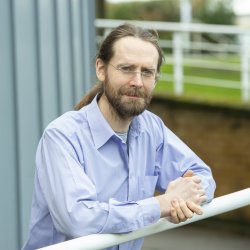
Dr Frank Guerin
Academic and research departments
Computer Science Research Centre, School of Computer Science and Electronic Engineering.About
Biography
Frank Guerin joined the Computer Science Department at Surrey in 2020. Before this he was Lecturer/Senior Lecturer at the University of Aberdeen and before that a PhD student in Imperial College.
His research interest is in Artificial Intelligence, where he has published in robotics, vision, language processing, and machine learning. He has established a track record of taking ideas from psychology research and importing them to Artificial Intelligence areas such as developmental robotics, and more recently mainstream robotics; for example learning spatial relationships autonomously, inspired by infant early means-ends behaviour, and applying the psychologist Barsalou's ideas to allow a robot system to use context effectively.
Guerin devoted significant time to learning about psychology, and engaging with Psychologists.
He initiated and was the main organiser for a 2013 Dagstuhl seminar bringing together leading international psychologists and roboticists.
His IEEE TAMD journal paper bringing developmental psychology ideas to the robotics community is his most cited paper.
He has been invited to speak at international meetings to provide a perspective that bridges the gap between psychology and robotics, e.g., OpenEASE Fall School 2019, Xperience Summer School 2013; workshops: ICRA 2013, ICDL-EpiRob 2014, RSS 2015, IROS 2015, FEEL project Paris 2014.
ResearchResearch interests
I am an AI researcher interested in the kinds of tasks that are easy for humans but hard for AI: in robotics, computer vision, and language. I have interest in psychology, and how humans do things, and I like to borrow ideas from human processes to implement them in AI systems that tackle real-world tasks.
I am interested in new approaches to knowledge representation and reasoning for AI systems which get over the rigidity and brittleness of classical approaches. Human knowledge of a concept such as "container" is very flexible to be applied to a wide range of objects (pots, cups, bags, boxes, rooms, buildings, ...) and applied in more abstract domains (political parties, controls on disease spread, damage from a scandal). The actions associated with container (insert, remove, escape, seal, breach, etc.) can also be adapted appropriately. These are not special or unusual or effortful applications of a concept for humans. Every human concept is effortlessly applied to a wide range of situations, and examples are everywhere in everyday cognition. It suggests that the human representation and reasoning machinery has a design which facilitates this.
I am looking for (non-classical) knowledge representation and reasoning which could allow AI systems to transfer knowledge of basic concepts in a human-like way. Vision example: give a system some knowledge of the types of tool (e.g. spatulas) that can lift pancakes or eggs from a pan, and enable it to transfer the concept to other objects which afford the same action. Manipulation example: give a system some knowledge of containers and container actions and enable it to apply this across a variety of scenarios. Language processing example: in understanding, given knowledge of concepts such as container and associated actions, to be able to recognise it in varied instantiations, e.g. where not literally used.
Paper about Projection idea in Artificial Intelligence https://arxiv.org/abs/2103.13512
Paper about task-driven representation in robotics Robot Manipulation in Open Environments
Highlighted Research
Research interests
I am an AI researcher interested in the kinds of tasks that are easy for humans but hard for AI: in robotics, computer vision, and language. I have interest in psychology, and how humans do things, and I like to borrow ideas from human processes to implement them in AI systems that tackle real-world tasks.
I am interested in new approaches to knowledge representation and reasoning for AI systems which get over the rigidity and brittleness of classical approaches. Human knowledge of a concept such as "container" is very flexible to be applied to a wide range of objects (pots, cups, bags, boxes, rooms, buildings, ...) and applied in more abstract domains (political parties, controls on disease spread, damage from a scandal). The actions associated with container (insert, remove, escape, seal, breach, etc.) can also be adapted appropriately. These are not special or unusual or effortful applications of a concept for humans. Every human concept is effortlessly applied to a wide range of situations, and examples are everywhere in everyday cognition. It suggests that the human representation and reasoning machinery has a design which facilitates this.
