real-time latency requirements of 5G-NR, but we also compare it side-by-side in a real-time and over-the-air environment against traditional linear methods. We show that the gains of non-linear processing include substantially enhanced throughput with insignificant computational power overhead, the halving of the base-station antennas without performance degradation, and overloading factors of up to 300%.
The vision, as we move to future wireless communication systems, embraces diverse qualities targeting significant enhancements from the spectrum, to user experience. Newly-defined air-interface features, such as large number of base station antennas and computationally complex physical layer approaches come with a non-trivial development effort, especially when scalability and flexibility need to be factored in. In addition, testing those features without commercial, off-the-shelf equipment has a high deployment, operational and maintenance cost. On one hand, industry-hardened solutions are inaccessible to the research community due to restrictive legal and financial licensing. On the other hand, researchgrade real-time solutions are either lacking versatility, modularity and a complete protocol stack, or, for those that are full-stack and modular, only the most elementary transmission modes are on offer (e.g., very low number of base station antennas). Aiming to address these shortcomings towards an ideal research platform, this paper presents SWORD, a SoftWare Open Radio Design that is flexible, open for research, low-cost, scalable and software-driven, able to support advanced large and massive Multiple-Input Multiple- Output (MIMO) approaches. Starting with just a single-input single-output air-interface and commercial off-the-shelf equipment, we create a software-intensive baseband platform that, together with an acceleration/ profiling framework, can serve as a research-grade base station for exploring advancements towards future wireless systems and beyond.
We introduce the concept of Space-Time Super-Modulation according to which additional lowrate and highly reliable information can be transmitted on top of traditionally modulated and spacetime encoded information, without increasing the transmitted block length or degrading their error-rate performance. This is achieved by exploiting the temporal redundancy introduced by the space-time block codes and, specifically, by efficiently mapping transmission patterns to specific information content. We show that Space-Time Super-Modulation can be efficiently used in the context of machine-type communications to enable “one-shot”, “grant-free" joint medium access and rateless data transmission while reducing or even eliminating the need for transmitting preamble sequences. As a result, compared with traditional approaches that use correlatable preamble sequences or encoded preambles to transmit the signature information of transmitted packets, Space-Time Super-Modulation can achieve significant throughput gains. For example, we show up to 35% throughput gains from the second best examined preamble-based scheme when transmitting blocks of 200 bits.
This paper presents the design and implementation of Geosphere, a physical- and link-layer design for access point-based MIMO wireless networks that consistently improves network throughput. To send multiple streams of data in a MIMO system, prior designs rely on a technique called zero-forcing, a way of "nulling" the interference between data streams by mathematically inverting the wireless channel matrix. In general, zero-forcing is highly effective, significantly improving throughput. But in certain physical situations, the MIMO channel matrix can become "poorly conditioned," harming performance. With these situations in mind, Geosphere uses sphere decoding, a more computationally demanding technique that can achieve higher throughput in such channels. To overcome the sphere decoder's computational complexity when sending dense wireless constellations at a high rate, Geosphere introduces search and pruning techniques that incorporate novel geometric reasoning about the wireless constellation. These techniques reduce computational complexity of 256-QAM systems by almost one order of magnitude, bringing computational demands in line with current 16- and 64-QAM systems already realized in ASIC. Geosphere thus makes the sphere decoder practical for the first time in a 4 x 4 MIMO, 256-QAM system. Results from our WARP testbed show that Geosphere achieves throughput gains over multi-user MIMO of 2x in 4 x 4 systems and 47% in 2 x 2 MIMO systems. © 2014 ACM.
