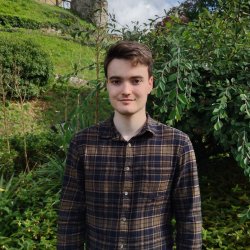
James King
About
My research project
Information theoretic learning for sound analysisThe aim of this PhD project is to investigate information theoretic methods for analysis of sounds. The Information Bottleneck (IB) method has emerged as an interesting approach to investigate learning in deep learning networks and autoencoders. As well as traditional Shannon entropy, the Information Bottleneck method also applies to Renyi and other entropies. Fast and accurate estimation of information is still an active area of research. This project will investigate information-theoretic approaches to analyse sound sequences, both for supervised learning methods such convolutive and recurrent networks, and unsupervised methods such as variational autoencoders. The project will also investigate direct information loss estimators, and new information-theoretic processing structures for sound processing, for example involving both feed-forward and feedback connections inspired by transfer information in biological neural networks.
Supervisors
The aim of this PhD project is to investigate information theoretic methods for analysis of sounds. The Information Bottleneck (IB) method has emerged as an interesting approach to investigate learning in deep learning networks and autoencoders. As well as traditional Shannon entropy, the Information Bottleneck method also applies to Renyi and other entropies. Fast and accurate estimation of information is still an active area of research. This project will investigate information-theoretic approaches to analyse sound sequences, both for supervised learning methods such convolutive and recurrent networks, and unsupervised methods such as variational autoencoders. The project will also investigate direct information loss estimators, and new information-theoretic processing structures for sound processing, for example involving both feed-forward and feedback connections inspired by transfer information in biological neural networks.
Publications
Continuously learning new classes without catastrophic forgetting is a challenging problem for on-device environmental sound classification given the restrictions on computation resources (e.g., model size, running memory). To address this issue, we propose a simple and efficient continual learning method. Our method selects the historical data for the training by measuring the per-sample classification uncertainty. Specifically, we measure the uncertainty by observing how the classification probability of data fluctuates against the parallel perturbations added to the classifier embedding. In this way, the computation cost can be significantly reduced compared with adding perturbation to the raw data. Experimental results on the DCASE 2019 Task 1 and ESC-50 dataset show that our proposed method outperforms baseline continual learning methods on classification accuracy and computational efficiency, indicating our method can efficiently and incrementally learn new classes without the catastrophic forgetting problem for on-device environmental sound classification.