I am looking for (non-classical) knowledge representation and reasoning which could allow AI systems to transfer knowledge of basic concepts in a human-like way. Vision example: give a system some knowledge of the types of tool (e.g. spatulas) that can lift pancakes or eggs from a pan, and enable it to transfer the concept to other objects which afford the same action. Manipulation example: give a system some knowledge of containers and container actions and enable it to apply this across a variety of scenarios. Language processing example: in understanding, given knowledge of concepts such as container and associated actions, to be able to recognise it in varied instantiations, e.g. where not literally used.
Paper about Projection idea in Artificial Intelligence https://arxiv.org/abs/2103.13512
Paper about task-driven representation in robotics Robot Manipulation in Open Environments
Highlighted Research
Teaching
BSc:
COM3013 COMPUTATIONAL INTELLIGENCE
(not currently teaching) COM3025 DEEP LEARNING AND ADVANCED AI
COM3001 FINAL YEAR PROJECT
MSc Data Science:
COMM002 MSC DISSERTATION
COMM062 COMPUTATIONAL INTELLIGENCE
COMM056 ALIGNING BUSINESS VALUE WITH RESEARCH AND DEVELOPMENT
Publications
Self-supervised learning (SSL) techniques have recently produced outstanding results in learning visual representations from unlabeled videos. However, despite the importance of motion in supervised learning techniques for action recognition, SSL methods often do not explicitly consider motion information in videos. To address this issue, we propose MOFO (MOtion FOcused), a novel SSL method for focusing representation learning on the motion area of a video for action recognition. MOFO automatically detects motion areas in videos and uses these to guide the self-supervision task. We use a masked autoencoder that randomly masks out a high proportion of the input sequence and forces a specified percentage of the inside of the motion area to be masked and the remainder from outside. We further incorporate motion information into the finetuning step to emphasise motion in the downstream task. We demonstrate that our motion-focused innovations can significantly boost the performance of the currently leading SSL method (VideoMAE) for action recognition. Our proposed approach significantly improves the performance of the current SSL method for action recognition, indicating the importance of explicitly encoding motion in SSL.
End-to-end training with Deep Neural Networks (DNN) is a currently popular method for metaphor identification. However, standard sequence tagging models do not explicitly take advantage of linguistic theories of metaphor identification. We experiment with two DNN models which are inspired by two human metaphor identification procedures. By testing on three public datasets, we find that our models achieve state-of-the-art performance in end-to-end metaphor identification.
A robot can feasibly be given knowledge of a set of tools for manipulation activities (e.g. hammer, knife, spatula). If the robot then operates outside a closed environment it is likely to face situations where the tool it knows is not available, but alternative unknown tools are present. We tackle the problem of finding the best substitute tool based solely on 3D vision data. Our approach has simple hand-coded models of known tools in terms of superquadrics and relationships among them. Our system attempts to fit these models to point clouds of unknown tools, producing a numeric value for how good a fit is. This value can be used to rate candidate substitutes. We explicitly control how closely each part of a tool must match our model, under direction from parameters of a target task. We allow bottom-up information from segmentation to dictate the sizes that should be considered for various parts of the tool. These ideas allow for a flexible matching so that tools may be superficially quite different, but similar in the way that matters. We evaluate our system's ratings relative to other approaches and relative to human performance in the same task. This is an approach to knowledge transfer, via a suitable representation and reasoning engine, and we discuss how this could be extended to transfer in planning.