—Non-linear detection schemes can substantially improve the achievable throughput and connectivity capabilities of uplink MU-MIMO systems that employ linear detection. However, the complexity requirements of existing non-linear soft detectors that provide substantial gains compared to linear ones are at least an order of magnitude more complex, making their adoption challenging. In particular, joint soft information computation involves solving multiple vector minimization problems, each with a complexity that scales exponentially with the number of users. This work introduces a novel ultra-low-complexity, non-linear detection scheme that performs joint Detection and Approximate Reliability Estimation (DARE). For the first time, DARE can substantially improve the achievable throughput (e.g., 40%) with less than 2× the complexity of linear MMSE, making non-linear processing extremely practical. To enable this, DARE includes a novel procedure to approximate the reliability of the received bits based on the region of the received observable that can efficiently approach the accurately calculated soft detection performance. In addition, we show that DARE can achieve a better throughput than linear detection when using just half the base station antennas, resulting in substantial power savings (e.g., 500 W). Consequently, DARE is a very strong candidate for future power-efficient MU-MIMO developments, even in the case of software-based implementations, as in the case of emerging Open-RAN systems. Furthermore, DARE can achieve the throughput of the state-of-the-art non-linear detectors with complexity requirements that are orders of magnitude lower.
State-of-the-art channel coding schemes promise data rates close to the wireless channel capacity. However, efficient link adaptation techniques are required in order to deliver such throughputs in practice. Traditional rate adaptation schemes, which are reactive and try to “predict” the transmission mode that maximizes throughput based on “transmission quality indicators”, can be highly inefficient in an evolving wireless ecosystem where transmission can become increasingly dynamic and unpredictable. In such scenarios, “rateless” link adaptation can be highly beneficial. Here, we compare popular rateless approaches in terms of gains and practicality in both traditional and more challenging operating scenarios. We also discuss challenges that need to be addressed to make such systems practical for future wireless communication systems.
The recent studies on hybrid beamformers with a combination of switches and phase shifters indicate that such methods can reduce the cost and power consumption of massive multiple-input multiple-output (MIMO) systems. However, most of the works have focused on the scenarios with frequency-flat channel models. This letter proposes an effective approach for such systems in frequency-selective channels and presents the closed-form expressions of the beamformer and the corresponding sum-rates. Compared to the traditional subconnected structures, our approach with a significantly smaller number of phase shifters results in a promising performance.
The paper introduces the concept of Space-Time Super-Modulation according to which additional low rate and highly reliable information can be transmitted by further supermodulating blocks of traditionally modulated and space-time encoded information. This is achieved by exploiting the redundant information introduced by the space-time-block codes and, specifically, by efficiently mapping transmission patterns to specific information content. It is shown that Space-Time SuperModulation can be efficiently used in the context of MachineType-Communications to enable joint medium access and rateless data transmission while minimizing or even eliminating the need for transmitting preamble sequences. Compared with traditional approaches that use encoded preambles or preambles based on Zadoff-Chu sequences to transmit the signature information of transmitted packets, Space-Time Super-Modulation can achieve throughput gains of more than 45% when transmitting blocks of 200 symbols.
MIMO mobile systems, with a large number of antennas at the base-station side, enable the concurrent transmission of multiple, spatially separated information streams and, therefore, enable improved network throughput and connectivity both in uplink and downlink transmissions. Traditionally, to efficiently facilitate such MIMO transmissions, linear base-station processing is adopted, that translates the MIMO channel into several single-antenna channels. Still, while such approaches are relatively easy to implement, they can leave on the table a significant amount of unexploited MIMO capacity. Recently proposed non-linear base-station processing methods claim this unexplored capacity and promise a substantially increased network throughput. Still, to the best of the authors' knowledge, non-linear base-station processing methods not only have not yet been adopted by actual systems, but have not even been evaluated in a standard-compliant framework, involving of all the necessary algorithmic modules required by a practical system. This work, outlines our experience by trying to incorporate and evaluate the gains of non-linear base-station processing in a 3GPP standard environment. We discuss the several corresponding challenges and our adopted solutions, together with their corresponding limitations. We report gains that we have managed to verify, and we also discuss remaining challenges, missing algorithmic components and future research directions that would be required towards highly efficient, future mobile systems that can efficiently exploit the gains of non-linear, base-station processing.
Typical receiver processing, targeting always the best achievable bit error rate performance, can result in a waste of resources, especially, when the transmission conditions are such that the best performance is orders of magnitude better than the required. In this work, a processing framework is proposed which allows adjusting the processing requirements to the transmission conditions and the required bit error rate. It applies a-posteriori probability receivers operating over multiple-input multiple-output channels. It is demonstrated that significant complexity savings can be achieved both at the soft, sphere-decoder based detector and the channel decoder with only minor modifications.
