11am - 12 noon
Wednesday 21 May 2025
Sign Language Representation Learning in Low-Resource Settings: From Recognition to Translation
PhD Viva Open Presentation - Ryan Wong
Online event - All Welcome!
Free
University of Surrey
Guildford
Surrey
GU2 7XH
Sign Language Representation Learning in Low-Resource Settings: From Recognition to Translation
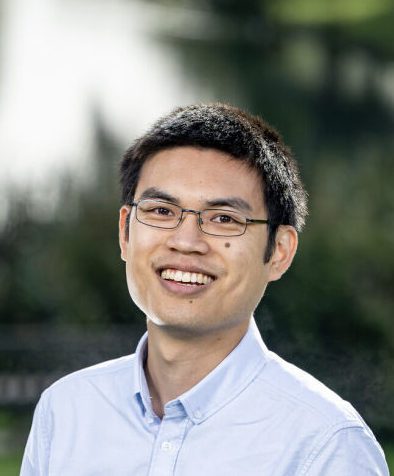
Abstract:
In recent years, the field of sign language recognition and translation for machine learning has gained increasing attention, with methods aimed at aiding communication between hearing and Deaf communities. However, the reliance on manually annotated labelled data remains a significant barrier, particularly in the context of sign language, where such data is often scarce and labour-intensive to produce. This thesis proposes novel methodologies aimed at learning sign representations, reducing the dependency on limited labelled datasets while enhancing sign spotting, recognition and translation systems. The first contribution chapter addresses domain adaptation from Isolated Sign Recognition (ISR) to sign spotting, focusing on identifying and localising signs in continuous sign language videos. While ISR models effectively recognise isolated signs, they lack the localisation precision required for sign spotting. To bridge this gap, we transfer knowledge from pretrained ISR models trained on larger sign datasets to the smaller sign spotting datasets. We introduce Hierarchical Sign I3D (HS-I3D), a hierarchical framework that refines spatio-temporal features through a multi-level temporal representation. Built atop the I3D backbone, HS-I3D enhances localisation accuracy across different layers, enabling more precise sign spotting.
Building on the previous chapter’s focus on precise localisation for sign spotting, we recognise that sign language understanding extends beyond identification, it also involves capturing linguistic semantics. This requires sign representations that encode both visual and linguistic aspects. The second contribution chapter, the Learnt Contrastive Concept (LCC) framework, introduces learnable sign embeddings, analogous to word embeddings in Natural Language Processing (NLP), to connect visual sign representations with their meanings. While existing approaches treat ISR as an extension to the visual gesture recognition task, our method integrates linguistic knowledge to address this limitation. This approach facilitates individual sign localisation without explicit localisation labels, aligns sign representations with their linguistic semantics and improves ISR performance.
The third contribution extends the previous chapter’s focus on localising isolated signs for ISR to the broader task of Sign Language Translation (SLT), which involves the translation of continuous sign videos into spoken language sentences. A major challenge in SLT is the reliance on manually annotated glosses to achieve strong SLT performance. To address this, we propose Sign2GPT, a gloss-free SLT framework which follows a two-stage process: first, we train a sign representation model using pseudo-gloss automatically extracted from spoken language sentences, eliminating the need for manual gloss annotations. Then, we integrate this pretrained model with a Generative Pretrained Transformers (GPT) language model to generate spoken language translations. This strategy not only removes reliance on gloss annotations but also leverages linguistic knowledge from large language models to enhance sign translation performance.
The final contribution addresses learning sign representations from large-scale unlabelled sign language datasets by proposing the SignRep Framework, a self-supervised approach using masked representation learning. An RGB-based model learns sign features with keypoint-based sign priors, eliminating the need for labelled data while producing a generalisable model for various sign tasks, such as finetuning for ISR or a feature extractor for dictionary retrieval and SLT. By utilising SignRep as a feature extractor in existing SLT models, we enhance translation performance while reducing computational costs during training. SignRep increases efficiency and scalability, supporting accessible sign language processing techniques and lowering resource barriers for future research and applications.