Robots performing everyday tasks such as cooking in a kitchen need to be able to deal with variations in the household tools that may be available. Given a particular task and a set of tools available, the robot needs to be able to assess which would be the best tool for the task, and also where to grasp that tool and how to orient it. This requires an understanding of what is important in a tool for a given task, and how the grasping and orientation relate to performance in the task. A robot can learn this by trying out many examples. This learning can be faster if these trials are done in simulation using tool models acquired from the Web. We provide a semi-automatic pipeline to process 3D models from the Web, allowing us to train from many different tools and their uses in simulation. We represent a tool object and its grasp and orientation using 21 parameters which capture the shapes and sizes of principal parts and the relationships among them. We then learn a `task function' that maps this 21 parameter vector to a value describing how effective it is for a particular task. Our trained system can then process the unsegmented point cloud of a new tool and output a score and a way of using the tool for a particular task. We compare our approach with the closest one in the literature and show that we achieve significantly better results.
Metaphoric expressions are widespread in natural language, posing a significant challenge for various natural language processing tasks such as Machine Translation. Current word embedding based metaphor identification models cannot identify the exact metaphorical words within a sentence. In this paper, we propose an unsupervised learning method that identifies and interprets metaphors at word-level without any preprocessing, outperforming strong baselines in the metaphor identification task. Our model extends to interpret the identified metaphors, paraphrasing them into their literal counterparts, so that they can be better translated by machines. We evaluated this with two popular translation systems for English to Chinese, showing that our model improved the systems significantly.
We tackle the problem disentangling the latent space of an autoencoder in order to separate labelled attribute information from other characteristic information. This then allows us to change selected attributes while preserving other information. Our method, matrix subspace projection, is much simpler than previous approaches to latent space factorisation, for example not requiring multiple discriminators or a careful weighting among their loss functions. Furthermore our new model can be applied to autoencoders as a plugin, and works across diverse domains such as images or text. We demonstrate the utility of our method for attribute manipulation in autoencoders trained across varied domains, using both human evaluation and automated methods. The quality of generation of our new model (e.g. reconstruction, conditional generation) is highly competitive to a number of strong baselines.
We address the problem of executing tool-using manipulation skills in scenarios where the objects to be used may vary. We assume that point clouds of the tool and target object can be obtained, but no interpretation or further knowledge about these objects is provided. The system must interpret the point clouds and decide how to use the tool to complete a manipulation task with a target object; this means it must adjust motion trajectories appropriately to complete the task. We tackle three everyday manipulations: scraping material from a tool into a container, cutting, and scooping from a container. Our solution encodes these manipulation skills in a generic way, with parameters that can be filled in at run-time via queries to a robot perception module; the perception module abstracts the functional parts of the tool and extracts key parameters that are needed for the task. The approach is evaluated in simulation and with selected examples on a PR2 robot.
The emergence of ChatGPT has generated much speculation in the press about its potential to disrupt social and economic systems. Its astonishing language ability has aroused strong curiosity among scholars about its performance in different domains. There have been many studies evaluating the ability of ChatGPT and GPT-4 in different tasks and disciplines. However, a comprehensive review summarizing the collective assessment findings is lacking. The objective of this survey is to thoroughly analyze prior assessments of ChatGPT and GPT-4, focusing on its language and reasoning abilities, scientific knowledge, and ethical considerations. Furthermore, an examination of the existing evaluation methods is conducted, offering several recommendations for future research in evaluating large language models.
Nominal metaphors are frequently used in human language and have been shown to be effective in persuading, expressing emotion, and stimulating interest. This paper tackles the problem of Chinese Nominal Metaphor (NM) generation. We introduce a novel multitask framework, which jointly optimizes three tasks: NM identification, NM component identification, and NM generation. The metaphor identification module is able to perform a self-training procedure, which discovers novel metaphors from a large-scale unlabeled corpus for NM generation. The NM component identification module emphasizes components during training and conditions the generation on these NM components for more coherent results. To train the NM identification and component identification modules, we construct an annotated corpus consisting of 6.3k sentences that contain diverse metaphorical patterns. Automatic metrics show that our method can produce diverse metaphors with good readability, where 92% of them are novel metaphorical comparisons. Human evaluation shows our model significantly outperforms baselines on consistency and creativity.
