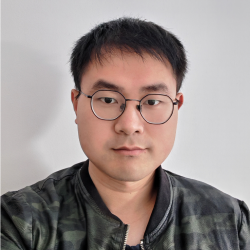
Dr Chen Tang
About
My research project
Computer scienceNatural Language Processing related to common sense inferences and text generation.
Supervisors
Natural Language Processing related to common sense inferences and text generation.
Publications
One of the key challenges of automatic story generation is how to generate a long narrative that can maintain fluency, relevance, and coherence. Despite recent progress, current story generation systems still face the challenge of how to effectively capture contextual and event features, which has a profound impact on a model's generation performance. To address these challenges, we present EtriCA, a novel neural generation model, which improves the relevance and coherence of the generated stories through residually mapping context features to event sequences with a cross-attention mechanism. Such a feature capturing mechanism allows our model to better exploit the logical relatedness between events when generating stories. Extensive experiments based on both automatic and human evaluations show that our model significantly outperforms state-of-the-art baselines, demonstrating the effectiveness of our model in leveraging context and event features.
Medical dialogue generation aims to generate responses according to a history of dialogue turns between doctors and patients. Unlike open-domain dialogue generation, this requires background knowledge specific to the medical domain. Existing generative frameworks for medical dialogue generation fall short of incorporating domain-specific knowledge, especially with regard to medical terminology. In this paper, we propose a novel framework to improve medical dialogue generation by considering features centered on domain-specific terminology. We leverage an attention mechanism to incorporate terminologically centred features, and fill in the semantic gap between medical background knowledge and common utterances by enforcing language models to learn terminology representations with an auxiliary terminology recognition task. Experimental results demonstrate the effectiveness of our approach, in which our proposed framework outperforms SOTA language models. Additionally, we provide a new dataset with medical terminology annotations to support the research on medical dialogue generation. Our dataset and code are available at https://github.com/tangg555/meddialog.
Story generation aims to generate a long narrative conditioned on a given input. In spite of the success of prior works with the application of pre-trained models, current neural models for Chinese stories still struggle to generate high-quality long text narratives. We hypothesise that this stems from ambiguity in syntactically parsing the Chinese language, which does not have explicit delimiters for word segmentation. Consequently, neural models suffer from the inefficient capturing of features in Chinese narratives. In this paper, we present a new generation framework that enhances the feature capturing mechanism by informing the generation model of dependencies between words and additionally augmenting the semantic representation learning through synonym denoising training. We conduct a range of experiments, and the results demonstrate that our framework outperforms the state-of-the-art Chinese generation models on all evaluation metrics, demonstrating the benefits of enhanced dependency and semantic representation learning.
To improve the performance of long text generation, recent studies have leveraged automatically planned event structures (i.e. storylines) to guide story generation. Such prior works mostly employ end-to-end neural generation models to predict event sequences for a story. However, such generation models struggle to guarantee the narrative coherence of separate events due to the hallucination problem, and additionally the generated event sequences are often hard to control due to the end-to-end nature of the models. To address these challenges, we propose NGEP, an novel event planning framework which generates an event sequence by performing inference on an automatically constructed event graph and enhances generalisation ability through a neural event advisor. We conduct a range of experiments on multiple criteria, and the results demonstrate that our graph-based neural framework outperforms the state-of-the-art (SOTA) event planning approaches, considering both the performance of event sequence generation and the effectiveness on the downstream task of story generation.
In recent years much effort has been devoted to applying neural models to the task of natural language generation. The challenge is to generate natural human-like text, and to control the generation process. This paper presents a task-agnostic survey of recent advances in neural text generation. These advances have been achieved by numerous developments, which we group under the following four headings: data construction, neural frameworks, training and inference strategies, and evaluation metrics. Finally we discuss the future directions for the development of neural text generation including neural pipelines and exploiting back-ground knowledge.