This paper presents the algorithmic design, experimental evaluation, and VLSI implementation of Geosphere, a depth-first sphere decoder able to provide the exact maximumlikelihood solution in dense (e.g., 64) and very dense (e.g., 256, 1024) QAM constellations by means of a geometrically inspired enumeration. In general, linear detection methods can be highly effective when the MIMO channel is well-conditioned. However, this is not the case when the size of the MIMO system increases and the number of transmit antennas approaches the number of the receive antennas. Via our WARP testbed implementation we gather indoor channel traces in order to evaluate the performance gains of sphere detection against zero-forcing and MMSE in an actual indoor environment. We show that Geosphere can nearly linearly scale performance with the number of user antennas; in 4 × 4 multi-user MIMO for 256-QAM modulation at 30 dB SNR there is a 1.7× gain over MMSE and 2.4× over zeroforcing and a 14% and 22% respective gain in 2 × 2 systems. In addition, by using a new node labeling based enumeration technique, low-complexity integer arithmetic and fine-grained clock gating, we implement for up to 1024-QAM constellations and compare in terms of area, delay, power characteristics, the Geosphere VLSI architecture and the best-known best-scalable exact ML sphere decoder. Results show that Geosphere is twice as area-efficient and 70% more energy efficient in 1024-QAM. Even for 16-QAM Geosphere is 13% more area efficient than the best-known implementation for 16-QAM and it is at least 80% more area efficient than state-of-the-art K-best detectors for 64-QAM.
In the last few years, Internet of Things, Cloud computing, Edge computing, and Fog computing have gained a lot of attention in both industry and academia. However, a clear and neat definition of these computing paradigms and their correlation is hard to find in the literature. This makes it difficult for researchers new to this area to get a concrete picture of these paradigms. This work tackles this deficiency, representing a helpful resource for those who will start next. First, we show the evolution of modern computing paradigms and related research interest. Then, we address each paradigm, neatly delineating its key points and its relation with the others. There after, we extensively address Fog computing, remarking its outstanding role as the glue between IoT, Cloud, and Edge computing. In the end, we briefly present open challenges and future research directions for IoT, Cloud, Edge, and Fog computing.
The complexity of depth-first sphere decoders (SDs) is determined by the employed tree search and pruning strategies. Proposed is a new SD approach for maximum-likelihood (ML) detection of spatially multiplexed, high-order, QAM symbols. In contrast to typical ML approaches, the proposed tree traversal skips the computationally intensive requirement of visiting the nodes in ascending order of their partial distances (PDs). Then, a new pruning method efficiently narrows the search space and preserves the ML performance despite the non-ordered tree traversal. This proposed approach results in substantially reduced PD calculations when compared to typical ML SDs and, for high SNRs, the necessary calculations can be reduced down to the number of transmit antennas. © 2012 The Institution of Engineering and Technology.
The intensifying demand for data rate and connectivity has resulted in multi-user multiple-input multiple-output (MU-MIMO) deployments. MU-MIMO allows multiple data streams to transmit concurrently in the same spectrum band. These mutually interfering streams need to be processed at the base station (BS), leading to substantial computational complexity requirements. Linear MIMO detectors/precoders are popular due to their relatively low complexity. However, matrix inversion is a challenging task in linear detectors/precoders. Especially in experimental platforms, software-based inversions are infeasible for a large number of users. This work presents Matrix Inversion on Channel Approximation (MICA), a novel method that aims to reduce the complexity of matrix inversion by exploiting the characteristics of channel correlation in the time domain. In low-mobility scenarios (user speeds less than 20km/h), MICA can reduce the average complexity and processing latency required for computing the inverse of 64 × 12 channel matrices by about 90% compared to a conventional scheme, while maintaining almost the same error rate performance.
