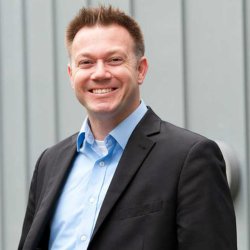
Dr Wolfgang Garn
About
Biography
I have held roles such as Programme Director of Business Analytics and Acting Head of the Business Transformation Department at the University of Surrey. My research interests include applied mathematics, operational research, and business analytics, with a particular focus on technology-related fields such as Virtual Reality, Artificial Intelligence, Game Engines, Discrete Event Simulation (DES), and Unmanned Aerial Vehicles (UAVs), including quadcopters. In my industry work, I have developed analytics solutions, optimizations, and process simulations. I have led knowledge transfer partnerships with Tilda Rice, Royal Mail, Hastoe, Surrey County Council, and Basemap.
Earlier in my career, I worked as a Mathematician in the Department of Operations Research at Telekom Austria, where I focused on network optimizations, transportation modelling, and market analysis. One of my key achievements was developing a mathematical model for a nationwide strategy to transition from a copper to a fibre-optic network.
At the Defence Technology Centre (DTC), I contributed to research on MoD-funded Agent and Decision Support Systems. A significant outcome of this work was enabling autonomous agents to evaluate and respond to military effects using Artificial Intelligence (Bayesian Networks).
As a Senior Scientist and Project Manager at Eurobios, I worked with key clients such as Serco, Biffa, Unilever, DHL, and BP, implementing mathematical solutions for environmental and delivery services.
I am also the CEO and founder of Smartana, a company that provides businesses with SMART analytics solutions and consulting services. I am a current/former member of the Institute for Operations Research and the Management Sciences (INFORMS) and the Society for Modeling & Simulation International (SCS).
I earned my PhD in the Simulation and Optimization of Telecommunications Processes from the Vienna University of Technology, specializing in Technical Mathematics and Computer Science.
Affiliations and memberships
ResearchResearch interests
- Business Analytics such as optimisations and mathematical programming and modelling
- Operational Research such as logistics, transportation, routing, scheduling
- Management Science, combinatorial optimisations, network flows, meta heuristics, e.g. genetic algorithms, simulated annealing
- Applied mathematics, Artificial Intelligence, Discrete Event Simulation (DES), Queueing Systems
- Kernel Density Estimators (KDE), Decision Support Systems (DSS), Bayesian Networks, Statistical Learning and Machine Learning.
Research projects
To develop a holistic logistics management routing software tool which combines scheduling and routing with critical vehicle and environmental performance factors to enable rapid entry and growth in the commercial electric transport market.
Bus Analytics to increase the number of bus passenger.
Factors such as fare, frequency and reliability are analysed. Changes to existing routes (e.g. moving stops) are investigated. New routes are proposed. The complete network in Surrey is considered.
Machine learning techniques to gain insights into the sustainability of homes
The rise of the machines - learning for environmental good and cost savings (Blog, May 24, 2017)
Are we “boiled” for choice? (Blog, August 3, 2017)
AI detects roof status using Drones (BA MSc dissertation summary, October 10, 2017)
To build an advanced predictive tool to provide improved business decision making that promotes sustainable living for the social housing sector and provides efficient savings to housing providers.
Business Analytics combined with Swift Even Flow. Optimisations and simulations of letter/parcel sorting process.
Tilda Rice - KTP projectInventory optimisations
Drone Delivery Service - NEMODE fundedoperational comparison of traditional vs. drone delivery services
Engine Export - ConsultancyExpert System for Actionable Data Insights
Indicators of esteem
Reviewer for the ...
- European Journal of Operational Research
- Neurocomputing Journal
- International Journal of Production Economics
- International Conference on Information Systems
- and many more
Research interests
- Business Analytics such as optimisations and mathematical programming and modelling
- Operational Research such as logistics, transportation, routing, scheduling
- Management Science, combinatorial optimisations, network flows, meta heuristics, e.g. genetic algorithms, simulated annealing
- Applied mathematics, Artificial Intelligence, Discrete Event Simulation (DES), Queueing Systems
- Kernel Density Estimators (KDE), Decision Support Systems (DSS), Bayesian Networks, Statistical Learning and Machine Learning.
Research projects
To develop a holistic logistics management routing software tool which combines scheduling and routing with critical vehicle and environmental performance factors to enable rapid entry and growth in the commercial electric transport market.
Bus Analytics to increase the number of bus passenger.
Factors such as fare, frequency and reliability are analysed. Changes to existing routes (e.g. moving stops) are investigated. New routes are proposed. The complete network in Surrey is considered.
Machine learning techniques to gain insights into the sustainability of homes
The rise of the machines - learning for environmental good and cost savings (Blog, May 24, 2017)
Are we “boiled” for choice? (Blog, August 3, 2017)
AI detects roof status using Drones (BA MSc dissertation summary, October 10, 2017)
To build an advanced predictive tool to provide improved business decision making that promotes sustainable living for the social housing sector and provides efficient savings to housing providers.
Business Analytics combined with Swift Even Flow. Optimisations and simulations of letter/parcel sorting process.
Inventory optimisations
operational comparison of traditional vs. drone delivery services
Expert System for Actionable Data Insights
Indicators of esteem
Reviewer for the ...
- European Journal of Operational Research
- Neurocomputing Journal
- International Journal of Production Economics
- International Conference on Information Systems
- and many more
Supervision
Postgraduate research supervision
PhD - students
I am looking for PhD students! You should have a strong quantitative background (e.g. mathematics, computer science, physics, etc.). You should be interested in Business Analytics, Artificial Intelligence and/or Management Science (Operational Research).
Potential PhD topic areas:
- Business Analytics,
- Operational Research, or
- Artificial Intelligence.
Please send me a short email to express your interest.
- PhD studentships, PhD scholarships, PhD funds or PhD sponsorships might be possible,
- general information for PhD applicants.
