2pm - 3pm
Monday 8 January 2024
Towards 3D VR sketch
PhD Viva Open Presentation by Ling Luo.
All welcome!
Free
This event has passed
Abstract
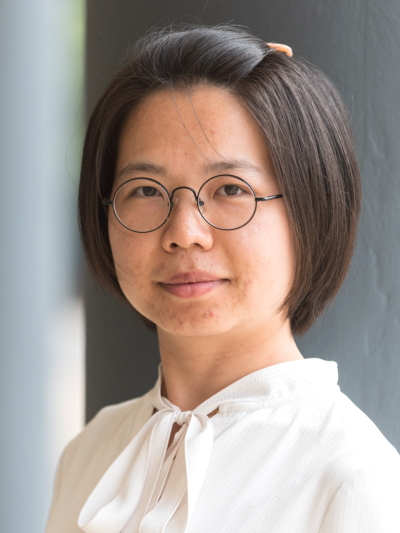
Have you ever tried 3D modelling from scratch? 3D content creation often requires specialized knowledge and training, posing a challenge for the average individual to quickly obtain 3D models that align with their creative ideas. Consequently, researchers have turned their attention to leveraging simple and easily accessible modalities such as text and images to control 3D model retrieval, generation, and editing. Currently, the most widely used modalities include text, photographs, and 2D sketches. However, text inherently lacks specificity when describing 3D structures, and 2D images can only capture the structure of a 3D object from a single viewpoint. In contrast, immersive VR/AR technologies are revolutionizing 3D content creation, surpassing the intuitiveness of text and 2D interactions, particularly in VR settings. As a result, we introduce an innovative modality for 3D content creation known as the 3D VR sketch.
In this thesis, our focus is on facilitating 3D shape modelling by employing 3D VR sketches as the input modality for both 3D shape retrieval and generation. We have designed a user-friendly VR sketching environment where sketches consist of sparse lines, eliminating the need for specific sketching skills, extensive training, or meticulous precision. Our overarching vision is to empower users to effortlessly retrieve or generate 3D models through this informal air-doodling approach within a VR setting.
Our study begins with the development of a VR interface to gather a dataset of VR sketches. To facilitate the training of retrieval methods, we introduce the first synthetic method for generating VR sketches from open shape datasets. We demonstrate that through training on synthetic sketches, we can achieve reasonable category-level shape retrieval accuracy for human VR sketches. Furthermore, we emphasize that in the context of 3D sketch to 3D shape retrieval, the point-cloud representation outperforms multi-view approaches. Additionally, despite our capacity to generate data with varying levels of abstractness, our experiments revealed a performance drop when using human sketches compared to synthetic sketches. This observation underscores the significance of designing retrieval techniques tailored specifically for human VR sketches.
Secondly, aligning with the recent trend in fine-grained data analysis within the sketch community, we introduce the first fine-grained 3D VR sketch dataset containing 1,497 pairs of 3D VR sketches and 3D shapes within the chair category, showcasing a wide diversity of shapes. Utilizing this dataset, we delve into fine-grained 3D shape retrieval based on 3D VR sketches. We demonstrate that 3D sketches can significantly enhance the accuracy of instance-level shape retrieval when compared to 2D sketches. By experimenting with carefully selected combinations of design factors on this new problem, we draw important conclusions to help follow-on work.
Furthermore, we enhance the structural similarity of retrieval results by establishing a novel connection between adaptive margin values and shape similarities. We noticed that previous retrieval results tend to closely align with input sketches but may not always match the ground truth due to potential sketch distortions. To address this issue, we propose the use of a triplet loss with an adaptive margin value determined by a 'fitting gap'. This fitting gap measures the similarity between two shapes under structure-preserving deformations. Additionally, we conduct a user study to validate the suitability of the fitting gap as a criterion for assessing the structural similarity of shapes.
Expanding on our accomplishments in fine-grained 3D shape retrieval, we extend our research to the domain of 3D shape generation. We propose a 3D shape generation network conditioned on 3D VR sketches and introduce a dedicated loss function that encourages the generated 3D shapes to faithfully match the input sketch. We meticulously crafted our approach, adopting a step-by-step model training strategy and utilizing multi-modal 3D shape representation to support training with limited data. To ensure the realism of the generated 3D shapes, we harness conditional normalizing flow, which models the distribution of the latent space of 3D shapes. Furthermore, we introduce a dedicated loss function that promotes the fidelity of the generated 3D shapes to an input sketch.
We aspire to provide an initial and substantiated exploration of this innovative modality, leveraging it to augment 3D modelling processes and laying a robust foundation for future research endeavours.