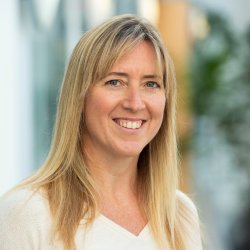
Dr Eleanor Mill
About
My research project
A human-AI collaboration method to inform fraud strategyMy work focuses on how best to implement artificial intelligence in a practical way so as to ensure transparency of what can sometimes be highly complex and opaque automated decision-making. This transparency is particularly relevant in financial decision-making where decisions can be impactful to people's lives, therefore requiring an element of human oversight (and hence understanding) to satisfy moral and regulatory obligations.
Supervisors
My work focuses on how best to implement artificial intelligence in a practical way so as to ensure transparency of what can sometimes be highly complex and opaque automated decision-making. This transparency is particularly relevant in financial decision-making where decisions can be impactful to people's lives, therefore requiring an element of human oversight (and hence understanding) to satisfy moral and regulatory obligations.
University roles and responsibilities
- University Ethics Committee
My qualifications
Publications
This paper demonstrates a design and evaluation approach for delivering real world efficacy of an explainable artificial intelligence (XAI) model. The first of its kind, it leverages three distinct but complimentary frameworks to support a user-centric and context-sensitive, post-hoc explanation for fraud detection. Using the principles of scenario-based design, it amalgamates two independent real-world sources to establish a realistic card fraud prediction scenario. The SAGE (Settings, Audience, Goals and Ethics) framework is then used to identify key context-sensitive criteria for model selection and refinement. The application of SAGE reveals gaps in the current XAI model design and provides opportunities for further model development. The paper then employs a functionally-grounded evaluation method to assess its effectiveness. The resulting explanation represents real-world requirements more accurately than established models.
Regulatory and technological changes have recently transformed the digital footprint of credit card transactions, providing at least ten times the amount of data available for fraud detection practices that were previously available for analysis. This newly enhanced dataset challenges the scalability of traditional rule-based fraud detection methods and creates an opportunity for wider adoption of artificial intelligence (AI) techniques. However, the opacity of AI models, combined with the high stakes involved in the finance industry, means practitioners have been slow to adapt. In response, this paper argues for more researchers to engage with investigations into the use of Explainable Artificial Intelligence (XAI) techniques for credit card fraud detection. Firstly, it sheds light on recent regulatory changes which are pivotal in driving the adoption of new machine learning (ML) techniques. Secondly, it examines the operating environment for credit card transactions, an understanding of which is crucial for the ability to operationalise solutions. Finally, it proposes a research agenda comprised of four key areas of investigation for XAI, arguing that further work would contribute towards a step-change in fraud detection practices.
Scholars often recommend incorporating context into the design of an ex-plainable artificial intelligence (XAI) model in order to deliver the successful integration of an explainable agent into a real-world operating environment. However, few in the field of XAI have expanded upon the meaning of context, or provided clarification as to what they consider its constituent parts. This paper answers that question by providing a thematic review of the extant literature , revealing an interaction between the contextual elements of Setting, Audience, Goals and Ethics (SAGE). This paper therefore proposes SAGE as a conceptual framework that enables researchers to build audience-centric and context-sensitive XAI, thereby strengthening the prospects for successful adoption of an XAI solution.
This paper offers preliminary reflections on the sustainability tensions present in Artificial Intelligence (AI) and suggests that Paradox Theory, an approach borrowed from the strategic management literature, may help guide scholars towards innovative solutions. The benefits of AI to our society are well documented. Yet those benefits come at environmental and sociological cost, a fact which is often overlooked by mainstream scholars and practitioners. After examining the nascent corpus of literature on the sustainability tensions present in AI, this paper introduces the Accuracy-Energy Paradox and suggests how the principles of paradox theory can guide the AI community to a more sustainable solution.
Machine learning (ML, a type of artificial intelligence) is increasingly being used to support decision making in a variety of applications including recruitment and clinical diagnoses. While ML has many advantages, there are concerns that in some cases it may not be possible to explain completely how its outputs have been produced. This POSTnote gives an overview of ML and its role in decision-making. It examines the challenges of understanding how a complex ML system has reached its output, and some of the technical approaches to making ML easier to interpret. It also gives a brief overview of some of the proposed tools for making ML systems more accountable.