Current PhD students
- Melanie Rich - investigates collaborative workspaces
- Sheeba Pathak - works on sustainable supply chains
- Lin Fu - researches marketing, operations and systems
Previous doctoral students
- Eleanor Mill - researched explainable AI in fraud detection (completed successfully in 2024)
- Athary Alwasel - researched hybrid simulation and behaviour in health care (completed successfully in 2023)
- Vasilis Nikolaou - researched machine learning applications to medicine (completed successfully in 2023)
- Daniel Boos - researched predicting bankruptcy of banks (completed successfully in 2022)
- Angélique Gatsinzi – researched young faces in dangerous places : a critical re-appraisal of the child labour debate in Africa’s artisanal and small-scale mining sector (completed successfully in 2019)
- Martin Schreiner – researched Managing purchasing efficacy through reasoning : an action research on the impact of the TOC logical thinking processes to increase purchasing efficacy (completed successfully in 2018)
- Katja Hiltl – Decision Making in the Aviation Industry: Deriving the Operational Factor Approach to Determine Critical Spare Parts Inventory: The Case of XYZ Cargo (completed successfully in 2014)
- Dirk Muehlenmeister (temporarily withdrawn)
- Frank Altmeyer (temporarily withdrawn)
- Iman Roozbeh (withdrawn)
- Naveed Akhtar Qureshi (completed)
Research/doctoral student examinations
- PhD confirmation - Internal Chair for Eyup Kar (2023 in the UK)
- PhD confirmation – Internal Chair for Abdulaziz Alshammari (2023 in the UK)
- PhD – Internal Chair for Dimitra Pappa (2017 in the UK)
- PhD – External examiner for Olubusola Tejumola (Oct. 2016 in the UK)
- DBA – Internal examiner for Julia Bartels (2015 in the UK)
- PhD - Internal examiner and chair for Natasha Mashanovic (2011 in the UK)
Teaching
- Book recommendations:
- Business Analytics (MSc programme)
- Business Analytics arms you with the expertise in analysing data and creating knowledge - leading to competitive advantages for businesses. Artificial intelligence, machine learning and management science power decisions in business. You’ll gain data-led insights and optimise businesses by using descriptive, predictive and prescriptive analytics. It equips you with state-of-the-art and new emerging tools to solve business-transforming challenges. General enquiries: admissions@surrey.ac.uk, programme enquiries: Colin Fu (c.fu@surrey.ac.uk)
- Machine Learning/AI and Visualisation - Semester 2 & 3 (2024 - 2025)
- This module dives into the intrinsic details of machine learning and artificial intelligence methods to apply and fine-tune them. Implementation details of regression trees, kNN, SVM, genetic algorithms and many more algorithms are discussed.
- Module catalogue (MANM547)
- Book: Data Analytics for Business: AI-ML-PBI-SQL-R available via Routledge
- Principles of Analytics - Semester 1 & 2 (2022 - 2024)
- Industry solutions are based on data insights. Guided by the frameworks CRISP-DM, KDD and the Machine Learning roadmap the module goes from the backend (databases) to the frontend (PowerBI) and uses the data science language R and SPSS Modeler to generate solutions (e.g target, classify and profile potential customers, predict house prices, predict drug use).
- Module catalogue (MANM530)
- Book: Data Analytics for Business: AI-ML-PBI-SQL-R available via Routledge
- Manager Decision Making and Insight - Semester 1 & 2 (2022, MBA)
- The module's main aim is to introduce topics in the area of Business Intelligence and Analytics. It is a combination of generic (strategic) information & approaches and practical (tactical) tools.
- Module catalogue (MANM342)
- Book: Data Analytics for Business: AI-ML-PBI-SQL-R available via Routledge and Introduction to Management Science: Modelling, Optimisation and Probability available via BibliU
- Machine Learning and Visualisations - Semester 2 (until 2020, MSc)
- Machine Learning & Visualisations are used to gain business insights for decision-making. Data will be sliced, diced and visually analysed. Artificial Intelligence and statistical learning will be introduced. Techniques will be used for prediction, estimation or classification.
- Module catalogue (MANM354)
- Data Analytics - Semester 1 & 2 (2020 - 2022, MSc) [with interactive online tutorial]
- This module is the science of examining raw data to support businesses and organisations in their decision-making. On one hand this module looks at relationships of entities in databases using the Structured Query Language to extract relevant information efficiently. On the other hand it introduces unstructured data concepts. Special focus is given to Big Data providing knowledge, analysis and practical skills to gain additional business and customer insights. Fundamental statistical techniques to extract the essential management information are shown.
- Module catalogue (MANM301)
- Book: Data Analytics for Business: AI-ML-PBI-SQL-R available via Routledge
- Supply Chain Analytics (level M) - Semester 2 (until 2019, now known as Operational Analytics, MSc)
- Operational Research/Management Science is used to solve supply chain (SC) aspects analytically. Mathematical programming techniques examine the Supply Chain's underlying transportation network which connects suppliers via transshipment nodes to its demand locations. Best locations for warehouses (or transshipment nodes) are determined using quantitative methods. Decision Science is used for in rational decision making under uncertainty. For instance optimal inventory levels are determined for warehouses and manufacturing using mathematical models. All kinds of business activities are optimised to give businesses a competitive advantage by maximising profit and minimising costs.
- Module catalogue (MANM304)
- Book: Introduction to Management Science: Modelling, Optimisation and Probability available via BibliU
- Introduction to Management Science - Semester 1 (until 2019, BA)
- Methods and tools are used to tackle challenges occurring in the business and industrial environment. The obtained results are used for qualified decision making. This is an Applied Mathematics course.
- Module catalogue (MAN2093)
- Book recommendation: Introduction to Management Science: Modelling, Optimisation and Probability
- Book: Introduction to Management Science: Modelling, Optimisation and Probability available via BibliU
- Information Systems Development - Semester 2 (2012, MSc)
- A hands-on approach to the development of Information Systems - using practical State-of-the-Art methods, tools and techniques.
- Module catalogue (MAN114)
- Issues in Operations Management (UL2) - Semester 1 (2010/2011, MSc)
- This lecture explores a set of critical areas in Operations Management in depth which is based on mathematical models (Operational Research/Management Science approach).
- Module catalogue (MAN2086)
- Project Management & Computer Lab. (PG, UG) - Semester 2 (2012, BA, MSc, MBA)
- A practical approach to managing projects (using MS Project)
- Module catalogue: (MAN3104-UG), (MANM020-PG)
- Business Research Project (FHEQ6 - year 3) - Semester 2 (2014, BA)
- To analyse and critically evaluate existing work in order to deliver value to businesses. This module introduces statistical and quantitative methods.
- Module catalogue (MAN3116)
- Business Process Management - Semester 2 (2013, MSc)
- Shows the relationship between operations management and information systems, with hands-on experience in SAP. This include Discrete Event Simulations.
- Module catalogue
Publications
Highlights
Books
- Garn, W. (2024). Data Analytics for Business: AI-ML-PBI-SQL-R. Routledge
- Garn, W. (2018). Introduction to Management Science: Modelling, Optimisation and Probability. Smartana Ltd Publishing.