End-to-end training with Deep Neural Networks (DNN) is a currently popular method for metaphor identification. However, standard sequence tagging models do not explicitly take advantage of linguistic theories of metaphor identification. We experiment with two DNN models which are inspired by two human metaphor identification procedures. By testing on three public datasets, we find that our models achieve state-of-the-art performance in end-to-end metaphor identification.
The ACL Anthology is an online repository that serves as a comprehensive collection of publications in the field of natural language processing (NLP) and computational linguistics (CL). This paper presents a tool called ``ACL Anthology Helper''. It automates the process of parsing and downloading papers along with their meta-information, which are then stored in a local MySQL database. This allows for efficient management of the local papers using a wide range of operations, including "where," "group," "order," and more. By providing over 20 operations, this tool significantly enhances the retrieval of literature based on specific conditions. Notably, this tool has been successfully utilised in writing a survey paper (Tang et al.,2022a). By introducing the ACL Anthology Helper, we aim to enhance researchers' ability to effectively access and organise literature from the ACL Anthology. This tool offers a convenient solution for researchers seeking to explore the ACL Anthology's vast collection of publications while allowing for more targeted and efficient literature retrieval.
Metaphorical expressions are difficult linguistic phenomena, challenging diverse Natural Language Processing tasks. Previous works showed that paraphrasing a metaphor as its literal counterpart can help machines better process metaphors on downstream tasks. In this paper, we interpret metaphors with BERT and WordNet hypernyms and synonyms in an unsupervised manner, showing that our method significantly outperforms the state-of-the-art baseline. We also demonstrate that our method can help a machine translation system improve its accuracy in translating English metaphors to 8 target languages.
Large language models (LLMs) achieved remarkable performance across various tasks. However, they face challenges in managing long documents and extended conversations, due to significantly increased computational requirements, both in memory and inference time, and potential context truncation when the input exceeds the LLM's fixed context length. This paper proposes a method called Selective Context that enhances the inference efficiency of LLMs by identifying and pruning redundancy in the input context to make the input more compact. We test our approach using common data sources requiring long context processing: arXiv papers, news articles, and long conversations, on tasks of summarisation, question answering, and response generation. Experimental results show that Selective Context significantly reduces memory cost and decreases generation latency while maintaining comparable performance compared to that achieved when full context is used. Specifically, we achieve a 50\% reduction in context cost, resulting in a 36\% reduction in inference memory usage and a 32\% reduction in inference time, while observing only a minor drop of .023 in BERTscore and .038 in faithfulness on four downstream applications, indicating that our method strikes a good balance between efficiency and performance.
Medical dialogue generation aims to generate responses according to a history of dialogue turns between doctors and patients. Unlike open-domain dialogue generation, this requires background knowledge specific to the medical domain. Existing generative frameworks for medical dialogue generation fall short of incorporating domain-specific knowledge, especially with regard to medical terminology. In this paper, we propose a novel framework to improve medical dialogue generation by considering features centered on domain-specific terminology. We leverage an attention mechanism to incorporate terminologically centred features, and fill in the semantic gap between medical background knowledge and common utterances by enforcing language models to learn terminology representations with an auxiliary terminology recognition task. Experimental results demonstrate the effectiveness of our approach, in which our proposed framework outperforms SOTA language models. Additionally, we provide a new dataset with medical terminology annotations to support the research on medical dialogue generation. Our dataset and code are available at https://github.com/tangg555/meddialog.
