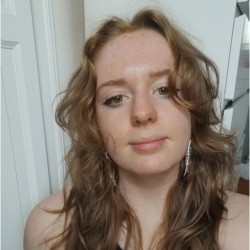
Anna Worthy
Academic and research departments
School of Mathematics and Physics, Soft Matter, Biological and Medical Physics Group, Nuclear applications.About
My research project
Development and use of new and derived data to facilitate the optimisation and evaluation of breast screening AI toolsI will be altering unprocessed mammograms to appear as though they were acquired at a lower dose, or at a different beam quality, to supplement and enrich training datasets comprised of clinical data from the OPTIMAM Mammographic Image Database (OMI-DB). In order to alter the images to appear as though acquired with a reduced dose, the electronic, quantum and structural noise is categorised and altered. Image processing is then applied to the simulated images to alter them to appear as if they are 'for presentation' images. The simulated data is added to the training dataset with the aim of improving the performance and generalisability of an in-house AI tool for breast cancer risk.
Supervisors
I will be altering unprocessed mammograms to appear as though they were acquired at a lower dose, or at a different beam quality, to supplement and enrich training datasets comprised of clinical data from the OPTIMAM Mammographic Image Database (OMI-DB). In order to alter the images to appear as though acquired with a reduced dose, the electronic, quantum and structural noise is categorised and altered. Image processing is then applied to the simulated images to alter them to appear as if they are 'for presentation' images. The simulated data is added to the training dataset with the aim of improving the performance and generalisability of an in-house AI tool for breast cancer risk.
Publications
Artificial intelligence has proven useful in the diagnosis of breast cancer from screening mammograms. This paper reports a computational method to correctly simulate training data compatible with the OMI-DB database; one of the largest databases of mammography images for medical research worldwide. Different mammography equipment has varying in-built quality that affects the noise and sharpness properties of an image. The simulation will alter an image to appear as if taken on a different detector, at a different dose or with a different image quality. A Python code has been developed to isolate the electronic, quantum and structural noise coefficients associated with digital mammography detectors for this purpose, building on previous work. A fit between noise power spectra and air kerma is used to find the noise coefficients, which are used with a random phase contribution to create noise images. These noise images are combined with flat-field signals to form simulated images. To simulate the results obtained at one dose from another, a dose factor is introduced to scale the noise contributions. Simulating a mammogram to appear as if taken under different conditions allows a more general training dataset to be created with minimal loss of biological information and without the ethical concerns of taking multiple images of a breast. A tailored dataset could be generated to facilitate an assessment of the performance of artificial intelligence tools for breast cancer detection or breast density calculations.