As we progress towards Industry 5.0, technological advancements are converging; this movement is realised by the increasing collaboration between humans and intelligent digital platforms and further enabled by the interactive visualisation modes provided by Metaverse technology. This research examines the practical applications and limitations of Metaverse technology providing insights into the transformative possibilities it offers for the manufacturing sector. Specifically, the research was guided by the core objective to trace the evolution of Metaverse technology within manufacturing. This study provides a comprehensive and state-of-the-art analysis of the adoption and impact of Metaverse technologies in the manufacturing sector. While previous research has explored aspects of Industry 4.0 and digital transformation, this study specifically focuses on human-centric manufacturing (human-in-the-loop) applications of Metaverse technology, including augmented reality, virtual reality, digital twins, and cyber-physical robotic systems. Findings from the systematic literature review indicate that Metaverse technologies, primarily augmented reality and virtual reality, have evolved into powerful tools in manufacturing. They are widely adopted across sectors in the industry, transforming processes such as product design, quality control, and maintenance. Augmented reality and virtual reality offer intuitive ways to visualise data and interact with digital twins, bridging the gap between physical and virtual realms in manufacturing. A roadmap and scenarios for the introduction of Metaverse technology in manufacturing are provided with suggested adoption timespans. Furthermore, the systematic literature review identified barriers hindering the wider adoption of Metaverse technology in manufacturing.
Public transport (PT) is crucial for enhancing the quality of life and enabling sustainable urban development. As part of the UK Transport Investment Strategy , increasing PT usage is critical to achieving efficient and sustainable mobility. This paper introduces Machine Learning Influence Flow Analysis (MIFA), a novel framework for identifying the key influencers of PT usage. Using survey data from bus passengers in Southern England, we evaluate machine learning models. Subsequently, MIFA uncovers that easy payments, e-ticketing, and mobile applications can substantially improve the PT service. MIFA's implementation demonstrates that strength and importance lead to specific insights into how service characteristics impact user decisions. Practical implications include deploying smart ticketing systems and contactless payments to streamline bus usage. Our results suggest that these strategies can enable bus operators to allocate resources more effectively, leading to increased ridership and enhanced user satisfaction.
What would you like to get out of Data Analytics? Data processing, data mining, tools, data structuring, data insights and data storage are typical first responses. So, we defnitely want to analyse, manipulate, visualise and learn about tools to help us in this endeavour. We do not want to analyse the data for the sake of analysing it; the insights need to be actionable for businesses, organisations or governments. How do we achieve this? The process of discovering knowledge in databases and CRISP-DM helps us with this. Of course, we need to know about databases. There are tools such as Power BI which allow us to transform, analyse and visualise data. So we are "analysing" the data - analysing ranges from formulating a data challenge in words to writing a simple structured query and up to applying mathematical methods to extract knowledge. Of course, the "fun" part is refected in state-of-the-art methods implemented in data mining tools. But in Data Analytics, your mind is set to ensure your findings are actionable and relevant to the business. For instance, can we: find trading opportunities, figure out the most important products, identify relevant quality aspects and many more so that the management team can devise actions that benefit the business? This motivates the following definition: Data Analytics is the discipline of extracting actionable insights by structuring, processing, analysing and visualising data using methods and software tools. Where does Data Analytics "sit" in the area of Business Analytics? Often, Data Analytics is mentioned in conjunction with Business Analytics. Data Analytics can be seen as part of Business Analytics. Business Analytics also includes Operational Analytics. It has become fashionable to divide analytics into Descriptive, Predictive, and Prescriptive Analytics. Sometimes these terms are further refined by adding Diagnostic and Cognitive Analytics. What is what?
This paper demonstrates a design and evaluation approach for delivering real world efficacy of an explainable artificial intelligence (XAI) model. The first of its kind, it leverages three distinct but complimentary frameworks to support a user-centric and context-sensitive, post-hoc explanation for fraud detection. Using the principles of scenario-based design, it amalgamates two independent real-world sources to establish a realistic card fraud prediction scenario. The SAGE (Settings, Audience, Goals and Ethics) framework is then used to identify key context-sensitive criteria for model selection and refinement. The application of SAGE reveals gaps in the current XAI model design and provides opportunities for further model development. The paper then employs a functionally-grounded evaluation method to assess its effectiveness. The resulting explanation represents real-world requirements more accurately than established models.
Many business challenges require advanced, adapted or new data-mining techniques. For this endeavour, Data Science languages are needed. Often Business or Data Analytics tasks have to be automatised, and programming using Data Science languages such as R or Python comes in handy.
Business Intelligence (BI) uses Data Analytics to provide insights to make business decisions. Tools like Power Business Intelligence (PBI) and Tableau allow us to gain an understanding of a business scenario rapidly. This is achieved by first allowing easy access to all kinds of data sources (e.g. databases, text files, Web). Next, the data can be visualised via graphs and tables, which are connected and interactive. Then, analytical components such as forecasts, key influencers and descriptive statistics are built in, and access to the most essential Data Science languages (R and Python) is integrated.
We are drowning in data but are starved for knowledge. Data Analytics is the discipline of extracting actionable insights by structuring, processing, analysing and visualising data using methods and software tools. Hence, we gain knowledge by understanding the data. A roadmap to achieve this is encapsulated in the knowledge discovery in databases (KDD) process. Databases help us store data in a structured way. The structure query language (SQL) allows us to gain first insights about business opportunities. Visualising the data using business intelligence tools and data science languages deepens our understanding of the key performance indicators and business characteristics. This can be used to create relevant classification and prediction models; for instance, to provide customers with the appropriate products or predict the eruption time of geysers. Machine learning algorithms help us in this endeavour. Moreover, we can create new classes using unsupervised learning methods, which can be used to define new market segments or group customers with similar characteristics. Finally, artificial intelligence allows us to reason under uncertainty and find optimal solutions for business challenges. All these topics are covered in this book with a hands-on process, which means we use numerous examples to introduce the concepts and several software tools to assist us. Several interactive exercises support us in deepening the understanding and keep us engaged with the material. This book is appropriate for master students but can also be used for undergraduate students. Practitioners will also benefit from the readily available tools. The material was especially designed for Business Analytics degrees with a focus on Data Science and can also be used for machine learning or artificial intelligence classes. This entry-level book is ideally suited for a wide range of disciplines wishing to gain actionable data insights in a practical manner.
In this chapter, unsupervised learning will be introduced. In contrast to supervised learning, no target variable is available to guide the learning via quality measures.