Self-supervised learning (SSL) techniques have recently produced outstanding results in learning visual representations from unlabeled videos. Despite the importance of motion in supervised learning techniques for action recognition, SSL methods often do not explicitly consider motion information in videos. To address this issue, we propose MOFO (MOtion FOcused), a novel SSL method for focusing representation learning on the motion area of a video, for action recognition. MOFO automatically detects motion areas in videos and uses these to guide the self-supervision task. We use a masked autoencoder which randomly masks out a high proportion of the input sequence; we force a specified percentage of the inside of the motion area to be masked and the remainder from outside. We further incorporate motion information into the finetuning step to emphasise motion in the downstream task. We demonstrate that our motion-focused innovations can significantly boost the performance of the currently leading SSL method (VideoMAE) for action recognition. Our method improves the recent self-supervised Vision Transformer (ViT), VideoMAE, by achieving +2.6%, +2.1%, +1.3% accuracy on Epic-Kitchens verb, noun and action classification, respectively, and +4.7% accuracy on Something-Something V2 action classification. Our proposed approach significantly improves the performance of the current SSL method for action recognition, indicating the importance of explicitly encoding motion in SSL.
We tackle the problem of identifying metaphors in text, treated as a sequence tagging task. The pre-trained word embeddings GloVe, ELMo and BERT have individually shown good performance on sequential metaphor identification. These embeddings are generated by different models, training targets and corpora, thus encoding different semantic and syntactic information. We show that leveraging GloVe, ELMo and feature-based BERT based on a multi-channel CNN and a Bidirectional LSTM model can significantly outperform any single word embedding method and the combination of the two embeddings. Incorporating linguistic features into our model can further improve model performance, yielding state-of-the-art performance on three public metaphor datasets. We also provide in-depth analysis on the effectiveness of leveraging multiple word embeddings, including analysing the spatial distribution of different embedding methods for metaphors and literals, and showing how well the embeddings complement each other in different genres and parts of speech.
Video activity recognition by deep neural networks is impressive for many classes. However, it falls short of human performance, especially for challenging to discriminate activities. Humans differentiate these complex activities by recognising critical spatio-temporal relations among explicitly recognised objects and parts, for example, an object entering the aperture of a container. Deep neural networks can struggle to learn such critical relationships effectively. Therefore we propose a more human-like approach to activity recognition, which interprets a video in sequential temporal phases and extracts specific relationships among objects and hands in those phases. Random forest classifiers are learnt from these extracted relationships. We apply the method to a challenging subset of the something-something dataset and achieve a more robust performance against neural network baselines on challenging activities.
AACL 2022 Story generation aims to generate a long narrative conditioned on a given input. In spite of the success of prior works with the application of pre-trained models, current neural models for Chinese stories still struggle to generate high-quality long text narratives. We hypothesise that this stems from ambiguity in syntactically parsing the Chinese language, which does not have explicit delimiters for word segmentation. Consequently, neural models suffer from the inefficient capturing of features in Chinese narratives. In this paper, we present a new generation framework that enhances the feature capturing mechanism by informing the generation model of dependencies between words and additionally augmenting the semantic representation learning through synonym denoising training. We conduct a range of experiments, and the results demonstrate that our framework outperforms the state-of-the-art Chinese generation models on all evaluation metrics, demonstrating the benefits of enhanced dependency and semantic representation learning.
AACL 2022 To improve the performance of long text generation, recent studies have leveraged automatically planned event structures (i.e. storylines) to guide story generation. Such prior works mostly employ end-to-end neural generation models to predict event sequences for a story. However, such generation models struggle to guarantee the narrative coherence of separate events due to the hallucination problem, and additionally the generated event sequences are often hard to control due to the end-to-end nature of the models. To address these challenges, we propose NGEP, an novel event planning framework which generates an event sequence by performing inference on an automatically constructed event graph and enhances generalisation ability through a neural event advisor. We conduct a range of experiments on multiple criteria, and the results demonstrate that our graph-based neural framework outperforms the state-of-the-art (SOTA) event planning approaches, considering both the performance of event sequence generation and the effectiveness on the downstream task of story generation.
