11am - 12 noon
Wednesday 22 January 2025
Multimodal Representation Learning and Image Interpretation with Applications in the Medical Domain
PhD Viva Open Presentation - Sergio Sanchez Santiesteban
Hybrid event - All Welcome!
Free
University of Surrey
Guildford
Surrey
GU2 7XH
This event has passed
Multimodal Representation Learning and Image Interpretation with Applications in the Medical Domain
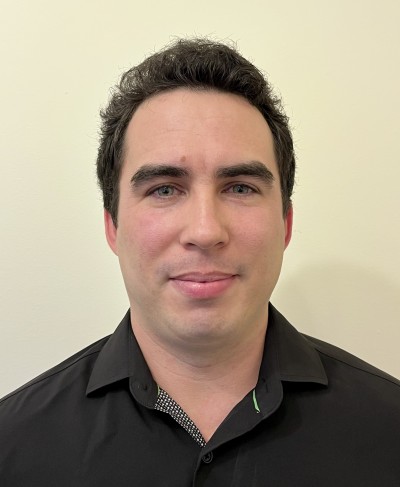
Abstract:
Recent advancements in artificial intelligence have led to the development of multimodal AI systems capable of integrating diverse types of data such as images, text, and structured knowledge. These systems offer significant potential across various domains, particularly in medicine, where complex and heterogeneous data types—ranging from histological images to genomic sequences and radiology reports—are critical for accurate diagnosis, prognosis, and treatment planning. However, current multimodal models face challenges in scalability, knowledge integration, and effective cross-modal alignment, particularly when applied to the intricacies of medical data. This thesis addresses these challenges by proposing novel methodologies for enhancing multimodal AI models through knowledge integration, self-supervised learning, and fine-grained alignment techniques.
First, we introduce a knowledge graph-augmented multimodal model that enables efficient and scalable access to external knowledge, reducing the need for ever-larger model architectures. This approach is demonstrated to outperform state-of-the-art models on vision-language tasks using a fraction of the training data and parameters.
Next, we adapt Self-Supervised Learning (SSL) techniques to the medical domain, specifically for the integration of histological images and genomic data in cancer prognosis. The Self supervised Histology-Genomic (SHG) model is introduced, leveraging specialized SSL tasks designed to capture complex relationships between phenotypic and genomic information. Empirical evaluations on multiple cancer datasets from The Cancer Genome Atlas (TCGA) show that the SHG model significantly improves survival prediction across five cancer types.
Finally, we focus on enhancing radiology report generation by developing a framework that improves the alignment between medical images and their corresponding textual reports. Our proposed methods introduce a region-specific Retrieval Augmented Generation (RAG) approach to enhance the generation of clinical reports by incorporating relevant retrieved information. Additionally, we integrate locally aligned phrase grounding annotations to ensure the generated content is more precise and contextually aligned with clinical data. Evaluation on large-scale public datasets demonstrate that the proposed framework produces more accurate and clinically relevant radiology reports.
Overall, this thesis contributes to the development of more efficient and scalable multimodal AI models, particularly for applications in the medical domain. By integrating external knowledge, adapting SSL to medical data, and improving image-text alignment, the proposed methodologies offer significant advancements in the capabilities of AI systems for medical diagnostics and decision-making.