This chapter introduces databases and the Structured Query Language (SQL). Most of today's data is stored in databases within tables. In order to gain knowledge and make valuable business decisions, it is necessary to extract information efficiently. This is achieved using the Structured Query Language. Good relational databases avoid repetitive data using standard normalisation approaches: for instance, by splitting raw data into multiple tables. These tables (=entities) are linked (=related) using business rules. Structured queries make use of entity relationships to obtain data. This is one of the fundamental concepts of data mining of structured data.
It is always good to follow a strategic roadmap. We motivate Data Analytics roadmaps by first developing a business scenario and introducing associated modelling. Here, formal definitions for real and prediction models are provided. These are of foremost importance. We will use them to derive one of the most commonly used measures - the mean squared error (MSE) - to evaluate the quality of a data mining model. Several business applications are mentioned to give an idea of which kind of projects can be built around a simple linear model. The models and quality measures provide us with a solid foundation for the frameworks. This chapter introduces the methodology of knowledge discovery in databases (KDD), which identifies essential steps in the Data Analytics life-cycle process. We discuss KDD with the help of some examples. The different stages of KDD are introduced, such as data preprocessing and data modelling. We explore the cross-industry standard process for data mining (CRISP-DM).
Artificial intelligence (AI) is concerned with: Enabling computers to "think"; Creating machines that are "intelligent"; Enabling computers to perceive, reason and act; Synthesising intelligent behaviour in artefacts. Previously, we looked at statistical and machine learning. Learning is an essential area of AI. But this is only one part; it also includes problem solving (e.g. optimisations), planning, communicating, acting and reasoning.
Classic statistical learning methods are linear and logistic regression. Both methods are supervised learners. That means the target and input features are known. Linear regression is used for predictions, whilst logistic regression is a classifier. These methods are well-established and have many benefits. For instance, a lot of the theory of the methods is understood and the resulting models are easy to interpret. To evaluate the quality of the prediction and classification models, we need to understand the errors. This chapter uses an example to introduce the concept of errors.
Machine learning (ML) is mainly concerned with identifying patterns in data in order to predict and classify. Previously, we used linear regression and logistic regression to achieve this. However, we heard about them in the context of statistical learning (SL) since they are widely used for inference about the population and hypotheses. Typically, SL relies on assumptions such as normality, homoscedasticity, independent variables and others, whereas ML often ignores these. We continue with supervised learning (i.e. the response is known) approaches. Please be sure you have done the statistical learning chapter. Particularly, we will look at tree-based methods such as the decision tree and random forest. Then we will look at a nearest neighbour approach.
While the move towards Industry 4.0 has motivated a re-evaluation of how a manufacturing organization should operate in light of the availability of a new generation of digital production equipment, the new emphasis is on human worker inclusion to provide decision making activities or physical actions (at decision nodes) within an otherwise automated process flow; termed by some authors as Industry 5.0 and seen as related to the earlier Japanese Society 5.0 concept (seeking to address wider social and environmental problems with the latest developments in digital system, artificial Intelligence and automation solutions). As motivated by the EU the Industry 5.0 paradigm can be seen as a movement to address infrastructural resilience, employee and environmental concerns in industrial settings. This is coupled with a greater awareness of environmental issues, especially those related to Carbon output at production and throughout manufactured products lifecycle. This paper proposes the concept of dynamic Life Cycle Assessment (LCA), enabled by the functionality possible with intelligent products. A particular focus of this paper is that of human in the loop assisted decision making for end-of-life disassembly of products and the role intelligent products can perform in achieving sustainable reuse of components and materials. It is concluded by this research that intelligent products must provide auditable data to support the achievement of net zero carbon and circular economy goals. The role of the human in moving towards net zero production, through the increased understanding and arbitration powers over information and decisions, is paramount; this opportunity is further enabled through the use of intelligent products.
Abstract The mTSP is solved using an exact method and two heuristics, that balances the number of nodes per route. The first heuristic uses a nearest node approach and the second assigns the closest salesman. A comparison of heuristics with test-instances being in the Euclidean plane showed that the closest node approach delivers better solutions and a faster runtime. On average, the closest node solutions are approximately one percent better than the other heuristic. Furthermore, it is found that increasing the number of salesman or customers results in a distance growth for uniformly distributed nodes in an Euclidean grid plane. The distance growth is almost proportional to the square root of number of customers (nodes). In this context we reviewed the expected distance of two uniformly distributed random (real and integer) points. The minimum distance of a node to n uniformly distributed random (real and integer) points was derived and expressed as functional relationship. This gives theoretical underpinnings for - previously - empirical distance to salesmen growth insights.
Cardiovascular diseases are a significant global health concern, responsible for one-third of deaths worldwide and posing a substantial burden on society and national healthcare systems. To effectively address this challenge and develop targeted intervention strategies, the ability to predict cardiovascular diseases from standardized assessments, such as occupational health encounters or national surveys, is critical. This study aims to assist these efforts by identifying a set of biomarkers, which together with known risk factors, can predict cardiovascular diseases on the onset. We used a sample of 7,767 individuals from the UK household longitudinal study ‘Understanding Society’ to train several machine learning models able to pinpoint biomarkers and risk factors at baseline that predict cardiovascular diseases at a ten-year follow-up. A logistic regression model was trained for comparison. A gaussian naïve bayes classifier returned 82% recall in contrast to 48% of the logistic regression, allowing us to identify the most prominent biomarkers predicting cardiovascular diseases. These findings show the opportunity to use machine learning to identify a wide range of previously overlooked biomarkers associated with cardiovascular diseases onset and thus encourage the implementation of such a model in the early diagnosis and prevention of cardiovascular diseases in future research and practice.
Regulatory and technological changes have recently transformed the digital footprint of credit card transactions, providing at least ten times the amount of data available for fraud detection practices that were previously available for analysis. This newly enhanced dataset challenges the scalability of traditional rule-based fraud detection methods and creates an opportunity for wider adoption of artificial intelligence (AI) techniques. However, the opacity of AI models, combined with the high stakes involved in the finance industry, means practitioners have been slow to adapt. In response, this paper argues for more researchers to engage with investigations into the use of Explainable Artificial Intelligence (XAI) techniques for credit card fraud detection. Firstly, it sheds light on recent regulatory changes which are pivotal in driving the adoption of new machine learning (ML) techniques. Secondly, it examines the operating environment for credit card transactions, an understanding of which is crucial for the ability to operationalise solutions. Finally, it proposes a research agenda comprised of four key areas of investigation for XAI, arguing that further work would contribute towards a step-change in fraud detection practices.