Incorporating external graph knowledge into neural chatbot models has been proven effective for enhancing dialogue generation. However, in conventional graph neural networks (GNNs), message passing on a graph is independent from text, resulting in the graph representation hidden space differing from that of the text. This training regime of existing models therefore leads to a semantic gap between graph knowledge and text. In this study, we propose a novel framework for knowledge graph enhanced dialogue generation. We dynamically construct a multi-hop knowledge graph with pseudo nodes to involve the language model in feature aggregation within the graph at all steps. To avoid the semantic biases caused by learning on vanilla subgraphs, the proposed framework applies hierarchical graph attention to aggregate graph features on pseudo nodes and then attains a global feature. Therefore, the framework can better utilise the heterogeneous features from both the post and external graph knowledge. Extensive experiments demonstrate that our framework outperforms state-of-the-art (SOTA) baselines on dialogue generation. Further analysis also shows that our representation learning framework can fill the semantic gap by coagulating representations of both text and graph knowledge. Moreover, the language model also learns how to better select knowledge triples for a more informative response via exploiting subgraph patterns within our feature aggregation process. Our code and resources are available at https://github.com/tangg555/SaBART.
EMNLP 2022 Findings One of the key challenges of automatic story generation is how to generate a long narrative that can maintain fluency, relevance, and coherence. Despite recent progress, current story generation systems still face the challenge of how to effectively capture contextual and event features, which has a profound impact on a model's generation performance. To address these challenges, we present EtriCA, a novel neural generation model, which improves the relevance and coherence of the generated stories through residually mapping context features to event sequences with a cross-attention mechanism. Such a feature capturing mechanism allows our model to better exploit the logical relatedness between events when generating stories. Extensive experiments based on both automatic and human evaluations show that our model significantly outperforms state-of-the-art baselines, demonstrating the effectiveness of our model in leveraging context and event features.
In recent years, considerable research has been dedicated to the application of neural models in the field of natural language generation (NLG). The primary objective is to generate text that is both linguistically natural and human-like, while also exerting control over the generation process. This paper offers a comprehensive and task-agnostic survey of the recent advancements in neural text generation. These advancements have been facilitated through a multitude of developments, which we categorize into four key areas: data construction, neural frameworks, training and inference strategies, and evaluation metrics. By examining these different aspects, we aim to provide a holistic overview of the progress made in the field. Furthermore, we explore the future directions for the advancement of neural text generation, which encompass the utilization of neural pipelines and the incorporation of background knowledge. These avenues present promising opportunities to further enhance the capabilities of NLG systems. Overall, this survey serves to consolidate the current state of the art in neural text generation and highlights potential avenues for future research and development in this dynamic field.
Artificial Intelligence systems cannot yet match human abilities to apply knowledge to situations that vary from what they have been programmed for, or trained for. In visual object recognition, methods of inference exploiting top-down information (from a model) have been shown to be effective for recognising entities in difficult conditions. Here a component of this type of inference, called 'projection', is shown to be a key mechanism to solve the problem of applying knowledge to varied or challenging situations, across a range of AI domains, such as vision, robotics, or language. Finally, the relevance of projection to tackling the commonsense knowledge problem is discussed.
There is at present no standard benchmarking for assessing and comparing the various existing works in developmental robotics. Developmental robotics is more of a “basic science” research endeavour than mainstream robotics, which is more application focussed. For this reason benchmarking for developmental robotics will need a more scientific basis, rather than a specific application focus. The solution we propose is to benchmark developmental robotics efforts against human infant capabilities at various ages. The proposal here may allow the community to showcase their efforts by demonstration on common tasks, and so to enable the comparison of approaches. It may also provide an agenda of incremental targets for research in the field.
