11am - 12 noon
Monday 14 November 2022
Deep Domain Adaptation for Visual Recognition with Unlabeled Data
PhD viva Open Presentation by Mr Zhongying Deng
All welcome!
Free
This event has passed
Speakers
Deep Domain Adaptation for Visual Recognition with Unlabeled Data
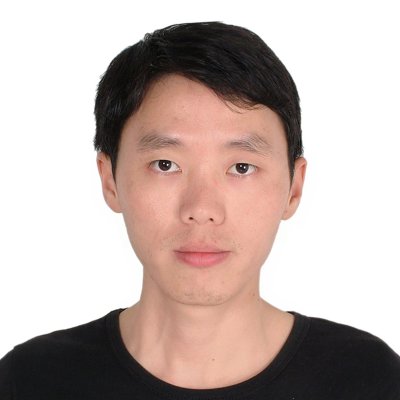
Abstract:
Visual recognition is the task of analyzing and understanding images or videos for objects, text, and other subjects. A remarkable success for visual recognition is achieved by deep convolutional neural networks (CNNs). The success of CNNs is usually built upon the independent and identically distributed (I.I.D) assumption that the training images follow the same distribution as that of test data. However, in real-world applications, we often need to apply a trained model to a new target environment where the test data follow a different distribution from the training data. This violates the I.I.D assumption, and thus often results in a significant performance drop of CNN models. A popular solution to tackling such distribution mismatch (also known as domain gap) issue is unsupervised domain adaptation (UDA).
UDA aims to transfer the knowledge learned from one or multiple labeled source domains to a target domain in which only unlabeled data are given for model adaptation. Most UDA studies address the challenge of domain gap by enforcing feature distribution alignment between different domains. However, when the number of source domains increases, aligning the feature distribution of a target domain to all the source domains can even harm the discriminative feature learning, thus counter-productive.
To tackle UDA, we make four contributions by proposing four novel methods from different perspectives: loss function, network architecture, data/feature augmentation and optimization/training strategy. First, we delve into how to better apply the domain alignment loss, considering that most UDA studies enforce the feature alignment loss across domains. To this end, the first contribution proposes a domain attention consistency loss to align the distributions of channel-wise attention weights in each pair of source-target domains for learning transferable latent attributes. Since the channel-wise attention is essentially a dynamic network, we then investigate the dynamic networks for learning domain-invariant features. To achieve this, the second contribution proposes a dynamic neural network, of which convolutional kernels are conditioned on each input instance, to adapt domain-invariant deep features to each individual instance.
In the above two contributions, we exploit pseudo-labels for the unlabeled target data and find them work well for UDA. But pseudo-labels inevitably contain noise. Therefore, in the third and fourth contributions, we focus on tackling the label noise in addition to reducing the domain gap. For the third contribution, we propose a distribution-based class-wise feature augmentation to generate intermediate features between different domains to bridge the domain gap, and meanwhile, leverage such feature augmentation to downplay noisy target labels for noise-robust training. For the fourth contribution, we propose a two-step training strategy for UDA to overcome the source-domain-bias in a UDA model, where the second step adopts a label noise-robust strategy to fine-tune such a UDA model on the pseudo-labeled target domain data only.
Extensive experiments on UDA datasets demonstrate the effectiveness of these four proposed methods, leading to a clear performance gain over the other state-of-the-art methods.
Attend the event
This is a free online event open to everyone. You can attend via Zoom.