Scholars often recommend incorporating context into the design of an ex-plainable artificial intelligence (XAI) model in order to deliver the successful integration of an explainable agent into a real-world operating environment. However, few in the field of XAI have expanded upon the meaning of context, or provided clarification as to what they consider its constituent parts. This paper answers that question by providing a thematic review of the extant literature , revealing an interaction between the contextual elements of Setting, Audience, Goals and Ethics (SAGE). This paper therefore proposes SAGE as a conceptual framework that enables researchers to build audience-centric and context-sensitive XAI, thereby strengthening the prospects for successful adoption of an XAI solution.
Industrial practices and experiences highlight that demand is dynamic and non-stationary. Research however has historically taken the perspective that stochastic demand is stationary therefore limiting its impact for practitioners. Manufacturers require schedules for multiple products that decide the quantity to be produced over a required time span. This work investigated the challenges for production in the framework of a single manufacturing line with multiple products and varying demand. The nature of varying demand of numerous products lends itself naturally to an agile manufacturing approach. We propose a new algorithm that iteratively refines production windows and adds products. This algorithm controls parallel genetic algorithms (pGA) that find production schedules whilst minimizing costs. The configuration of such a pGA was essential in influencing the quality of results. In particular providing initial solutions was an important factor. Two novel methods are proposed that generate initial solutions by transforming a production schedule into one with refined production windows. The first method is called factorial generation and the second one fractional generation method. A case study compares the two methods and shows that the factorial method outperforms the fractional one in terms of costs.
In this paper a demand time series is analysed to support Make-To-Stock (MTS) and Make-To-Order (MTO) production decisions. Using a purely MTS production strategy based on the given demand can lead to unnecessarily high inventory levels thus it is necessary to identify likely MTO episodes. This research proposes a novel outlier detection algorithm based on special density measures. We divide the time series' histogram into three clusters. One with frequent-low volume covers MTS items whilst a second accounts for high volumes which is dedicated to MTO items. The third cluster resides between the previous two with its elements being assigned to either the MTO or MTS class. The algorithm can be applied to a variety of time series such as stationary and non-stationary ones. We use empirical data from manufacturing to study the extent of inventory savings. The percentage of MTO items is reflected in the inventory savings which were shown to be an average of 18.1%.
This paper offers preliminary reflections on the sustainability tensions present in Artificial Intelligence (AI) and suggests that Paradox Theory, an approach borrowed from the strategic management literature, may help guide scholars towards innovative solutions. The benefits of AI to our society are well documented. Yet those benefits come at environmental and sociological cost, a fact which is often overlooked by mainstream scholars and practitioners. After examining the nascent corpus of literature on the sustainability tensions present in AI, this paper introduces the Accuracy-Energy Paradox and suggests how the principles of paradox theory can guide the AI community to a more sustainable solution.
The rise in EVs popularity, combined with reducing emissions and cutting costs, encouraged delivery companies to integrate them in their fleets. The fleet heterogeneity brings new challenges to the Capacitated Vehicle Routing Problem (CVRP). Driving range and different vehicles' capacity constraints must be considered. A cluster-first, route-second heuristic approach is proposed to maximise the number of parcels delivered. Clustering is achieved with two algorithms: a capacitated k-median algorithm that groups parcel drop-offs based on customer location and parcel weight; and a hierarchical constrained minimum weight matching clustering algorithm which considers EVs' range. This reduces the solution space in a meaningful way for the routing. The routing heuristic introduces a novel Monte-Carlo Tree Search enriched with a custom objective function, rollout policy and a tree pruning technique. Moreover, EVs are preferred overother vehicles when assigning parcels to vehicles. A Tabu Search step further optimises the solution. This two-step procedure allows problems with thousands of customers and hundreds of vehicles to be solved accomodating customised vehicle constraints. The solution quality is similar to other CVRP implementations when applied to classic VRP test-instances; and its quality is superior in real-world scenarios when constraints for EVs must be used.
This research examines the application of the Theory of Swift, Even Flow (TSEF) by a distribution company to improve the performance of its processes for parcels. TSEF was deployed by the company after experiencing lean improvement fatigue and diminishing returns from the time and effort invested. This case study combined quantitative and qualitative approaches to develop a good understanding of the operation. This approach enabled the business to utilise Discrete Event Simulation (DES), which facilitated the implementation of TSEF. From this study, the development of a novel DES application revealed the primacy of process variation and throughput time, key factors in TSEF, in driving improvements. The derived DES approach is reproducible and demonstrates its utility with production improvement frameworks. TSEF, through the visualisations and analysis provided by DES, broadened the scope of improvements to an enterprise level, therefore assisting the business managers in driving forward when lean improvement techniques stagnated. The impact of the research is not limited to the theoretical contribution, as the combination of DES and TSEF led to significant managerial insights on how to overcome obstacles and substantiate change.
Scheduling multiple products with limited resources and varying demands remain a critical challenge formany industries. This work presents mixed integer programs(MIPs) that solve the Economic Lot SizingProblem (ELSP) and other Dynamic Lot-Sizing (DLS) models with multiple items. DLS systems are clas-sified, extended and formulated as MIPs. Especially, logical constraints are a key ingredient in succeedingin this endeavour. They were used to formulate the setup/changeover of items in the production line. Min-imising the holding, shortage and setup costs is the primaryobjective for ELSPs. This is achieved by findingan optimal production schedule taking into account the limited manufacturing capacity. Case studies for aproduction plants are used to demonstrate the functionality of the MIPs. Optimal DLS and ELSP solutionsare given for a set of test-instances. Insights into the runtime and solution quality are given.
Purpose Chest x-rays are a fast and inexpensive test that may potentially diagnose COVID-19, the disease caused by the novel coronavirus. However, chest imaging is not a first-line test for COVID-19 due to low diagnostic accuracy and confounding with other viral pneumonias. Recent research using deep learning may help overcome this issue as convolutional neural networks (CNNs) have demonstrated high accuracy of COVID-19 diagnosis at an early stage. Methods We used the COVID-19 Radiography database [36], which contains x-ray images of COVID-19, other viral pneumonia, and normal lungs. We developed a CNN in which we added a dense layer on top of a pre-trained baseline CNN (EfficientNetB0), and we trained, validated, and tested the model on 15,153 X-ray images. We used data augmentation to avoid overfitting and address class imbalance; we used fine-tuning to improve the model’s performance. From the external test dataset, we calculated the model’s accuracy, sensitivity, specificity, positive predictive value, negative predictive value, and F1-score. Results Our model differentiated COVID-19 from normal lungs with 95% accuracy, 90% sensitivity, and 97% specificity; it differentiated COVID-19 from other viral pneumonia and normal lungs with 93% accuracy, 94% sensitivity, and 95% specificity. Conclusions Our parsimonious CNN shows that it is possible to differentiate COVID-19 from other viral pneumonia and normal lungs on x-ray images with high accuracy. Our method may assist clinicians with making more accurate diagnostic decisions and support chest X-rays as a valuable screening tool for the early, rapid diagnosis of COVID-19.