The problem of performing everyday manipulation tasks robustly in open environments is currently beyond the capabilities of artificially intelligent robots; humans are required. The difficulty arises from the high variability in open environments; it is not feasible to program for, or train for, every variation. This correspondence paper presents the case for a new approach to the problem, based on three mutually dependent ideas: 1) highly transferable manipulation skills; 2) choice of representation: a scene can be modeled in several different ways; and 3) top-down processes by which the robot's task can influence the bottom-up processes interpreting a scene. The approach we advocate is supported by evidence from what we know about humans, and also the approach is implicitly taken by human designers in designing representations for robots. We present brief results of an implementation of these ideas in robot vision, and give some guidelines for how the key ideas can be implemented more generally in practical robot systems.
We propose a novel RoBERTa-based model, RoPPT, which introduces a target-oriented parse tree structure in metaphor detection. Compared to existing models, RoPPT focuses on semantically relevant information and achieves the state-of-the-art on several main metaphor datasets. We also compare our approach against several popular denoising and pruning methods, demonstrating the effectiveness of our approach in context denoising. Our code and dataset can be found at https://github.com/MajiBear000/RoPPT
In this paper, we propose FrameBERT, a RoBERTa-based model that can explicitly learn and incorporate FrameNet Embeddings for concept-level metaphor detection. FrameBERT not only achieves better or comparable performance to the state-of-the-art, but also is more explainable and interpretable compared to existing models, attributing to its ability of accounting for external knowledge of FrameNet.
Metaphors are proven to have stronger emotional impact than literal expressions. Although this conclusion is shown to be promising in benefiting various NLP applications, the reasons behind this phenomenon are not well studied. This paper conducts the first study in exploring how metaphors convey stronger emotion than their literal counterparts. We find that metaphors are generally more specific than literal expressions. The more specific property of metaphor can be one of the reasons for metaphors' superiority in emotion expression. When we compare metaphors with literal expressions with the same specificity level, the gap of emotion expressing ability between both reduces significantly. In addition, we observe specificity is crucial in literal language as well, as literal language can express stronger emotion by making it more specific.
Visual storytelling is a creative and challenging task, aiming to automatically generate a story-like description for a sequence of images. The descriptions generated by previous visual storytelling approaches lack coherence because they use word-level sequence generation methods and do not adequately consider sentence-level dependencies. To tackle this problem, we propose a novel hierarchical visual storytelling framework which separately models sentence-level and word-level semantics. We use the transformer-based BERT to obtain embeddings for sentences and words. We then employ a hierarchical LSTM network: the bottom LSTM receives as input the sentence vector representation from BERT, to learn the dependencies between the sentences corresponding to images, and the top LSTM is responsible for generating the corresponding word vector representations, taking input from the bottom LSTM. Experimental results demonstrate that our model outperforms most closely related baselines under automatic evaluation metrics BLEU and CIDEr, and also show the effectiveness of our method with human evaluation. (C) 2020 Elsevier Ltd. All rights reserved.
Robots acting in everyday environments need a good knowledge of how a manipulation action can affect pairs of objects in a relationship, such as "inside" or "behind" or "on top." These relationships afford certain means-end actions such as pulling a container to retrieve the contents, or pulling a tool to retrieve a desired object. We investigate how these relational affordances could be learned by a robot from its own action experience. A major challenge in this approach is to reduce the number of training samples needed to achieve accuracy, and hence we investigate an approach which can leverage past knowledge to accelerate current learning (which we call bootstrapping). We learn random forest-based affordance predictors from visual inputs and demonstrate two approaches to knowledge transfer for bootstrapping. In the first approach [direct bootstrapping (DB)], the state-space for a new affordance predictor is augmented with the output of previously learned affordances. In the second approach [category-based bootstrapping (CB)], we form categories that capture underlying commonalities of a pair of existing affordances and augment the state-space with this category classifier's output. In addition, we introduce a novel heuristic, which suggests how a large set of potential affordance categories can be pruned to leave only those categories which are most promising for bootstrapping future affordances. Our results show that both bootstrapping approaches outperform learning without bootstrapping. We also show that there is no significant difference in performance between DB and CB.