10am - 11am
Friday 7 February 2025
Machine Learning for Depression Detection and Emotion Recognition
PhD Viva Open Presentation - Andrew Bailey
Online event - All Welcome!
Free
Guildford
Surrey
GU2 7XH
This event has passed
Speakers
Machine Learning for Depression Detection and Emotion Recognition
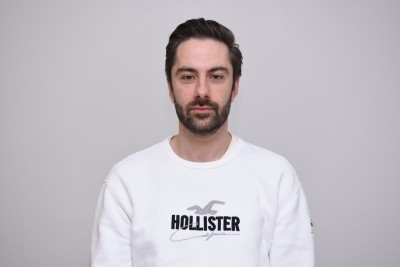
Abstract:
Mental health disorders, such as depression, bipolar, or post-traumatic stress disorder cause millions of people worldwide to suffer. Artificial intelligence has advanced significantly in many areas, including health care and social signal processing and could be used to help detect the early onset of mental health disorders or recognise emotion. This thesis will explore the issues of reproducibility in the domain of depression detection before exploring the classification power of artificial intelligence for depression detection using an audio-only approach. I will show that audio data can be used to detect depression and that performance gains can be obtained by using raw audio rather than hand-crafted features, such as mel spectrograms. When making decisions involving people, whether it be admissions to a university or a medical diagnosis, these decisions should be made fairly and without bias. This thesis will explore how fairness in the decision-making process of depression detection plays an important role in any future application. Specifically, I will show that the depression dataset that I use contains bias and the effects of this bias. I will rectify bias in the depression dataset by applying data balancing techniques. Also, I will show that my raw audio model is more resilient to data biases in the depression dataset than a model with hand-crafted features. This thesis will explore the broader topic of emotion recognition. Emotions are an integral part of human life, therefore, gaining a better understanding of categorising emotions may help in detecting mental health disorders; specifically, disorders such as depression can accentuate or suppress certain emotions. I utilise ideas from ensembling techniques to show that adapting existing architectures to result in individual emotion experts can improve emotion recognition performance. These experts can be fused together to give rise to a number of new architectures that provide an interesting parameter to performance trade-off. In parallel with this work, a systematic review (formal, unbiased, and repeatable literature review) on the use of AI for detecting depression will be explored. The systematic review will be conducted with a team from a local hospital. Our systematic review will give insight into the current state of medical literature in order to find gaps in knowledge and to propose areas of future research.