Background: Chronic obstructive pulmonary disease (COPD) is a heterogeneous group of lung conditions challenging to diagnose and treat. Identification of phenotypes of patients with lung function loss may allow early intervention and improve disease management. We characterised patients with the ‘fast decliner’ phenotype, determined its reproducibility and predicted lung function decline after COPD diagnosis. Methods: A prospective 4 years observational study that applies machine learning tools to identify COPD phenotypes among 13 260 patients from the UK Royal College of General Practitioners and Surveillance Centre database. The phenotypes were identified prior to diagnosis (training data set), and their reproducibility was assessed after COPD diagnosis (validation data set). Results: Three COPD phenotypes were identified, the most common of which was the ‘fast decliner’—characterised by patients of younger age with the lowest number of COPD exacerbations and better lung function—yet a fast decline in lung function with increasing number of exacerbations. The other two phenotypes were characterised by (a) patients with the highest prevalence of COPD severity and (b) patients of older age, mostly men and the highest prevalence of diabetes, cardiovascular comorbidities and hypertension. These phenotypes were reproduced in the validation data set with 80% accuracy. Gender, COPD severity and exacerbations were the most important risk factors for lung function decline in the most common phenotype. Conclusions: In this study, three COPD phenotypes were identified prior to patients being diagnosed with COPD. The reproducibility of those phenotypes in a blind data set following COPD diagnosis suggests their generalisability among different populations.
Dynamic routing occurs when customers are not known in advance, e.g. for real-time routing. Two heuristics are proposed that solve the balanced dynamic multiple travelling salesmen problem (BD-mTSP). These heuristics represent operational (tactical) tools for dynamic (online, real-time) routing. Several types and scopes of dynamics are proposed. Particular attention is given to sequential dynamics. The balanced dynamic closest vehicle heuristic (BD-CVH) and the balanced dynamic assignment vehicle heuristic (BD-AVH) are applied to this type of dynamics. The algorithms are applied to a wide range of test instances. Taxi services and palette transfers in warehouses demonstrate how to use the BD-mTSP algorithms in real-world scenarios. Continuous approximation models for the BD-mTSP’s are derived and serve as strategic tools for dynamic routing. The models express route lengths using vehicles, customers, and dynamic scopes without the need of running an algorithm. A machine learning approach was used to obtain regression models. The mean absolute percentage error of two of these models is below 3%.
In this paper we study the profitability of car manufacturers in relation to industry-wide profitability targets such as industry averages. Specifically we are interested in whether firms adjust their profitability in the direction of these targets, whether it is possible to detect any such change, and, if so, what the precise nature is of these changes. This paper introduces several novel methods to assess the trajectory of profitability over time. In doing so we make two contributions to the current body of knowledge regarding the dynamics of profitability. First, we develop a method to identify multiple profitability targets. We define these targets in addition to the commonly used industry average target. Second, we develop new methods to express movements in the profitability space from t to t + j, and define a notion of agreement between one movement and another. We use empirical data from the car industry to study the extent to which actual movements are in alignment with these targets. Here we calculate the three targets that we have previously identified, and contrast them with the actual profitability movements using our new agreement measure. We find that firms tend to move more towards to the new targets we have identified than to the common industry average. © 2012 Elsevier B.V. All rights reserved.
Decision making is often based on Bayesian networks. The building blocks for Bayesian networks are its conditional probability tables (CPTs). These tables are obtained by parameter estimation methods, or they are elicited from subject matter experts (SME). Some of these knowledge representations are insufficient approximations. Using knowledge fusion of cause and effect observations lead to better predictive decisions. We propose three new methods to generate CPTs, which even work when only soft evidence is provided. The first two are novel ways of mapping conditional expectations to the probability space. The third is a column extraction method, which obtains CPTs from nonlinear functions such as the multinomial logistic regression. Case studies on military effects and burnt forest desertification have demonstrated that so derived CPTs have highly reliable predictive power, including superiority over the CPTs obtained from SMEs. In this context, new quality measures for determining the goodness of a CPT and for comparing CPTs with each other have been introduced. The predictive power and enhanced reliability of decision making based on the novel CPT generation methods presented in this paper have been confirmed and validated within the context of the case studies.
Since the bus deregulation (Transport Act 1985) the patronage for bus services has been decreasing in a county in South of England. Hence, methods that increase patronage, focus subsidies and stimulate the bus industry are required. Our surveys and market research identified and quantified essential factors. The top three factors are price, frequency, and dependability. The model was further enhanced by taking into account real time passenger information (RTPI), socio-demographics and ticket machine data along targeted bus routes. These allowed the design of predictive models. Here, feature engineering was essential to boost the solution quality. We compared several models such as regression, decision tress and random forest. Additionally, traditional price elasticity formulas have been confirmed. Our results indicate that more accuracy can be gained using prediction methods based on the engineered features. This allows to identify routes that have the potential to increase in profitability - allowing a more focused subsidy strategy.
