11am - 12 noon
Wednesday 11 January 2023
Pose estimation and novel view synthesis of humans
PhD Viva Open Presentation by Guillaume Rochette.
All Welcome!
Free
This event has passed
Abstract
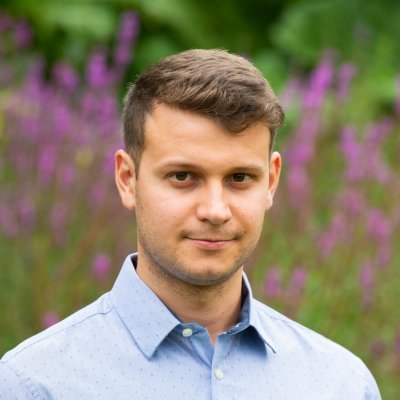
This thesis addresses pose estimation and novel view synthesis of humans. We evaluate our approaches on large-scale massively multi-view datasets, namely Panoptic Studio, Human3.6M and AIST Dance.
The first contribution presents a data-driven regularizer for weakly-supervised learning of 3D human pose estimation that eliminates the drift problem that affects existing approaches. We do this by moving the stereo reconstruction problem into the loss of the network itself. This avoids the need to reconstruct 3D data prior to training and unlike previous semi-supervised approaches, avoids the need for a warm-up period of supervised training. We evaluate our approach, on Panoptic Studio, in a comparative experiment and obtain an accuracy that is essentially indistinguishable from a strongly-supervised approach making full use of 3D groundtruth in training.
The second contribution presents an approach to synthesizing novel views of people in new poses. Our novel differentiable renderer enables the synthesis of highly realistic images from any viewpoint. Rather than operating over mesh-based structures, our renderer makes use of diffuse Gaussian primitives that directly represent the underlying skeletal structure of a human. Rendering these primitives gives results in a high-dimensional latent image, which is then transformed into an RGB image by a decoder network. We demonstrate the effectiveness of our approach to image reconstruction on both the Human3.6M and Panoptic Studio datasets. We show how our approach can be used for motion transfer between individuals, novel view synthesis of individuals captured from just a single camera, to synthesize individuals from any virtual viewpoint, and to re-render people in novel poses.
The third and final contribution presents an approach to estimating 3D human pose from sequence of images using polyhedral skeletal structures. Human pose is usually defined as a graph of lines symbolising the limbs, instead we represent the human skeleton as a graph composed of polyhedral sub-graphs. Moreoever, learning from videos rather than isolated images allows to exploit temporal cues to reduce jittery predictions as well as prevent models from predicting implausible poses, and it may also benefit downstream tasks such as image synthesis and enable synthesis of more temporally stable images. We show that polyhedral structures outperform linear structures, as such formulations reduces the ill-posed nature of the problem. We evaluate our approach on Human3.6M, but also on Panoptic Studio and AIST Database and obtain state-of-the-art results on these datasets.
Attend the event
This is a free online event open to everyone.