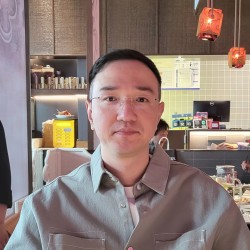
Hongxin Gao
About
My research project
Developing Machine Learning Tools for Predicting Cognitive Impairment from Survey Response BehavioursUnderdiagnosis of cognitive impairment (CI), including mild CI (MCI) and dementia, has become a public health challenge. With the emergence of new treatments aimed at alleviating early cognitive decline, the demand for assessments may increase sharply, putting pressure on our healthcare system.
His PhD project focuses on combining psychometric methods with data science techniques to facilitate early identification of CI in non-clinical settings, such as communities. The project aims to develop behavioural markers of early cognitive deficits by identifying latent inconsistent responses in large-scale surveys, and employing them to establish a deep learning-based prediction tool.
Supervisors
Underdiagnosis of cognitive impairment (CI), including mild CI (MCI) and dementia, has become a public health challenge. With the emergence of new treatments aimed at alleviating early cognitive decline, the demand for assessments may increase sharply, putting pressure on our healthcare system.
His PhD project focuses on combining psychometric methods with data science techniques to facilitate early identification of CI in non-clinical settings, such as communities. The project aims to develop behavioural markers of early cognitive deficits by identifying latent inconsistent responses in large-scale surveys, and employing them to establish a deep learning-based prediction tool.
ResearchResearch interests
Health Informatics · Deep Learning · Large Language Model · Agile Web Development
Research projects
Testing early markers of cognitive decline and dementia derived from survey response behaviorsResearcher - Subaward from US NIH/NIA
University of Surrey PI: Jin
Project PI: Schneider
Research interests
Health Informatics · Deep Learning · Large Language Model · Agile Web Development
Research projects
Researcher - Subaward from US NIH/NIA
University of Surrey PI: Jin
Project PI: Schneider
Publications
Background The underdiagnosis of cognitive impairment hinders timely intervention of dementia. Health professionals working in the community play a critical role in the early detection of cognitive impairment, yet still face several challenges such as a lack of suitable tools, necessary training, and potential stigmatization. Objective This study explored a novel application integrating psychometric methods with data science techniques to model subtle inconsistencies in questionnaire response data for early identification of cognitive impairment in community environments. Methods This study analyzed questionnaire response data from participants aged 50 years and older in the Health and Retirement Study (waves 8-9, n=12,942). Predictors included low-quality response indices generated using the graded response model from four brief questionnaires (optimism, hopelessness, purpose in life, and life satisfaction) assessing aspects of overall well-being, a focus of health professionals in communities. The primary and supplemental predicted outcomes were current cognitive impairment derived from a validated criterion and dementia or mortality in the next ten years. Seven predictive models were trained, and the performance of these models was evaluated and compared. Results The multilayer perceptron exhibited the best performance in predicting current cognitive impairment. In the selected four questionnaires, the area under curve values for identifying current cognitive impairment ranged from 0.63 to 0.66 and was improved to 0.71 to 0.74 when combining the low-quality response indices with age and gender for prediction. We set the threshold for assessing cognitive impairment risk in the tool based on the ratio of underdiagnosis costs to overdiagnosis costs, and a ratio of 4 was used as the default choice. Furthermore, the tool outperformed the efficiency of age or health-based screening strategies for identifying individuals at high risk for cognitive impairment, particularly in the 50- to 59-year and 60- to 69-year age groups. The tool is available on a portal website for the public to access freely. Conclusions We developed a novel prediction tool that integrates psychometric methods with data science to facilitate “passive or backend” cognitive impairment assessments in community settings, aiming to promote early cognitive impairment detection. This tool simplifies the cognitive impairment assessment process, making it more adaptable and reducing burdens. Our approach also presents a new perspective for using questionnaire data: leveraging, rather than dismissing, low-quality data.
Questionnaires are ever present in survey research. In this study, we examined whether an indirect indicator of general cognitive ability could be developed based on response patterns in questionnaires. We drew on two established phenomena characterizing connections between cognitive ability and people’s performance on basic cognitive tasks, and examined whether they apply to questionnaires responses. (1) The worst performance rule (WPR) states that people’s worst performance on multiple sequential tasks is more indicative of their cognitive ability than their average or best performance. (2) The task complexity hypothesis (TCH) suggests that relationships between cognitive ability and performance increase with task complexity. We conceptualized items of a questionnaire as a series of cognitively demanding tasks. A graded response model was used to estimate respondents’ performance for each item based on the difference between the observed and model-predicted response (“response error” scores). Analyzing data from 102 items (21 questionnaires) collected from a large-scale nationally representative sample of people aged 50+ years, we found robust associations of cognitive ability with a person’s largest but not with their smallest response error scores (supporting the WPR), and stronger associations of cognitive ability with response errors for more complex than for less complex questions (supporting the TCH). Results replicated across two independent samples and six assessment waves. A latent variable of response errors estimated for the most complex items correlated .50 with a latent cognitive ability factor, suggesting that response patterns can be utilized to extract a rough indicator of general cognitive ability in survey research.
This paper examined the magnitude of differences in performance across domains of cognitive functioning between participants who attrited from studies and those who did not, using data from longitudinal ageing studies where multiple cognitive tests were administered. Individual participant data meta-analysis. Data are from 10 epidemiological longitudinal studies on ageing (total n=209 518) from several Western countries (UK, USA, Mexico, etc). Each study had multiple waves of data (range of 2-17 waves), with multiple cognitive tests administered at each wave (range of 4-17 tests). Only waves with cognitive tests and information on participant dropout at the immediate next wave for adults aged 50 years or older were used in the meta-analysis. For each pair of consecutive study waves, we compared the difference in cognitive scores (Cohen's d) between participants who dropped out at the next study wave and those who remained. Note that our operationalisation of dropout was inclusive of all causes (eg, mortality). The proportion of participant dropout at each wave was also computed. The average proportion of dropouts between consecutive study waves was 0.26 (0.18 to 0.34). People who attrited were found to have significantly lower levels of cognitive functioning in all domains (at the wave 2-3 years before attrition) compared with those who did not attrit, with small-to-medium effect sizes (overall d=0.37 (0.30 to 0.43)). Older adults who attrited from longitudinal ageing studies had lower cognitive functioning (assessed at the timepoint before attrition) across all domains as compared with individuals who remained. Cognitive functioning differences may contribute to selection bias in longitudinal ageing studies, impeding accurate conclusions in developmental research. In addition, examining the functional capabilities of attriters may be valuable for determining whether attriters experience functional limitations requiring healthcare attention.
In a conventional power grid, energy theft is difficult to detect due to limited communication and data transition. The smart meter along with big data mining technology leads to significant technological innovation in the field of energy theft detection (ETD). This article proposes a convolutional long short-term memory (ConvLSTM)-based ETD model to identify electricity theft users. In this work, electricity consumption data are reshaped quarterly into a 2-D matrix and used as the sequential input to the ConvLSTM. The convolutional neural network (CNN) embedded into the long short-term memory (LSTM) can better learn the features of the data on different quarters, months, weeks, and days. Besides, the proposed model incorporates batch normalization. This technique allows the proposed ETD model to support raw format electricity consumption data input, reducing training time and increasing the efficiency of model deployment. The result of the case study shows that the proposed ConvLSTM model exhibits good robustness. It outperforms the multilayer perceptron (MLP) and CNN-LSTM in terms of performance metrics and model generalization capability. Moreover, the result also demonstrates that K -fold cross validation can improve the ETD prediction accuracy.