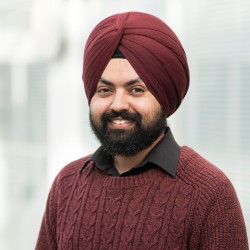
Dr Arshdeep Singh
Academic and research departments
Centre for Vision, Speech and Signal Processing (CVSSP), School of Computer Science and Electronic Engineering, Institute for Sustainability.About
Biography
Arshdeep Singh is employed as a Research Fellow A at the Centre for Vision Speech and Signal Processing (CVSSP) at the University of Surrey, working on the project “AI for Sound” funded through an Established Career Fellowship awarded by the Engineering and Physical Sciences Research Council (EPSRC) to Prof Mark Plumbley (Principal Investigator). He is also selected as a Sustainability Fellow at the Institute for Sustainability. In May 2023, Arshdeep has been selected as a Early career Acoustic champion for AI within EPSRC funded UK acoustic network, UKAN+.
Previously, Arshdeep has completed his PhD from IIT Mandi, India. His research focuses on designing machine learning frameworks for audio scene classification and compression of neural networks. During his PhD, he worked on sound-based health monitoring to identify the health of an industrial machine as a part of his internship work in Intel Bangalore. Earlier, he has completed his M.E from Panjab University, India and bagged a Gold medal. He has also worked as a Project fellow in CSIR-CSIO Chandigarh.
Areas of specialism
University roles and responsibilities
- Fire warden
Supervision
Postgraduate research supervision
MSc students:
(Co-supervisor, Primary Supervisor: Prof Mark D Plumbley)
Soham Bhattacharya, Dissertation title: Efficient Convolutional Neural Networks for Audio Classification,
Bars Szegedi, Dissertation title: Classification of Sounds Heard at Home using Convolutional neural networks.
Undergraduate (Y3) Students:
(Co-supervisor, Primary Supervisor: Prof Mark D Plumbley)
Kristaps Redmers, Dissertation Title: Visualising Soundscapes using Machine learning, website link: www.soundseek.pro/
Yan-Ping, Liao, Dissertation Title: Recognizing sound events in the home or workplace.
Teaching
Teaching assistanship (tutorials) in 2023 EEE3008 Fundamentals of Digital Signal Processing(DSP).
Publications
Highlights
For full list of publications, please visit the link https://sites.google.com/view/arshdeep-singh/home/publications?authuser=0
Convolutional neural networks (CNNs) are commonplace in high-performing solutions to many real-world problems, such as audio classification. CNNs have many parameters and filters, with some having a larger impact on the performance than others. This means that networks may contain many unnecessary filters, increasing a CNN's computation and memory requirements while providing limited performance benefits. To make CNNs more efficient, we propose a pruning framework that eliminates filters with the highest "commonality". We measure this commonality using the graph-theoretic concept of "centrality". We hypothesise that a filter with a high centrality should be eliminated as it represents commonality and can be replaced by other filters without affecting the performance of a network much. An experimental evaluation of the proposed framework is performed on acoustic scene classification and audio tagging. On the DCASE 2021 Task 1A baseline network, our proposed method reduces computations per inference by 71\% with 50\% fewer parameters at less than a two percentage point drop in accuracy compared to the original network. For large-scale CNNs such as PANNs designed for audio tagging, our method reduces 24\% computations per inference with 41\% fewer parameters at a slight improvement in performance.
Environmental sound classification (ESC) aims to automatically recognize audio recordings from the underlying environment, such as " urban park " or " city centre ". Most of the existing methods for ESC use hand-crafted time-frequency features such as log-mel spectrogram to represent audio recordings. However, the hand-crafted features rely on transformations that are defined beforehand and do not consider the variability in the environment due to differences in recording conditions or recording devices. To overcome this, we present an alternative representation framework by leveraging a pre-trained convolutional neural network, SoundNet, trained on a large-scale audio dataset to represent raw audio recordings. We observe that the representations obtained from the intermediate layers of SoundNet lie in low-dimensional subspace. However, the dimensionality of the low-dimensional subspace is not known. To address this, an automatic compact dictionary learning framework is utilized that gives the dimensionality of the underlying subspace. The low-dimensional embeddings are then aggregated in a late-fusion manner in the ensemble framework to incorporate hierarchical information learned at various intermediate layers of SoundNet. We perform experimental evaluation on publicly available DCASE 2017 and 2018 ASC datasets. The proposed ensemble framework improves performance between 1 and 4 percentage points compared to that of existing time-frequency representations
This paper presents a novel approach to make convolutional neural networks (CNNs) efficient by reducing their computational cost and memory footprint. Even though large-scale CNNs show state-of-the-art performance in many tasks, high computational costs and the requirement of a large memory footprint make them resource-hungry. Therefore, deploying large-scale CNNs on resource-constrained devices poses significant challenges. To address this challenge, we propose to use quaternion CNNs, where quaternion algebra enables the memory footprint to be reduced. Furthermore, we investigate methods to reduce the memory footprint and computational cost further through pruning the quaternion CNNs. Experimental evaluation of the audio tagging task involving the classification of 527 audio events from AudioSet shows that the quaternion algebra and pruning reduce memory footprint by 90% and computational cost by 70% compared to the original CNN model while maintaining similar performance.