11am - 12 noon
Friday 18 October 2024
Visual Content Provenance and Change Summarisation
PhD Viva Open Presentation - Alexander Black
Hybrid event - All Welcome!
Free
University of Surrey
Guildford
Surrey
GU2 7XH
This event has passed
Visual Content Provenance and Change Summarisation
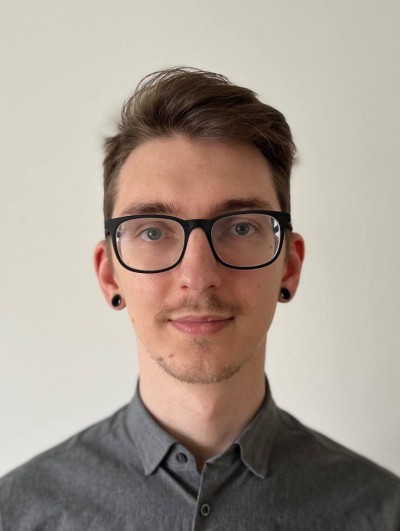
Abstract:
This thesis explores the problems of robust visual content provenance re-attribution and change summarisation, both visual and textual. Our first contribution is a novel scalable image provenance framework to match a query image back to a trusted database of originals and identify possible manipulations on the query. Our approach consists of three stages: scalable search stage; re-ranking and near-duplicate detection; and a manipulation detection and visualisation stage for localising regions within the query that may have been manipulated.
We extend our approach to videos and present VADER. VADER matches and coarsely aligns partial video fragments to candidate videos using a robust audio-visual descriptor and scalable search using an inverted index. A transformer-based alignment module then refines the temporal localisation of the query fragment within the matched video. A space-time comparator module identifies regions of manipulation between aligned content, invariant to any changes due to any residual temporal misalignments or artifacts arising from non-editorial changes of the content.
We also explore the problem of image difference captioning with text. Initially, we address the problem in the context of only two images with VIXEN – a technique that succinctly summarises in text the differences between two images in order to highlight any content manipulation present. Our proposed network linearly maps image features in a pairwise manner, constructing a soft prompt for a pretrained large language model. We show that VIXEN produces succinct, comprehensible difference captions for diverse image contents and edit types.
Finally, we extend our approach to a sequence of images in FVTC - a technique for image difference captioning that is able to benefit from additional visual and/or textual inputs. FVTC is able to succinctly summarise multiple manipulations that were applied to an image in a sequence. Optionally, it can take several intermediate thumbnails of the image editing sequence as input, as well as coarse machine-generated annotations of the individual manipulations. To train FVTC, we introduce METS - a new dataset of image editing sequences, with machine annotations of each editorial step and human edit summarisation captions.