11am - 12 noon
Thursday 26 October 2023
Exploring sketch traits for democratising sketch based image retrieval
PhD Viva Open Presentation by Aneeshan Sain.
All welcome!
Free
University of Surrey
Guildford
Surrey
GU2 7XH
This event has passed
Abstract
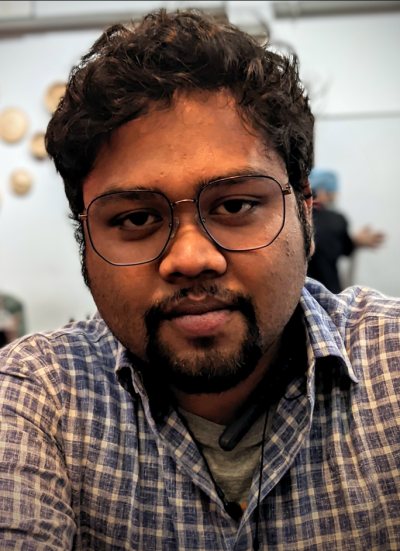
Sketches provide an efficient mode of abstracting the visual semantics of an object, by modelling its fine-grained details easily -- even better than texts which usually become longer and more complex in to describe those details. Naturally, with view of the commercial potential it holds, sketch has surfaced as an efficient query medium via the task of sketch based image retrieval (SBIR). Contrary to category-based image retrieval that fetches any image but of the same category as that of the query-sketch, research has shifted to the fine-grained setting (FG-SBIR) that involves retrieving the matching instance corresponding to the query-sketch out of a gallery of photos of the same category. With the existing scenario of touchscreen devices, the world is already ready to accept sketch as a query modality for various commercial applications. However, there are still a few setbacks leeching away at its potential. For instance, the general amateur population (like me!), sketches the same object in highly differing styles, which often proves detrimental for retrieval systems in identifying the target image or concept (e.g., a bird vs. an airplane). Furthermore, sketch-collection being quite a cumbersome process, existing sketches are extremely inadequate compared to the immense global scale of images present, thereby leading to shortage of training data holding sketch-photo association. Such issues prove to be a major bottleneck when pushing sketch research to real-world adoption in various fields of commerce, creativity or communication. This thesis therefore addresses these concerns from two perspectives putting forth five overall contributions. The first theme consists of two chapters that discuss certain traits specific to sketches that have been ignored till date, addressing which would boost accuracy of SBIR (FG) models. The second theme offers three chapters that cater towards the deployability of sketches for real-world applications, discussing the obstacles faced and accordingly certain acceptable solutions.
Walking on the first theme, our first chapter starts by addressing a trait particular to sketches that has been ignored till date -- they hold an inherent hierarchical structure within that relates to the extent of detailing imparted. Addressing this would ensure a stable retrieval accuracy irrespective of the extent of detail a user sketches to. We thus propose a paradigm that attends to a sketch-photo pair at different hierarchical levels of abstraction based on the extent of detail sketched. Accordingly, we devise a novel cross-modal co-attention based end-to-end trainable network that models this hierarchy as a learnable module for every sketch-photo pair.
Following the same theme, the second chapter explores yet another trait, very specific to amateur sketches at large -- being a result of unconstrained human subjectivity, they hold a unpredictably large diversity in the style they represent the same object. These multiple sketches representing the same object would confuse SBIR models unequipped to handle style diversity. We thus propose a paradigm that aims to disentangle the style component from the semantic one in a sketch and therefore use the latter for retrieval thus mitigating the ambiguity existing due to style-diversity for better retrieval accuracy. Specifically, we propose a novel meta-learning based variational auto-encoder network that learns to dynamically condition the encoder into extracting sketch-feature in a style-agnostic fashion.
Moving on to our second theme in the third chapter we address the problem of data scarcity in the field of fine-grained sketch-based image retrieval and how we can use the potential of unlabelled photos towards strengthening the FG-SBIR paradigm. In this regard, we put forward two contributions, (i) a knowledge-distillation paradigm that harnesses the knowledge of inter-instance distances between photo-features in an embedding space trained from unlabelled photos, and enriches the cross-modal embedding space of a FG-SBIR model. (ii) We challenge the standard triplet-loss based training paradigm of FG-SBIR and propose a stronger paradigm that ensures stable training as well as better performance of FG-SBIR models.
Keeping with the theme of extending SBIR to the scope real world scenarios, in the fourth chapter we focus on the setup of zero-shot (ZS) SBIR -- a field that primarily addresses the problem of data-scarcity. We go beyond the existing practice and identify the problem that every sketch being unique, existing models trained for ZS-SBIR by definition are not compatible with the inherent abstract and subjective nature of sketches -- the model might transfer well to new categories, but will not understand sketches existing in different test-time distribution as a result. We thus extend ZS-SBIR asking it to transfer to both categories and sketch distributions and propose a paradigm where the trained model would be adapted to every sketch during inference, thus reducing the distribution gap between train and test data. Accordingly, we propose a novel meta-learning based test-time training paradigm that adapts the model to query-sketch during inference using a self-supervised objective towards delivering a better and generalisable ZS-SBIR model.
The final chapter concludes the second theme by tailoring recent advances on foundation models and their unparalleled generalisation ability to benefit the sketch community. In particular, it leverages the foundation model CLIP for the task of zero-shot sketch based image retrieval (ZS-SBIR) and opens up an avenue of sketch-research towards dealing with data scarcity in various sketch applications. We therefore put forward novel designs on how best to achieve this synergy, for both the category setting as well as the fine-grained setting.
Accordingly, we propose a novel prompt-learning setup that adapts CLIP for the task of zero-shot SBIR. The take-home message, if any, is the proposed CLIP and prompt learning paradigm carries great promise in tackling other sketch-related tasks (not limited to ZS-SBIR) where data scarcity remains a great challenge.
Featured Academics
Visitor information
Find out how to get to the University, make your way around campus and see what you can do when you get here.