While the realization of 5G has already began, we are also experiencing a paradigm shift towards Open Radio Access Network (RAN) environments. This new paradigm promotes open and flexible RAN architectures, which can benefit by the physical layer processing being executed on open-source software and general purpose processors. However, meeting the real-time latency requirements for the computationally intensive process of Low Density Parity Check (LDPC) Decoding, as defined in the 3GPP 5G New Radio (NR), poses a challenge for such software based systems. Based on that, we report the design and implementation our novel FPGA LDPC offloading prototype which we integrate and evaluate with the OpenAirInterface (OAI) codebase, the fastest growing open-source project towards supporting 5G NR. For the first time, we present a detailed quantitative analysis of the capabilities of LDPC offloading into an FPGA in the context of a 3GPP compliant system, with up to 5 times faster execution times for the decoding. Our contribution includes the finding that offloading to specialized hardware does not necessarily improve processing latency in all LDPC configurations, due to overheads related to data transfers.
Conference Title: ICC 2022 - IEEE International Conference on Communications Conference Start Date: 2022, May 16 Conference End Date: 2022, May 20 Conference Location: Seoul, Korea, Republic ofMulti-user (MU), multiple-input, multiple-output (MIMO) detection has been extensively investigated, and many techniques have been proposed. However, further performance improvements may be constrained by limitations in classical computation. The motivation for this work is to test whether a machine that exploits quantum principles can offer improved performance over conventional detection approaches. This paper presents an evaluation of MIMO detection based on quantum annealing (QA) when run on an actual QA quantum processing unit (QPU) and describes the challenges and potential improvements. The evaluations show promising results in some cases, such as near-optimality in a QPSK-modulated 8×8 MIMO case, but poor results in other cases, such as for larger systems or when using 16-QAM. We show that some challenges of QA detection include dealing with integrated control errors (ICE), the limited dynamic range of QA QPUs, an exponential increase in the number of qubits to the problem size, and a high computation overhead. Solving these challenges could make QA-based detection superior to conventional approaches and bring a new generation of MU-MIMO detection methods.
The simultaneous perturbation of an orthogonal frequency-division multiplexing receiver by phase noise plus a residual frequency offset (due to synchronization errors) is modeled here as a combined phase impairment, whose effect is evaluated analytically for the case of a frequency-selective fading channel. A nonpilot-aided (decision-directed) scheme is proposed, which compensates for the common (over all the subcarriers) phase-impairment effect. By representing the resulting intercarrier interference as an uncorrelated, unequal-variance process in the frequency domain, maximum-likelihood (ML) and approximate ML estimators of the complex-vector and phase-only types are derived and analytically evaluated. The present schemes are also compared with other current methods based on individual phase trackers, one per subcarrier. Finally, two suggestions are introduced for increasing the robustness of the algorithms to tentative-decision errors. It is demonstrated through simulations that the analysis is accurate, and that the proposed schemes achieve error-rate performance close to that of ideal compensation. © 2005 IEEE.
To avoid unnecessarily using a massive number of base station antennas to support a large number of users spatially multiplexed multi-user MIMO systems, optimal detection methods are required to demultiplex the mutually interfering information streams. Sphere decoding (SD) can achieve this, but its complexity and latency becomes impractical for large MIMO systems. Low complexity detection solutions such as linear detectors (e.g., MMSE) or likelihood ascendant search (LAS) approaches, have significantly lower latency requirements than SD but their achievable throughput is far from optimal. This work presents the concept of Antipodal detection and decoding, that can deliver very high throughput with practical latency requirements, even in systems where the number of user antennas reaches the number of base station antennas. The Antipodal detector either results in a highly reliable vector solution, or it does not find a vector solution at all (i.e., it results in an erasure), skipping the heavy processing load related to finding vector solutions that have a very high likelihood to be erroneous. Then, a belief-propagation-based decoder is proposed, that restores these erasures and further corrects remaining erroneous vector solutions. We show that for 32⇥32, 64-QAM modulated systems, and for packet error rates below 10%, Antipodal detection and decoding requires 9 dB less transmitted power than systems employing soft MMSE or LAS detection and LDPC decoding with similar complexity requirements. For the same scenario, our Antipodal method achieves practical throughput gains of more than 50% compared to soft MMSE and soft LAS-based methods.
© 2022 IEEE. Personal use of this material is permitted. Permission from IEEE must be obtained for all other uses, in any current or future media, including reprinting/republishing this material for advertising or promotional purposes, creating new collective works, for resale or redistribution to servers or lists, or reuse of any copyrighted component of this work in other works.”