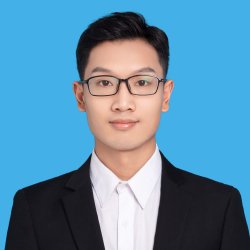
Jiantao Wu
Academic and research departments
Surrey Institute for People-Centred Artificial Intelligence (PAI).About
My research project
Foundation Models for Visual UnderstandingMuch of the success of the AI is attributed to supervised-pretraining (SP) of deep neural networks and then adopting them for downstream applications specific tasks. However, there are several challenges and issues with labelled data, e.g, labelling cost, noisy-labels, incomplete and inadequate labels (focusing on only dominant concept/concepts in images), inherent human labelling bias. An alternative to supervised-learning is self/un-supervised-learning which learns without labels. Two of the key principles of self-supervised-learning in (computer) vision-based AI systems are a) get augmented views of the same input and enforce some notion of consistency between the views, b) mask part of the input and recover that part from rest of the unmasked input.
Although SSL is predicted to be the future of AI research, as put by AI pioneers, “The revolution will not be supervised". However, until very recently self-supervised-pretraining fell behind supervised-pretraining for computer vision applications hindering the realisation of SSL dream. This was changed by Group Masked Model Learning (GMML) which was proposed in our seminal work SiT: Self-supervised vIsion Transformers in 2021. The GMML marked a milestone in AI development as it is the first method to outperform supervised-pretraining and learned semantic concepts without using any labels. The core idea of GMML is adopted by tech giants like Microsoft, Facebook and several others for application areas including, computer vision, audio, medical-image analysis, anomaly detection, multimodal analysis and many more.
Supervisors
Much of the success of the AI is attributed to supervised-pretraining (SP) of deep neural networks and then adopting them for downstream applications specific tasks. However, there are several challenges and issues with labelled data, e.g, labelling cost, noisy-labels, incomplete and inadequate labels (focusing on only dominant concept/concepts in images), inherent human labelling bias. An alternative to supervised-learning is self/un-supervised-learning which learns without labels. Two of the key principles of self-supervised-learning in (computer) vision-based AI systems are a) get augmented views of the same input and enforce some notion of consistency between the views, b) mask part of the input and recover that part from rest of the unmasked input.
Although SSL is predicted to be the future of AI research, as put by AI pioneers, “The revolution will not be supervised". However, until very recently self-supervised-pretraining fell behind supervised-pretraining for computer vision applications hindering the realisation of SSL dream. This was changed by Group Masked Model Learning (GMML) which was proposed in our seminal work SiT: Self-supervised vIsion Transformers in 2021. The GMML marked a milestone in AI development as it is the first method to outperform supervised-pretraining and learned semantic concepts without using any labels. The core idea of GMML is adopted by tech giants like Microsoft, Facebook and several others for application areas including, computer vision, audio, medical-image analysis, anomaly detection, multimodal analysis and many more.
Publications
Self-supervised pretraining (SSP) has emerged as a popular technique in machine learning, enabling the extraction of meaningful feature representations without labelled data. In the realm of computer vision, pretrained vision transformers (ViTs) have played a pivotal role in advancing transfer learning. Nonetheless, the escalating cost of finetuning these large models has posed a challenge due to the explosion of model size. This study endeavours to evaluate the effectiveness of pure self-supervised learning (SSL) techniques in computer vision tasks, obviating the need for finetuning, with the intention of emulating human-like capabilities in generalisation and recognition of unseen objects. To this end, we propose an evaluation protocol for zero-shot segmentation based on a prompting patch. Given a point on the target object as a prompt, the algorithm calculates the similarity map between the selected patch and other patches, upon that, a simple thresholding is applied to segment the target. Another evaluation is intra-object and inter-object similarity to gauge discriminatory ability of SSP ViTs. Insights from zero-shot segmentation from prompting and discriminatory abilities of SSP led to the design of a simple SSP approach, termed MMC. This approaches combines Masked image modelling for encouraging similarity of local features, Momentum based self-distillation for transferring semantics from global to local features, and global Contrast for promoting semantics of global features, to enhance discriminative representations of SSP ViTs. Consequently, our proposed method significantly reduces the overlap of intra-object and inter-object similarities, thereby facilitating effective object segmentation within an image. Our experiments reveal that MMC delivers top-tier results in zero-shot semantic segmentation across various datasets.