This book explores a set of critical areas of Operations Management in depth. The contents covers topics such as: - Waiting Line Models (e.g. Multiple Server Waiting Line) - Transportation and Network Models (e.g. Shortest Route) - Inventory Management (e.g. Economic Order Quantity model) This will enable the reader: - To increase the efficiency and productivity of business firms - To observe and define “challenges” in a concise, precise and logical manner - To be familiar with a selected number of classical and state-of-the art Management Science methods and tools to solve management problems - To create solution models, to develop and create procedures that offer competitive advantage to the business/organisation - To communicate and provide results to the management for decision making and implementation
This research examines the application of the Theory of Swift, Even Flow (TSEF) by a distribution company to improve the performance of its processes for parcels. TSEF was deployed by the company after experiencing improvement fatigue and diminishing returns from the time and effort invested. The fatigue was resolved through the deployment of swift, even flow and the adoption of "focused factories". The case study conducted semi-structured interviews, mapped the parcel processes and applied Discrete Event Simulation (DES). From this study we not only documented the value of TSEF as a strategic tool but we also developed insights into the challenges that the firm encountered when utilising the concept. DES confirmed the feasibility of change and its cost savings. This research demonstrates DES as tool for TSEF to stimulate management thinking about productivity
Drone delivery services (DDS) are an upcoming reality. Companies such as Amazon, DHL and Google are investing in developments in this area. German’s parcel delivery company DHL has begun applying them commercially. This emphasises the importance to have insights in the economic benefits of drone delivery services. This study compares drone delivery services with traditional delivery services. It looks at the cost effectiveness of integrating drones into a delivery services’ operations. The study identifies and categorise factors that control the delivery operations. It develops a mathematical model that compares 3D flight paths of a fleet of drones with a fleet of 2D van routes. The developed method can be seen as an extension of the Vehicle Routing Problem (VRP). Efficiency savings of drone services of real world rural postcode sectors are analysed. The study limits itself to the use of small unmanned aerial vehicles (UAVs) with a low payload, which are GPS controlled and autonomous. The case study shows time, distance and cost savings when using drones rather than delivery vans. The model reveals efficiency factors to operate DDS. The study shows the economic necessity for delivering low weight goods via DDS. The primary methodological novelty of this study is a model that integrates factors relevant to drones into the VRP.
In the United Kingdom, local councils and housing associations provide social housing at secure, low-rent housing options to those most in need. Occasionally tenants have difficulties in paying their rent on time and fall into arrears. The lost revenue can cause financial burden and stress to tenants. An efficient arrear management scheme is to target those who are more at risk of falling into long-term arrears so that interventions can avoid lost revenue. In our research, a Long Short-Term Memory Network (LSTM) based time series prediction model is implemented to differentiate the high-risk tenants from temporary ones. The model measures the arrear risk for each individual tenant and differentiates between short-term and long-term arrears risk. Furthermore it predicts the trajectory of arrears for each individual tenant. The arrears analysis investigates factors that provide assistance to tenants to trigger preventions before their debt becomes unmanageable. A five-years rent arrears dataset is used to train and evaluate the proposed model. The root mean squared error (RMSE) punishes large errors by measuring differences between actually observed and predicted arrears. The novel model benefits the sector by allowing a decrease in lost revenue; an increase in efficiency; and protects tenants from unmanageable debt.
Background: Chronic Obstructive Pulmonary Disease (COPD) is a heterogeneous group of lung conditions that are challenging to diagnose and treat. As the presence of comorbidities often exacerbates this scenario, the characterization of patients with COPD and cardiovascular comorbidities may allow early intervention and improve disease management and care. Methods: We analysed a 4-year observational cohort of 6883 UK patients who were ultimately diagnosed with COPD and at least one cardiovascular comorbidity. The cohort was extracted from the UK Royal College of General Practitioners and Surveillance Centre database. The COPD phenotypes were identified prior to diagnosis and their reproducibility was assessed following COPD diagnosis. We then developed four classifiers for predicting cardiovascular comorbidities. Results: Three subtypes of the COPD cardiovascular phenotype were identified prior to diagnosis. Phenotype A was characterised by a higher prevalence of severe COPD, emphysema, hypertension. Phenotype B was char-acterised by a larger male majority, a lower prevalence of hypertension, the highest prevalence of the other cardiovascular comorbidities, and diabetes. Finally, phenotype C was characterised by universal hypertension, a higher prevalence of mild COPD and the low prevalence of COPD exacerbations. These phenotypes were reproduced after diagnosis with 92% accuracy. The random forest model was highly accurate for predicting hypertension while ruling out less prevalent comorbidities. Conclusions: This study identified three subtypes of the COPD cardiovascular phenotype that may generalize to other populations. Among the four models tested, the random forest classifier was the most accurate at predicting cardiovascular comorbidities in COPD patients with the cardiovascular phenotype.
The global financial crisis of 2007–2009 is widely regarded as the worst since the Great Depression and threatened the global financial system with a total collapse. This article distinguishes itself from the vast literature of bankruptcy, bank failure, and bank exit prediction models by introducing novel categorical parameters inspired by Switzerland's banking landscape. We evaluate data from 274 banks in Switzerland from 2007 to 2017 using generalized linear model logit and multinomial logit regressions and examine the determinants of corporate restructuring and financial distress. We complement our results with a robustness test via a Bayesian inference framework. We find that total assets and net interest margin affect bank exit and mergers and acquisitions, and that banks operating in the Zurich area have a higher likelihood of exiting and becoming takeover targets relative to banks operating in the Geneva area.
Lean and Swift-Even-Flow (SEF) operations are compared in the context of sorting facilities. Lean approaches tend to attack parts of their processes for improvement and waste reduction, sometimes overlooking the impact this will have on their overall pipeline. A SEF approach on the other hand is driven by a desire to reduce variations by enabling the practitioner to visualise himself as the material that flows through the system thus unearthing all the problems that occur in the process as a whole. This study integrates Discrete Event Simulations (DES) into the lean and SEF framework. A real world case study with high levels of variations is used to gain insights and to derive relevant simulation models. The models were used to find the optimal configuration of machines and labour such that the operational costs are minimised. It was found that DES and SEF have a common basis. Lean processes as well as SEF processes both converge to similar solutions. However, SEF arrives faster at a near optimum solution. DES is a valuable tool to model, support and implement the lean and SEF approach. The SEF approach is superior to lean processes in the initial phases of a business process optimisation. The primary novelty of this study is the usage of DES to compare the lean and SEF approach. This study presents a systematic approach of how DES and optimisation can be applied to lean and SEF operations.
Existing works in the supply chain complexity area have either focused on the overall behavior of multi-firm complex adaptive systems (CAS) or on listing specific tools and techniques that business units (BUs) can use to manage supply chain complexity, but without providing a thorough discussion about when and why they should be deployed. This research seeks to address this gap by developing a conceptually sound model, based on the literature, regarding how an individual BU should reduce versus absorb supply chain complexity. This research synthesizes the supply chain complexity and organizational design literature to present a conceptual model of how a BU should respond to supply chain complexity. We illustrate the model through a longitudinal case study analysis of a packaged foods manufacturer. Regardless of its type or origin, supply chain complexity can arise due to the strategic business requirements of the BU (strategic) or due to suboptimal business practices (dysfunctional complexity). Consistent with the proposed conceptual model, the illustrative case study showed that a firm must first distinguish between strategic and dysfunctional drivers prior to choosing an organizational response. Furthermore, it was found that efforts to address supply chain complexity can reveal other system weaknesses that lie dormant until the system is stressed. The case study provides empirical support for the literature-derived conceptual model. Nevertheless, any findings derived from a single, in-depth case study require further research to produce generalizable results. The conceptual model presented here provides a more granular view of supply chain complexity, and how an individual BU should respond, than what can be found in the existing literature. The model recognizes that an individual BU can simultaneously face both strategic and dysfunctional complexity drivers, each requiring a different organizational response. We are aware of no other research works that have synthesized the supply chain complexity and organizational design literature to present a conceptual model of how an individual business unit (BU) should respond to supply chain complexity. As such, this paper furthers our understanding of supply chain complexity effects and provides a basis for future research, as well as guidance for BUs facing complexity challenges.
The catchment area along a bus route is key in predicting bus journeys. In particular, the aggregated number of households within the catchment area are used in the prediction model. The model uses other factors, such as head-way, day-of-week and others. The focus of this study was to classify types of catchment areas and analyse the impact of varying their sizes on the quality of predicting the number of bus passengers. Machine Learning techniques: Random Forest, Neural Networks and C5.0 Decision Trees, were compared regarding solution quality of predictions. The study discusses the sensitivity of catchment area size variations. Bus routes in the county Surrey in the United Kingdom were used to test the quality of the methods. The findings show that the quality of predicting bus journeys depends on the size of the catchment area.
In this paper we study the optimality of production schedules in the food industry. Specifically we are interested whether stochastic economic lot scheduling based on aggregated forecasts outperforms other lot sizing approaches. Empirical data on the operation’s customer side such as product variety, demand and inventory is used. Hybrid demand profiles are split into make-to-order (MTO) and make-to-stock (MTS) time series. We find that the MTS demand aggregation stabilizes, minimizes change-overs, and optimizes manufacturing.
You can play against "Edi" a chess program using Matlab. It uses a greedy heuristic to find the "best" move.
Background: Theranostic approaches—the use of diagnostics for developing targeted therapies—are gaining popularity in the field of precision medicine. They are predominately used in cancer research, whereas there is little evidence of their use in respiratory medicine. This study aims to detect theranostic biomarkers associated with respiratory-treatment responses. This will advance theory and practice on the use of biomarkers in the diagnosis of respiratory diseases and contribute to developing targeted treatments. Methods: We performed a cross-sectional analysis on a sample of 13,102 adults from the UK household longitudinal study ‘Understanding Society’. We used recursive feature selection to identify 16 biomarkers associated with respiratory treatment responses. We then implemented several machine learning algorithms using the identified biomarkers as well as age, sex, body mass index, and lung function to predict treatment response. Results: Our analysis shows that subjects with increased levels of alkaline phosphatase, glycated haemoglobin, high-density lipoprotein cholesterol, c-reactive protein, triglycerides, hemoglobin, and Clauss fibrinogen are more likely to receive respiratory treatments, adjusting for age, sex, body mass index, and lung function. Conclusions: These findings offer a valuable blueprint on why and how the use of biomarkers as diagnostic tools can prove beneficial in guiding treatment management in respiratory diseases.
The core goal of this paper is to identify guidance on how the research community can better transition their research into payment card fraud detection towards a transformation away from the current unacceptable levels of payment card fraud. Payment card fraud is a serious and long-term threat to society (Ryman-Tubb and d’Avila Garcez, 2010) with an economic impact forecast to be $416bn in 2017 (see Appendix A).1 The proceeds of this fraud are known to finance terrorism, arms and drug crime. Until recently the patterns of fraud (fraud vectors) have slowly evolved and the criminals modus operandi (MO) has remained unsophisticated. Disruptive technologies such as smartphones, mobile payments, cloud computing and contactless payments have emerged almost simultaneously with large-scale data breaches. This has led to a growth in new fraud vectors, so that the existing methods for detection are becoming less effective. This in turn makes further research in this domain important. In this context, a timely survey of published methods for payment card fraud detection is presented with the focus on methods that use AI and machine learning. The purpose of the survey is to consistently benchmark payment card fraud detection methods for industry using transactional volumes in 2017. This benchmark will show that only eight methods have a practical performance to be deployed in industry despite the body of research. The key challenges in the application of artificial intelligence and machine learning to fraud detection are discerned. Future directions are discussed and it is suggested that a cognitive computing approach is a promising research direction while encouraging industry data philanthropy.
In this paper we introduce a research agenda to guide the development of the next generation of Discrete Event Simulation (DES) systems. Interfaces to digital twins are projected to go beyond physical representations to become blueprints for the actual “objects” and an active dashboard for their control. The role and importance of real-time interactive animations presented in an Extended Reality (XR) format will be explored. The need for using game engines, particularly their physics engines and AI within interactive simulated Extended Reality is expanded on. Importing and scanning real-world environments is assumed to become more efficient when using AR. Exporting to VR and AR is recommended to be a default feature. A technology framework for the next generation simulators is presented along with a proposed set of implementation guidelines. The need for more human centric technology approaches, nascent in Industry 4.0, are now central to the emerging Industry 5.0 paradigm; an agenda that is discussed in this research as part of a human in the loop future, supported by DES. The potential role of Explainable Artificial Intelligence is also explored along with an audit trail approach to provide a justification of complex and automated decision-making systems with relation to DES. A technology framework is proposed, which brings the above together and can serve as a guide for the next generation of holistic simulators for manufacturing.
As a first contribution the mTSP is solved using an exact method and two heuristics, where the number of nodes per route is balanced. The first heuristic uses a nearest node approach and the second assigns the closest vehicle (salesman). A comparison of heuristics with test-instances being in the Euclidean plane showed similar solution quality and runtime. On average, the nearest node solutions are approximately one percent better. The closest vehicle heuristic is especially important when the nodes (customers) are not known in advance, e.g. for online routing. Whilst the nearest node is preferable when one vehicle has to be used multiple times to service all customers. The second contribution is a closed form formula that describes the mTSP distance dependent on the number of vehicles and customers. Increasing the number of salesman results in an approximately linear distance growth for uniformly distributed nodes in a Euclidean grid plane. The distance growth is almost proportional to the square root of number of customers (nodes). These two insights are combined in a single formula. The minimum distance of a node to n uniformly distributed random (real and integer) points was derived and expressed as functional relationship dependent on the number of vehicles. This gives theoretical underpinnings and is in agreement with the distances found via the previous mTSP heuristics. Hence, this allows to compute all expected mTSP distances without the need of running the heuristics.